Preoperative systemic immune-inflammation index and prognostic nutritional index predict prognosis of patients with pulmonary neuroendocrine tumors after surgical resection
Introduction
Lung cancer is one of the most common malignant tumors, which has attracted more and more attention due to its high morbidity and mortality. Pulmonary neuroendocrine tumors (PNETs) are a special subtype of lung cancer and their incidence is about 25% of primary lung cancer, and also account for 20–25% of primary NETs (1). Small cell lung cancer (SCLC), large cell neuroendocrine carcinoma (LCNEC), atypical carcinoid (AC) and typical carcinoid are recognized as PNETs. SCLC account for 20% of lung cancer, LCNEC 3%, AC 0.3% and typical carcinoid 2% (2,3). PNETs have a variety of clinical manifestations of pulmonary symptoms, and diagnosis is often delayed. A minority (3–5%) of patients may have hormone-related symptoms, but limited information for the diagnosis of PNETs (4). The treatment of PNETs is mainly based on surgical excision, while adjuvant treatment has therapeutic value for advanced patients (4). Nevertheless, the clinical progress of PNETs is not easy to predict due to the limitations of histopathologic value and the low number of histological or blood biomarkers that can effectively predict the prognosis (5).
The interaction between immune system and inflammation with cancer cells not only affects the occurrence, proliferation, development and metastasis of tumors (6-8), but also affects the treatment of malignant tumors, especially the use of immunotherapy for tumors (9,10). In recent years, biomarkers of inflammatory have attracted much attention. In addition to the finding that the neutrophil-lymphocyte ratio (NLR) and platelet-lymphocyte ratio (PLR) are effective for the early prognosis prediction of solid tumors, like hepatocellular carcinoma, oesophageal squamous cell carcinoma, breast cancer and colorectal cancer (11-17), researchers have also reported that the systemic immune-inflammation index (SII), which is a composite index integrating platelet counts, neutrophil and lymphocyte, can better predict the prognosis of solid neoplasms, such as oesophageal squamous cell carcinoma, hepatocellular carcinoma, and germ cell tumors (18-20). Additionally, the prognostic nutritional index (PNI) was found effectively in predicting the survival of colorectal cancer, oesophageal squamous cell carcinoma and other tumors by former researches (21-23). Similarly, NLR, PLR, SII and PNI were also found to have predictive value in NSCLC and SCLC after surgery. Researchers have also found that increases in the NLR, PLR, SII and PNI usually indicate a poor prognosis (24,25).
According to our best knowledge of the literature, we found that there are no relevant research reports on NLR, PLR, SII, PNI for predicting the prognosis of PNETs so far. To find new predictive indicators, we would like to focus on the predictive value of SII and PNI in the prognosis of PNETs through this retrospective study. We present the following article in accordance with the STROBE reporting checklist (available at http://dx.doi.org/10.21037/atm-19-4476).
Methods
Patients
The clinical records of 381 patients with surgically resected PNETs (143 LCNEC, 181 SCLC, and 57 AC) between 2005 and 2015 was retrospectively reviewed. Patients who met the following criteria were initially included in the study: (I) histopathological diagnosis of PNETs, which included LCNEC, SCLC, and AC; and (II) availability of preoperative serum laboratory results 5 days before operation. Patients with the following conditions will be excluded from the study: (I) the patient had accepted chemotherapy and/or radiotherapy before surgery; (II) the patient had chronic and/or acute infection; (III) the patient had haematological or autoimmune disease; (IV) the patient had hepatic disease or urinary disease; (V) the patient lacked detailed clinical information; and (VI) the patient was failed to postoperative follow-up. Of the 77 cases excluded, 15 cases accepted radiotherapy and/or chemotherapy before surgery; 20 cases had acute and/or chronic infection, haematological or autoimmune conditions; 7 cases lacked complete clinical data; and 35 cases were failed to post-operation follow-up. A total of 381 patients were included in this study at last (Figure 1).
All included subjects provided written informed consent before surgery. And this retrospective study was approved by the ethics committee of National Cancer Center/Cancer Hospital, Chinese Academy of Medical Sciences and Peking Union Medical College. All patients were recommended to have a follow-up visit every 3 months in the first 2 years, and half a year or once a year after 2 years. During the follow-up, the patient’s condition was recorded in detail, and physical examination and computed tomography were performed normally. In addition, some patients needed additional examination, like brain/bone scanning or PET-CT scanning. In this study, the deadline for follow-up was July 31, 2019, and the primary endpoint was the 5-year overall survival (OS).
