Novel tumor mutation score versus tumor mutation burden in predicting survival after immunotherapy in pan-cancer patients from the MSK-IMPACT cohort
Introduction
A high tumor mutation burden (TMB), which represents genomic instability, has the potential to induce neoantigen production and further improvements in immunogenicity (1). Emerging evidence has proved that TMB measured by next-generation sequencing (NGS) cancer gene panels is highly consistent with TMB measured by whole-exome sequencing (WES), and TMB can serve as a candidate biomarker of clinical outcomes from immune checkpoint inhibitors (ICIs) (2,3) in melanoma (4,5), lung cancer (6-9), and urothelial carcinoma (10). A recent study reported that a high TMB was associated with improved overall survival (OS) in patients receiving ICIs across a wide variety of cancer types, but there may not be one universal definition of high TMB (11).
TMB is defined as the total number of mutations found in the DNA of cancer cells (12). Nonselective TMB includes all nonsynonymous somatic mutations in the calculation (12,13). However, growing evidence has proved that not all mutations correlate with favorable outcomes after immunotherapy. Mutations including those in EGFR and STK11 are not correlated with the clinical benefit of ICIs or even predicted primary resistance to ICIs (14-16). EGFR-mutant non-small cell lung cancer (NSCLC) is likely to have decreased PD-L1 expression (14), and ICI treatment does not improve OS compared with docetaxel (17). STK11 mutation is significantly correlated with a high TMB; however, few tumor-infiltrating lymphocytes and low PD-L1 expression are also detected in STK11-mutant NSCLC (18). STK11 alterations are reported to be the most prevalent genomic driver of primary resistance to PD-1 axis inhibitors in KRAS-mutant lung adenocarcinoma (15).
All the nonsynonymous somatic mutations are taken into account in the TMB calculation, which we believe significantly attenuates the power of TMB as a biomarker for ICIs. Therefore, we developed tumor mutation score (TMS), defined as the number of genes with nonsynonymous somatic mutations among certain candidate genes. Compared with TMB, TMS as defined in this study allowed us to exclude adverse mutations and even unnecessary mutations and would not be affected across platforms. In this study, we compared TMS and TMB in predicting survival after ICI treatment in the large Memorial Sloan Kettering Cancer Center (MSKCC) Integrated Mutation Profiling of Actionable Cancer Targets (MSK-IMPACT) cohort (11,19).
Methods
Patients and data curation
The MSK-IMPACT cohort involves a large-scale, prospective clinical sequencing initiative using a comprehensive assay, MSK-IMPACT, in more than 10,000 patients. The original report was published in 2017 and released clinical and sequencing data consisting of 10,945 samples from 10,336 patients with 62 principal cancer types between January 2014 and May 2016 (19). Patients enrolled underwent MSK-IMPACT testing using tumor samples and blood as matched normal (germline) DNA. Recently, the MSK-IMPACT cohort was updated (11) with important follow-up data from 1,661 advanced cancer patients treated with at least one dose of an ICI (atezolizumab, avelumab, durvalumab, ipilimumab, nivolumab, pembrolizumab or tremelimumab). These 1,661 ICI-treated patients were classified into ten major cancer types. Accordingly, we extracted 3,840 matched non-ICI-treated patients with the same cancer types. The clinical and mutation profiles of the cohort are available at cBioPortal (http://www.cbioportal.org).
MSK-IMPACT testing
The MSK-IMPACT test involves a custom NGS gene panel that is being investigated in clinical trials at MSKCC. Three versions of the MSK-IMPACT panels, which include 341 genes, 410 genes and 468 genes, respectively, were used in this study (11). Recently, the MSK-IMPACT test was authorized by the U.S. Food and Drug Administration (FDA) as an in vitro NGS test for various cancers (12). Generally, the MSK-IMPACT test requires tumor and matched blood samples and is capable of detecting somatic single nucleotide variants, insertions, deletions, copy number alterations and select structural rearrangements in genes in human genomic DNA obtained from tumor tissue (19).
TMB and TMS calculation
TMB was calculated according to the MSK-IMPACT workflow. The total number of somatic mutations, including driver mutations in oncogenes, was normalized to the exonic coverage of the respective MSK-IMPACT panel in megabases (Mb). The total exonic coverage of the three versions of the MSK-IMPACT panels varied little and was approximately 0.89 Mb for each panel (12).
