Nine hub genes related to the prognosis of HBV-positive hepatocellular carcinoma identified by protein interaction analysis
Introduction
Hepatocellular carcinoma (HCC) has dismal prognosis, remaining one of the leading causes of cancer-related deaths worldwide (1). Chronic persistent infection of hepatitis B virus (HBV) is the main risk factor associated with a sharp increase in the HCC rate (2). Recent extensive studies focused on the treatment of HBV-positive (HBV+) HCC have reported numerous biomarkers that are effective for the diagnosis of HBV infection and HBV+ HCC (3-5). Nevertheless, despite all the efforts HCC is still projected to become the third major cause of cancer-associated deaths by 2030 (6). Therefore, further elucidation of the mechanisms underlying the HBV oncogenic activity should aid in the development of effective therapies for HBV-associated HCC, which has become increasingly urgent.
Recent advances of microarray and sequencing technologies have made possible simultaneous detection of thousands of genes, which are deregulated at the transcriptional level in cancer and which play a pivotal role in carcinogenesis and disease progression (7). Joint analysis of gene expression profiles and clinical data allows identification of many potential prognostic biomarkers and drug targets. Although a number of gene expression profiling studies on HCC have been conducted in recent years (8), few of them distinguished between HBV-positive and HBV-negative HCC and explored the precise oncogenic mechanisms triggered by HBV. Moreover, these studies may show inconsistent results because of tissue or sample heterogeneity, and some of them lack detailed clinical information necessary to determine the clinical value of differentially expressed genes (DEGs). To date, integrative analysis combining high-throughput technologies and bioinformatics makes it possible to overcome some of these disadvantages. Thus, Halgand et al. (9) carried out comparative microarray and transcriptomics analyses of HBV+ HCC and non-tumor liver samples. However, functional annotation and exploration of key genes among the DEGs and comprehensive evaluation of their clinical value remain to be performed.
In the present work, we identified hub genes upregulated in HBV+ HCC compared with normal tissue and explored potential pathways in which these genes might be involved. Our results indicate that 9 key genes might be used as new prognostic biomarkers and treatment targets for HBV+ HCC, and 5 compounds might serve as novel drugs for HBV+ HCC therapy.
Methods
Microarray data
Gene expression profiles were extracted from the GSE47197 dataset, which was downloaded from the publicly available Gene Expression Omnibus (GEO) database. GSE47197 is based on the Agilent GPL16699 platform (Agilent-039494 SurePrint G3 Human GE v2 8x60K Microarray) and has been submitted by Halgand et al. (9); it contains 124 HBV-positive samples, including HCC and matched non-tumor liver tissues.
Screening of DEGs
To identify DEGs, transcriptional profiles of HCC and non-tumor tissues were compared using GEO2R (https://www.ncbi.nlm.nih.gov/geo/geo2r/), which is a freely available online tool enabling comparison of multiple sample groups after submission of a GEO series accession number (10). The DEG list was created based on the limma R package in GEO2R; P<0.05 and fold-change (FC) >2.0 were used as the cut-off criteria.
Gene ontology (GO) and Kyoto Encyclopedia of Genes and Genomes (KEGG) pathway enrichment analysis of DEGs
Multiple open online databases were used to annotate candidate DEGs and analyze their biological roles and pathway enrichment. Database for Annotation, Visualization, and Integrated Discovery (DAVID; https://david.ncifcrf.gov/) (11) was used to analyze molecular functions (MFs), biological processes (BPs), and cellular components (CCs); P<0.05 was set as the cut-off criterion. KEGG Orthology-Based Annotation System (KOBAS) v3.0 (http://kobas.cbi.pku.edu.cn) (12) was used for annotation and identification of the enriched pathways in the submitted gene set.
Construction of the protein-protein interaction (PPI) network and module analysis
Search Tool for the Retrieval of Interacting Genes (STRING; https://string-db.org/) is a freely available online database designed to explore functional interactions among proteins. STRING version 10.5, which includes 9,643,763 proteins from 2,031 organisms, was used to construct the PPI network to determine potential relationships among the 666 identified DEGs; medium confidence ≥0.4 was selected as the cut-off criterion. The obtained PPI network was visualized using the Cytoscape software; the Molecular Complex Detection (MCODE) plugin was applied to screen the top 2 modules based on the following parameters: degree cut-off =2, node score cut-off =0.2, K-Core =2, and maximum depth from seed =100. Pathway enrichment analysis of the DEGs in the modules was performed to explore the corresponding potential biological functions.