Clinicopathological parameters
From clinical records, sex, age, smoking status, drinking history, histopathologic result, tumor size, TNM stage, lymph node metastasis status, distant metastasis status, intraoperative blood loss, treatment strategies and operation time of patients as clinicopathological parameters were included in our study. We assessed the histopathologic results, T stage, N stage, and M stage of tumors according to the 8th edition of the tumor, node, and metastasis (TNM) classification of lung cancer.
Blood sample analysis and PNI, SII, NLR, and PNI evaluations
We retrospectively extracted the laboratory data on complete blood count and plasma albumin from the patients’ medical records. Platelet count/lymphocyte count, neutrophil count/lymphocyte count, platelet count × neutrophil count/lymphocyte count and albumin level (g/L) + 5 × total lymphocyte count (109/L) were defined as PLR, NLR, SII and PNI respectively.
Statistical analysis
The statistical analysis of the data in our study through the SPSS version 23.0 (IBM Corp., Armonk, NY, USA). The receiver operating characteristic (ROC) curves was used to find out the cut-off values for PLR, NLR, SII and PNI with the highest Youden’s index. Categorical variables were compared using the Pearson Chi-square test. The Kaplan-Meier method was used for survival analysis through univariate analysis, and log-rank test was used to assess the difference. Cox regression model was used for multivariate analysis to explore the independent risk factors associated with PLR, NLR, SII and PNI. The correlation degree between the factors and OS was assessed by hazard ratios (HRs) and 95 percent confidence intervals (CIs). P value <0.05 was regarded as Statistical difference significantly.
Results
Samples’ characteristics
A total of 381 patients underwent surgery for PNETs, 294 (77.2%) patients were male and 87 (22.8%) patients were female. The median age was 60 years old, ranging from 19 to 94 years (Table 1). Among these patients, 266 patients (69.8%) had smoking experience, while 115 patients (30.2%) never smoked. The median maximum tumor diameter was 4 cm. Based on the pathologic results, there were 143 (37.5%) LCNEC, 181 (47.5%) SCLC and 57 (15.0%) AC tumors. Based on the standard of the 8th TNM staging system, 128 (33.6%), 150 (39.4%), 61 (16.0%) and 42 (11.0%) patients were classified as pT1, pT2, pT3 and pT4 disease, respectively. A total of 190 (49.9%), 86 (22.6%) and 105 (27.6%) patients were classified as pN0, pN1 and pN2, respectively. A total of 377 (99.0%) and four (1.0%) patients were classified as pM0 and pM1, respectively. In addition, 142 (37.3%) cases were in stage I, 93 (24.4%) cases were in stage II, 142 (37.3%) cases were in stage III, and only four (1.0%) cases were in stage IV. The median PLR, NLR, SII and PNI value were 134.6 (range, 42.31–427.1), 2.52 (range, 0.72–13.93), 592.3 (range, 108.04–3,765.8) and 51.78 (range, 32.75–66.35), respectively.
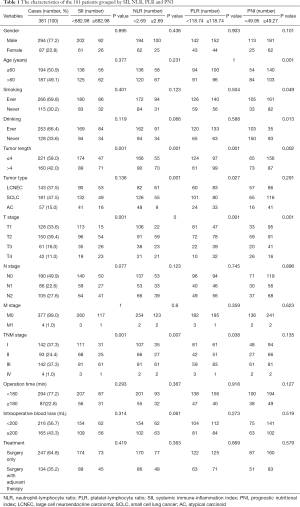
Full table
Optimal cut-off points for the PLR, NLR, SII and PNI
The optimal cut-off point of inflammation related indexes was determined by using ROC curve and OS as the end point. The optimal cut-off points for the prediction survival were 118.74, 2.69, 682.98 and 49.95 for PLR, NLR, SII and PNI, respectively. The area under the curve (AUC) for OS were 0.598, 0.611, 0.614, and 0.585 for PLR, NLR, SII and PNI, respectively (Figure 2). Therefore, based on the optimal cut-off value, we divided all patients into high-level group and low-level group. As shown in Table 1, 118 patients (31.00%) had SII ≥682.98, 125 patients (33.97%) had NLR ≥2.69, 196 patients (53.26%) had PLR ≥118.74, and 243 patients (66.03%) had PNI ≥49.27.
