High RPS11 level in hepatocellular carcinoma associates with poor prognosis after curative resection
Introduction
Hepatocellular carcinoma (HCC), which accounts for 70–85% of primary liver cancer, is an extremely malignant neoplasm with high mortality worldwide (1). Although early diagnosis and treatment of this disease has improved recently, the prognosis of HCC patients is still dismal. According to the Barcelona staging system, surgical resection remains the first-line therapy considering the good internal environment in early stage of HCC (2). Regretfully, the current detection rate of early HCC is less than 40% and most patients are in advanced stage of liver cancer at the time of diagnosis (3). These patients usually could not meet the indications of curative treatment like radical resection, liver transplantation or image-guided thermal ablation (4-6). Moreover, the frequent occurrence of metastasis and microvascular invasion is responsible for the poor prognosis of patients receiving curative resection. Therefore, it is imperative to discover novel candidate markers and drug targets for targeted therapy and prognosis prediction of HCC (7).
Eukaryotic ribosome is a major intracellular translation site mediating protein biosynthesis. It is composed of four ribosomal RNA (rRNAs) and 79 ribosomal proteins (RPs) (8). Over the decades, accumulative studies have shown that RPs not only serve as a structural protein in construction of ribosome, but also play important roles in cell cycle, proliferation, apoptosis/death, tumorigenesis, DNA repair, and anti-inflammatory reaction (9-11). It was reported that aberrant expression of RPs could result in cell transformation and was associated with poor prognosis and therapeutic resistance in some types of cancers including colon and pancreatic cancer (11-13). Elevated tumorous ribosomal protein S11 (RPS11) level has been found to be correlated to the terrible prognosis of glioblastoma patients (14). However, the expression status of RPS11 in HCC and whether RPS11 could predict prognosis for HCC patients have not been fully investigated.
In this study, immunohistochemistry (IHC) was performed in HCC tissues collected from 182 patients of the training cohort and 90 patients of the validation cohort to detect RPS11 expression level. The mRNA and protein level of RPS11 were also investigated in liver cell lines by Western blot and quantitative reverse transcription PCR (qRT-PCR). The relationship between RPS11 expression level and other clinical parameters in HCC patients was further analyzed. Additionally, we established two prognostic nomograms integrating RPS11 expression level and other significant prognostic variables to help achieve more accurate predictions of survival and recurrence for HCC patients after hepatectomy.
Methods
Patients enrollment
A total of 182 HCC patients who underwent curative resection from January 2009 to January 2010 in Zhongshan Hospital, Fudan University were recruited in this study as training cohort. Additionally, 90 HCC patients who underwent hepatectomy from June 2012 to July 2012 in Zhongshan Hospital were recruited as validation cohort. The detailed inclusion and exclusion criteria for HCC patients were shown as follows: (I) no systematic and local treatments before surgery, (II) without evidence of extra-hepatic metastasis before surgery, (III) a definite diagnosis of HCC for each individual, (IV) all of them received surgical resection, (V) no suffering from infection or severe inflammation except for viral hepatitis, (VI) and all cases’ clinical, pathological and follow-up information could be obtained. The Ethics Committee of Zhongshan Hospital, Fudan University approved this study, and written informed consents were signed by all enrolled patients.
Clinicopathologic parameters like age, gender, alpha-fetoprotein (AFP), carcinoembryonic antigen (CEA), tumor number, presence of hepatitis B virus (HBV) surface antigen (HBsAg), carbohydrate antigen 19-9 (CA19-9), liver cirrhosis, tumor size, ascites, microvascular invasion, tumor differentiation, alkaline phosphatase (ALP), alanine aminotransferase (ALT), tumor encapsulation, and aspartate aminotransferase (AST) were retrospectively collected. All laboratory indicators were tested before operation in 5 days. According to the staging systems of Barcelona Clinic Liver Cancer (BCLC) (6), and Tumor Node Metastasis (TNM) of AJCC 7th edition (15), we determined the clinical stages of HCC patients.
