Dual-mode ultrasound radiomics and intrinsic imaging phenotypes for diagnosis of lymph node lesions
Introduction
Lymph nodes are essential organs that regulate the core functions of the immune system. Lymph nodes are widely present throughout the human body and allow for the trapping and presentation of foreign antigens from peripheral tissues to prime the adaptive immune response (1). Lymph node lesions are divided into benign lesions and malignant lesions, of which, the malignant lesions are further subdivided into lymphoma and metastatic cancer (2). The precise diagnosis of benign lymph nodes, lymphomatous nodes, and metastatic nodes is critical for making decisions of clinical treatment. In the past, the diagnosis of lymph node lesions was usually through palpation, but clinically, some lymph nodes are often too small, deep, and inaccessible. Nowadays, the gold standard for evaluating the state of lymph nodes is pathology by biopsy. Lymph node biopsies are divided into needle biopsy and surgical excisional biopsy. However, excisional biopsy often causes wound infection, tumor spread, and complications, while needle biopsy has a risk of producing false negative (FN) results (3-5). Therefore, there is a need to develop an accurate, feasible, and noninvasive system for the assessment of lymph node status.
Imaging examinations, ultrasound, computed tomography, magnetic resonance imaging, among many others, play a crucial role in the differential diagnosis of lymph node lesions. Among all the imaging techniques, ultrasound has become the preferred method for lymph node examination due to its fast, painless, noninvasive, and convenient nature. Conventional B-mode ultrasound can evaluate lymph nodes and provide valuable structural information about the number, shape, size, boundary, and internal echogenicity of lymph nodes (6,7). Recently, another ultrasound modality, real-time elastography (RTE), has been applied for the assessment of tissue elasticity, which calculates and displays tissue strains in real time when operators compress and decompress tissues. Compared with conventional B-mode ultrasound, the RTE provides additional information concerning the biomechanical properties of lymph nodes (8-10).
In common clinical practice, the B-mode and RTE images are visually observed and graded by experienced radiologists for diagnosis (11,12); however, the diagnostic results suffer from large inter- and intra-observer variability because of the subjective nature of visual observation and the lack of a standard grading scheme for lymph nodes (13). Therefore, a computer-aided diagnosis (CAD) system is needed to reduce subjectivity and dependence of observers so that lymph nodes can be assessed more accurately and effectively.
Recent advancements in machine learning algorithms allow for a more accurate and more objective description of ultrasound images, which could be used to comprehensively assess lymph node size, shape, intensity, texture, and other less apparent features (14). Radiomics is a quantitative method that can enhance the objectiveness of image representation (15). Radiomics refers to quantitatively extracting high-throughput features from medical images and analyzing the features for disease diagnosis, prognosis, or treatment effect evaluation (14-16). Recently, intrinsic imaging phenotypes have been further derived from the high-throughput radiomics features using hierarchical clustering to depict distinct inherent patterns of diseases. It has been demonstrated that intrinsic imaging phenotypes are valuable for identifying distinct clinicopathological characteristics, molecular pathways, and patient prognoses (17-20).
At present, radiomics has been widely used in single-mode medical images, such as B-mode ultrasound or RTE, but combining it with dual-mode images remains a challenge. Dual-mode ultrasound radiomics combines the features of the 2 modalities rendering their information complementary, and thus improving the accuracy and disease classification performance of the quantitative analysis of the lesion (13,21). Working from this basis, we endeavored to create a dual-mode ultrasound radiomics method for CAD of benign, lymphomatous, and metastatic lymph nodes. We extracted the high-throughput radiomics features from dual-mode ultrasound images and select a few of the most important features by using computerized algorithms. We further produced intrinsic ultrasound imaging phenotypes of lymph nodes via the hierarchical clustering of the high-throughput radiomics features. We then combined the selected radiomics features with the imaging phenotypes to achieve an integrated CAD system for lymph node lesions. We hypothesized that the dual-mode radiomics features and intrinsic imaging phenotypes could capture distinct differences in various types of lymph nodes and may thus have a discriminative ability in lymph node classification.
We present the following article in accordance with the STARD reporting checklist (available at http://dx.doi.org/10.21037/atm-19-4630).
Methods
Figure 1 shows the framework of this study. First, radiomics features were extracted from B-mode and RTE images, including morphological features, intensity statistics, binary image features, gray level co-occurrence matrix (GLCM) texture features, and local variance (LV) texture features. Then, based on the extracted radiomics features, the least absolute shrinkage and selection operator (LASSO) method and the analysis of variance (ANOVA) method were used to select a few most important features. After that, the study got the intrinsic imaging phenotypes by using the hierarchical clustering of all radiomics features. Finally, the phenotypes were combined with the selected radiomics features for classification of benign, lymphomatous, and metastatic lymph nodes.