TMS was defined as the number of genes with nonsynonymous somatic mutations among certain candidate genes. The total TMS was defined as the number of genes with nonsynonymous somatic mutations among all the genes sequenced, while TMS55 was defined as the number of genes with nonsynonymous somatic mutations among 55 favorable prognostic genes (listed below) uncovered in the survival analysis in patients treated with ICIs. The unsequenced genes in the earlier versions of the MSK-IMPACT test were assumed to be wild-type or not mutant.
Survival and statistical analyses
Among the 1,661 ICI-treated patients, to identify the favorable prognostic genes, only genes with a mutation rate higher than or equal to 5% in each type of cancer or in pan-cancer were selected (to generate enough number of patients with mutations), and survival analysis was performed on these genes by dividing patients into a nonmutant group and a mutant group based on each gene. A total of 67 genes were significantly associated with OS in at least one tumor type, among which 55 genes were favorable in at least one and were not adverse factors in all tumor types. In the comparison between TMS55 and TMB, TMS55 was divided into three groups by universal cutoff values (TMS55 =0, 1≤ TMS55 ≤5, and TMS55 >5), while TMB was divided into three subgroups by quantiles within each histology group as originally reported (11), as there is no universal cutoff value of TMB across different cancer types.
The 55 favorable prognostic genes were EPHA3, EPHA5, EPHA7, MGA, NTRK3, PTPRD, ZFHX3, ATM, CDKN2A, CDKN2Ap16INK4A, CREBBP, KDR, LATS1, NCOR1, BRCA1, CIC, CTCF, DNMT1, EPHB1, FANCA, IRS1, NCOA3, NOTCH3, PTCH1, RAD54L, RNF43, SMO, SPEN, TET1, NF1, CARD11, IGF1R, MLL2, PTPRT, TERT, VHL, PIK3CG, ALK, ARID1B, ARID2, BRAF, BRCA2, ERBB4, FAT1, GRIN2A, MLL, MLL3, NOTCH2, NOTCH4, NRAS, PAK7, PIK3C2G, POLE, SETD2, and TP63. The 12 adverse prognostic genes were PBRM1, ELF3, PIK3CA, APC, CSF3R, TP53, ROS1, BAP1, GNAQ, ARID1A, KEAP1, and STK11.
The log-rank test was determined to analyze the difference in OS, and the hazard ratio (HR) was determined from a Cox proportional hazards model using the survival package (version 2.43-3). Survival models were compared with the survival ROC package (version 1.0.3) and survcomp package (version 1.36.0). Cross-validation of the survival analysis was performed using the caret package (version 6.0-84). All the statistical analysis was performed in R (version 3.5.1) and the reported P value was two-sided.
Results
Overview of the MSK-IMPACT cohort
The MSK-IMPACT cohort includes 10,336 advanced cancer patients, among which, a total of 1,661 patients treated with ICIs (termed the ICI group) were included. This study mainly compared TMS and TMB as biomarkers for immunotherapy in the 1,661 ICI-treated patients and in 3,840 matched patients (termed the non-ICI group) (Table 1, Figure S1). The ICI group included 215 bladder cancer patients, 44 breast cancer patients, 110 colorectal cancer patients, 126 esophagogastric cancer patients, 117 glioma patients, 139 head and neck cancer patients, 350 NSCLC patients, 321 melanoma patients, 151 renal cell carcinoma patients, and 88 patients with an unknown cancer (Table 1, Figure S1). Among these patients, 1,307 (78.7%) were treated with the PD-1/PD-L1 ICI, 99 (6.0%) were treated with the CTLA4 ICI and 255 (15.3%) were treated with both PD-1/PD-L1 and CTLA4 ICIs (Table 1). Most patients were examined by the 410-gene panel (IMPACT410). Compared with the latest version of the 468-gene panel (IMPACT468), the unsequenced genes in the earlier versions were assumed to be wild-type or not mutant (Table 1).