Follow-up
Blood routine, biochemical examination, tumor biomarkers, chest imaging examination and abdominal ultrasound were carried out after operation with intervals of 3 months in the first year, 3 to 6 months in the following 2 years, and once a year thereafter. Suspected recurrences were further confirmed by magnetic resonance imaging (MRI) or enhanced abdominal computed tomography (CT) scans. Overall survival (OS) was calculated as the time from surgery to the death of the patient or the last follow-up time (training cohort: June 2016; validation cohort: June 2017). The interval between the date of operation and recurrence was calculated as recurrence-free survival (RFS). For patients who had not recurred tumors, RFS was censored at the time of death or last follow-up.
Cell lines
Cell lines L02, SMMC-7721, SK-Hep1, Huh7, PLC/PRF/5 and Hep3B were purchased from the cell bank of the Chinese Academy of Sciences (Shanghai, China). MHCC97H and MHCCLM3 cell lines were established and got from Fudan Liver Cancer Institute of Zhongshan Hospital (Shanghai, China). With the atmosphere of 37 °C and 5% CO2, 10% fetal bovine serum (Invitrogen, USA) were mixed in Dulbecco’s modified Eagle’s medium (DMEM) (HyClone, USA) to culture the cells.
RNA extraction and quantitative reverse transcription PCR (qRT-PCR)
TRIzol reagent (Invitrogen, USA) was used to isolate RNA from corresponding eight cell lines. Then 1 µg of total RNA was reverse-transcribed by using PrimeScript RT reagent kit (Takara, Japan). Afterwards, we used qRT-PCR to measure the mRNA expression in an ABI Prism 7500 Sequence Detection system (Applied Biosystems, USA) using SYBR® Premix ExTaq™ (Takara, Japan). The designed primers sequences were as follows: RPS11-forward: 5'-TCACTGGTAATGTGTCCATTCG-3'; RPS11-reverse: 5'-CTTGCGGATGTAGTGCAGATAG-3'; ACTB-forward: 5'-GGACCTGACTGACTACCTCAT-3'; ACTB-reverse: 5'-CGTAGCACAGCTTCTCCTTAAT-3'. The data of our experiments were carried out in triplicate.
Western blot
Cell lines were harvested and then lysed in cell lysis solution using RIPA (Pierce Biotechnology, USA) combined with phenylmethylsulfonyl fluoride. The proteins were then mixed with a loading buffer and loaded to 10% SDS-PAGE pores with total 20 µg proteins per well. Separated proteins bands were then transferred to 0.45 µm PVDF membranes (Millipore, USA). Then 5% nonfat dry milk blocked the membranes. After washing, PVDF membranes were incubated with primary antibodies against RPS11 (1:1,000, Abcam, Cat No# ab175213) or Tubulin (1:5,000, Cell Signaling Technology, Cat No# 2125S) overnight in 4 °C fridge, followed by incubation with secondary antibody at room temperature for one hour. To make the bands with blotted proteins visible, we exposed the bands with enhanced chemiluminescence assay (Pierce Biotechnology, USA) to Tanon-5200 Chemiluminescent Imaging System (Tanon, China).
IHC
Briefly, sections fixed by paraffin were dewaxed in xylene and then hydrated by ethanol with decreasing concentration gradient. Endogenous catalases were inactivated by immersing the sections into 3% H2O2 solution at room temperature for 15 min. Then RPS11 antibody (1:100, Abcam, Cat No# ab175213) incubated on retrieved sections overnight at 4 °C, followed by horseradish peroxidase (HRP)-conjugated secondary antibody for 45 min at 37 °C. Subsequently, diaminobenzidine (DAB) solution (Dako REALTM EnVisionTM Detection System, Denmark) was used to incubate sections. Nuclei were stained with Harris’ Hematoxylin. The assessment of IHC staining was conducted by two independent pathologists who were blinded to the patients’ clinical data. The H-score method was used to evaluate the RPS11 IHC staining, which was to multiply the staining intensity (negative: 0, weak: 1, moderate: 2, strong: 3) by the staining extent (0–100%). We divided the samples into four groups based on the H-score: negative (0), weak (0–100%), moderate (100–150%), and strong (150–300%). Samples with the negative or weak H-score were distinguished as the low RPS11 expression group, while those with moderate or strong H-score were regarded as the high RPS11 expression group.