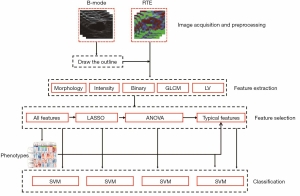
Image acquisition and preprocessing
From December 2013 to December 2016, 538 patients (53.26±14.39 years old; age range, 15–83 years) with suspicious lymph node enlargement, who underwent examination conventional ultrasound and RTE and subsequently underwent the ultrasound-guided core needle biopsy (US-CNB) to get pathological results, were enrolled in this study. In total, 543 lymph nodes were finally confirmed by pathology, where 142 were benign, 258 were lymphomatous, and 143 were metastatic. These lymph nodes were located in the armpit (281 cases), neck (203 cases), and groin (59 cases). Among the metastatic nodes, 131 originated from breast cancer, 4 from nasopharyngeal cancer, 3 from gastric cancer, 2 from skin cancer, 1 from malignant melanoma, 1 from lung cancer, and 1 from testicular cancer. The majority of cases were metastatic breast cancer because our hospital mainly specializes in the diagnosis and treatment of breast tumor. This retrospective study was approved by the Ethics Committee of Tongren Hospital (approval number Tongrenlunshen2018-046-02) and was carried out in adherence with the Declaration of Helsinki. The need for informed consent from all patients was waived due to the study’s retrospective nature.
We randomly divided the data set into a training set and a validation set in a 3:1 ratio. The training set included 107 benign, 193 lymphomatous, and 107 metastatic nodes, while the validation set included 35 benign, 65 lymphomatous, and 36 metastatic nodes.
Ultrasonography was performed by 1 radiologist with more than 5 years of experience in conventional ultrasound and 3 years in RTE. The L523 probe with a frequency of 4–13 MHz was used in the Mylab 90 system (Esaote, Genoa, Italy). The target lymph node was selected on the B-mode ultrasound and subsequently examined with the RTE. During the RTE examination, the radiologist manually applied slight axial compression to the lymph node using an ultrasound probe until the pressure indicator traced a sinus curve with 4–5 cycles and it was stabilized for 2 or 3 seconds (bottom right in Figure 2A). The imaging system provides dual-mode visualization in a full screen (Figure 2A), where the left part is a grayscale B-mode image, and the right is elastography. To obtain the region of interest, the lymph node boundary was delineated on the B-mode image (Figure 2B) by another radiologist with more than 5 years of experience in conventional ultrasound (Figure 2C), and the same radiologist performed all delineations. The elastography produces a composite color image displayed as a translucent color elastogram superimposed on the grayscale B-mode image (Figure 2D). According to the rule of pseudo-color coding in the Mylab 90 system, the color elastography was converted into a softness map, which was a grayscale image ranging from 0 (hardest) to 1 (softest) (Figure 2E) (13).
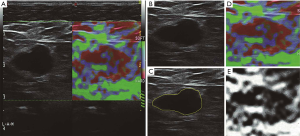
Feature extraction
We extracted a total of 430 features from B-mode and RTE images (223 from B-mode and 207 from RTE), including 16 morphological features, 132 intensity statistics, 142 binary image features, 120 GLCM texture features, and 20 LV texture features. Because the delineation of the lymph nodes was performed on the B-mode image, the morphological features were calculated solely on B-mode ultrasound. Other features were calculated on both modalities of the B-mode and RTE. If the extracted feature value was none, replace with 0.
The morphological features quantitatively depicted the shape of a lymph node. They included the area, convex area, long- and short-axis diameters, the ratio of long-to-short-axis diameter (L/S), equivalent diameter, perimeter, eccentricity, solidity, and orientation, in addition to the median and maximal thicknesses, and the mean, median, and maximal widths.
The intensity statistics represented the intensity distribution of image pixels within and outside a lymph node. They were calculated from the image intensities; i.e., the grayscale values on B-mode ultrasound or the softness values on RTE. They included the center deviation degree, the radial deviation degree (22), and a variety of first-order statistics within a lymph node, such as the mean, median, max, standard deviation, coefficient of variance, skewness, kurtosis, the entropy of histogram, the entropy of brightness (EnBrt), and several percentiles. Other features included the normalized mean (MeanNorm), a group of area ratios, and combined area ratios (23). The area ratio was the ratio of the area of the pixels with grayscales greater than the Otus’ threshold to the area of the entire lymph node.
The binary image features were calculated from a binary image that was converted from a B-mode image or an RTE softness map by a certain threshold. The pixel values larger than the threshold were set to 1 (white), and the others were set to 0 (black) in the binary image. The binary image features were then calculated as the proportion of white pixels within a lymph node. The thresholds ranged from 20 to 90 in an interval of 1.
The GLCM texture features were calculated based on the GLCM G(i, j) (24), which measured the co-occurrence frequency of a 2-pixel pair within a lymph node with intensities i and j at a specific relative direction and distance. To more efficiently represent the texture, the B-mode image or the RTE softness map was requantized to 8 intensities, and thus the size of GLCM was 8×8. The GLCM was normalized to acquire the joint conditional probability
In addition to the GLCM texture features, we also computed the LV texture features to further describe the heterogeneity of intensities in a lymph node. For a pixel inside a lymph node, its local area was defined as the area covering its neighboring pixels with a q-value determining the half-width of the neighborhood window (25). The local area variance for each pixel was defined as the variance of intensities within the local area of the pixel. We took q from 1 to 10, and the LV index (LVIq) of the entire lymph node was calculated as the following:
| [1] |
where VOV was the variance of the local area variances for all pixels within the entire node, and MOV was the mean of the local area variances.