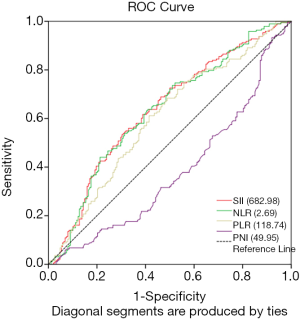
The relationship between the PLR, NLR, SII and PNI with characteristics of PNETs
As shown in Table 1, we take age, sex, smoking status, drinking history, tumor length, histopathological results, TNM stage, T stage, N stage, M stage, operation time, treatment strategies and intraoperative blood loss as important clinicopathological features to analyse their correlation with PLR, NLR, SII and PNI. Preoperative PLR has a significant correlation with tumor length (P=0.001), tumor type (P=0.027), T stage (P=0.001) and TNM stage (P=0.038). Preoperative NLR has a significant correlation with tumor length (P=0.001), tumor type (P=0.001), TNM stage (P=0.007), and T stage (P=0.000). preoperative SII has a significant correlation with T stage (P=0.001), tumor length (P=0.001) and TNM stage (P=0.001). Preoperative PNI has a significant correlation with age (P=0.001), smoking status (P=0.049), drinking status (P=0.013), tumor length (P=0.002), and T stage (P=0.001). However, the other parameters did not show statistical significance with PLR, NLR, SII and PNI.
Prognostic values of the PLR, NLR, SII and PNI for PNETs and subgroups
To explore whether SII, NLR, PLR and PNI affect the prognosis of pulmonary neuroendocrine carcinoma, we used Kaplan-Meier methodology to depict the 5 year OS of the 381 patients. The results showed that there was significant statistical difference between high SII and high NLR with poor prognosis (P=0.001 and P=0.001) (Figure 3A,B), while, low PLR and low PNI suggest poor prognosis (P=0.001 and P<0.001) (Figure 3C,D).
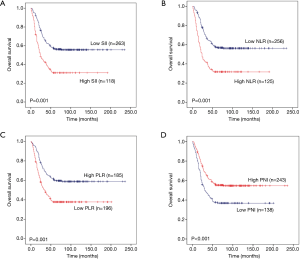
Then, we further analysed whether PLR, NLR, SII and PNI have predictive value in three subtypes of pulmonary neuroendocrine carcinoma (LCNEC, SCLC and AC). The total survival time of the three subgroups was also used Kaplan-Meier methodology and the log-rank test to describe. We found that high PLR, high NLR and high SII were significantly associated with worse 5-year OS (P=0.001, P=0.001 and P<0.001, respectively) for LCNEC (Figure 4A,B,C), while low PNI has significant statistical difference with worse 5-year OS (P=0.002) (Figure 4D). Elevated PLR, NLR and SII were significantly associated with poor 5-year OS of SCLC (P=0.003, P=0.001 and P=0.001, respectively) (Figure 5A,B,C), but this association does not exist with PNI (P=0.055) (Figure 5D). As shown in Figure 6A,B,C,D, SII, NLR, PLR and PNI were not significantly different for the OS values of AC patients.
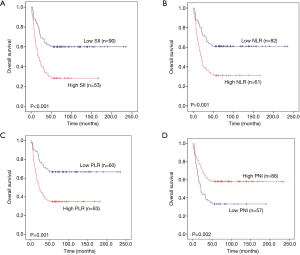
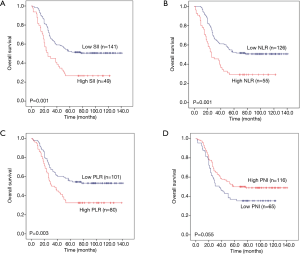
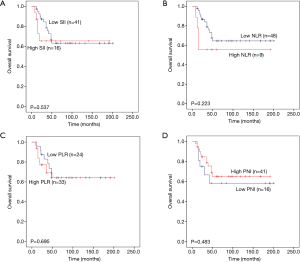
Predictive ability of coSII-PNI for the prognosis of PNETs and its subgroups
Considering that SII and PNI are two dimensional composite parameters, which have their own predictive value, in order to increase the accuracy and stability of prognosis prediction for PNETs and its subgroups, we further combine these two parameters, namely coSII-PNI. Elevated SII and reduced PNI were recorded as 0,Elevated SII and PNI or deduced SII and PNI were recorded as 1, and reduced SII and elevated PNI were recorded as 2. After calculated by the K-M curves and tested by log-rank test, the PNET patients were classified into three different groups according to the coSII-PNI values (P<0.001). In addition, both the subgroup of patients with coSII-PNI =1 and the subgroup of patients with coSII-PNI =2 have a better prognosis than the subgroup of patients with coSII-PNI =0 (P<0.001) (Figure 7A).