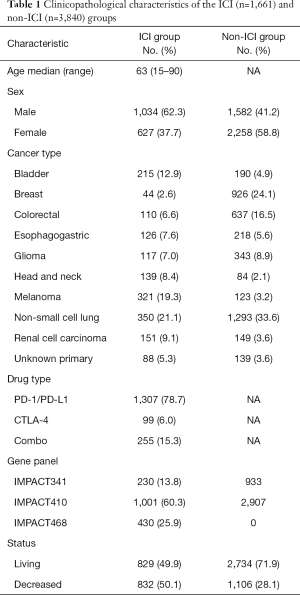
Full table
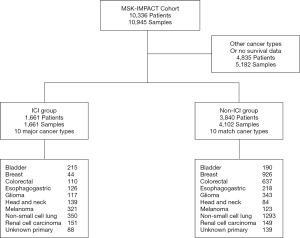
Characteristics of TMS- and survival-related genes in the ICI pan-cancer group
Among the 1,661 patients treated with ICIs, we first calculated the total TMS based on all sequenced genes, and the total TMS was highly correlated with TMB (R=0.98, P<2e-16, Figure 1A). To determine the relationship between the number of genes and correlations between TMS and TMB, we performed correlation analysis based on 1 to 300 randomly selected genes, and the results showed that the correlation coefficient values increased with the number of genes (Figure 1B). To select favorable genes that predicted prolonged OS, survival analysis was performed among genes with mutation rates equal or greater than 5% in each cancer type and in pan-cancer. As a result, a total of 67 genes were significantly associated with OS in at least one tumor type (Figure 1C), among which 55 were favorable in at least one and were not adverse factors in all tumor types (Figure 1C). For example, TERT was significantly associated with prolonged survival in melanoma and pan-cancer (Figure 1C). Notably, 12 genes were removed since these genes were adverse prognostic factors in at least one cancer type, such as TP53, which was favorable in bladder cancer but adverse in colorectal cancer and pan-cancer (Figure 1C). The top 10 survival-associated genes specific to each cancer type are shown in Figure S2.
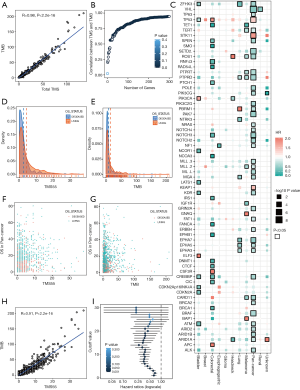
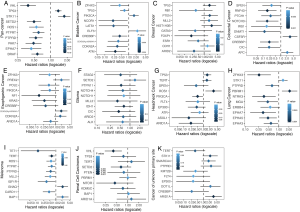
Then, we calculated TMS55 based on these 55 favorable prognostic genes. The majority of patients had low TMS55 or TMB, and the difference in the median TMS55 (dashed line) seemed larger than the median TMB (dashed line) between living and deceased patients (Figure 1D,E). In particular, the living patients shifted right based on TMS55 compared to TMB (Figure 1F,G). Dot plots of the distribution of OS in each cancer type are shown in Figure S3. More importantly, TMS55 was also significantly correlated with TMB (R=0.91, P<2×10−16, Figure 1H). Notably, compared with the correlation coefficient based on the 55 randomly selected genes shown in Figure 1B, the correlation coefficient of TMS55 was much higher (0.91 vs. 0.73). Last, to determine the cutoff value of TMS55, we performed survival analysis in which patients were divided from 1 to 30 based on TMS55. As shown in Figure 1I, the HR was 0.26 (P=2.93×10−8) at a cutoff of 14 and 0.43 (P=1.04×10−11) at a cutoff of 5. Based on the HR, P value and distribution of TMS55, a cutoff of 5 might be better definition of a high TMS55 with the smallest HR and 95% CI (Figure 1I). Therefore, the significant correlation and divergence between TMS55 and TMB was the basis for TMS55 as a biomarker for immunotherapy.
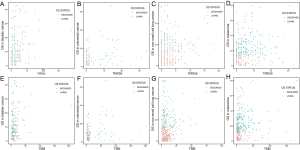
Mutation landscape of the top 20 favorable genes in the ICI pan-cancer group
The 55 favorable genes are listed in the Methods section. Only genes with the top 20 mutation rates in the 1,661 ICI-treated patients are presented in Figure 2. In the OncoPrint plot, patients were arranged by cancer type followed by OS, resulting in a high proportion of deceased patients with short OS in each cancer type (Figure 2). However, no obvious trend of a high TMB or mutation count in patients with prolonged OS was observed based on cancer type (Figure 2). Notably, most melanoma patients received combined ICIs or the CTLA-4 ICI alone, whereas other cancer patients received mainly the PD-1/PD-L1 ICI (Figure 2). The most commonly mutated genes were TERT (31%), MLL2 (14%) and NF1 (11%), with mutation rates all higher than 10%. Notably, the dominant mutation type in TERT was promoter mutation, and TERT mutation was detected mainly in bladder cancer, glioma and melanoma (Figure 2). Similarly, VHL mutation was observed mainly in renal cell carcinoma (Figure 2). Moreover, the ZFHX3 mutation profile was not available in some patients because different versions of the MSK-IMPACT test were used (Figure 2). Therefore, this glimpse into the mutation landscape of the top 20 favorable genes demonstrated that the mutation profile varied greatly among different cancer types. The detailed mutation profiles are provided in Figure S4.