Feature selection
Correlation and redundancy exist between high dimensional features, and these redundant features lead to over-fitting and the reduction of classification accuracies. Therefore, a few of the most informative and important features for discriminating between different types of lymph nodes need to be selected to acquire an optimized subset. In this study, the LASSO method and the ANOVA method were combined to select the features; we named the combined method as the LASSO + ANOVA method. We performed LASSO + ANOVA in the training set, recorded the selected features, and then applied them to the validation set.
The LASSO method, which is suitable for the regression of high-dimensional data, was used to select the most useful predictive features from the data set (26). It compressed the regression coefficients by using the absolute value function of the model coefficients as a penalty, and thereby screened the feature subset from the data set of lymph nodes with a strong discriminating ability.
The feature subset selected with the LASSO method was further optimized by using the ANOVA. That is, the features with P values of ANOVA less than 0.001 were further selected.
Identification of intrinsic imaging phenotypes
Hierarchical clustering has been widely adopted in genomics, where the gene expression data is used (27-29). Here, we propose employing hierarchical clustering to identify the intrinsic ultrasound imaging phenotypes in lymph nodes. We performed unsupervised hierarchical clustering on the feature vector consisting of all 430 features of the training set. We obtained the hierarchical clusters that were represented by a dendrogram (30,31), which is a multilevel hierarchy in which clusters at 1 level are joined to form clusters at the next level. The generation of the dendrogram is described as follows. Given the training set of the lymph nodes, each lymph node was represented by a 430-dimensional standardized feature vector [i.e., z-score normalized features (32)]. Each lymph node was regarded as a tree node of the dendrogram, and Pearson’s correlation was calculated between every pair of lymph nodes. The pair with the highest correlation was merged, and a new parent tree node was created; the correlations among the feature vectors were then updated, and the process was repeated until a single node remained (18).
From the dendrogram, the lymph nodes were divided into several non-overlap clusters called imaging phenotypes (18). We initially grouped lymph nodes into 10 clusters, in which 2 clusters included samples lower than 5, and they were merged into their neighboring clusters. Thus finally, there were 8 total clusters of imaging phenotypes generated. Phenotype order was determined in descending order of the percentage of the benign samples in each phenotype (Table 1).
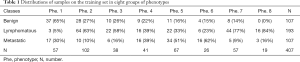
Full table
We used the hierarchical clustering to produce a heat map matrix for visualizing the properties of the detected imaging phenotypes (Figure 3) (33). In the heat map matrix, each column represented a 430-dimensional standardized feature vector for each lymph node, and each row represented 1 feature across all training samples.
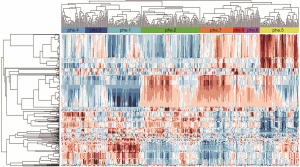
A Pearson’s correlation was calculated between each validation sample and each training sample. For a validation sample, its corresponding phenotype was determined by the largest Pearson’s correlation calculated between the validation sample and all training samples.
Classification
By utilizing the support vector machine (SVM) as the computerized classifier, we compared 3 sets of radiomics features for classification of lymph nodes: (I) all features, (II) features selected by using the LASSO method, and (III) features selected by using the LASSO + ANOVA method. The classification tasks covered the following 4 circumstances: benign vs. lymphomatous, benign vs. metastatic, lymphomatous vs. metastatic, and benign vs. malignant (lymphomatous + metastatic). The differences between each 2 classes were examined with the Kruskal-Wallis test to determine the non-normal distribution of the variables. In disease classification, according to the combination of the real category and predicted category, there are 4 kinds of situations: true positive (TP), false positive (FP), true negative (TN), and FN. The accuracy of classification can be measured by accuracy (ACC). ACC is defined as follows:
| [2] |
However, in clinical practice, sensitivity (SEN), specificity (SPC), and Youden’s index (YI) are more suitable for being evaluation indicators. They are defined as the following:
| [3] |
| [4] |
| [5] |
For a specific feature, if its SEN increased, then its SPC is reduced (34,35). Therefore, an indicator that can measure the performance comprehensively is need. In this case, drawing a receiver operating characteristic (ROC) curve is the best solution. The ROC curve reflects the relationship between SEN and SPC (36). Its abscissa (X) and ordinate (Y) are defined as follows:
| [6] |
| [7] |
The ROC curve generates a set of key points (X, Y) on the curve by constantly moving the “cutoff” of the classifier. The best cutoff value is when the YI value reaches the maximum value. According to the position of the curve, the entire graph is divided into 2 parts. The area under the curve is called AUC, and this can intuitively evaluate the quality of the classifier (Figure 4). The larger the AUC values, the better the performance of classification (36).
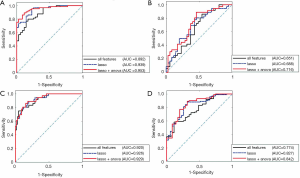
The AUC values from the validation set were computed for the intrinsic imaging phenotypes and for each radiomics feature selected with the LASSO + ANOVA. Furthermore, we used the cutoff values for each feature obtained from the training set to yield its final SENs, SPCs, ACCs, and YIs at the validation set. The phenotypes were combined with each feature selected with the LASSO + ANOVA, and the classification results were compared between the features combined and not combined with phenotypes.