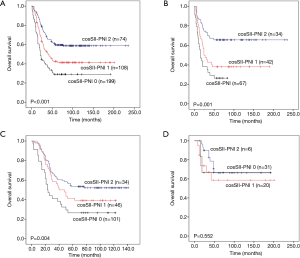
We then evaluated the prognostic value of CosII-PNI in patients with LCNEC, SCLC and AC. As shown in Figure 7B, for LCNEC patients, the coSII-PNI =1 group and the coSII-PNI =2 group have better prognosis than the coSII-PNI =0 group (P=0.001). Similarly, for SCLC patients, the coSII-PNI =1 group and the coSII-PNI =2 group have better prognosis than the coSII-PNI =0 group (P=0.004) (Figure 7C). However, this kind of statistical difference among the three subgroups was no found in AC patients from our study (P=0.552) (Figure 7D).
All patients were included in the follow-up plan after operation, and the follow-up deadline was death or to July 31, 2019. The median survival of these patients was 48 months, ranging from 1 to 235 months.
From Table 2, there were nine factors significantly associated with 5-year OS through the univariate Cox regression analysis. These factors included neoplasms size (P=0.002), T stage (P=0.001), lymph node status (P<0.001), TNM stage (P<0.001), operation time (P=0.028), PLR (P<0.001), NLR (P<0.001), SII (P<0.001) and PNI (P<0.001).
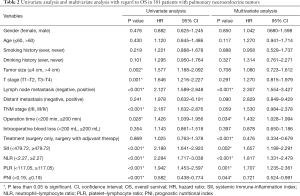
Full table
Moreover, in the multivariate analysis, lymph node metastasis (P<0.001), PLR (P=0.001), NLR (P<0.001), SII (P=0.002), treatment (P<0.001), operation time (P=0.034), and PNI (P=0.044) were found as independent risk factors for 5-year OS in this study.
Discussion
With the increasing incidence and morbidity of PNETs (26), there are not enough biomarkers to forecast the prognosis of patients, except Ki67, which can provide some prognostic value (27). Therefore, patients with high-risk recurrence factors or poor prognosis cannot be well identified before or after surgery and often miss the timeframe for appropriate and effective adjuvant therapy, which can improve the prognosis of patients (28). This indicates that there is an urgent requirement to seek effective prognostic biomarkers for positive and suitable treatment. Fortunately, NLR, PLR, SII and PNI were verified to have prognostic value for patients with PNETs after surgery in the current study. Although several studies have already confirmed that PLR, NLR, SII and PNI have predictive value in other solid tumors, which including hepatocellular carcinoma, oesophageal cancer and colorectal cancer (12,19,21,23,29), no similar study has focused on PNETs.
It is well known that to effectively apply these predictors in clinical practice, we need to determine the optimal cut-off point of the corresponding tumors for patient stratification. Previous studies on NSCLC calculated the appropriate values for SII, NLR, and PLR were 395.4–660, 1.9–3.57 and 108.0–147, respectively (30-33), and the optimal value for PNI was 45–52.95 (32,34,35). In current research, the optimal cut-off values for PLR, NLR, SII and PNI were 118.74, 2.69, 682.98 and 49.95, respectively. This finding indicates that the optimal cut-off values for PLR, NLR, SII and PNI in our study of PNETs are consistent with the results of NSCLC. In addition, the optimal cut-off values for PLR, NLR and SII in the research by Suzuki et al. on SCLC are similar to those in other studies (36) However, the optimal cut-off values for PLR, NLR and SII in the research by Hong et al. on SCLC are slightly higher than those in other studies, while the cut-off value for PNI is closer to that in previous studies (37).
Neutrophils, platelets and other cells can promote the malignant proliferation, invasion and drug resistance of tumors in local tumor environments. Besides, these immune-inflammatory cells also assist the extravasation of tumor cells, survival in peripheral blood and subsequent distant dissemination for tumor metastasis (38). Neutrophils are involved in enhancing the proliferation diffusion of cancer cells, and helping tumor cells escape from surveillance (39). Platelets deliver adenosine triphosphate to the circulation and promote tumorigenesis (40). Lymphocytes use their cytolytic activity to participate in the inhibition of tumor proliferation, and also recruit other immune cells to assist in this process (39,41). Therefore, lymphocytopenia is a marker of impaired immune surveillance and a favourable environment for the spread of tumors. This also explains the findings of our study. NLR, PLR and SII are mainly integrated inflammatory indicators consist of neutrophil, platelet and lymphocyte. Elevated SII and NLR were associated with worse prognosis in PNETs (P<0.05). The same results were found in the subgroups. High SII, high NLR and high PLR suggested poor prognosis. which is consistent with previous studies on hepatocellular carcinoma, lung cancer and other solid tumors (12,29). Compared with PLR and NLR, SII consists of three peripheral blood parameters. It can reflect the balance between host immunity and the inflammatory state comprehensively and is an objective index with good predictive reliability for prognosis. In addition, SII changes dynamically in tumor progression and treatment (12), so detection of SII can obtain information about host inflammation, immune response and clinical response to treatment. Therefore, SII index can work as an effective index to predict the prognosis of PNET patients.