Hub gene identification and expression level validation
To determine the key genes among the DEGs, we used cytoHubba, a Cytoscape plugin enabling identification of the core genes in a biological network by ranking nodes with 11 different analysis methods (13). Among these methods, maximal clique centrality (MCC) showed greater accuracy in predicting essential proteins in the PPI network and was used to identify the key candidate genes among the DEGs, which might play vital roles in physiological regulatory functions. To confirm the precision of hub gene identification, mRNA expression levels of the selected genes were validated using the Chen Liver dataset in the Oncomine database (https://www.oncomine.org), an online interactive cancer database for analysis of DNA and RNA expression data (14,15), and GSE14520 and GSE121248 datasets of HBV+ HCC, HBV− HCC, and non-tumor samples.
Survival analysis and prognostic value assessment of the hub genes
To further explore the prognostic value of the hub genes confirmed to be differentially expressed in the Oncomine database and GSE14520 and GSE121248 datasets, Kaplan-Meier Plotter (http://kmplot.com/analysis/) was used. This database integrates published microarray datasets from GEO, European Genome-Phenome Archive (EGA), and The Cancer Genome Atlas (TCGA), enabling to assess the effect of genes on survival using samples of patients with cancer, including liver cancer. Hazard ratios (HRs) with 95% confidence intervals (CIs) and log rank P values were computed and plotted.
Patients
This retrospective study involved 77 patients with the pathological diagnosis of HCC who underwent surgery between 2011 and 2018 at the Fujian Medical University Union Hospital (Fuzhou, China); patients with alcoholic hepatitis and other types of hepatitis (such as caused by HCV) were excluded. Demographic and clinical characteristics of the 77 patients with HCC are shown in Table 1. The patients were followed up every six months, beginning at three months after operation until December, 2018. Tissue samples were taken from resected primary tumors and survival data were collected through telephone and the Social Security Death Index. This research was approved by the Ethics Committee of the Fujian Medical University Union Hospital.
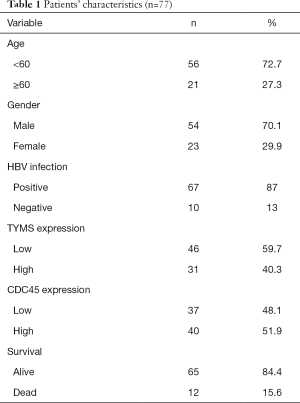
Full table
Immunohistochemistry (IHC)
The expression of thymidylate synthase (TYMS) and CDC45 proteins was analyzed in paraffin-embedded HCC tissues by IHC using rabbit polyclonal anti-human antibodies against TYMS and CDC45 (1:100 dilution; Proteintech, Chicago, IL, USA) as previously described (16). Each section was independently assessed by two pathologists blind to the patients’ clinical information and was assigned a mean score (0 to 3) based on staining intensity and the proportion of tumor cells demonstrating unequivocal positive reaction; scores 0 or 1 indicated low expression and 2 or 3—high expression.
Exploration of co-regulated expression patterns and gene set enrichment analysis (GSEA)
Co-regulated gene expression models contain two sets of genes: those with the same expression patterns and identical behavior (up- or downregulation) and those with different expression patterns and contrary behavior. To explore the co-regulated expression model of the hub genes, we used the “corrplot” R package. The KEGG pathways for each hub gene were determined using GSEA (http://www.broadinstitute.org/gsea/index.jsp), an efficient method to identify BPs regulated by highly expressed genes in a large gene set, which may be closely related to a disease phenotype (17). P<0.05 and the False Discovery Rate (FDR) <0.05 were used as the cut-off criteria.
Drug mining
Potential drugs for the treatment of HBV+ HCC were selected using the Connectivity Map (CMap) database (https://portals.broadinstitute.org/CMap/) containing numerous gene expression profiles from cultured cells treated with bioactive small-molecule compounds (18). By matching gene expression patterns of HCC and drug-treated cells, we determined whether they had the same (positive score) or opposite (negative score) gene expression signatures, which would identify drugs with a potential to cause or treat the disease, respectively. The selection criteria were P<0.001 and Mean >0.5.
