Preliminary study to identify severe from moderate cases of COVID-19 using combined hematology parameters
Introduction
Coronavirus was first isolated and named in the 1960s. It is a zoonotic RNA virus that can spread between animals and humans. It can cause respiratory and intestinal infections in mammals and birds. There are currently 7 known coronaviruses that can infect humans, 4 of which are common pathogens of human colds, which usually do not cause serious illness. Patients with the virus show common cold symptoms such as fever and swelling of the throat (1). Coronavirus initially received attention from the cases of severe acute respiratory syndrome (SARS) caused by the SARS coronavirus (SARS-CoV) that broke out in Asia between 2002 and 2003, with more than 8,000 people being infected and a mortality rate of approximately 9.6% (2-5). It garnered further notice from the subsequent the Middle East respiratory syndrome (MERS) caused by the Middle East respiratory syndrome coronavirus (MERS-CoV) that broke out in 2012 in the Middle East, Africa, and other regions, with more than 2,000 diagnosed cases and a mortality rate of about 34.4% (6,7). The third fatal coronavirus is the novel coronavirus (SARS-CoV-2) that causes novel coronavirus pneumonia (COVID-19) which first broke out in December 2019 (1,8). Fever, dry cough, and fatigue are the main manifestations. Severe patients often have dyspnea and/or hypoxemia 1 week after the onset of symptoms. In severe cases, they can quickly progress to acute respiratory distress syndrome (ARDS), septic shock, difficult-to-correct metabolic acidosis, coagulation dysfunction, and multiple organ failure (9-11). As of March 16, 2020, there have been more than 80,000 confirmed cases in various provinces and cities in China, with a mortality rate of approximately 3.97%. The number of confirmed patients outside China has also increased rapidly. About 24,000 cases were diagnosed on March 8, but by March 16, more than 86,000 cases had been diagnosed. The mortality rate also increased from 1.96% to 3.92% (12).
According to the Guidelines for the Diagnosis and Treatment of Novel Coronavirus (2019-nCoV) Infection (trial version 6) (13) (hereinafter referred to as “Diagnosis and Treatment Guidelines”) issued by the National Health Commission of the People’s Republic of China, COVID-19 cases can be divided, based on the clinical manifestations of the patient’s disease and treatment with different measures, into the four following types: mild, moderate, severe, and critical. Patients with mild clinical manifestations may not initially need to be admitted for treatment, but may show respiratory symptoms within the second week, so all patients should be closely monitored. The World Health Organization (WHO) has reported that about 80% of infected people have mild to moderate infections (including those with or without pneumonia), 13.8% of infected people have severe infections, and 6.1% of infected people have critical illness (14). A meta-analysis of more than 50,000 cases showed that severe cases account for 18.1% of all infected patients (15). It has also been reported (16) that patients with mild-to-moderate infection, severe infection, and critical infection account for 80.9%, 13.8%, and 4.7% of confirmed cases respectively. Experts from China have reported that approximately 26.1–32.0% of confirmed cases could develop into severe or critical cases (9,11), and the fatality rate of critical cases could reach an alarming 61.5% (17). It has been reported that with the increase of the age of infected patients, the mortality rate also increases, and the crude mortality rate in people over 80 years old is 21.9% (14). Thus, the identification and diagnosis of severe or critical patients is critical. Hematology analysis is the most widely performed test in the clinic, and in almost all laboratories, large and small, outpatient and inpatient, the first thing clinicians want to see is a CBC results. In the current novel coronavirus pandemic, if the most routine and inexpensive laboratory tests can be used to provide clinicians with the convenient assistance in determining the patient’s condition, it will be very beneficial for clinicians to make a reasonable allocation of medical resources, and the early clinical intervention is expected to reduce the patient mortality.
The Diagnosis and Treatment Guidelines (trial version 6) (13) indicate that the total number of peripheral white blood cells is normal or while the lymphocyte count is reduced in patients at the early stage of COVID-19. Tan et al. (18) conducted a descriptive and predictive study and found that lymph cell % (Lym%) was inversely related to the severity and prognosis of patients, and could be used to predict the severity and prognosis of patients with COVID-19. This suggests that some changes in the peripheral blood will occur in patients with SARS-CoV-2 infections. These changes have the potential to provide clues or guidance for the diagnosis, treatment, and prognosis of COVID-19 patients. BC-6900 is newest hematology analyzer of Mindray Medical International Co., Ltd (Shenzhen, China). It uses the principle of nucleic acid fluorescence staining and flow cytometry to detect white blood cells, red blood cells, and platelets in peripheral venous blood in 3 dimensions (3D). The blood cells are identified and quantitatively analyzed according to the volume of the cells, the complexity of the contents, and the nucleic acid content. In addition to providing the most routine hematology parameters, it can also quantitatively detect immature granulocytes, nucleated red blood cells and naive platelets, and blast cells. Based on the analysis of the results from BC-6900, this study intended to discover the characteristic changes of the peripheral blood and explore the value of routine hematological parameters in the diagnosis and treatment of COVID-19 patients.