Growing evidence suggests that the preoperative status, especially the nutritional and immune status, is associated with the general prognosis of aggressive tumors (42). PNI is calculated based on albumin and lymphocytes, which can reflect the inflammation status and nutritional status (43). Albumin plays a vital role in binding and transporting metabolites, scavenging free radicals, inhibiting platelet function and providing an anti-thrombosis effect (44). Malnutrition is often associated with hypoproteinaemia. It was found that IL-1, IL-6 and other cytokines play an important role in the formation of albumin, and also participate in neovascularization and tumor proliferation (45). In addition, different subtypes of lymphocytes have different effects on cancer, and some studies suggest that lymphocytes are involved in the cell-mediated immune destruction of cancer cells (46). This indicates that there is an important relationship between nutritional status and inflammatory status, and PNI is an important indicator of nutritional status and immune status. Previous studies found that low PNI predicts better survival in several solid tumors, like colorectal cancer, NSCLC and oesophageal carcinoma (21,23,32). For patients with PNETs, elevated PNI suggests that patients can survive longer. Moreover, in the LCNEC subgroup, elevated PNI also suggests a better prognosis of patients. However, in the SCLC and AC subgroups, elevated PNI did not show an advantage in patient OS (P>0.05). Perhaps this may be due to the limited number of patients.
Additionally, PLR, NLR, SII and PNI are all independent predictive indicators for PNETs through the univariate and multivariate survival analyses in our study. Considering that PNI has some limitations in the prognostic prediction of SCLC and AC, this study also assessed the predictive value of coSII-PNI index in patients with PNETs. And we found that in both LCNEC and SCLC subgroups, elevated PNI and reduced SII mean better prognosis, and vice versa. Therefore, the coSII-PNI index can be better used to predict the prognosis of PNET patients.
Recently, immunotherapy has made breakthroughs in cancer treatment, which makes the research on immune-specific biomarkers more urgent. It was found that there has a significant difference between elevated PLR and NLR levels with shorter OS and PFS in patients with advanced NSCLC treated with nivolumab (46). Pre-treatment PNI has also been found to be an independent predictive biomarker for NSCLC patients after treatment with immune checkpoint inhibitors and may help to identify which patients can obtain good therapeutic effect from immune checkpoint inhibitors (47). Although there is no similar study on PNETs, immunotherapy is gradually emerging in SCLC research. It was found that acetazolamide monoclonal antibody could improve OS and PFS of SCLC patients more effectively than chemotherapy alone (48). Therefore, NLR, PLR, SII and PNI will be potential biomarkers for predicting the future immunotherapy of PNETs based on SCLC.
However, there are several limitations in our research. First, because of the inherent limitations of retrospective studies and non multicenter research, the bias of patient selection and the differences between subgroups inevitably exist. Second, SII, NLR, PLR, PNI and coSII-PNI did not show prognostic value in the AC subgroup, which may be due to insufficient sample size included in the study, or to the fact that only AC was included in our study, but no typical carcinoid cases. A larger sample of studies on AC and typical carcinoid tumors will help to verify the predictive value of these inflammatory markers for prognosis. Third, owing to the lack of patient disease-free survival data, this study did not analyse the correlation between SII and PNI and patient disease-free survival. In addition, despite similar histopathological findings, LCNEC, AC, typical carcinoid and SCLC still have differences in incidence, treatment and prognosis, which need further study and analysis.
Conclusions
In conclusion, our study confirmed that PLR, NLR, SII and PNI have an effect predictive value for prognosis of patients with PNETs after surgery, specifically for those in the LCNEC and SCLC subgroups. However, there is no predictive value in AC. It is noteworthy that these inflammatory indicators have the advantages of low cost, simple calculation, good repeatability and easy implementation.