Data acquisition from The Cancer Genome Atlas (TCGA)
The mRNA expression (RNA-seq with Illumina HiSeq) of different tumor types was obtained from TCGA database (https://tcga-data.nci.nih.gov/tcga/). We analyzed RPS11 expression in tumor and/ or normal tissues of diverse tumors in TCGA. Box plots were applied to show the distributions of RPS11 expression levels, and Wilcoxon test was also used in this study. In addition, Gene Expression Profiling Interactive Analysis (GEPIA) (http://gepia.cancer-pku.cn/index.html) which is an online database including the TCGA HCC dataset was used to confirm the prognostic value of RPS11 and analyze the gene expression correlation (16). The correlation coefficient was determined by Pearson method.
Gene enrichment analysis
To find out the different gene sets between the high and low-risk group, single-sample gene-set enrichment analysis (ssGSEA, http://www.bioconductor.org) was carried out in our study (17). We achieved the most significantly different gene sets (P<0.001, |Fold change| ≥2) by applying the package of “GSVA” and method of ssGSEA for further study (18).
Statistical analysis
Statistical analyses were implemented by SPSS software (23.0; IBM, USA) and R (version 3.5.1). Student’s t-test and Mann-Whitney U test were used to analyze quantitative variables. Pearson Chi-squared test and Fisher’s exact test were used to analyze discrete variables. After plotting the survival curves of OS and RFS by Kaplan-Meier method, the prognostic difference between subgroups was analyzed using log-rank test. Then univariate Cox proportional hazards regression was conducted. For significant variables, we further performed multivariate Cox regression analysis in a stepwise manner. Data was expressed as mean ± standard derivation (SD).
Nomograms were established using the package of “rms” in R software (http://www.r-project.org/) according to the results of multivariate analysis. Then we used calibration curves, concordance index (C-index) and decision curve analysis (DCA) to evaluate the superiority of constructed nomograms. Hanley-McNeil test was conducted to compare the difference between C-indexes (19). A two-tailed P<0.05 was considered as statistically significant.
Results
Expression level and prognostic value of RPS11 in HCC
We first performed IHC staining to evaluate the expression of RPS11 in tumor tissues from 182 HCC patients who underwent curative resection. According to the H-scores, tumorous RPS11 levels were separated into four groups (Figure 1A). The survival analyses of the training cohort demonstrated that distinctly shorter OS and RFS were observed in patients with high RPS11 when compared to those with low RPS11 level (P<0.001; P<0.001, respectively) (Figure 1B,C). Similar results were observed in our validation cohort (Figure S1A,B). Additionally, we also investigated the mRNA and protein levels of RPS11 in HCC and non-transformed hepatic cell lines. As shown in Figure 1D,E, both mRNA and protein levels of RPS11 in most HCC cell lines (MHCC97H, MHCCLM3, SK-Hep1, PLC/PRF/5, Hep3B) were significantly higher than normal hepatic cell line (L02).
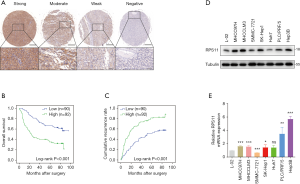
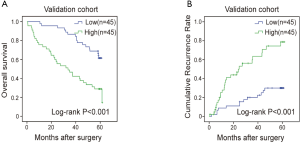
Besides, several cancers in the TCGA database, such as cholangiocarcinoma (CHOL), colon adenocarcinoma (COAD), kidney renal clear cell carcinoma (KIRC), kidney renal papillary cell carcinoma (KIRP), prostate adenocarcinoma (PRAD) and thyroid carcinoma (THCA) also exhibited a higher RPS11 expression level in tumor tissues when compared to normal tissues (Figure S2). Moreover, Kaplan-Meier survival analyses in TCGA HCC database showed that patients in the high RPS11 group experienced markedly worse OS and disease-free survival (DFS) durations than did those in the low RPS11 group (P=0.046 and P=0.0083, respectively) (Figure S3A,B).