All computerized image interpretations, including the preprocessing, feature extraction, feature selection, identification of imaging phenotypes, and lymph node classification, in addition to the statistical analyses and validation, were performed by using programs implemented with the MATLAB software (MathWorks, Natick, MA, USA).
Results
Classification results of three feature sets
Based on all 430 features, the AUC values of the validation set were given as follows: 0.892 for benign vs. lymphomatous, 0.651 for benign vs. metastatic, 0.920 for lymphomatous vs. metastatic, and 0.774 for benign vs. malignant. The ACC values of the validation set were 80.00% for benign vs. lymphomatous, 57.75% for benign vs. metastatic, 80.20% for lymphomatous vs. metastatic, and 68.38% for benign vs. malignant (Table 2).
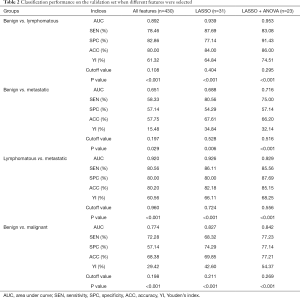
Full table
From the 430 features, 31 features were selected with the LASSO method. By using the selected features, the AUC values of the validation set were 0.939 for benign vs. lymphomatous, 0.688 for benign vs. metastatic, 0.926 for lymphomatous vs. metastatic, and 0.827 for benign vs. malignant. The ACC values were 84.00% for benign vs. lymphomatous, 67.61% for benign vs. metastatic, 82.18% for lymphomatous vs. metastatic, and 69.85% for benign vs. malignant. Compared with all 430 features, the LASSO-selected features improved the AUC values of benign vs. lymphomatous, benign vs. metastatic, lymphomatous vs. metastatic and benign vs. malignant by 4.70%, 3.70%, 0.60%, and 5.30%, respectively; meanwhile, they improved the ACC-value of the 4 above classification tasks by 4.00%, 9.86%, 1.98%, and 1.47%, respectively (Table 2).
We further performed the ANOVA on the LASSO-selected features and finally selected 23 features with the LASSO + ANOVA method to obtain an optimal feature subset. The AUC values of the validation set were 0.953 for benign vs. lymphomatous, 0.716 for benign vs. metastatic, 0.929 for lymphomatous vs. metastatic, and 0.842 for benign vs. malignant. The ACC values were 86.00% for benign vs. lymphomatous, 66.20% for benign vs. metastatic, 85.15% for lymphomatous vs. metastatic, and 77.21% for benign vs. malignant (Table 2).
By using the LASSO + ANOVA method, the P values for all 4 classification tasks were less than 0.001 (Table 2). Compared with all 430 features, the features selected with the LASSO + ANOVA improved the AUC values for classification tasks of benign vs. lymphomatous, benign vs. metastatic, lymphomatous vs. metastatic, and benign vs. malignant by 6.10%, 6.50%, 0.90%, and 6.80%, respectively. Compared with the LASSO-selected features, the features selected with the LASSO + ANOVA improved the AUC values of the above 4 classification tasks by 1.40%, 2.80%, 0.30%, and 1.50%, respectively; meanwhile, they improved the ACC values of benign vs. lymphomatous, lymphomatous vs. metastatic, and benign vs. malignant by 2.00%, 2.97%, and 7.36%, respectively (Table 2).
In summary, by using the LASSO + ANOVA method, a preferable radiomics feature subset was obtained for the classification of lymph node lesions.
Typical features selected with LASSO + ANOVA
Table 3 lists 7 typical features among the LASSO + ANOVA-selected features, including 5 features from B-mode and 2 features from RTE. The definitions and implications are also given in Table 3. The medians and interquartile ranges (IQR) of these typical features, along with their P values from the Kruskal-Wallis test, are shown in Table 4, while the classification results are shown in Table 5.
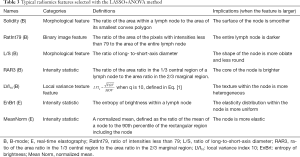
Full table
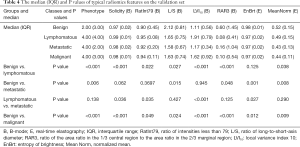
Full table
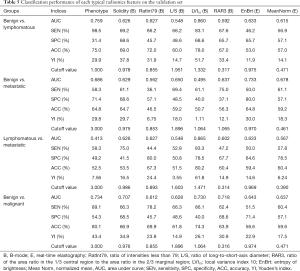
Full table
Results of intrinsic imaging phenotypes
We obtained 8 phenotypes on the validation set as categorical features and calculated their corresponding P values (Table 4), cut-off values, AUCs, SENs, SPCs, ACCs, and YIs (Table 5). There were significant differences between benign and lymphomatous lymph nodes (P<0.001), benign and metastatic lymph nodes (P=0.006), and benign and malignant lymph nodes (P<0.001). However, there was no difference between the lymphomatous and metastatic lymph nodes (P=0.138). The AUC values on the validation set were 0.759 for benign vs. lymphomatous, 0.686 for benign vs. metastatic, 0.413 for lymphomatous vs. metastatic, and 0.734 for benign vs. malignant.