Statistical analysis
Differences between groups were statistically analyzed with one-way ANOVA. The difference in TYMS and CDC45 expression between HBV+ HCC and HBV− HCC was determined using Chi-square test and the correlation between TYMS and CDC45 levels and patient survival was evaluated by the Kaplan-Meier estimator followed by log-rank test. P<0.05 was considered to indicate statistical significance.
Results
Overview of gene expression profiles and identification of DEGs
The overall workflow of data processing is presented in Figure 1A. Comparison of HBV+ HCC and non-tumor liver samples revealed 137 upregulated and 529 downregulated DEGs in HCC (Figure 1B); the top 50 upregulated and 50 downregulated DEGs are shown on a heat map (Figure 1C).
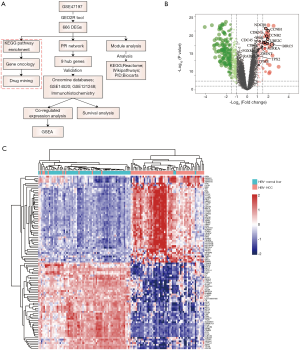
Functional annotation of DEGs by GO and KEGG pathway analyses
GO analysis of DEGs induced by HBV revealed that the upregulated DEGs were particularly enriched in such CCs as the nucleus, cytoplasm, and nucleoplasm, whereas at the MF level, they were enriched in protein binding, DNA binding, and ATP binding, and at the BP level—in cell division, mitotic nuclear division, and cell proliferation (Figure 2A).
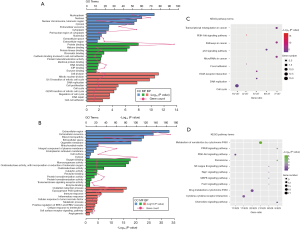
The downregulated DEGs were mainly associated with extracellular exosomes, the extracellular region, and the cytosol at the CC level, protein homodimerization activity, and receptor binding at the MF level, and oxidation-reduction process, immune response, and inflammatory response at the BP level (Figure 2B).
To further analyze the pathogenic mechanism of HBV, the identified DEGs were subjected to KEGG pathway analysis. The results showed that the upregulated genes were mainly associated with such KEGG terms as cell cycle, P53 signaling pathway, and extracellular matrix-receptor interaction (Figure 2C), whereas the downregulated genes were mostly enriched in metabolism pathways associated with P450 and pathways related to cancer (Figure 2D).
Identification of core modules and hub genes based on the PPI network
As the highest expression differences do not necessarily correlate with the biological significance of the gene, we analyzed the PPI network to discover core regulatory genes. Based on the STRING database, a total of 447 nodes and 3,535 protein pairs were found with medium confidence ≥0.4. The PPI network was visualized using Cytoscape and subjected to module analysis using MCODE; as a result, the top 2 modules were identified and protein interactions of DEGs from these modules were established (data not shown). Signaling pathway enrichment analysis showed that the first module was mostly related to the cell cycle, transcriptional regulation by p53, and FOXM1 transcription factor network (Table 2), whereas the second module was significantly enriched in platelet degranulation, response to elevated platelet cytosolic Ca2+, and platelet activation, signaling, and aggregation (Table 3). Using the MCC method, we identified top 15 hub genes, including CDK1, CCNB1, NDC80, TYMS, and AURKA (Table 4), which might play pivotal roles in the initiation and progression of HBV+ HCC, and constructed their interaction network using STRING. The results revealed the interactive relationship between the hub genes and the supporting sources of evidence, including experimental findings, databases, predicted interactions, and text mining (Figure 3A). Furthermore, there were differences in the expression of the hub genes between HBV-positive normal liver tissues and HCC (Figure 3B), suggesting that these genes may be involved in tumorigenesis and, thus, deserve further analysis.