In order to explore the value of these routine hematological parameters, we retrospectively analyzed the epidemiological and laboratory test results of 45 moderate and severe cases. The differences between the different groups found in most of the routine laboratory tests were further analyzed with the hope that the information yielded could aid clinicians in the diagnosis and treatment of this disease. We present the following article in accordance with the STROBE reporting checklist (available at http://dx.doi.org/10.21037/atm-20-3391).
Methods
Patients
In this study, complete medical data of 45 hospitalized cases were collected between January 23, 2020, and February 13, 2020 from the Department of Laboratory Medicine, Jingzhou Central Hospital, The 2nd Clinical Medical College, Yangtze University (Jingzhou, Hubei province, China). Among these 45 patients, 35 cases were moderate and 10 cases were severe. All patients were confirmed by viral detections using novel coronavirus 2019-nCoV nucleic acid detection kit (fluorescent PCR method, Shanghai BioGerm Medical Biotechnology Co., Ltd). Coinfections by other respiratory viruses, including respiratory syncytial virus, adenovirus, influenza virus A, influenza virus B, parainfluenza virus, chlamydia pneumoniae, legionella pneumoniae, and mycoplasma pneumoniae were ruled out using the serological method. All case were diagnosed and classified according to the Diagnosis and Treatment Guidelines (Trial Sixth Edition). The clinical standards for the identification of moderate cases were fever, respiratory track symptoms, and pneumonia imaging (multiple small patches and stromal changes, and the lung extraneous zone was obvious); meanwhile, in addition to having the symptoms and signs of moderate cases, severe cases also showed (I) respiratory distress and respiratory rate (RR) R30 times/minute; (II) oxygen saturation ≤93% under rest state; (III) oxygen partial pressure (PaO2)/oxygen concentration (FiO2) in the arterial blood ≤300 mmHg; and (IV) lung imaging progress >50% in the short term (24–48 h).
Data collection
The characteristic epidemiological information (including recent exposure history, such as travel history and contact with patients with fever or respiratory symptoms from other cities in Hubei province or confirmed cases within 2 weeks) and basic information such as gender, age, clinical symptoms, and signs were collected from the admission records. All the laboratory data including complete blood count (BC-6900, Mindray, Shenzhen, China), serum biochemistry (AU5800, Beckman Coulter, CA, USA), and coagulation tests (ACL TOP 700, Werfen, Barcelona, Spain), were collected from the laboratory information system (LIS).
In this study, a total of 161 results detected on the Mindray BC-6900 hematology analyzer, along with biochemistry and coagulation tests, were collected from 45 patients with SARS-CoV-2 infection between January 23, 2020 and February 13, 2020. The laboratory test results were divided into two groups based on the sources of the samples, from moderate or severe patients, on which retrospective analysis and comparative analysis were performed.
Statistical analysis
In this study, SPSS statistics software (version 19.0) was used for statistical data analysis and Graphpad Prism software (version 8.0.2) was used for mapping. Age was represented in median (range), and other demographic and clinical characteristics were expressed in frequency and percentage. The significance was tested by chi squared or Fisher’s exact test. The quantized variables of blood parameters were expressed as mean ± standard deviation. The significance between the two groups was tested by Student’s t-test. P value <0.05 was considered statistically significant in all statistical analyses. Linear discriminant analysis (LDA) was employed to conduct a linear combination of each of the two parameters and extract the best data features that could distinguish the moderate COVID-19 cases from the severe ones. The diagnostic values of valuable parameters for differential mild and severe cases of COVID-19 patients were assessed by receiver operating characteristic (ROC) and area under the ROC curve (AUC).
LDA for combined parameters
The combined parameters were analyzed using LDA. LDA, also known as Fisher’s linear discriminant (FLD), is a supervised learning model. It was invented by Ronald Fisher in 1936 (19). LDA functions according to the following principles: Using multi-parameter linear combination, the high-dimensional pattern samples composed of multi-parameters are projected to the optimal discrimination vector space to achieve the effect of extracting classification information and compressing the feature space dimension. The new subspace has the largest inter-class distance and the smallest intra-class distance; that is, the pattern has the best separability in this space. Therefore, it is an effective feature extraction method.