Results of combining radiomics features with phenotypes
The phenotypes were combined with each typical radiomics feature selected with the LASSO + ANOVA. The results are presented in Table 6. Compared with a single radiomics feature alone, the combination of the phenotypes and a single feature increased the AUCs of benign vs. lymphomatous by 0.30–24.90%, benign vs. metastatic by 1.30–18.80%, and benign vs. malignant by 0.30–9.50%. Finally, all 23 radiomics features selected with the LASSO + ANOVA were combined with the phenotypes, and the AUC values were 0.960 for benign vs. lymphomatous, 0.716 for benign vs. metastatic, 0.933 for lymphomatous vs. metastatic, and 0.856 for benign vs. malignant.
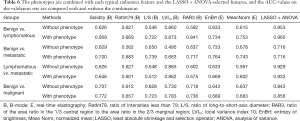
Full table
Discussion
In recent years, a few advancements have been made in the study of lymph node differentiation. Zakaria et al. studied 177 cervical lymph nodes in 128 children, using a histological assessment of lymph nodes through fine-needle aspiration cytology (FNAC) to compare the histology results with the features of weather B-mode, Doppler, and elastography. The results showed that elastography has high accuracy in distinguishing benign from malignant nodes, reaching 90.23% (37). Latif et al. evaluated the role of B-mode ultrasound, elastography, and diffusion-weighted imaging (DWI) in differentiating benign and malignant axillary lymph nodes. ROC analysis was constructed to obtain the best cutoff values for B-mode criteria, elasticity score, strain ratio, and ADC value. The results showed that DWI and elastography significantly aided in improving the characterization of metastatic axillary nodes when compared with B-mode ultrasound (38). However, only two types of lymph nodes, benign and malignant, were classified in these studies. Also, the features used for the evaluation of the studies relied on the individual experience of the investigators, which might have led to high subjectivity and large inter- and intra-observer variability. Liu et al. used radiomics features from sonoelastography and B-mode ultrasound to objectively diagnose the lymph node metastasis of papillary thyroid carcinoma with an accuracy of 85% (39). However, unlike in our study, their diagnosis only looked at the images of the thyroid tumors themselves and not those of the lymph nodes, and was also limited to examining the benign vs. metastatic distinction. In 2017, our research team applied radiomics to classify the benign and malignant axillary lymph nodes in ultrasound images, and achieved good results (40). The present study further studied the classification of multiple types of lymph nodes. Compared with the previous study, we collected more lymph node data, and optimized the algorithm of feature extraction and feature selection. Although the clustering algorithm has been used in earlier research, the concept of phenotype was not included, and this addition of phenotype is helpful in describing the inherent pattern of disease and improving the whole classification system.
To our knowledge, our current study is the first to use computer-assisted methods to extract dual-mode ultrasound radiomics features from lymph nodes for differentiating various types of lymph node lesions, and includes 4 distinctions: benign vs. lymphomatous, benign vs. metastatic, lymphomatous vs. metastatic, and benign vs. malignant. We also combined the radiomics features with the intrinsic imaging phenotypes from hierarchical clustering to enhance the classification performance. To some extent, this method realizes the combination of supervised and unsupervised learning. The results of this study have demonstrated that our CAD system can reduce FNs and FPs and thus may help to effectively diagnose 3 types of lymph nodes and assist in clinical decision-making.
We used a radiomics approach on dual-mode ultrasound for generating high-throughput quantitative features and obtained the subsets of optimized features with the LASSO method and the LASSO + ANOVA method. The features selected with the LASSO + ANOVA achieved better results than all non-selected and LASSO-selected features, indicating the superiority of the LASSO + ANOVA feature selection.
When visually interpreting B-mode ultrasound images, radiologists mainly consider the size, shape, echo uniformity, and boundary of lymph nodes (41); when interpreting elastograms, they mainly consider the overall hardness of the lesions and the heterogeneity of the hardness (42,43). Based on these visual experiences from radiologists, we list in Table 3 a few typical features that have been selected by the LASSO + ANOVA. These typical features have medically explainable meanings that may be useful for revealing characteristics of lymph nodes and thus may help diagnosis. For example, on B-mode ultrasound, benign lymph nodes tend to be elliptical with the long-to-short axis ratios (L/S) greater than 2.0, while metastatic nodes are round in shape (L/S <2.0) (44,45). Malignant lymph nodes tend to be more heterogeneous in B-mode intensities and have more mixed textures, resulting in higher LVI10 (25). Benign lymph nodes tend to have stronger echogenicity than malignant lymph nodes, especially lymphomas, and thus the benign nodes have lower RatInt79 values. The core areas of benign lymph nodes tend to be brighter than those of malignant nodes, and thus they have higher RAR3 values. On elastograms, benign lymph nodes are more elastic, and malignant nodes are stiffer (13), which is consistent with the higher MeanNorm values found in benign nodes. In short, these typical radiomics features have meaningful diagnostic capability for lymph nodes.