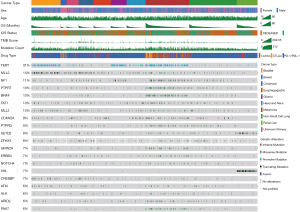
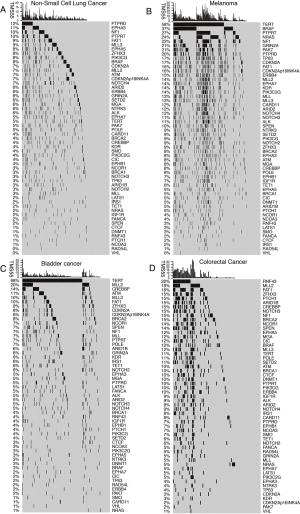
Comparison between TMS and TMB in predicting OS after immunotherapy
We compared TMS versus TMB using similar parameters from the original report (11). Here, patients were divided into three subgroups by TMB quartiles within each histology group (11), and the specific cutoff values are listed in Table 2. We set universal cutoff values for TMS55 (TMS55 =0, 1≤ TMS55 ≤5, and TMS55 >5) in the survival analysis (Table 2). Surprisingly, TMS55 dramatically enhanced the power of distinguishing poor and good OS in pan-cancer and each histology (Figure 3A and B). Compared with nonmutant patients (TMS55 =0), patients with 1 to 5 mutated genes (1≤ TMS55 ≤5, HR=0.772, P=0.001) and more than 5 mutated genes (TMS55 >5, HR=0.307, P<2×10−16) were significantly associated with improved OS (Figure 3A). In addition, our TMB results in the survival analysis were consistent with those in the original report (11) (i.e., significant differences between the top 10-20% (HR=0.730, P=0.007) and top 10% (HR=0.529, P=1×10−6) with the bottom 80%) (Figure 3B). More importantly, TMS55, compared with TMB, not only generated smaller HRs (Table 2) but also provided more patients with clinical benefits since the bottom 80% of patients were defined as controls (Figure 3B). The AUC of TMS55 was 0.65, which was significantly higher than 0.58 in TMB (Figure 3C). The C-index of TMS55 was significantly higher than that of TMB (TMS55 0.65 vs. TMB 0.54, P<0.001). We also investigated TMS55 and TMB in each cancer type (Figure 3D). Generally, the HRs of TMS55 were smaller than those of TMB (Figure 3D, Table 2) in each cancer type and in pan-cancer. Moreover, TMS55 was a significant prognostic factor in more types of tumors compared with TMB (Figure 3D, Table 2). Notably, TMS55 was significant in colorectal cancer, melanoma and renal cell carcinoma, whereas TMB was not (Figure 3D), indicating that TMS55 is more robust than TMB in predicting OS after ICI treatment in certain types of cancer. In addition, cross-validation by 1,000 randomizations in half of the patients was also performed for the survival analysis, and the results proved that TMS55 was a significant predictor of OS (Figure 3E). Representative Kaplan–Meier plots of TMS55 in NSCLC, melanoma, bladder cancer and colorectal cancer are shown in Figure 3F,G,H,I.
