Prediction of nosocomial infection incidence in the Department of Critical Care Medicine of Guizhou Province with a time series model
Introduction
Nosocomial infection refers to the infection acquired in the hospital, which is the latent infection occurred during or after the patient was discharged from hospital (1). The Department of Critical Care Medicine has become the department with the highest risk of nosocomial infection because of the characteristics of underlying severe diseases, extended hospital stay, many invasive operations, and long-time antibiotic use, as it is a special department for rescuing patients in critical condition (2,3). Among them, ventilator-associated pneumonia, vascular catheter-related bloodstream infections, and urinary tract catheter-related urinary tract infections are the most common hospital infections (4). At present, the prevention of nosocomial infections in intensive care units focuses on the standardized use of antibacterial drugs, the strict implementation of such prevention and control measures in the Hand Hygiene Standards for Medical Personnel, the Management Measures for Disinfection, and the Technical Standards for Hospital Isolation (5).
As early as 1986, the hospital infection monitoring system was set up in China. However, until now, research on hospital infections’ incidence in the Department of Critical Care Medicine in Guizhou Province has remained in the cross-sectional survey. There have been no studies on the trends or predictions of hospital infection incident rates there. Autoregressive Integrated Moving Average (ARIMA) model was proposed by Box and Jenkins, also known as box-Jenkins model, which is an important time series forecasting model. As a mixture of multiple models, in ARIMA (p,d,q) model, AR represents autoregression model, and p is the order. MA represents the sliding average model, and q is the order; d refers to the difference times in the differential stationary processing of the original time series data. Because the ARIMA model comprehensively considers the time trend change, periodic change and other factors, it can get better prediction effect (6-8). In this paper, the ARIMA model was used for the analysis of nosocomial infection incidence rate in the Department of Critical Care Medicine of Guizhou Province, including ventilator-associated pneumonia, vascular catheter-related bloodstream infections, and urinary catheterization-related urinary tract infections. The optimal model was fitted to describe and predict the incidence rate, and dig the occurrence regular, improving the work efficiency of nosocomial infections and reducing nosocomial infection incidence rate in critical medicine. We present the following article in accordance with the TRIPOD reporting checklist (available at http://dx.doi.org/10.21037/atm-20-4171).
Methods
Source
Infection incidence data were collected from the Department of Critical Care Medicine of tertiary A comprehensive hospitals in nine prefecture-level cities of Guizhou province from January 2014 to December 2019, including Guiyang Second People’s Hospital, Guiyang Second People’s Hospital, Bijie First People’s Hospital, Liupanshui People’s Hospital, Tongren People’s Hospital, Anshun People’s Hospital, Qiannan Prefecture People’s Hospital, Qiandongnan Prefecture People’s Hospital, Qianxinan Prefecture People’s Hospital. The territorial scope of the hospitals for data collection in this study covered the whole province of Guizhou province. All the involved hospitals belonged to tertiary A hospitals and were comprehensive treatment centers in the region. The study was conducted in accordance with the Declaration of Helsinki.
Diagnostic criteria
The diagnosis of nosocomial infections was from the “Hospital Infection Diagnostic Standard (Trial)” issued by the Ministry of Health of the People’s Republic of China.
The diagnosis criteria of ventilator-associated pneumonia had several parts. (I) ventilator-assisted breathing >48 hours. (II) The clinical diagnosis was made, and the patient has clinical symptoms including cough and sputum and with body temperature ≥38 °C, increased white blood cells, and inflammatory infiltrative changes in the lungs by X-ray images. Three, the pathogenic diagnosis was confirmed, and the quantitative bacterial culture of sputum specimens was ≥106 colony-forming units CFU/mL, and the bacterial culture concentration of alveolar lavage fluid was ≥104–105 CFU/mL (9,10).
The diagnosis criteria of vascular catheter-related bloodstream infection: (I) clinical diagnosis: discharge of pus or diffuse erythema at the site of venipuncture. Also, the erythema diffuse appears along the subcutaneous route of the catheter, which excludes physical and chemical factors. Meanwhile, the vascular interventional operation required a fever >38 °C, and local tenderness and with no other reason. (II) Pathogen diagnosis: meaningful pathogens are isolated by catheter tip culture and blood culture (4).