The unsupervised hierarchical clustering offers a method for detecting intrinsic imaging phenotypes. In our study, along the columns (samples) of the data matrix, the hierarchical clustering agglomerated the samples into 8 clusters, and each cluster was treated as an imaging phenotype. This method is similar to the hierarchical clustering analysis of gene expression data that may discover the intrinsic molecular subtypes of tumors (18). It is indicated from Table 6 that the combination of the phenotypes and the radiomics features was helpful in improving the AUC values for diagnostic tasks of benign vs. lymphomatous, benign vs. metastatic, and benign vs. malignant. However, the combination did not meaningfully improve the lymphomatous vs. metastatic task. This may be explained by the fact that the order of the phenotypes was determined by the descending order of the percentage of the benign samples in each phenotype (Table 1); hence, the phenotypes could not adequately distinguish between lymphoma and metastatic nodes (P=0.138; Table 4). More complex phenotypes need to be established in the future for better discrimination between lymphomas and metastatic lymph nodes.
There are some limitations and areas for further work. First, the size of samples was small, and the distribution of samples was unbalanced across the 3 types of lymph nodes. However, this preliminary yet promising study warrants future studies with large samples. Second, the lymph node boundaries were manually delineated on B-mode images by an experienced radiologist. We plan to propose an automated method for lymph node segmentation based on convolutional neural networks to more objectively and reproducibly assess the lymph node lesions (46-48). Third, although more features can be obtained in dual mode ultrasound radiomics than the individual mode, it is inevitable that some features are useless and redundant. In this paper, the best and most suitable feature selection method was adopted, but there were still some features without statistical significance that could have been ignored. Therefore, in a future study, we will focus more on advanced statistical methods and machine learning methods to perform more effective dimensionality reduction and feature screening on data. Fourth, this study focused on B-mode and elastic ultrasound, while other ultrasound modes, such as Doppler ultrasound, contrast-enhanced ultrasound, and shear-wave elastography, are also effective methods for diagnosing lymph nodes (9). We also only acquired dual-mode images from 1 ultrasound imaging device, and our CAD methods were tailored for this device. In the future, more ultrasound modalities will be combined, and images from multiple devices at multiple centers will be enrolled to develop a more comprehensive CAD system (49).
Conclusions
We proposed a dual-mode radiomics approach on both B-mode ultrasound and sonoelastography for generating high-throughput quantitative features of lymph nodes, from which an optimized feature subset is selected with the LASSO + ANOVA method. Meanwhile, the intrinsic ultrasound imaging phenotypes are obtained by using hierarchical clustering. The dual-mode radiomics features and imaging phenotypes can capture distinctions between benign, lymphomatous, and metastatic lymph nodes and are valuable in lymph node discrimination.
Acknowledgments
Funding: This work was supported by the National Natural Science Foundation of China (grant numbers 61671281, 61911530249, and 81627804).
Footnote
Reporting Checklist: The authors have completed the STARD reporting checklist. Available at http://dx.doi.org/10.21037/atm-19-4630
Data Sharing Statement: Available at http://dx.doi.org/10.21037/atm-19-4630
Conflicts of Interest: All authors have completed the ICMJE uniform disclosure form (available at http://dx.doi.org/10.21037/atm-19-4630). The authors have no conflicts of interest to declare.
Ethical Statement: The authors are accountable for all aspects of the work in ensuring that questions related to the accuracy or integrity of any part of the work are appropriately investigated and resolved. The study was conducted in accordance with the Declaration of Helsinki (as revised in 2013) and approved by the Ethics Committee of Tongren Hospital (approval number Tongrenlunshen 2018-046-02). The need for informed consent from all patients was waived due to the study’s retrospective nature.
Open Access Statement: This is an Open Access article distributed in accordance with the Creative Commons Attribution-NonCommercial-NoDerivs 4.0 International License (CC BY-NC-ND 4.0), which permits the non-commercial replication and distribution of the article with the strict proviso that no changes or edits are made and the original work is properly cited (including links to both the formal publication through the relevant DOI and the license). See: https://creativecommons.org/licenses/by-nc-nd/4.0/.