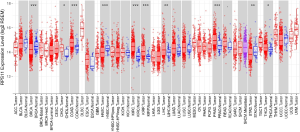
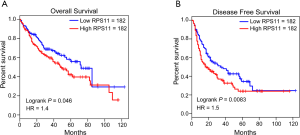
Association between clinicopathologic characteristics and RPS11 level
Patients were separated into two subgroups according to the IHC staining score (patients with strong and moderate IHC score were considered as RPS11-high group while those with weak and negative staining score as RPS11-low group). In the training cohort, we found that high RPS11 expression level was associated with elevated AFP level (P=0.021), CA19-9 level (P=0.002), and ALP level (P=0.003), as well as poor tumor differentiation (P=0.022) (Table 1). However, other clinicopathologic characteristics were not found to be statistically associated with RPS11 expression level. In our validation cohort, similar results were observed. As showed in Table S1, high RPS11 expression was positively correlated with high serum AFP level (P=0.010) and CA19-9 (P=0.021). Besides, we also found that high expression of RPS11 was markedly associated with the presence of microvascular invasion (P=0.018). All these data indicated that upregulated RPS11 expression in HCC tumor tissues may be related to aggressive tumor phenotypes.
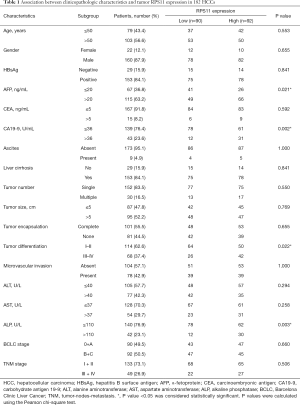
Full table
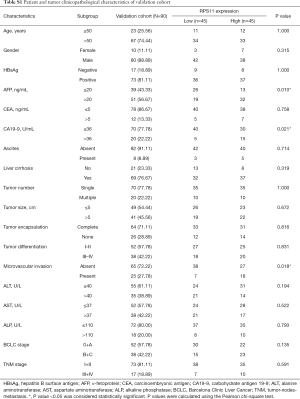
Full table
Univariate and multivariate analyses of RPS11 prognostic value
To further assess the prognostic value of RPS11 for HCC patients, we performed univariate and multivariate analyses in the training cohort. According to univariate analysis data shown in Table 2, AFP, CA19-9, tumor size, AST, TNM stage and RPS11 level were significantly associated with OS and RFS of HCC patients after hepatectomy. ALP, microvascular invasion and tumor differentiation were also found to keep a close relationship with OS of HCC patients. Additionally, the presence of ascites was related to RFS. Other indicators showed no significant association with the prognosis. Furthermore, multivariate Cox proportional hazards analysis was performed to analyze the significant indicators from univariate analysis. The results demonstrated that RPS11, as well as AFP, tumor size and TNM stage were considered as independent factors for predicting OS [hazard ratio (HR) =2.673, 95% confidence interval (CI): 1.751–4.081, P<0.001] and RFS (HR =2.127, 95% CI: 1.462–3.095, P<0.001) in HCC.
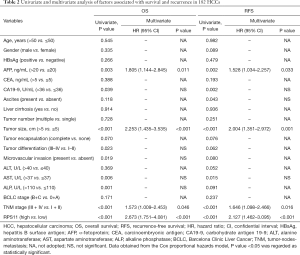
Full table
Nomograms for predicting OS and RFS of HCC patients
To develop a powerful predicting model for HCC patients, we constructed two nomograms for predicting OS and RFS after HCC resection by incorporating with RPS11 level and other identified vital independent factors (AFP, tumor size and TNM stage) selected from multivariate analyses in the training cohort (Figure 2A,B). For internal validation, calibrate curves were drawn to validate the accuracy of the nomogram models. It was shown that predictive values of RPS11-based nomograms were in well compactness with actual observations (Figure 2C,D,E,F). To further affirm the predictive accuracy, we assessed the created nomogram in the validation cohort. The calibration curves for OS and RFS prediction at 3, 5 years by the nomogram also fitted well with the ideal model (Figure S4A,B,C,D).