Results
Demographic and clinical characteristics of COVID-19 patients
The 45 confirmed COVID-19 patients were divided into moderate (35 cases, 77.8%) and severe (10 cases, 22.2%) infection groups (Table 1). There was no significant difference in the median age between the two groups (P>0.05). Of the patients, 23 were male (51.1%) and 22 were female (48.9%). There was also no significant difference in the gender composition between the two groups of patients (P>0.05). From the total, 26 patients had been to Wuhan within 2 weeks before their hospitalization, and of theses 26 patients, 20 were moderate patients (57.1%) and 6 were severe patients (60.0%). There was no significant difference in Wuhan contact history between the two groups (P>0.05). Only 3 (6.7%) patients had ever visited the South China Seafood Market, and all 3 of these patients were moderate patients. It is worth noting that there were 4 patients with hypertension-based diseases, 3 of whom (30%) were severe patients. Of all the 45 patients, 40 (89%), 27 (60%), 19 (42%), 15 (33%), and 13 (28.9%) cases had fever, dry cough, fatigue, chills, and myalgia respectively, with no significant differences in symptoms between the two groups (P>0.05).
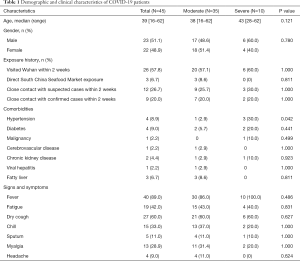
Full table
Hematology findings of COVID-19 patients
In total, 161 venous blood samples anticoagulated by EDTA-K2 were collected from the 45 patients between January 23, 2020 and February 13, 2020 in our laboratory. Among them, 131 venous blood samples were collected from 35 moderate patients, and the other 30 were collected from 10 severe patients. The hematology characteristics of those samples from the two groups are presented in Table 2.
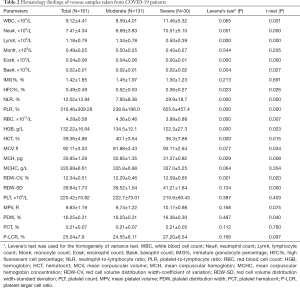
Full table
As the disease progressed, WBC, Neu#, NLR, PLR, RDW-CV and RDW-SD parameters in the severe group were significantly higher than those in the moderate group (P<0.05); meanwhile, Lym#, Eos#, HFC%, RBC, HGB and HCT parameters in the severe group were significantly lower than those in the moderate group (P<0.05). The scatter plots for the significant parameters are presented in Figure 1.
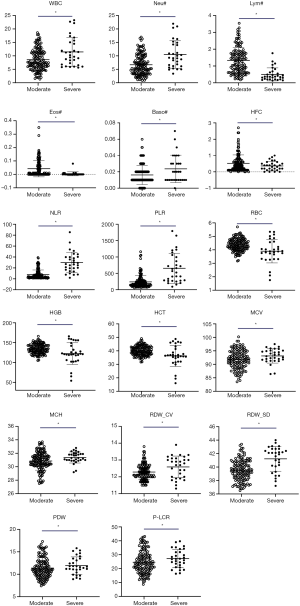
Biochemical and coagulation test findings of COVID-19 patients
The Biochemical and coagulation test results of those 45 patients between January 23, 2020 and February 13, 2020 in our laboratory are presented in Table 3. The biochemical and coagulation test samples were routinely collected and tested once every 1–3 days. As the disease progressed, Direct Bilirubin (DBil), Globulin (GLO), Blood Urea Nitrogen (BUN), Creatinine (Cr), Cystatin C (Cys C), Creatine Kinase (CK), Myoglobin(Mb), Lactate Dehydrogenase (LDH), and Fasting Blood Glucose (FBG) levels in the severe group were significantly higher than those in the moderate group (P<0.05); meanwhile, Albumin (ALB), Sodium (Na+) and Calcium(Ca2+) levels in the severe group were significantly lower than those in the moderate group (P<0.05). There was no significant difference in the coagulation test results between the two groups (P>0.05).
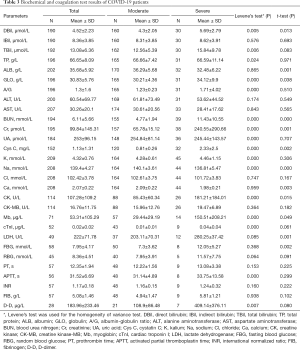
Full table
The ROC curve was used to analyze the hematological parameters with significant differences between the two groups. The parameters with AUC <0.6 and no statistical significance (P>0.05) with AUC =0.5 were excluded. Next, we analyzed the diagnostic efficacy of other hematology parameters (Table 4) in distinguishing moderate from severe COVID-19 cases. Taking the Youden index and the purpose of clinical screening, the best diagnostic cutoff was selected. The results showed that NLR was the best single parameter in distinguishing moderate from severe cases. For NLR parameter, its AUC, cut-off, sensitivity and specificity were 0.890, 13.39, 83.3% and 82.4% respectively when taking the Youden index and the purpose of clinical screening. Followed by PLR parameter, its AUC, cut-off, sensitivity and specificity of identifying moderate and severe COVID-19 patients were 0.842 267.03, 83.3% and 74.0% respectively. The combined parameters fitted by the LDA method were also used for the diagnostic efficacy analysis in the differentiation between the severe and the moderate groups. Among the parameters mentioned above, it was found that the combined parameters of NLR and RDW-SD, as generated by linear fitting of NLR and RDW-SD with the formula listed below Table 4, had the best diagnostic efficiency (AUC =0.938). When cut-off value was 1.046, the sensitivity for distinguishing the severe from the moderate cases of COVID-19 was 90.0% while the specificity was 84.7%. The second most effective parameter was fitting the combined parameter of NLR and RDW-CV (AUC =0.923). When the cut-off value was 0.62, the sensitivity of distinguishing the severe from the moderate cases of COVID-19 was 90.0% while the specificity was 82.4%. When the combined parameters of NLR and RDW-SD ≥1.046 or NLR and RDW-CV ≥0.62, it was more likely that the patient was a severe case. The combined parameters performed better than the single parameters, and are thus more useful for informing clinicians on the patient’s condition (Figure 2).