References
- Liou HLR, Myers JT, Barkauskas DS, et al. Intravital Imaging of the Mouse Popliteal Lymph Node. J Vis Exp 2012;60:e3720. [PubMed]
- Ahuja AT, Ying M, Ho SY, et al. Ultrasound of malignant cervical lymph nodes. Cancer Imaging 2008;8:48-56. [Crossref] [PubMed]
- Wong SL, Faries MB, Kennedy EB, et al. Sentinel Lymph Node Biopsy and Management of Regional Lymph Nodes in Melanoma: American Society of Clinical Oncology and Society of Surgical Oncology Clinical Practice Guideline Update. J Clin Oncol 2018;36:399-413. [Crossref] [PubMed]
- Deurloo E, Tanis P, Gilhuijs KG, et al. Reduction in the number of sentinel lymph node procedures by preoperative ultrasonography of the axilla in breast cancer. Eur J Cancer 2003;39:1068-73. [Crossref] [PubMed]
- Nielsen Moody A, Bull J, Culpan AM, et al. Preoperative sentinel lymph node identification, biopsy and localisation using contrast enhanced ultrasound (CEUS) in patients with breast cancer: a systematic review and meta-analysis. Clin Radiol 2017;72:959-71. [Crossref] [PubMed]
- Li Q, Wang L, Wu H, et al. Controlled Study of Traditional Ultrasound and Ultrasound Elastography on the Diagnosis of Breast Masses. Ultrasound Q 2015;31:250-4. [Crossref] [PubMed]
- Dietrich CF, Mathis G, Cui XW, et al. Ultrasound of the Pleurae and Lungs. Ultrasound Med Biol 2015;41:351-65. [Crossref] [PubMed]
- Qian X, Ma T, Shih CC, et al. Ultrasonic Microelastography to Assess Biomechanical Properties of the Cornea. IEEE Trans Biomed Eng 2019;66:647-55. [Crossref] [PubMed]
- Zhang Q, Xiao Y, Dai W, et al. Deep learning based classification of breast tumors with shear-wave elastography. Ultrasonics 2016;72:150-7. [Crossref] [PubMed]
- Chen Z, Chen Y, Huang Q. Development of a Wireless and Near Real-Time 3D Ultrasound Strain Imaging System. IEEE Trans Biomed Circuits Syst 2016;10:394-403. [Crossref] [PubMed]
- Adamietz BR, Meier-Meitinger M, Fasching P, et al. New diagnostic criteria in real-time elastography for the assessment of breast lesions. Ultraschall Med 2011;32:67-73. [Crossref] [PubMed]
- Mansour N, Bas M, Stock KF, et al. Multimodal Ultrasonographic Pathway of Parotid Gland Lesions. Multimodaler sonografischer Diagnosepfad für Parotisläsionen. Ultraschall Med 2017;38:166-73. [PubMed]
- Zhang Q, Suo J, Chang W, et al. Dual-modal computer-assisted evaluation of axillary lymph node metastasis in breast cancer patients on both real-time elastography and B-mode ultrasound. Eur J Radiol 2017;95:66-74. [Crossref] [PubMed]
- Zhang Q, Xiao Y, Suo J, et al. Sonoelastomics for Breast Tumor Classification: A Radiomics Approach with Clustering-Based Feature Selection on Sonoelastography. Ultrasound Med Biol 2017;43:1058-69. [Crossref] [PubMed]
- Li Y, Liu Y, Zhang M, et al. Radiomics With Attribute Bagging for Breast Tumor Classification Using Multimodal Ultrasound Images. J Ultrasound Med 2020;39:361-71. [Crossref] [PubMed]
- Lambin P, Rios-Velazquez E, Leijenaar R, et al. Radiomics: Extracting more information from medical images using advanced feature analysis. Eur J Cancer 2012;48:441-6. [Crossref] [PubMed]
- Rios Velazquez E, Parmar C, Liu Y, et al. Somatic Mutations Drive Distinct Imaging Phenotypes in Lung Cancer. Cancer Res 2017;77:3922-30. [Crossref] [PubMed]
- Ashraf AB, Daye D, Gavenonis S, et al. Identification of Intrinsic Imaging Phenotypes for Breast Cancer Tumors: Preliminary Associations with Gene Expression Profiles. Radiology 2014;272:374-84. [Crossref] [PubMed]
- Ben-Hamo R, Efroni S. MicroRNA regulation of molecular pathways as a generic mechanism and as a core disease phenotype. Oncotarget 2015;6:1594-604. [Crossref] [PubMed]
- Wu J, Cui Y, Sun X, et al. Unsupervised Clustering of Quantitative Image Phenotypes Reveals Breast Cancer Subtypes with Distinct Prognoses and Molecular Pathways. Clin Cancer Res 2017;23:3334-42. [Crossref] [PubMed]
- Guo Y, Hu Y, Qiao M, et al. Radiomics Analysis on Ultrasound for Prediction of Biologic Behavior in Breast Invasive Ductal Carcinoma. Clin Breast Cancer 2018;18:e335-44. [Crossref] [PubMed]
- Zhang Q, Li C, Han H, et al. Spatio-temporal Quantification of Carotid Plaque Neovascularization on Contrast Enhanced Ultrasound: Correlation with Visual Grading and Histopathology. Eur J Vasc Endovasc Surg 2015;50:289-96. [Crossref] [PubMed]
- Otsu N. A Threshold Selection Method from Gray-Level Histograms. IEEE Trans Syst Man Cybern 1979;9:62-6. [Crossref]
- Zhang X, Xu X, Tian Q, et al. Radiomics assessment of bladder cancer grade using texture features from diffusion-weighted imaging. J Magn Reson Imaging 2017;46:1281-8. [Crossref] [PubMed]
- Chen KY, Chen CN, Wu MH, et al. Computerized Quantification of Ultrasonic Heterogeneity in Thyroid Nodules. Ultrasound Med Biol 2014;40:2581-9. [Crossref] [PubMed]
- Sauerbrei W, Royston P, Binder H. Selection of important variables and determination of functional form for continuous predictors in multivariable model building. Stat Med 2007;26:5512-28. [Crossref] [PubMed]
- Priya R, Dass JFP, Siva R. Gene Expression Prediction and Hierarchical Clustering Analysis of Plant CCD genes. Plant Mol Biol Report 2016;34:618-27. [Crossref]
- žurauskienė J, Yau C. pcaReduce: hierarchical clustering of single cell transcriptional profiles. BMC Bioinformatics 2016;17:140. [Crossref] [PubMed]
- Xia W, Chen Y, Zhang R, et al. Radiogenomics of hepatocellular carcinoma: multiregion analysis-based identification of prognostic imaging biomarkers by integrating gene data—a preliminary study. Phys Med Biol 2018;63:035044. [Crossref] [PubMed]
- Farrelly CM, Schwartz SJ, Lisa Amodeo A, et al. The analysis of bridging constructs with hierarchical clustering methods: An application to identity. J Res Pers 2017;70:93-106. [Crossref]
- Chuan ZL, Ismail N, Shinyie WL, et al. The efficiency of average linkage hierarchical clustering algorithm associated multi-scale bootstrap resampling in identifying homogeneous precipitation catchments. IOP Conf. Series: Materials Science and Engineering 2018;342:012070.