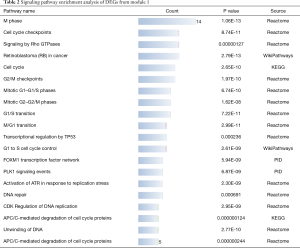
Full table
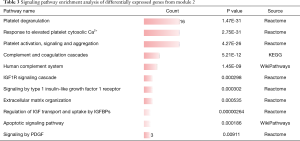
Full table
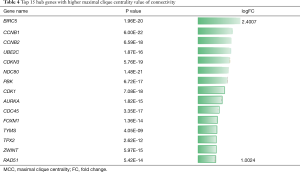
Full table
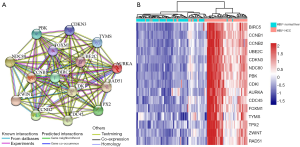
Expression validation and survival analysis of the identified hub genes
To further verify the reliability of the identified hub genes, we analyzed the data on HBV-positive HCC samples and non-tumor liver tissues from the Oncomine database and other HBV-related HCC datasets (GSE14520 and GSE121248). The results indicated that 10 upregulated hub genes were also significantly upregulated in all three databases (Figure 4A,B,C). We also assessed the prognostic potential of the hub genes based on Kaplan-Meier survival curves and found that high expression of CDK1, NDC80, TYMS, AURKA, FOXM1, CDC45, ZWINT, TPX2, and PBK was significantly associated with shorter overall survival of patients with HCC (Figure 4D).
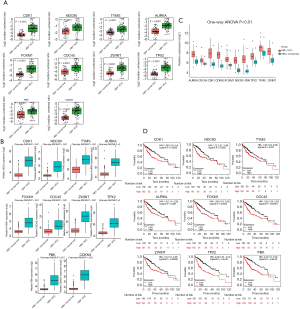
Association of TYMS and CDC45 protein expression with HBV+ HCC and patient survival
The results obtained from the analysis of public datasets were validated in 77 randomly selected HBV-positive and -negative HCC samples and normal liver tissues by IHC. The data indicated that the expression of TYMS and CDC45 proteins was higher in HBV+ HCC than in HBV− HCC (P<0.05 by Chi-square test) or normal liver tissue (P<0.05 by Chi-square test) (Figure 5A). Kaplan-Meier survival analysis showed that high TYMS and CDC45 expression was associated with poor patient survival (Figure 5B). These results are consistent with database analyses.
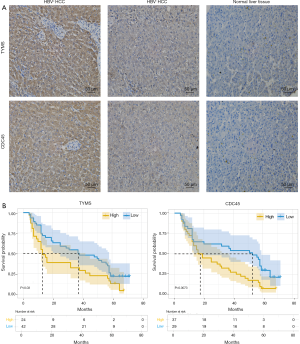
Exploration of co-expression pattern and GSEA
Co-expression models of the 9 hub genes were evaluated by correlation analysis, which revealed that all these genes had positive relationship with each other (Figure 6A), suggesting that they might be involved in the same signaling pathway regulating the development of HBV+ HCC. To verify this hypothesis, the KEGG pathways for the 9 genes were searched using GSEA. Consistent with the correlation analysis, the results indicated that these genes participated in eight signaling pathways (normalized P<0.05 and FDR <0.25; Figure 6B).
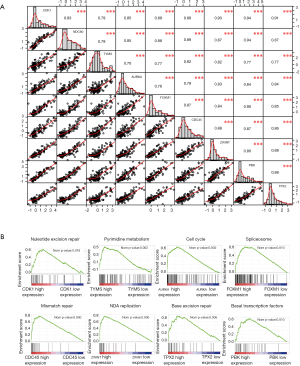
Mining potential drugs for patients with HBV+ HCC
In total, 16 small compounds that met the inclusion criteria were selected from the CMap database; among them, 11 and 5 had positive and negative scores, respectively (Table 5). The gene expression patterns induced by the compounds with a positive score, such as adiphenine and isoflupredone were similar to that of HBV+ HCC, indicating that these drugs should not be used to treat patients with HBV+ HCC. In contrast, drugs with inverse gene expression signatures (negative score) could have a therapeutic effect on HBV+ HCC; among them, apigenin and phenoxybenzamine had the highest negative score and enrichment value, indicating that they might have better therapeutic effects than the other compounds.