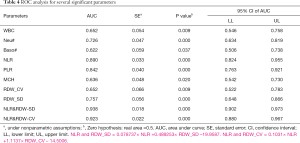
Full table
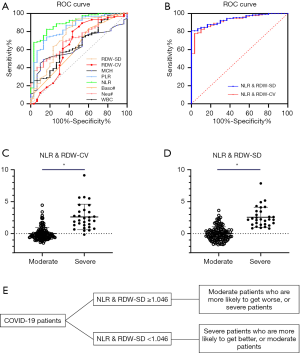
Meanwhile, we used the cutoff values of the best single-parameter NLR and the combined parameter of NLR and RDW-SD as the judgment thresholds and listed the four-fold tables (Tables 5,6) to calculate the other diagnostic items. It was found that the combined parameter of NLR and RDW-SD had better diagnostic performance (diagnostic accuracy rate =85.7%), predictive value, and likelihood ratio than the single parameter NLR (Table 7).
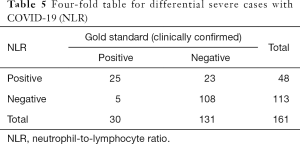
Full table
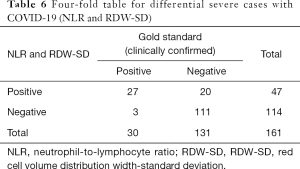
Full table
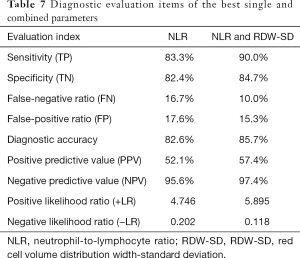
Full table
Discussion
SARS-CoV-2 belongs to the beta-type RNA coronavirus. Like SARS-CoV that caused the outbreak of SARS in 2003 and MERS-CoV that caused the outbreak of MERS in 2012, it is different from the 4 human coronaviruses that previously caused the common cold in humans, and can lead to severe respiratory diseases in humans (20). Although the current epidemiological situation shows that the SARS-CoV-2 is more contagious than SARS-CoV and MERS-CoV, with clusterings of outbreaks among people, its lethality is less severe (21-25). Compared with the outbreak of SARS in 2003 which caused 8,098 confirmed diagnoses and 774 deaths (mortality rate 9.6%) in 37 countries (5) and the outbreak of MERS in 2012 which caused 2,494 confirmed diagnoses and 858 deaths (mortality rate 34.4%) in 27 countries (7), the COVID-19 outbreak also showed a high mortality rate in the early stage in some areas. The first 41 confirmed infection cases admitted to hospitals in Wuhan from December 16, 2019 to January 2, 2020 showed that the COVID-19 patients had a 15% mortality rate at the beginning of the outbreak, and 32% of patients required ICU monitoring and treatment (11). As of the latest data published by the WHO on March 10, 2020, the mortality rates of COVID-19 patients in different countries and regions were 3.88% in China, 5.05% in Italy, 4.03% in the United States, 0.75% in South Korea, 3.81% in Iran, 2.73% in Spain, 2.14% in France, 1.75% in Japan, 0.93% in Britain, and 0.18% in Germany. The global mortality rate (including that of the Diamond Princess cruise ship) is 2.66% (12). Therefore, many experts believe that SARS-CoV-2 may coexist with humans for a long time (21), and the potential infection risk needs special attention with the increase in the number of asymptomatic infections (26). Marc Lipsitch, a professor of epidemiology at Harvard University, predicted that the SARS-CoV-2 would infect 40–70% of people worldwide by 2020 (27). Therefore, the tracking and screening of suspected cases are very important because not all COVID-19 patients have symptoms such as fever or dry cough in the early stage, and it is possible for some patients to develop into severe or critical cases. The current epidemic situation in China appears controlled, but the global epidemic outside China is still in the early to middle stages of the outbreak (22). Neither the detection capability of viral nucleic acid kits nor the popularity rate of computed tomography (CT) imaging (28) can support large-scale screening of all populations. Therefore, if the most conventional peripheral hematology test methods have characteristic changes or prompts for infected patients, especially those with severe infections, they will be very helpful for clinicians to intervene early to reduce the mortality of patients and relieve the pressure of the epidemic. Currently, the classification of patients with COVID-19 disease were performed according to the Diagnosis and Treatment Guidelines by clinicians (13), which depend on the following indicators: respiratory rate (RR), oxygen saturation, PaO2/FiO2 in the arterial blood, lung imaging (CT), and clinical indications, etc., all of which require a good medical environment.