- Yin H, Fan Z, Li X, et al. Phylogenetic tree-informed microRNAome analysis uncovers conserved and lineage-specific miRNAs in Camellia during floral organ development. J Exp Bot 2016;67:2641-53. [Crossref] [PubMed]
- Kellom M, Raymond J. Using Dendritic Heat Maps to Simultaneously Display Genotype Divergence with Phenotype Divergence. PLoS One 2016;11:e0161292. [Crossref] [PubMed]
- Wittich W, Phillips N, Nasreddine ZS, et al. Sensitivity and Specificity of the Montreal Cognitive Assessment Modified for Individuals who are Visually Impaired. J Vis Impair Blind 2010;104:360-8. [Crossref]
- Tilton RC, Sand MN, Manak M. The Western Immunoblot for Lyme Disease: Determination of Sensitivity, Specificity, and Interpretive Criteria with Use of Commercially Available Performance Panels. Clin Infect Dis 1997;25:S31-4. [Crossref] [PubMed]
- Metz CE. ROC methodology in radiologic imaging. Invest Radiol 1986;21:720-33. [Crossref] [PubMed]
- Zakaria OM, Mousa A, AlSadhan R, et al. Reliability of sonoelastography in predicting pediatric cervical lymph node malignancy. Pediatr Surg Int 2018;34:885-90. [Crossref] [PubMed]
- Latif MA, Shady M, Hegazy MAE, et al. B-mode ultrasound, sono-elastography and diffusion-weighted MRI in differentiation of enlarged axillary lymph nodes in patients with malignant breast disease. Egypt J Radiol Nucl Med 2016;47:1137-49. [Crossref]
- Liu T, Ge X, Yu J, et al. Comparison of the application of B-mode and strain elastography ultrasound in the estimation of lymph node metastasis of papillary thyroid carcinoma based on a radiomics approach. Int J Comput Assist Radiol Surg 2018;13:1617-27. [Crossref] [PubMed]
- Suo J, Zhang Q, Chang W, et al. Evaluation of Axillary Lymph Node Metastasis by Using Radiomics of Dual-modal Ultrasound Composed of Elastography and B-mode. Zhongguo Yi Liao Qi Xie Za Zhi 2017;41:313-6. [PubMed]
- Huber S, Danes J, Zuna I, et al. Relevance of sonographic B-mode criteria and computer-aided ultrasonic tissue characterization in differential/diagnosis of solid breast masses. Ultrasound Med Biol 2000;26:1243-52. [Crossref] [PubMed]
- Aoyagi S, Izumi K, Hata H, et al. Usefulness of real-time tissue elastography for detecting lymph-node metastases in squamous cell carcinoma. Clin Exp Dermatol 2009;34:e744-7. [Crossref] [PubMed]
- Okasha H, Mansour M, Attia K, et al. Role of high resolution ultrasound/endosonography and elastography in predicting lymph node malignancy. Endosc Ultrasound 2014;3:58. [Crossref] [PubMed]
- Liu Z, Zeng W, Liu C, et al. Diagnostic accuracy of ultrasonographic features for lymph node metastasis in papillary thyroid microcarcinoma: a single-center retrospective study. World J Surg Oncol 2017;15:32. [Crossref] [PubMed]
- Kuna SK, Bracic I, Tesic V, et al. Ultrasonographic Differentiation of Benign From Malignant Neck Lymphadenopathy in Thyroid Cancer. J Ultrasound Med 2006;25:1531-7. [Crossref] [PubMed]
- Wang Y, Ni D, Dou H, et al. Deep Attentive Features for Prostate Segmentation in 3D Transrectal Ultrasound. IEEE Trans Med Imaging 2019;38:2768-78. [Crossref] [PubMed]
- Huang Q, Luo Y, Zhang Q. Breast ultrasound image segmentation: a survey. Int J Comput Assist Radiol Surg 2017;12:493-507. [Crossref] [PubMed]
- Chen H, Wu L, Dou Q, et al. Ultrasound Standard Plane Detection Using a Composite Neural Network Framework. IEEE Trans Cybern 2017;47:1576-86. [Crossref] [PubMed]
- Huang Q, Zhang F, Li X. Few-shot decision tree for diagnosis of ultrasound breast tumor using BI-RADS features. Multimed Tools Appl 2018;77:29905-18. [Crossref]