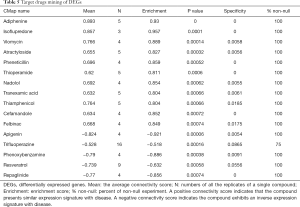
Full table
Discussion
Although many basic and clinical studies have explored the carcinogenic mechanism of HBV infection and treatment approaches for HBV-positive HCC in the past decades (19,20), most of them focused on a single gene or evaluated a single cohort. To improve the reliability of analytical results, in this work we integrated different cohort datasets from GEO and Oncomine databases. The 666 DEGs (137 upregulated and 529 downregulated) identified from the GSE47197 dataset were subjected to GO, KEGG pathway, and PPI analyses, and the top 2 modules and 15 hub genes were selected. Considering that the application of only KEGG pathway analysis may result in missing some critical pathways, the modules were then examined using multiple online databases (KEGG, Reactome, Wikipathways, PID, and Biocarta). The expression of the hub genes and their association with disease prognosis were further validated using several datasets (Oncomine, GSE14520, and GSE121248) and multiple cases collected in our hospital. The obtained data was used to identify 5 compounds that might serve as novel drugs for the treatment of HBV+ HCC.
It has been reported that the deregulation of the inflammatory response and cell cycle progression are the two main causes of HCC (21,22). By escaping host immune clearance, HBV can continuously replicate in patients, leading to chronic hepatitis, which creates a favorable environment for the initiation and development of cirrhosis and HCC. Moreover, HBV can also induce the proliferation of HCC cells by promoting the expression of cell cycle-associated proteins. Consistent with this notion, our GO analysis showed that the upregulated DEGs were significantly enriched in the BP of the cell cycle, whereas the downregulated DEGs were mainly enriched in the immune response and inflammatory response. In agreement with GO analysis, the results of KEGG analysis revealed that the upregulated DEGs were associated with the cell cycle pathway, P53 signaling pathway, and pathways in cancer, whereas the downregulated DEGs were involved in cytokine-cytokine receptor interaction, chemokine signaling pathway, and other inflammation-related pathways. Evaluation of DEGs from the first module in the PPI network further confirmed these results. However, analysis of DEGs in the second module showed that most of them were significantly enriched in platelet-associated signaling pathways, including platelet degranulation, platelet cytosolic Ca2+, and platelet activation. A meta-analysis study has revealed that an increased platelet to lymphocyte ratio indicates poor prognosis for patients with HCC (23) and growing evidence suggests that platelets are involved in cancer through crosstalk between tumor cells (24). Currently, there are no reports on the association of platelets with HBV+ HCC, which should be clarified in the future.
Validation of the identified hub genes using several HBV-related datasets revealed that 10 genes were significantly upregulated in HBV-positive HCC compared with normal liver tissue and survival analysis indicated that 9 of them, CDK1, NDC80, TYMS, AURKA, FOXM1, CDC45, ZWINT, PBK, and TPX2, were associated with poor prognosis for patients with HCC, proving their crucial role in carcinogenesis. The functional significance of some of these genes in HBV-induced HCC has been investigated in previous studies. Thus, Lei et al. (25) reported that HBV could enhance the activity of CDK1 and promote hepatocyte mitotic entry by interacting with C53, suggesting that CDK1 might play a pivotal role in HBV-induced HCC. Consistent with our findings, it was shown that the expression level of Kinetochore protein NDC80 homolog was significantly increased in HBV+ HCC, whereas NDC80 knockdown suppressed the proliferation of HepG2.2.15 hepatoma cells stably expressing HBV (26) and promoted cell apoptosis and cell cycle arrest in the S-phase (27). The upregulation of Aurora kinase A (AURKA) was shown to induce epithelial-mesenchymal transition and cancer stem cell properties via the PI3K/ATK pathway (28), which was identified by KEGG pathway analysis as associated with the upregulated DEGs in this study. High expression levels of FOXM1 were correlated with malignant characteristics and poor outcome of patients with HBV+ HCC and FOXM1 upregulation induced by the HBV X protein promoted the invasion and metastasis of HCC cells (29). Consistent with these findings, our pathway analysis of the genes in the first module showed that the FOXM1-regulated transcriptional network was associated with HBV+ HCC. A recent study revealed that ZWINT, known to be involved in kinetochore function, promoted HCC cell proliferation by regulating cell cycle proteins, including CDK1 (30), which is also an important hub gene identified in this study. TPX2, a microtubule-related protein, was found to contribute to the growth and metastasis of HCC, and targeting this gene suppressed carcinogenic processes by arresting mitosis and inducing genomic instability (31,32). It was also shown that the overexpression of a serine/threonine kinase PBK, another hub gene identified here, promoted the migration and invasion of HCC through the ETV4-uPAR pathway (33). However, there are no reports on the association of HCC with the hub genes TYMS and CDC45, which are involved in DNA biosynthesis. Therefore, we verified the expression levels of TYMS and CDC45 proteins in clinical samples and observed that they were higher in HBV+ HCC than in HBV− HCC or normal tissues and were associated with poor patient outcome.