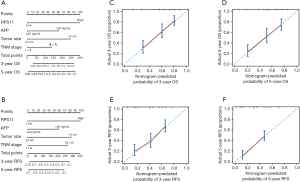
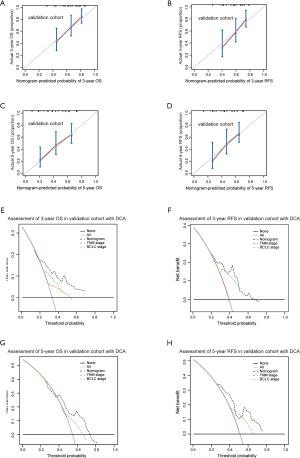
The superiority of RPS11-based nomograms for HCC patients
In order to further scrutinize the predictive accuracy of RPS11-based nomograms in HCC, the C-indexes of RPS11, TNM, TNM combined with RPS11, BCLC and BCLC combined with RPS11 for both OS and RFS were all taken into consideration. The results of the training cohort showed that RPS11-based nomogram showed superior predictive performance of OS (C-index =0.727) when compared to other conventional staging systems like TNM and BCLC (P<0.001, P<0.001, respectively). Additionally, in the prediction of RFS, RPS11-based nomogram also displayed better discriminatory capabilities than TNM and BCLC stage (P<0.001, P<0.001, respectively) (Table 3).
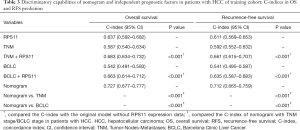
Full table
Moreover, DCA is usually employed to evaluate the clinical net benefit of prediction. The results of DCA in the training cohort revealed that RPS11-based nomograms could augment net benefits and exhibit a wider range of threshold probability in the prediction of 3- and 5-year OS and RFS (Figure 3A,B,C,D). Similar results were also observed in our validation cohort (Figure S4E,F,G,H).
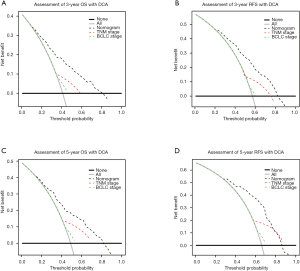
Identification of RPS11 associated biological pathways
To explore the potential biological pathways and involved molecular mechanisms, ssGSEA was performed in TCGA liver cancer database according to the mRNA expression of RPS11. As depicted in Figure 4, a panel of pathways pertinent to undifferentiated tumor, chemotherapy resistance, cancer recurrence, cancer metastasis, and tumor survival were enriched in high RPS11 expression group. Notably, several pathways related to molecules like MYC proto-oncogene (MYC), keratin 19 (KRT19), and epithelial cell adhesion molecule (EPCAM) were also gathered in patients with high RPS11 expression. In low RPS11 expression group, a set of pathways associated with the inhibition of vascular invasion and proliferation of liver cancer, as well as molecules like MET were observed. Given the association of RPS11 expression with significant pathways by ssGSEA, we next verified the correlations between the gene expression levels of molecules involved in significant pathways and RPS11 expression through GEPIA database. As listed in Figure S5A,B,C, RPS11 expression level has significant positive correlations with the expression levels of MYC (R=0.13, P=0.012), KRT19 (R=0.29, P=2.2e−08) and EPCAM (R=0.27, P=1.7e−07). MET was indicated to be negatively correlated with RPS11 expression (R=−0.15, P=0.0037) (Figure S5D). Recent research found that MET has the positive function in inhibiting HCC immune escape through downregulating PD-L1-mediated T cell inactivation (20). These results strongly suggested that RPS11 has the potential tumor-promoting role in HCC.
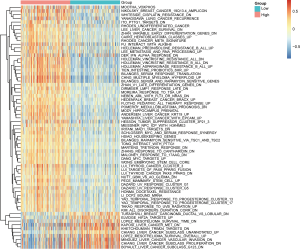
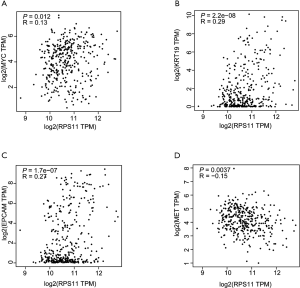
Discussion
As a component of 40S subunit of ribosome, RPS11 has been reported to play a vital role in RNA translation. RPS11 can regulate the synthesis of proteins involved in oncogene-induced senescence via ubiquitination pathway (21). In tumors including lung squamous-cell carcinoma and colon cancer, RPS11 was found to be constitutively overexpressed, which suggests a potential tumor-promoting role of RPS11 (22,23). In the present study, we found that patients with higher tumorous RPS11 expression were closely associated with poorer OS and RFS as compared to those with low RPS11 expression. Additionally, results of qRT-PCR and Western blot confirmed a higher mRNA and protein expression of RPS11 in most tumor cell lines. Results from multivariate Cox regression analyses further identified that RPS11 was indeed an independent indicator for predicting the prognosis of HCC. However, a relatively low expression of RPS11 was observed in breast cancer, suggesting that RPS11 may play different roles in diverse types of cancer (24).