This study reviewed the epidemiology of the underlying diseases and signs, along with changes in blood routine, biochemistry, and coagulation test results of 45 patients with different disease severities of SARS-CoV-2 infection in Jingzhou, Hubei province, China. There was no significant difference seen in the epidemiological findings between the two groups (P>0.05). For the underlying diseases, there were 4 patients with hypertension, 3 (30%) of whom were severe type. Of the 45 patients, 40 (89%) had fever, 27 (60%) had dry cough, 19 (42%) had fatigue, 15 (33%) had chills, and 13 (28.9%) had myalgia. Fever and dry cough were still the most common symptoms in patients with COVID-19. Although not many cases were reviewed, this trend is similar to that reported in the literature (29,30). In the comparison of hematological parameters, WBC, Neu#, NLR, PLR, RDW-CV, and RDW-SD in the severe group were significantly higher than those in the moderate group (P<0.05); meanwhile, Lym#, Eos#, HFC%, RBC, HGB, and HCT in the severe group were significantly lower than those in the moderate group (P<0.05). Among them, WBC and Neu # were significantly higher in severe patients. These results are consistent with the findings of Chen et al. (19) and may be related to persistent infection and prolonged hypoxia leading to compensatory hyperplasia of the bone marrow to release more granulocytes. The significant lymphopenia in the severe group may be caused by the SARS-CoV-2 continuing to invade more lymphocytes, proliferating and causing the lymphocytes to die or become depleted when they reach the spleen and other immune organs. This lymphopenia has been reported by many scholars (9,11,14,18,19,29,31,32). The same phenomenon was also seen in SARS-CoV and MERS-CoV infections (33,34). The LYM# trend we found is consistent with the description mentioned in the Diagnosis and Treatment Guidelines (Trial Version 6) (13). A study of 1,099 COVID-19 patients by the team led by Nanshan Zhong showed that the proportion of patients with lymphopenia reached 82.1% (19). There was no significant difference in platelet count between the moderate and severe patients (P>0.05). When SARS broke out in 2003 in Guangzhou, China, it was first reported that the while blood cell counts were normal or had decreased (80.2%), the lymphocytes and eosinophils decreased, monocytes increased, and thrombocytes decreased in some patients (35). Similarly, a report from Hong Kong indicated that of 157 patients, 153 (98%) had lymphopenia, 87 (55%) had thrombocytopenia, 77 (49%) had thrombocytosis, and 95 (61%) had hemoglobin decreased more than 20 g/L. The autopsy showed a decrease in lymphocytes in hemolymph organs, and multivariate analysis showed that old age and high concentration of LDH were independent predictors of poor prognosis. Lymphopenia and T lymphocyte subpopulation depletion may be related to the disease (36). The results of our study are similar those reported above (19,29,37). Lymphocytes and platelets are also important indicators for monitoring the peak viral load and immunopathological damage in the palliative treatment of lung disease with Abidol combined with lopinavir and ritonavir (38). In this study, both the ratio of NLR and PLR in the severe group were significantly higher (P<0.05), and showed the best single parameter differential diagnostic efficacy (NLR AUC =0.890, PLR AUC =0.842). Liu et al. (37) from Beijing Ditan Hospital Capital Medical University (Beijing, China) also suggested that NLR was helpful for early detection of severe COVID-19 patients and had a high prediction accuracy (AUC =0.849), which is consistent with the conclusion of this article. In addition, this study found that the red blood cell parameters (RBC, HGB, HCT) were significantly reduced in the severe group, while the morphological parameters (RDW-CV, RDW-SD) were significantly higher in the severe group than in moderate patients. This may arise from the immune damage that leads to the suppression of the bone marrow, resulting in the gradual increase of anemia which itself incurs a cascade of effects: compensatory hyperplasia of the erythroid cell line, a large number of immature red blood cells being released to the peripheral blood, and the activation of red blood cell apoptosis and peripheral phagocytosis. These phenomena ultimately result in the increase of the red blood cell distribution width (39). In the comparison of the biochemical and coagulation indexes tested, it was found that as the disease progressed, DBil, GLO, BUN, Cr, Cys C, CK, Mb, LDH, and FBG levels in the severe group were significantly higher those that in the moderate group (P<0.05); meanwhile, ALB, Na+, and Ca2+ levels in the severe group were significantly lower than those in the moderate group (P<0.05), which is also consistent with recent reports by most scholars (11,18,19,29,32).