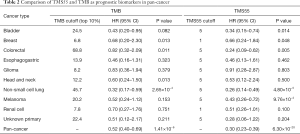
Full table
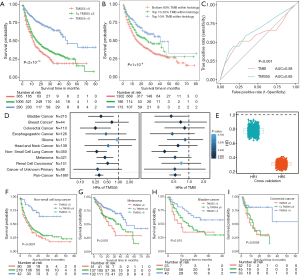
Mutation landscape of the top 20 genes and characteristics of TMS55 in the non-ICI pan-cancer group
We further explored TMS55 and the mutation landscape of the 55 favorable genes in the 3,840 matched non-ICI-treated patients. As shown in Figure 4A, patients were also arranged by cancer type followed by OS. Similarly, high mutation rates in TERT, MLL2 and NF1 remained (Figure 4A), although the specific rates varied from those of the ICI group. The mutation landscape of the top 20 genes in the non-ICI group was largely consistent with that in the ICI group, and the minor differences might be due to the different proportions of each cancer type. Furthermore, the non-normally distributed TMS55 was almost the same as that of TMB in non-ICI-treated patients, with no significant difference in the median TMS55 or TMB between living and deceased patients since patients did not receive ICI treatment (P=0.949, Figure 4B and C). Correlation analysis also proved that TMS55 and TMB were highly correlated (Figure 4D and E). These consistencies demonstrated that our analysis in ICI-treated patients was reliable. Further survival analysis demonstrated that a high TMS55 and TMB predicted poor OS in pan-cancer, especially in glioma, NSCLC, and colorectal cancer (Figure S5). These findings were largely consistent with those from a previous report (20). However, TMB seemed to be more powerful as a prognostic biomarker than TMS55 in non-ICI-treated patients, indicating that TMS55 is more suitable for patients receiving immunotherapy.
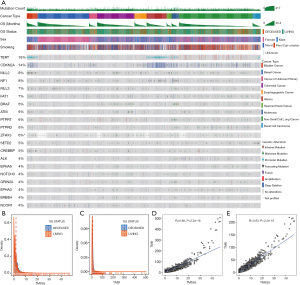
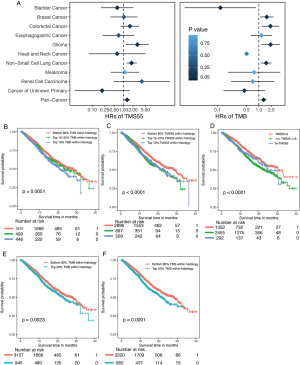
Discussion
In this study, we proposed a new concept of TMS as a prognostic biomarker for cancer patients treated with ICIs. Based on the accessible data of the MSK-IMPACT cohort, we first divided the patients into an ICI group of 1,661 patients and a matched non-ICI group of 3,840 patients. Then, in the ICI group, univariate survival analyses were performed for each gene in all 10 cancer types and in pan-cancer to identify gene mutations associated with improved OS. As a result, 67 genes were significantly associated with OS, among which 55 were favorable prognostic factors and 12 were removed since they predicted poor OS in at least one cancer type. Next, TMS55 was calculated based on these 55 favorable genes and compared with TMB as a prognostic factor in ICI-treated patients. A universal cutoff value was set for TMS55, while TMB was subgrouped by quantiles within each histology group. TMS55 was highly correlated with TMB and was more robust than TMB in the survival analysis. TMS55 was significantly associated with prolonged OS in more cancer types than TMB, with smaller HRs and P values. More importantly, cross-validation by randomization confirmed the predictive efficiency of TMS55 in pan-cancer. Finally, TMS55 and TMB were further explored in matched non-ICI-treated patients. Similarly, TMS55 was highly correlated with TMB, whereas a high TMS55 and TMB predicted poor OS in non-ICI-treated patients. Taken together, these results suggest that the novel TMS55 might be a better biomarker than TMB in patients treated with ICIs.
Currently, TMB is usually measured by NGS-targeted gene panels and has been proven to be significantly associated with TMB measured by WES (11). The MSK-IMPACT test and FoundationOne CDx have been authorized by the FDA and offer the reliable identification of true somatic mutations (12). The MSK-IMPACT test tabulates nonsynonymous mutations using sequencing data from both tumor and germline DNAs (for variant calling), while germline DNA is not sequenced in FoundationOne CDx, but filtering for both oncogenic driver events and the germline status was carried out using public and private variant databases (12,21). Therefore, it is worth noting that TMB may differ across platforms and gene panels. Furthermore, there was no universal cutoff value for a high TMB. Different thresholds for a high TMB were recently reported, with no consensus widely accepted worldwide (12,21). More importantly, the predictive efficiency of TMB as a biomarker for immunotherapy is not satisfactory (12,13). With more insights into the relationship between mutations and the response to ICIs in cancer, not all mutations correlate with a good response to ICIs. Driver mutations such as those in EGFR and other mutations including those in STK11, as mentioned above, are not favorable or even predict a poor response to ICIs (15,22). However, TMB nonselectively includes all nonsynonymous somatic mutations in the calculation, which attenuates its power in distinguishing a good or poor response to ICIs. Therefore, compared with TMB, the key concept of TMS55 is to select favorable gene mutations to achieve a better effect, and the scoring algorithm in TMS55, rather than normalization to gene length in TMB, allows us to overcome differences across platforms. Therefore, the easy calculation and universal cutoff value of TMS will not be affected across platforms and is more feasible in clinical practice.