Ferrari et al. reported that the mRNA level of RPS11 decreased rapidly in leukemic blast cells who induced to terminal differentiation (25). Our clinicopathologic analysis of the training cohort showed that high RPS11 expression was associated with poor tumor differentiation in “real-world” HCC patients. These observations suggested that RPS11 may help HCC cells remain dedifferentiated, which in turn promotes them to be more aggressive and resistant to treatment stress. Moreover, both in the training and validation cohort, high RPS11 expression levels were indicated to be positively correlated with other aggressive tumor phenotypes, such as high serum AFP level and CA19-9.
To further explore the potential tumor-promoting role of RPS11 in HCC, we then performed the gene enrichment analysis for RPS11 in TCGA liver cancer database. According to the results of ssGSEA, the enrichment of pathways related to cancer recurrence and metastasis, as well as drug resistance in RPS11-high group may partly explain the poor outcomes of HCC patients with elevated RPS11 level. The exact mechanism of RPS11 in promoting these biological processes in HCC is not clear, but evidence showed that some members of RPs, including RPS11, could bind to mouse double minute 2 homolog (MDM2) and activate p53 when under the nucleolar stress condition (14). The activation of MDM2/p53 signing pathway was reported to facilitate metastasis and drug resistance of cancer cells (26,27). Therefore, RPS11 may exert its oncogenic functions through MDM2/p53 pathway in HCC. Moreover, several key molecules in these enriched pathways may provide a foundation for a deeper understanding of mechanisms of RPS11. For example, EPCAM, a component of Wnt/β-catenin signaling pathway, could promote HCC growth and invasiveness (28,29) and could be pharmacologically targeted as a novel therapy for HCC (30). Another molecule KRT19 was also reported to facilitate the proliferation and metastasis of HCC (31). The oncogene MYC has been reported to extensively participate in multiple oncogenic pathways like Wnt/β-catenin signaling and ERK/c-Myc pathway (32,33). In addition, molecules like MET were enriched in low RPS11 expression group. A recent study has reported that MET could reduce the expression of PD-L1 through phosphorylation and deubiquitination of GSK3β, which is an immune checkpoint mediating immune evasion of liver cancer (20). Furthermore, according to the mRNA correlation analyses through GEPIA database, we found that RPS11 expression level was positively correlated with the expression levels of MYC, KRT19 and EPCAM, and negatively associated with MET expression levels. Hence, we hypothesized that RPS11 has the potential tumor-promoting role in HCC.
Recently, nomogram serves as a relatively effective model to predict prognosis in the field of oncology, with the ability of generating a probability of clinical events by using selective clinical indicators (34). Two nomograms, covering tumor RPS11 expression level, AFP, tumor size and TNM stage, were established in this study. The nomograms showed better predictive performance of OS and RFS in comparison with conventional staging systems for HCC patients in terms of C-index and clinical net benefit. Hence, these RPS11-based nomograms may be able to provide a more convenient and accurate prediction to HCC patients.
There are some limitations in our study. First, the limited number of enrolled patients and retrospective research design make it necessary to perform large-scale, prospective studies in the future. Second, although our results have set the groundwork for potential molecular mechanisms of RPS11, more supportive experiments are needed in the future to get a deep insight.
Conclusions
This is the first study that identifies RPS11 as an independent prognostic factor for HCC patients after curative resection. We also revealed that high tumorous RPS11 level was associated with poor OS and RFS. Our study further provided RPS11-based nomograms with improved predictive accuracy for HCC prognosis. Targeting RPS11 may be a potential effective strategy to prolong survival and inhibit tumor recurrence after surgical resection, which needs to be further investigated.
Acknowledgments
We thank the China Scholarship Council for supporting Dr. Chenhao Zhou as a visiting PhD candidate at The University of Texas MD Anderson Cancer Center.
Funding: This work was supported by grants from the Shanghai International Science and Technology Collaboration Program (No. 18410721900), the National Natural Science Foundation of China (No. 81472672), Program for Outstanding Academic Leader in Minhang District, Shanghai (No. 201709), and Shanghai Municipal Commission of Health and Family Planning (No. 201740238).