According to the changing trend of NLR and RDW-SD, we used LDA to perform linear fitting on the different hematological parameters. Our selection of this approach had following rationale: When the development or diagnosis of a disease is affected by two mutually masked parameters, the diagnostic performance will be lower if only one parameter is used, as in the case where one parameter has high sensitivity while the other has high specificity. The fitting of the two different parameters by the formula, ax + by + c = w, into 1 combined parameter simultaneously possesses the advantages of the two parameters in specificity and sensitivity and can be more effectively used for the diagnosis and prediction of diseases. In this study, after a fitting analysis of different parameters, the NLR and RDW-SD combined parameter was selected as the best indicator to distinguish moderate COVID-19 patients from severe cases. The AUC reached to 0.938 while the diagnostic accuracy reached to 85.7%. The combined parameter can help clinicians prejudge the staging of patients and take effective treatment measures in advance. For the difference detection between moderate and severe cases, Tan et al. reported that lymphopenia can be used as an indicator of disease severity and prognosis of COVID-19 patients (18). Zhang et al. reported that age (AUC =0.819), ESR (AUC =0.951), D-D (0.892), ALB (0.760), and il-6 (0.695) are closely related to the disease severity of COVID-19 patients, and could be used as a valuable indicator for distinguishing severe COVID-19 patients in early stage, so as to increase the survival of severe patients (40). Li et al. reported SAA (AUC =0.856), CRP (0.850) and lymphocytes (AUC =0.909) are sensitive indicators in evaluating the severity and prognosis of COVID-19 (41). More and more laboratory indicators are used to identify and predict the severity of COVID-19 patients, which is expected to be of great help to clinicians.
This study is a single-center exploratory retrospective study, and there were only 45 included cases, with 35 and 10 moderate and severe patients, respectively. Fever and dry cough were the most common symptoms, and the ages ranged from 16 to 62 years old. Pregnant women, children the elderly, and asymptomatic patients were not included in the study. Therefore, the specific application of our findings needs to be verified and confirmed by more clinical cases. Furthermore, we did not analyze and study the prognosis of the included cases. However, this study suggests that it may be possible to identify severe patients through the most routine and basic of hematological tests in the early stages of COVID-19. This would potentially facilitate early clinical intervention for patients, thereby reducing patient mortality and to aiding in the control and prevention of the epidemic. We further believe that this study may have reference value for research and epidemic prevention in other countries and regions.
Conclusions
As the SARS-CoV-2 virus spreads globally, there is no clinically effective drug for COVID-19. Before the SARS-CoV-2 vaccine can pass clinical trials and be widely and safely applied (21), it is inevitable that more patients will develop into severe or even critical patients. Establishing an effective treatment strategy for severe and critical patients depends on early diagnosis and early warning of disease progression and is the key in reducing the overall mortality of patients with COVID-19 (31). This study found that the combined parameter of NLR and RDW-SD can be used as an indicator to distinguish moderate COVID-19 patients from severe cases. The AUC reached 0.938, and based on its optimal cut-off value (1.046), the diagnostic accuracy reached 85.7%, with a good positive and negative likelihood ratio. That is to say, if the results of combined NLR and RDW-SD of a COVID-19 patient exceed 1.046, it suggests that there is a greater possibility that the patient’s situation is more likely to get worse or the patient is more likely to be a severe patient. If the result of NLR and RDW-SD is lower than 1.046, it suggests that the patient is more likely get better or to be a moderate patient. This information will help clinicians to predict the severity and disease classification of patients, to take effective treatment measures in advance, to carry out differential treatment, and to more effectively control the epidemic.
Acknowledgments
We would like to thank all patients who participated in this study and all the healthcare workers in our hospital for their efforts in caring for these patients. We are also deeply grateful to all those who are fighting bravely against the COVID-19 epidemic.
Funding: None.
Footnote
Reporting Checklist: The authors have completed the STROBE reporting checklist. Available at http://dx.doi.org/10.21037/atm-20-3391
Data Sharing Statement: Available at http://dx.doi.org/10.21037/atm-20-3391
Conflicts of Interest: All authors have completed the ICMJE uniform disclosure form (available at http://dx.doi.org/10.21037/atm-20-3391). The authors have no conflicts of interest to declare.