In the survival analysis to select favorable genes, the results were largely consistent with those from previous reports. For example, STK11 mutation predicted poor OS in NSCLC and cancer of an unknown primary site and was significantly associated with short OS in pan-cancer (Figure 1C). It is worth noting that PIK3CA, APC, TP53, ARID1A and KRAS, which were reported to be favorable factors in response to ICIs, were not among the 55 genes. The reason why these genes were removed was because these genes were significantly associated with poor OS in at least one cancer type (Figure 1C). PIK3CA mutation was an adverse prognostic factor in bladder cancer (Figure 1C), APC and TP53 mutations predicted poor OS in colorectal cancer (Figure 1C), and ARID1A was significantly associated with short OS in cancer of an unknown primary site (Figure 1C). In addition, KRAS was not significant in our survival analysis. As a proof-of-concept study, a specific gene list and mechanistic study of these genes are needed in further investigations.
In our analysis, we chose only genes with a mutation rate greater than or equal to 5% to generate enough patients with mutations to perform the survival analysis. Therefore, the number of candidate genes might be expanded with a larger cohort by including genes with lower mutation rates. Moreover, we did not define genes associated with poor OS with negative values in TMS and excluded only these genes in the analysis, since our concept was to make TMS easy to calculate and thus achieve a universal cutoff value. Last, it is also possible to identify whether specific mutations are associated with the clinical response and measure selective TMB at a higher resolution. Simple scoring system of TMS55 would not be affected with these changes since all favorable genes were regarded as independent factors. This finding was based only on the MSK-IMPACT cohort. Although it was a large cohort and cross-validation by randomization was performed, independent validation or even prospective clinical trials of TMS55 are needed before the application of TMS55 in clinical practice.
In summary, we developed a more feasible and robust algorithm than TMB, referred to as TMS, as a biomarker for cancer patients treated with ICIs. TMS55, consisting of 55 candidate genes, still needs further investigation in a larger cohort and more tumor types. This novel TMS may provide a universal measure to promote the application of mutation-based biomarkers for cancer patients after immunotherapy.
Acknowledgments
We thank Prof. Jie He at the National Cancer Center/Cancer Hospital, Chinese Academy of Medical Sciences and Peking Union Medical College, for his guidance and advice on this study.
Funding: This work was supported by the National Natural Science Foundation of China (Grant 81902369).
Footnote
Conflicts of Interest: All authors have completed the ICMJE uniform disclosure form (available at http://dx.doi.org/10.21037/atm.2020.03.163). The authors have no conflicts of interest to declare.
Ethical Statement: The authors are accountable for all aspects of the work in ensuring that questions related to the accuracy or integrity of any part of the work are appropriately investigated and resolved.
Open Access Statement: This is an Open Access article distributed in accordance with the Creative Commons Attribution-NonCommercial-NoDerivs 4.0 International License (CC BY-NC-ND 4.0), which permits the non-commercial replication and distribution of the article with the strict proviso that no changes or edits are made and the original work is properly cited (including links to both the formal publication through the relevant DOI and the license). See: https://creativecommons.org/licenses/by-nc-nd/4.0/.