Comprehensive gene expression profiling of HBV-positive tumors and non-cancerous tissues enabled us to reveal changes in gene activity and explore new candidate drug targets. Using CMap, we identified 16 bioactive molecules potentially capable of affecting, both positively and negatively, HBV-induced carcinogenesis. Several studies have discovered compounds targeting novel mechanisms involved in disease pathogenesis by using CMap analysis. Thus, apigenin was identified as a potential anti-fibrotic drug and its efficacy was proved in human hepatic stellate cells (34). Apigenin was also reported to have anti-liver cancer effects by inhibiting the expression and activity of NF-κB and Snail and to enhance the chemotherapeutic sensitivity of HCC cells through regulation of the miR-520b/ATG7 pathway (35,36). In this study, CMap analysis also revealed that apigenin had the highest negative score, suggesting its potential as a drug to treat HBV-related HCC. We also found that trifluoperazine and resveratrol might have therapeutic effects on HBV+ HCC. Previous studies reported that trifluoperazine inhibited the growth of two HCC cell lines by activating the FOXO1-related pathway (37), whereas resveratrol improved fatty liver conditions by downregulating lipogenesis and protected HBV transgenic mice against HCC by upregulating antioxidant activity (38). Although many studies showed therapeutic effects of phenoxybenzamine and repaglinide in different cancer types, there are no such reports on HCC. Thus, the compounds identified via CMap in this work deserve further investigation as candidate drugs for patients with HBV-related HCC.
In conclusion, our bioinformatics analysis indicated that HBV may promote the development of liver cancer through regulation of host gene expression. The selected 9 hub genes might serve as biomarkers and treatment targets in HBV+ HCC, whereas the 5 identified compounds could be considered as novel candidate drugs against HBV+ HCC. However, the potential HBV-associated carcinogenic mechanisms and the candidate drugs revealed by bioinformatics analysis need to be experimentally confirmed, and we aim to verify the functional activity of the 9 key genes associated with HBV+ HCC and the therapeutic effects of the 5 selected compounds in our future work.
Acknowledgments
Funding: This work was supported by National Natural Science Foundation of China (Grant number: 81870108 and 81902125), Fujian Provincial Natural Fund (Grant number: 2019J01460), Fujian Medicine Innovation Program (Grant number: 2018-CX-18), Joint Funds for the innovation of science and Technology, Fujian province (Grant number: 2017Y9053) and Startup Fund for Scientific Research at Fujian Medical University (Grant number: 2016QH034).
Footnote
Conflicts of Interest: All authors have completed the ICMJE uniform disclosure form (available at http://dx.doi.org/10.21037/atm.2020.03.94). The authors have no conflicts of interest to declare.
Ethical Statement: The authors are accountable for all aspects of the work in ensuring that questions related to the accuracy or integrity of any part of the work are appropriately investigated and resolved. The study was approved by the ethics review board of the Fujian Medical University Union Hospital and conforms to the Declaration of Helsinki.
Open Access Statement: This is an Open Access article distributed in accordance with the Creative Commons Attribution-NonCommercial-NoDerivs 4.0 International License (CC BY-NC-ND 4.0), which permits the non-commercial replication and distribution of the article with the strict proviso that no changes or edits are made and the original work is properly cited (including links to both the formal publication through the relevant DOI and the license). See: https://creativecommons.org/licenses/by-nc-nd/4.0/.