Ethical Statement: The authors are accountable for all aspects of the work in ensuring that questions related to the accuracy or integrity of any part of the work are appropriately investigated and resolved. This study was approved by Ethics Committee of Jingzhou Central Hospital. As this study was a retrospective study, there was no patient privacy data such as patient name, ID number, telephone, and address collected. Only demographic information and laboratory testing data of patients were collected and analyzed, so there was no need for informed consent in this study.
Open Access Statement: This is an Open Access article distributed in accordance with the Creative Commons Attribution-NonCommercial-NoDerivs 4.0 International License (CC BY-NC-ND 4.0), which permits the non-commercial replication and distribution of the article with the strict proviso that no changes or edits are made and the original work is properly cited (including links to both the formal publication through the relevant DOI and the license). See: https://creativecommons.org/licenses/by-nc-nd/4.0/.
References
- Available online: http://www.chinacdc.cn/jkzt/crb/zl/szkb_11803/jszl_2275/202001/t20200121_211326.html
- Chan-Yeung M, Xu RH. SARS: epidemiology. Respirology 2003;8:S9-14. [Crossref] [PubMed]
- Kuiken T, Fouchier R, Schutten M, et al. Newly discovered coronavirus as the primary cause of severe acute respiratory syndrome. Lancet 2003;362:263-70. [Crossref] [PubMed]
- Drosten C, Günther S, Preiser W, et al. Identification of a Novel Coronavirus in Patients with Severe Acute Respiratory Syndrome. N Engl J Med 2003;348:1967-76. [Crossref] [PubMed]
- Chowell G, Castillo-Chavez C, Fenimore PW, et al. Model parameters and outbreak control for SARS. Emerg Infect Dis 2004;10:1258-63. [Crossref] [PubMed]
- de Groot RJ, Baker SC, Baric RS, et al. Middle East Respiratory Syndrome Coronavirus (MERS-CoV): Announcement of the Coronavirus Study Group. J Virol 2013;87:7790-2. [Crossref] [PubMed]
- Killerby ME, Biggs HM, Midgley CM, et al. Middle East respiratory syndrome coronavirus transmission. Emerg Infect Dis 2020;26:191-8. [Crossref] [PubMed]
- Zhu N, Zhang D, Wang W, et al. A Novel Coronavirus from Patients with Pneumonia in China, 2019. N Engl J Med 2020;382:727-33. [Crossref] [PubMed]
- Chen N, Zhou M, Dong X, et al. Epidemiological and clinical characteristics of 99 cases of 2019 novel coronavirus pneumonia in Wuhan, China: a descriptive study. Lancet 2020;395:507-13. [Crossref] [PubMed]
- Wang D, Hu B, Hu C, et al. Clinical Characteristics of 138 Hospitalized Patients With 2019 Novel Coronavirus–Infected Pneumonia in Wuhan, China. JAMA 2020;323:1061-9. [Crossref] [PubMed]
- Huang C, Wang Y, Li X, et al. Clinical features of patients infected with 2019 novel coronavirus in Wuhan, China. Lancet 2020;395:497-506. [Crossref] [PubMed]
- WHO. Coronavirus disease (COVID-2019) situation reports. Available online: https://www.who.int/emergencies/diseases/novel-coronavirus-2019/situation-reports.
- General Office of National Health Commission, Office of the State Administration of Traditional Chinese Medicine. New Coronavirus Pneumonia Diagnosis and Treatment Plan (Trial Version 6). Feb 18, 2020. Available online: http://www.nhc.gov.cn/yzygj/s7653p/202002/8334a8326dd94d329df351d7da8aefc2/files /b218cfeb1bc54639af227f922bf6b817.pdf
- National Health Council. Report of the WHO-China Joint Mission on Coronavirus Disease 2019 (COVID-19). Feb 29, 2020. Available online: http://www.nhc.gov.cn/jkj/s3578/202002/87fd92510d094e4b9bad597608f5cc2c/files/e73a238d8eff45d5ab855fa078f4c0dd.pdf
- Sun PF, Qie SY, Liu ZJ, et al. Clinical characteristics of 50466 patients with 2019-nCoV infection. medRxiv 2020. doi: https://doi.org/. [Crossref]
- Epidemiology Working Group for NCIP Epidemic Response. The epidemiological characteristics of an outbreak of 2019 novel coronavirus diseases (COVID-19) in China. Zhonghua Liu Xing Bing Xue Za Zhi 2020;41:145-51. [PubMed]
- Yang X, Yu Y, Xu J, et al. Clinical course and outcomes of critically ill patients with SARS-CoV-2 pneumonia in Wuhan, China: a single-centered, retrospective, observational study. Lancet Respir Med 2020;8:475-81. [Crossref] [PubMed]
- Tan L, Wang Q, Zhang DY, et al. Lymphopenia predicts disease severity of COVID-19: a descriptive and predictive study. medRxiv 2020. doi: https://doi.org/. [Crossref]
- Chen G, Wu D, Guo W, et al. Clinical and immunologic features in severe and moderate forms of Coronavirus Disease 2019. medRxiv 2020. doi: https://doi.org/. [Crossref]
- Ma YL. Research progress on the characteristics of coronavirus and its pathogenesis to human. Chin J Clin Infect Dis 2018;11:305-15.