Footnote
Conflicts of Interest: All authors have completed the ICMJE uniform disclosure form (available at http://dx.doi.org/10.21037/atm.2020.03.92). The authors have no conflicts of interest to declare.
Ethical Statement: The authors are accountable for all aspects of the work in ensuring that questions related to the accuracy or integrity of any part of the work are appropriately investigated and resolved. This study was approved by the Ethics Committee of Zhongshan Hospital, Fudan University (No. 2018-155).
Open Access Statement: This is an Open Access article distributed in accordance with the Creative Commons Attribution-NonCommercial-NoDerivs 4.0 International License (CC BY-NC-ND 4.0), which permits the non-commercial replication and distribution of the article with the strict proviso that no changes or edits are made and the original work is properly cited (including links to both the formal publication through the relevant DOI and the license). See: https://creativecommons.org/licenses/by-nc-nd/4.0/.
References
- Marengo A, Rosso C, Bugianesi E. Liver Cancer: Connections with Obesity, Fatty Liver, and Cirrhosis. Annu Rev Med 2016;67:103-17. [Crossref] [PubMed]
- El-Serag HB, Marrero JA, Rudolph L, et al. Diagnosis and treatment of hepatocellular carcinoma. Gastroenterology 2008;134:1752-63. [Crossref] [PubMed]
- Li J, Han X, Yu X, et al. Clinical applications of liquid biopsy as prognostic and predictive biomarkers in hepatocellular carcinoma: circulating tumor cells and circulating tumor DNA. J Exp Clin Cancer Res 2018;37:213. [Crossref] [PubMed]
- Fu J, Wang H. Precision diagnosis and treatment of liver cancer in China. Cancer Lett 2018;412:283-8. [Crossref] [PubMed]
- Forner A, Reig M, Bruix J. Hepatocellular carcinoma. Lancet 2018;391:1301-14. [Crossref] [PubMed]
- Bruix J, Sherman M. Management of hepatocellular carcinoma: an update. Hepatology 2011;53:1020-2. [Crossref] [PubMed]
- Ye Q, Ling S, Zheng S, et al. Liquid biopsy in hepatocellular carcinoma: circulating tumor cells and circulating tumor DNA. Mol Cancer 2019;18:114. [Crossref] [PubMed]
- Zhou X, Liao WJ, Liao JM, et al. Ribosomal proteins: functions beyond the ribosome. J Mol Cell Biol 2015;7:92-104. [Crossref] [PubMed]
- Chen FW, Ioannou YA. Ribosomal proteins in cell proliferation and apoptosis. Int Rev Immunol 1999;18:429-48. [Crossref] [PubMed]
- Warner JR, McIntosh KB. How common are extraribosomal functions of ribosomal proteins? Mol Cell 2009;34:3-11. [Crossref] [PubMed]
- Yamamoto T. Roles of the ribosomal protein S19 dimer and the C5a receptor in pathophysiological functions of phagocytic leukocytes. Pathol Int 2007;57:1-11. [Crossref] [PubMed]
- Dong Z, Jiang H, Liang S, et al. Ribosomal Protein L15 is involved in Colon Carcinogenesis. Int J Med Sci 2019;16:1132-41. [Crossref] [PubMed]
- Yang J, Chen Z, Liu N, et al. Ribosomal protein L10 in mitochondria serves as a regulator for ROS level in pancreatic cancer cells. Redox Biol 2018;19:158-65. [Crossref] [PubMed]
- Yong WH, Shabihkhani M, Telesca D, et al. Ribosomal Proteins RPS11 and RPS20, Two Stress-Response Markers of Glioblastoma Stem Cells, Are Novel Predictors of Poor Prognosis in Glioblastoma Patients. PLoS One 2015;10:e0141334. [Crossref] [PubMed]
- Edge SB, Compton CC. The American Joint Committee on Cancer: the 7th edition of the AJCC cancer staging manual and the future of TNM. Ann Surg Oncol 2010;17:1471-4.