References
- Ferlay J, Soerjomataram I, Dikshit R, et al. Cancer incidence and mortality worldwide: sources, methods and major patterns in GLOBOCAN 2012. Int J Cancer 2015;136:E359-86. [Crossref] [PubMed]
- El-Serag HB, Rudolph KL. Hepatocellular carcinoma: epidemiology and molecular carcinogenesis. Gastroenterology 2007;132:2557-76. [Crossref] [PubMed]
- Li LM, Hu ZB, Zhou ZX, et al. Serum microRNA profiles serve as novel biomarkers for HBV infection and diagnosis of HBV-positive hepatocarcinoma. Cancer Res 2010;70:9798-807. [Crossref] [PubMed]
- Huang G, Li PP, Lau WY, et al. Antiviral Therapy Reduces Hepatocellular Carcinoma Recurrence in Patients With Low HBV-DNA Levels: A Randomized Controlled Trial. Ann Surg 2018;268:943-54. [Crossref] [PubMed]
- Hwang JP, Lok AS, Fisch MJ, et al. Models to Predict Hepatitis B Virus Infection Among Patients With Cancer Undergoing Systemic Anticancer Therapy: A Prospective Cohort Study. J Clin Oncol 2018.JCO2017756387. [Epub ahead of print]. [PubMed]
- Rahib L, Smith BD, Aizenberg R, Rosenzweig AB, et al. Projecting cancer incidence and deaths to 2030: the unexpected burden of thyroid, liver, and pancreas cancers in the United States. Cancer Res 2014;74:2913-21. [Crossref] [PubMed]
- Vogelstein B, Papadopoulos N, Velculescu VE, et al. Cancer genome landscapes. Science 2013;339:1546-58. [Crossref] [PubMed]
- Zhang C, Peng L, Zhang Y, et al. The identification of key genes and pathways in hepatocellular carcinoma by bioinformatics analysis of high-throughput data. Med Oncol 2017;34:101. [Crossref] [PubMed]
- Halgand B, Desterke C, Riviere L, et al. Hepatitis B Virus Pregenomic RNA in Hepatocellular Carcinoma: A Nosological and Prognostic Determinant. Hepatology 2018;67:86-96. [Crossref] [PubMed]
- Davis S, Meltzer PS. GEOquery: a bridge between the Gene Expression Omnibus (GEO) and BioConductor. Bioinformatics 2007;23:1846-7. [Crossref] [PubMed]
- Dennis G Jr, Sherman BT, Hosack DA, et al. DAVID: Database for Annotation, Visualization, and Integrated Discovery. Genome Biol 2003;4:3. [Crossref] [PubMed]
- Xie C, Mao X, Huang J, et al. KOBAS 2.0: a web server for annotation and identification of enriched pathways and diseases. Nucleic Acids Res 2011;39:W316-22. [Crossref] [PubMed]
- Chin CH, Chen SH, Wu HH, et al. cytoHubba: identifying hub objects and sub-networks from complex interactome. BMC Syst Biol 2014;8 Suppl 4:S11. [Crossref] [PubMed]
- Rhodes DR, Yu J, Shanker K, et al. ONCOMINE: a cancer microarray database and integrated data-mining platform. Neoplasia 2004;6:1-6. [Crossref] [PubMed]
- Rhodes DR, Kalyana-Sundaram S, Mahavisno V, et al. Oncomine 3.0: genes, pathways, and networks in a collection of 18,000 cancer gene expression profiles. Neoplasia 2007;9:166-80. [Crossref] [PubMed]
- Ma L, Wang X, Lan F, et al. Prognostic value of differential CCND1 expression in patients with resected gastric adenocarcinoma. Med Oncol 2015;32:338. [Crossref] [PubMed]
- Subramanian A, Kuehn H, Gould J, et al. GSEA-P: a desktop application for Gene Set Enrichment Analysis. Bioinformatics 2007;23:3251-3. [Crossref] [PubMed]
- Lamb J, Crawford ED, Peck D, et al. The Connectivity Map: using gene-expression signatures to connect small molecules, genes, and disease. Science 2006;313:1929-35. [Crossref] [PubMed]
- Makarova-Rusher OV, Medina-Echeverz J, Duffy AG, et al. The yin and yang of evasion and immune activation in HCC. J Hepatol 2015;62:1420-9. [Crossref] [PubMed]
- Chan SL, Wong VW, Qin S, et al. Infection and Cancer: The Case of Hepatitis B. J Clin Oncol 2016;34:83-90. [Crossref] [PubMed]