- Gates B. Responding to Covid-19 - A Once-in-a-Century Pandemic? N Engl J Med 2020;382:1677-9. [Crossref] [PubMed]
- Pullano G, Pinotti F, Valdano E, et al. Novel coronavirus (2019-nCoV) early-stage importation risk to Europe, January 2020. Euro Surveill 2020. [Crossref] [PubMed]
- Gralinski LE, Menachery VD. Return of the Coronavirus: 2019-nCoV. Viruses 2020. [Crossref] [PubMed]
- Riou J, Althaus CL. Pattern of early human-to-human transmission of Wuhan 2019 novel coronavirus (2019-nCoV), December 2019 to January 2020. Euro Surveill 2020. [Crossref] [PubMed]
- Chan JF, Yuan S, Kok KH, et al. A familial cluster of pneumonia associated with the 2019 novel coronavirus indicating person-to-person transmission: a study of a family cluster. Lancet 2020;395:514-23. [Crossref] [PubMed]
- Luo SH, Liu W, Liu ZJ, et al. A confirmed asymptomatic carrier of 2019 novel coronavirus (SARS-CoV-2). Chin Med J 2020;35:100672.
- Coronavirus may infect up to 70% of world's population, expert warns. CBS NEWS March 2, 2020.
- Binnicker MJ. Emergence of a Novel Coronavirus Disease (COVID-19) and the Importance of Diagnostic Testing: Why Partnership between Clinical Laboratories, Public Health Agencies, and Industry Is Essential to Control the Outbreak. Clin Chem 2020;66:664-6. [Crossref] [PubMed]
- Guan WJ, Ni ZY, Hu Y, et al. Clinical characteristics of 2019 novel coronavirus infection in China. medRxiv 2020. doi: https://doi.org/. [Crossref]
- Zhao R, Liang YG, Lin YR, et al. Clinical characteristics of 28 patients with novel coronavirus pneumonia. Chin J Infect Dis 2020;38. doi: 10.3760/cma.j.issn.1000-6680.2020.02.007
- Diagnosis and Clinical Management of 2019 Novel Coronavirus (2019-nCoV) Infection: An Operational Recommendation of Peking Union Medical College Hospital (V2.0). Working Group of 2019 Novel.
- Shi Q, Zhao KL, Yu J, et al. Clinical characteristics of 101 non-surviving hospitalized patients with COVID-19- A single center, retrospective study. medRxiv 2020. doi: https://doi.org/. [Crossref]
- Yang M, Li C, Li K, et al. Hematological findings in SARS patients and possible mechanisms Int J Mol Med 2004;14:311-5. (Review). [PubMed]
- Assiri A, Al-Tawfiq JA, Al-Rabeeah AA, et al. Epidemiological, demographic, and clinical characteristics of 47 cases of Middle East respiratory syndrome coronavirus disease from Saudi Arabia: a descriptive study. Lancet Infect Dis 2013;13:752-61. [Crossref] [PubMed]
- Sun DH, Qiu YR, Zeng FY, et al. Blood routine characteristics of 96 cases of infectious atypical pneumonia in Guangzhou. J First Mil Med Univ 2005;25:1333-4.
- Wong RSM, Wu A, To KF, et al. Haematological manifestations in patients with severe acute respiratory syndrome: retrospective analysis. BMJ 2003;326:1358-62. [Crossref] [PubMed]
- Liu JY, Liu Y, Xiang P, et al. Neutrophil-to-Lymphocyte Ratio Predicts Severe Illness Patients with 2019 Novel Coronavirus in the Early Stage. medRxiv 2020. doi: https://doi.org/. [Crossref]
- Deng LS, Li CN, Zeng Q, et al. Arbidol combined with LPV/r versus LPV/r alone against Corona Virus Disease 2019: a retrospective cohort study. J Infec 2020. [Epub ahead of print].
- Kim CH, Park JT, Kim EJ, et al. An increase in red blood cell distribution width from baseline predicts mortality in patients with severe sepsis or septic shock. Critical Care 2013;17:R282. [Crossref] [PubMed]
- Zhang HZ, Wang XY, Fu ZQ, et al. Potential Factors for Prediction of Disease Severity of COVID-19 Patients. Medrxiv 2020. doi: 10.1101/2020.03.20.20039818. [Crossref]
- Li H, Xiang XC, Ren HW, et al. Serum amyloid A is a biomarker of severe coronavirus disease and poor prognosis. J Infec 2020. [Epub ahead of print].