- Tang Z, Li C, Kang B, et al. GEPIA: a web server for cancer and normal gene expression profiling and interactive analyses. Nucleic Acids Res 2017;45:W98-102. [Crossref] [PubMed]
- Subramanian A, Tamayo P, Mootha VK, et al. Gene set enrichment analysis: a knowledge-based approach for interpreting genome-wide expression profiles. Proc Natl Acad Sci U S A 2005;102:15545-50. [Crossref] [PubMed]
- Hanzelmann S, Castelo R, Guinney J. GSVA: gene set variation analysis for microarray and RNA-seq data. BMC Bioinformatics 2013;14:7. [Crossref] [PubMed]
- Vickers AJ, Elkin EB. Decision curve analysis: a novel method for evaluating prediction models. Med Decis Making 2006;26:565-74. [Crossref] [PubMed]
- Li H, Li CW, Li X, et al. MET Inhibitors Promote Liver Tumor Evasion of the Immune Response by Stabilizing PDL1. Gastroenterology 2019;156:1849-1861.e13. [Crossref] [PubMed]
- Bengsch F, Tu Z, Tang HY, et al. Comprehensive analysis of the ubiquitinome during oncogene-induced senescence in human fibroblasts. Cell Cycle 2015;14:1540-7. [Crossref] [PubMed]
- Zhan C, Zhang Y, Ma J, et al. Identification of reference genes for qRT-PCR in human lung squamous-cell carcinoma by RNA-Seq. Acta Biochim Biophys Sin (Shanghai) 2014;46:330-7. [Crossref] [PubMed]
- Pogue-Geile K, Geiser JR, Shu M, et al. Ribosomal protein genes are overexpressed in colorectal cancer: isolation of a cDNA clone encoding the human S3 ribosomal protein. Mol Cell Biol 1991;11:3842-9. [Crossref] [PubMed]
- Nadano D, Aoki C, Yoshinaka T, et al. Electrophoretic characterization of ribosomal subunits and proteins in apoptosis: specific downregulation of S11 in staurosporine-treated human breast carcinoma cells. Biochemistry 2001;40:15184-93. [Crossref] [PubMed]
- Ferrari S, Manfredini R, Tagliafico E, et al. Noncoordinated expression of S6, S11, and S14 ribosomal protein genes in leukemic blast cells. Cancer Res 1990;50:5825-8. [PubMed]
- Tong H, Zhao K, Zhang J, et al. YB-1 modulates the drug resistance of glioma cells by activation of MDM2/p53 pathway. Drug Des Devel Ther 2019;13:317-26. [Crossref] [PubMed]
- Xing Y, Liu Y, Liu T, et al. TNFAIP8 promotes the proliferation and cisplatin chemoresistance of non-small cell lung cancer through MDM2/p53 pathway. Cell Commun Signal 2018;16:43. [Crossref] [PubMed]
- Terris B, Cavard C, Perret C. EpCAM, a new marker for cancer stem cells in hepatocellular carcinoma. J Hepatol 2010;52:280-1. [Crossref] [PubMed]
- Ji J, Tang J, Deng L, et al. LINC00152 promotes proliferation in hepatocellular carcinoma by targeting EpCAM via the mTOR signaling pathway. Oncotarget 2015;6:42813-24. [Crossref] [PubMed]
- Ogawa K, Tanaka S, Matsumura S, et al. EpCAM-targeted therapy for human hepatocellular carcinoma. Ann Surg Oncol 2014;21:1314-22. [Crossref] [PubMed]
- Tang J, Zhuo H, Zhang X, et al. A novel biomarker Linc00974 interacting with KRT19 promotes proliferation and metastasis in hepatocellular carcinoma. Cell Death Dis 2014;5:e1549. [Crossref] [PubMed]
- Zhang N, Chen X. A positive feedback loop involving the LINC00346/β-catenin/MYC axis promotes hepatocellular carcinoma development. Cell Oncol (Dordr) 2020;43:137-53. [Crossref] [PubMed]
- Chen Y, Lin C, Liu Y, et al. HMGB1 promotes HCC progression partly by downregulating p21 via ERK/c-Myc pathway and upregulating MMP-2. Tumour Biol 2016;37:4399-408. [Crossref] [PubMed]
- Balachandran VP, Gonen M, Smith JJ, et al. Nomograms in oncology: more than meets the eye. Lancet Oncol 2015;16:e173-80. [Crossref] [PubMed]