References
- Schumacher TN, Schreiber RD. Neoantigens in cancer immunotherapy. Science 2015;348:69-74. [Crossref] [PubMed]
- Yarchoan M, Johnson BA 3rd, Lutz ER, et al. Targeting neoantigens to augment antitumour immunity. Nat Rev Cancer 2017;17:209-22. [Crossref] [PubMed]
- Yarchoan M, Hopkins A, Jaffee EM. Tumor Mutational Burden and Response Rate to PD-1 Inhibition. N Engl J Med 2017;377:2500-1. [Crossref] [PubMed]
- Van Allen EM, Miao D, Schilling B, et al. Genomic correlates of response to CTLA-4 blockade in metastatic melanoma. Science 2015;350:207-11. [Crossref] [PubMed]
- Snyder A, Makarov V, Merghoub T, et al. Genetic basis for clinical response to CTLA-4 blockade in melanoma. N Engl J Med 2014;371:2189-99. [Crossref] [PubMed]
- Carbone DP, Reck M, Paz-Ares L, et al. First-Line Nivolumab in Stage IV or Recurrent Non-Small-Cell Lung Cancer. N Engl J Med 2017;376:2415-26. [Crossref] [PubMed]
- Rizvi NA, Hellmann MD, Snyder A, et al. Cancer immunology. Mutational landscape determines sensitivity to PD-1 blockade in non-small cell lung cancer. Science 2015;348:124-8. [Crossref] [PubMed]
- Hellmann MD, Ciuleanu TE, Pluzanski A, et al. Nivolumab plus Ipilimumab in Lung Cancer with a High Tumor Mutational Burden. N Engl J Med 2018;378:2093-104. [Crossref] [PubMed]
- Hellmann MD, Callahan MK, Awad MM, et al. Tumor Mutational Burden and Efficacy of Nivolumab Monotherapy and in Combination with Ipilimumab in Small-Cell Lung Cancer. Cancer Cell 2018;33:853-61 e4.
- Balar AV, Galsky MD, Rosenberg JE, et al. Atezolizumab as first-line treatment in cisplatin-ineligible patients with locally advanced and metastatic urothelial carcinoma: a single-arm, multicentre, phase 2 trial. Lancet 2017;389:67-76. [Crossref] [PubMed]
- Samstein RM, Lee CH, Shoushtari AN, et al. Tumor mutational load predicts survival after immunotherapy across multiple cancer types. Nat Genet 2019;51:202-6. [Crossref] [PubMed]
- Chan TA, Yarchoan M, Jaffee E, et al. Development of tumor mutation burden as an immunotherapy biomarker: utility for the oncology clinic. Ann Oncol 2019;30:44-56. [Crossref] [PubMed]
- Alexander M, Galeas J, Cheng H. Tumor mutation burden in lung cancer: a new predictive biomarker for immunotherapy or too soon to tell? J Thorac Dis 2018;10:S3994-S8. [Crossref] [PubMed]
- Soo RA, Lim SM, Syn NL, et al. Immune checkpoint inhibitors in epidermal growth factor receptor mutant non-small cell lung cancer: Current controversies and future directions. Lung Cancer 2018;115:12-20. [Crossref] [PubMed]
- Skoulidis F, Goldberg ME, Greenawalt DM, et al. STK11/LKB1 Mutations and PD-1 Inhibitor Resistance in KRAS-Mutant Lung Adenocarcinoma. Cancer Discov 2018;8:822-35. [Crossref] [PubMed]
- Garassino MC, Cho BC, Kim JH, et al. Durvalumab as third-line or later treatment for advanced non-small-cell lung cancer (ATLANTIC): an open-label, single-arm, phase 2 study. Lancet Oncol 2018;19:521-36. [Crossref] [PubMed]
- Lee CK, Man J, Lord S, et al. Checkpoint Inhibitors in Metastatic EGFR-Mutated Non-Small Cell Lung Cancer-A Meta-Analysis. J Thorac Oncol 2017;12:403-7. [Crossref] [PubMed]
- Scheel AH, Ansen S, Schultheis AM, et al. PD-L1 expression in non-small cell lung cancer: Correlations with genetic alterations. Oncoimmunology 2016;5:e1131379. [Crossref] [PubMed]
- Zehir A, Benayed R, Shah RH, et al. Mutational landscape of metastatic cancer revealed from prospective clinical sequencing of 10,000 patients. Nat Med 2017;23:703-13. [Crossref] [PubMed]
- Owada-Ozaki Y, Muto S, Takagi H, et al. Prognostic Impact of Tumor Mutation Burden in Patients With Completely Resected Non-Small Cell Lung Cancer: Brief Report. J Thorac Oncol 2018;13:1217-21. [Crossref] [PubMed]
- Truesdell J, Miller VA, Fabrizio D. Approach to evaluating tumor mutational burden in routine clinical practice. Transl Lung Cancer Res 2018;7:678-81. [Crossref] [PubMed]
- Offin M, Rizvi H, Tenet M, et al. Tumor Mutation Burden and Efficacy of EGFR-Tyrosine Kinase Inhibitors in Patients with EGFR-Mutant Lung Cancers. Clin Cancer Res 2019;25:1063-9. [Crossref] [PubMed]