Acknowledgments
We thank all the staff in the Department of Thoracic Surgery for their support during the study.
Funding: This study was supported by the Institutional Fundamental Research Funds (2018PT32033), the National Key R&D Programme of China (2016YFC0905400, 2017YFC1308702), the Beijing Municipal Science & Technology Commission (Z151100004015188, Z181100001918002), the CAMS Initiative for Innovative Medicine (2017-I2M-1-005), the Beijing Hope Run Special Fund of Cancer Foundation of China (LC2019B15).
Footnote
Reporting Checklist: The authors have completed the STROBE reporting checklist. Available at http://dx.doi.org/10.21037/atm-19-4476
Data Sharing Statement: Available at http://dx.doi.org/10.21037/atm-19-4476
Conflicts of Interest: All authors have completed the ICMJE uniform disclosure form (available at http://dx.doi.org/10.21037/atm-19-4476). The authors have no conflicts of interest to declare.
Ethical Statement: The authors are accountable for all aspects of the work in ensuring that questions related to the accuracy or integrity of any part of the work are appropriately investigated and resolved. Our study protocol was reviewed and approved by the ethics committee of National Cancer Center/Cancer Hospital, Chinese Academy of Medical Sciences and Peking Union Medical College (approval number: 20/044-2240). Written informed consent was obtained from all subjects in our study.
Open Access Statement: This is an Open Access article distributed in accordance with the Creative Commons Attribution-NonCommercial-NoDerivs 4.0 International License (CC BY-NC-ND 4.0), which permits the non-commercial replication and distribution of the article with the strict proviso that no changes or edits are made and the original work is properly cited (including links to both the formal publication through the relevant DOI and the license). See: https://creativecommons.org/licenses/by-nc-nd/4.0/.
References
- Reed NS. Bronchopulmonary neuroendocrine tumours. Clin Oncol (R Coll Radiol) 2015;27:222-4. [Crossref] [PubMed]
- Öberg KOberg K, Hellman P, Ferolla P, et al. Neuroendocrine bronchial and thymic tumors: ESMO Clinical Practice Guidelines for diagnosis, treatment and follow-up. Ann Oncol 2012;23 Suppl 7:vii120-3. [Crossref] [PubMed]
- Travis WD, Giroux DJ, Chansky K, et al. The IASLC Lung Cancer Staging Project: proposals for the inclusion of broncho-pulmonary carcinoid tumors in the forthcoming (seventh) edition of the TNM Classification for Lung Cancer. J Thorac Oncol 2008;3:1213-23. [Crossref] [PubMed]
- Caplin ME, Baudin E, Ferolla P, et al. Pulmonary neuroendocrine (carcinoid) tumors: European Neuroendocrine Tumor Society expert consensus and recommendations for best practice for typical and atypical pulmonary carcinoids. Ann Oncol 2015;26:1604-20. [Crossref] [PubMed]
- Oberg K, Modlin IM, De Herder W, et al. Consensus on biomarkers for neuroendocrine tumour disease. The Lancet Oncol 2015;16:e435-46. [Crossref] [PubMed]
- McAllister SS, Weinberg RA. Tumor-host interactions: a far-reaching relationship. J Clin Oncol 2010;28:4022-8. [Crossref] [PubMed]
- Crusz SM, Balkwill FR. Inflammation and cancer: advances and new agents. Nat Rev Clin Oncol 2015;12:584-96. [Crossref] [PubMed]
- Tuomisto AE, Makinen MJ, Vayrynen JP. Systemic inflammation in colorectal cancer: Underlying factors, effects, and prognostic significance. World J Gastroenterol 2019;25:4383-404. [Crossref] [PubMed]
- Mellman I, Coukos G, Dranoff G. Cancer immunotherapy comes of age. Nature 2011;480:480-9. [Crossref] [PubMed]
- Smyth MJ, Ngiow SF, Ribas A, et al. Combination cancer immunotherapies tailored to the tumour microenvironment. Nat Rev Clin Oncol 2016;13:143-58. [Crossref] [PubMed]
- Gomez D, Farid S, Malik HZ, et al. Preoperative neutrophil-to-lymphocyte ratio as a prognostic predictor after curative resection for hepatocellular carcinoma. World J Surg 2008;32:1757-62. [Crossref] [PubMed]