- Yang LY, Luo Q, Lu L, et al. Increased neutrophil extracellular traps promote metastasis potential of hepatocellular carcinoma via provoking tumorous inflammatory response. J Hematol Oncol 2020;13:3. [Crossref] [PubMed]
- Yan H, Li Z, Shen Q, et al. Aberrant expression of cell cycle and material metabolism related genes contributes to hepatocellular carcinoma occurrence. Pathol Res Pract 2017;213:316-21. [Crossref] [PubMed]
- Zheng J, Cai J, Li H, et al. Neutrophil to Lymphocyte Ratio and Platelet to Lymphocyte Ratio as Prognostic Predictors for Hepatocellular Carcinoma Patients with Various Treatments: a Meta-Analysis and Systematic Review. Cell Physiol Biochem 2017;44:967-81. [Crossref] [PubMed]
- Xu XR, Yousef GM, Ni H. Cancer and platelet crosstalk: opportunities and challenges for aspirin and other antiplatelet agents. Blood 2018;131:1777-89. [Crossref] [PubMed]
- Lei Y, Liu H, Yang Y, et al. Interaction of LHBs with C53 promotes hepatocyte mitotic entry: A novel mechanism for HBV-induced hepatocellular carcinoma. Oncol Rep 2012;27:151-9. [PubMed]
- Liu B, Yao Z, Hu K, et al. ShRNA-mediated silencing of the Ndc80 gene suppress cell proliferation and affected hepatitis B virus-related hepatocellular carcinoma. Clin Res Hepatol Gastroenterol 2016;40:297-303. [Crossref] [PubMed]
- Ju LL, Chen L, Li JH, et al. Effect of NDC80 in human hepatocellular carcinoma. World J Gastroenterol 2017;23:3675-83. [Crossref] [PubMed]
- Chen C, Song G, Xiang J, et al. AURKA promotes cancer metastasis by regulating epithelial-mesenchymal transition and cancer stem cell properties in hepatocellular carcinoma. Biochem Biophys Res Commun 2017;486:514-20. [Crossref] [PubMed]
- Xia L, Huang W, Tian D, et al. Upregulated FoxM1 expression induced by hepatitis B virus X protein promotes tumor metastasis and indicates poor prognosis in hepatitis B virus-related hepatocellular carcinoma. J Hepatol 2012;57:600-12. [Crossref] [PubMed]
- Ying H, Xu Z, Chen M, et al. Overexpression of Zwint predicts poor prognosis and promotes the proliferation of hepatocellular carcinoma by regulating cell-cycle-related proteins. Onco Targets Ther 2018;11:689-702. [Crossref] [PubMed]
- Liu Q, Tu K, Zhang H, et al. TPX2 as a novel prognostic biomarker for hepatocellular carcinoma. Hepatol Res 2015;45:906-18. [Crossref] [PubMed]
- Hsu CW, Chen YC, Su HH, et al. Targeting TPX2 Suppresses the Tumorigenesis of Hepatocellular Carcinoma Cells Resulting in Arrested Mitotic Phase Progression and Increased Genomic Instability. J Cancer 2017;8:1378-94. [Crossref] [PubMed]
- Yang QX, Zhong S, He L, et al. PBK overexpression promotes metastasis of hepatocellular carcinoma via activating ETV4-uPAR signaling pathway. Cancer Lett 2019;452:90-102. [Crossref] [PubMed]
- Hicks DF, Goossens N, Blas-Garcia A, et al. Transcriptome-based repurposing of apigenin as a potential anti-fibrotic agent targeting hepatic stellate cells. Sci Rep 2017;7:42563. [Crossref] [PubMed]
- Qin Y, Zhao D, Zhou HG, et al. Apigenin inhibits NF-kappaB and snail signaling, EMT and metastasis in human hepatocellular carcinoma. Oncotarget 2016;7:41421-31. [Crossref] [PubMed]
- Gao AM, Zhang XY, Hu JN, et al. Apigenin sensitizes hepatocellular carcinoma cells to doxorubic through regulating miR-520b/ATG7 axis. Chem Biol Interact 2018;280:45-50. [Crossref] [PubMed]
- Jiang J, Huang Z, Chen X, et al. Trifluoperazine Activates FOXO1-Related Signals to Inhibit Tumor Growth in Hepatocellular Carcinoma. DNA Cell Biol 2017;36:813-21. [Crossref] [PubMed]
- Lin HC, Chen YF, Hsu WH, et al. Resveratrol helps recovery from fatty liver and protects against hepatocellular carcinoma induced by hepatitis B virus X protein in a mouse model. Cancer Prev Res (Phila) 2012;5:952-62. [Crossref] [PubMed]