- Wang BL, Tian L, Gao XH, et al. Dynamic change of the systemic immune inflammation index predicts the prognosis of patients with hepatocellular carcinoma after curative resection. Clin Chem Lab Med 2016;54:1963-9. [Crossref] [PubMed]
- Yodying H, Matsuda A, Miyashita M, et al. Prognostic Significance of Neutrophil-to-Lymphocyte Ratio and Platelet-to-Lymphocyte Ratio in Oncologic Outcomes of Esophageal Cancer: A Systematic Review and Meta-analysis. Ann Surg Oncol 2016;23:646-54. [Crossref] [PubMed]
- Zhu Y, Li M, Bo C, et al. Prognostic significance of the lymphocyte-to-monocyte ratio and the tumor-infiltrating lymphocyte to tumor-associated macrophage ratio in patients with stage T3N0M0 esophageal squamous cell carcinoma. Cancer Immunol Immunother 2017;66:343-54. [Crossref] [PubMed]
- Takeuchi H, Kawanaka H, Fukuyama S, et al. Comparison of the prognostic values of preoperative inflammation-based parameters in patients with breast cancer. PLoS One 2017;12:e0177137. [Crossref] [PubMed]
- Akgül ÖAkgul O, Cetinkaya E, Yalaza M, et al. Prognostic efficacy of inflammation-based markers in patients with curative colorectal cancer resection. World J Gastrointest Oncol 2017;9:300-7. [Crossref] [PubMed]
- Chen JH, Zhai ET, Yuan YJ, et al. Systemic immune-inflammation index for predicting prognosis of colorectal cancer. World J Gastroenterol 2017;23:6261-72. [Crossref] [PubMed]
- Hu B, Yang XR, Xu Y, et al. Systemic immune-inflammation index predicts prognosis of patients after curative resection for hepatocellular carcinoma. Clin Cancer Res 2014;20:6212-22. [Crossref] [PubMed]
- Wang L, Wang C, Wang J, et al. A novel systemic immune-inflammation index predicts survival and quality of life of patients after curative resection for esophageal squamous cell carcinoma. J Cancer Res Clin Oncol 2017;143:2077-86. [Crossref] [PubMed]
- Chovanec M, Cierna Z, Miskovska V, et al. Systemic immune-inflammation index in germ-cell tumours. Br J Cancer 2018;118:831-8. [Crossref] [PubMed]
- Noh GT, Han J, Cho MS, et al. Impact of the prognostic nutritional index on the recovery and long-term oncologic outcome of patients with colorectal cancer. J Cancer Res Clin Oncol 2017;143:1235-42. [Crossref] [PubMed]
- Hirahara N, Tajima Y, Fujii Y, et al. Preoperative Prognostic Nutritional Index Predicts Long-Term Surgical Outcomes in Patients with Esophageal Squamous Cell Carcinoma. World J Surg 2018;42:2199-208. [Crossref] [PubMed]
- Nakatani M, Migita K, Matsumoto S, et al. Prognostic Significance of the Prognostic Nutritional Index in Patients with Recurrent Esophageal Squamous Cell Carcinoma. Nutr Cancer 2018;70:467-73. [Crossref] [PubMed]
- Tomita M, Ayabe T, Maeda R, et al. Systemic immune-inflammation index predicts survival of patients after curative resection for non-small cell lung cancer. In Vivo 2018;32:663-7. [PubMed]
- Deng M, Ma X, Liang X, et al. Are pretreatment neutrophil-lymphocyte ratio and platelet-lymphocyte ratio useful in predicting the outcomes of patients with small-cell lung cancer? Oncotarget 2017;8:37200-7. [Crossref] [PubMed]
- Yao JC, Hassan M, Phan A, et al. One hundred years after "carcinoid": epidemiology of and prognostic factors for neuroendocrine tumors in 35,825 cases in the United States. J Clin Oncol 2008;26:3063-72. [Crossref] [PubMed]
- Rindi G, Klersy C, Inzani F, et al. Grading the neuroendocrine tumors of the lung: an evidence-based proposal. Endocr Relat Cancer 2013;21:1-16. [Crossref] [PubMed]
- Ramirez RA, Chauhan A, Gimenez J, et al. Management of pulmonary neuroendocrine tumors. Rev Endocr Metab Disord 2017;18:433-42. [Crossref] [PubMed]
- Wu G, Yao Y, Bai C, et al. Combination of platelet to lymphocyte ratio and neutrophil to lymphocyte ratio is a useful prognostic factor in advanced non-small cell lung cancer patients. Thorac Cancer 2015;6:275-87. [Crossref] [PubMed]
- Gao Y, Zhang H, Li Y, et al. Preoperative increased systemic immune-inflammation index predicts poor prognosis in patients with operable non-small cell lung cancer. Clin Chim Acta 2018;484:272-7. [Crossref] [PubMed]
- Guo D, Zhang J, Jing W, et al. Prognostic value of systemic immune-inflammation index in patients with advanced non-small cell lung cancer. Future Oncol 2018;14:2643-50. [Crossref] [PubMed]
- Tong YS, Tan J, Zhou XL, et al. Systemic immune-inflammation index predicting chemoradiation resistance and poor outcome in patients with stage III non-small cell lung cancer. J Transl Med 2017;15:221. [Crossref] [PubMed]
- Liu J, Li S, Zhang S, et al. Systemic immune-inflammation index, neutrophil-to-lymphocyte ratio, platelet-to-lymphocyte ratio can predict clinical outcomes in patients with metastatic non-small-cell lung cancer treated with nivolumab. J Clin Lab Anal 2019;33:e22964. [Crossref] [PubMed]
- Shimizu K, Okita R, Saisho S, et al. Preoperative neutrophil/lymphocyte ratio and prognostic nutritional index predict survival in patients with non-small cell lung cancer. World J Surg Oncol 2015;13:291. [Crossref] [PubMed]
- Park W, Lopes G. Perspectives: Neutrophil-to-lymphocyte Ratio as a Potential Biomarker in Immune Checkpoint Inhibitor for Non-Small-Cell Lung Cancer. Clin Lung Cancer 2019;20:143-7. [Crossref] [PubMed]
- Suzuki R, Wei X, Allen PK, et al. Prognostic Significance of Total Lymphocyte Count, Neutrophil-to-lymphocyte Ratio, and Platelet-to-lymphocyte Ratio in Limited-stage Small-cell Lung Cancer. Clin Lung Cancer 2019;20:117-23. [Crossref] [PubMed]
- Hong X, Cui B, Wang M, et al. Systemic immune-inflammation index, based on platelet counts and neutrophil-lymphocyte ratio, is useful for predicting prognosis in small cell lung cancer. Tohoku J Exp Med 2015;236:297-304. [Crossref] [PubMed]
- Diakos CI, Charles KA, McMillan DC, et al. Cancer-related inflammation and treatment effectiveness. The Lancet Oncology 2014;15:e493-e503. [Crossref] [PubMed]
- Mantovani A, Allavena P, Sica A, et al. Cancer-related inflammation. Nature 2008;454:436-44. [Crossref] [PubMed]
- Stanger BZ, Kahn ML. Platelets and tumor cells: a new form of border control. Cancer Cell 2013;24:9-11. [Crossref] [PubMed]
- Ferrone C, Dranoff G. Dual roles for immunity in gastrointestinal cancers. J Clin Oncol 2010;28:4045-51. [Crossref] [PubMed]
- Schwegler I, von Holzen A, Gutzwiller JP, et al. Nutritional risk is a clinical predictor of postoperative mortality and morbidity in surgery for colorectal cancer. Br J Surg 2010;97:92-7. [Crossref] [PubMed]
- Feng Z, Wen H, Ju X, et al. The preoperative prognostic nutritional index is a predictive and prognostic factor of high-grade serous ovarian cancer. BMC Cancer 2018;18:883. [Crossref] [PubMed]
- Don BR, Kaysen G. Serum albumin: relationship to inflammation and nutrition. Semin Dial 2004;17:432-7. [Crossref] [PubMed]
- Nazha B, Moussaly E, Zaarour M, et al. Hypoalbuminemia in colorectal cancer prognosis: Nutritional marker or inflammatory surrogate? World J Gastrointest Surg 2015;7:370-7. [Crossref] [PubMed]
- Diem S, Schmid S, Krapf M, et al. Neutrophil-to-Lymphocyte ratio (NLR) and Platelet-to-Lymphocyte ratio (PLR) as prognostic markers in patients with non-small cell lung cancer (NSCLC) treated with nivolumab. Lung Cancer 2017;111:176-81. [Crossref] [PubMed]
- Shoji F, Takeoka H, Kozuma Y, et al. Pretreatment prognostic nutritional index as a novel biomarker in non-small cell lung cancer patients treated with immune checkpoint inhibitors. Lung Cancer 2019;136:45-51. [Crossref] [PubMed]
- Horn L, Mansfield AS, Szczesna A, et al. First-Line Atezolizumab plus Chemotherapy in Extensive-Stage Small-Cell Lung Cancer. N Engl J Med 2018;379:2220-9. [Crossref] [PubMed]