The diagnosis criteria of urinary tract intubation-related urinary tract infection were two-fold: (I) they were undergoing urinary tract intubation. (II) they underwent a clinical diagnosis in which the patient has symptoms of urinary tract irritation or has tenderness in the lower abdomen, percussion pain in the kidney area, with or without fever, and with urine test of leukocytes ≥5 in each high power field of male patients. Alternatively, they had a value of ≥10 in each high power of female patients, and the diagnosis of patients with catheterization should be combined with urine culture results. Afterward, they were clinically diagnosed as having urinary tract infection, or effective of antibacterial treatment. (III) Pathogen diagnosis: from clinical diagnosis, meet any of the following: the urine culture of clean mid-stage urine or catheterization collected urine has Gram-positive cocci ≥104 CFU/mL, or Gram-negative bacilli bacteria ≥105 CFU/mL. The urine culture of pubic bone combined with the upper bladder puncture has bacteria ≥103 CFU/mL. Fresh urine samples were examined by microscope after centrifugation to be 15 bacteria in 30 fields of vision. Asymptomatic bacteriuria was determined if the patient is asymptomatic but has a history of endoscopy or indwelling catheterization recently, and the concentration of Gram-positive cocci in a urine culture is ≥104 CFU/mL, or Gram-negative bacteria is ≥105 CFU/mL.
Data processing method
Data on ventilator-associated pneumonia, vascular catheter-related bloodstream infections, and urinary catheterization-related urinary tract infections were collected. The multi-center data were integrated and then analyzed. Among them, the ventilator-associated pneumonia infection rate (‰) = amounts of ventilator-associated pneumonia/numbers of days the patient used the ventilator × 1,000‰. Vascular catheter-related blood flow infection (‰) = number of vascular catheter-related blood flow infections/numbers of days of patients with central venous cannula × 1,000‰. Urinary tract intubation-related urinary tract infection (‰) = number of urinary tracts intubation-related urinary tract infections/numbers of days of patients with urinary tract intubation × 1,000‰.
Statistical methods and the ARIMA model introduction
The SPSS 23.0 software was used to build the ARIMA model. The basic idea was to treat the data series formed by the predicted objects over time as a random series, and this sequence is a set of random variables that depends on time (t). The dependency or autocorrelation (ACF) of random variables are described using corresponding mathematical models, and future values are predicted from the past and present values of the time series. This model can solve: (I) the randomness, stationarity, and seasonality of the time series; (II) choosing a suitable model to simulate and predict the data series.
The process of ARIMA model includes: (I) preprocessing of time series: the premise of ARIMA model is that the time series as prediction object is a stationary random series. First, combine the autocorrelogram and partial autocorrelogram to determine whether it is a stationary series. If not stationary, the time series is converted into a stationary series by difference and seasonal difference, or other ways. The method to observe whether the sequence is stable or not is to observe whether the mean value presented in the sequence diagram changes significantly. The method of observing autocorrelogram and partial autocorrelogram: whether it is one-step truncation and whether its coefficient value is in the confidence interval. Preliminary determination of model scope: The premise of the ARIMA model is that the time series as the prediction object is a stationary random sequence. Firstly, the sequence chart is combined with the chart of correlation and partial correlation analysis to determine whether it is a stationary series. If not stationary, it will be converted into a stationary series by difference and seasonal difference and other ways to determine the model range initially. The method of observing whether the sequence is stable is to observe whether the mean value of the sequence graph changes significantly. The method of observing the autocorrelation function (ACF) and partial autocorrelation (PACF) is to observe whether it is a one-step truncation and whether its coefficient value is in the confidence interval. (II) Model identification, and determination and fitting of the model parameters: model prediction is performed through the preliminary determination of the model range, and the Box-Ljung test is used to determine whether it is a white noise sequence. The model passed the Box-Ljung test if it was determined to have a white noise sequence and P>0.05. However, if multiple models passed the Box-Ljung test, Bayesian Information Criterion (BIC) was used to determine the optimal model, in which the smallest BIC value is the greatest. (III) The prediction model used to predict the value and the predicted values were then added to the actual value to observe its advantages and disadvantages.
Results
Ventilator-associated pneumonia
Model identification
The premise of the ARIMA method is that the predicted objects of time series are a stationary random sequence of zero means. By drawing a sequence diagram of the data, we found to find that the data varied from 2014 to 2019. Therefore, first-order difference processing was performed firstly to stabilize the variance of the sequence.
The sequence diagram after the first-order difference was distributed smoothly. After ACF analysis, the ACF coefficient and PACF coefficient both exceed the upper limit of the confidence interval in the correlation diagram at lag =1. When the lag value becomes larger, the coefficient value quickly decreases to the confidence interval, indicating that the sequence is stable. Also, the seasonality was not apparent, so the ARIMA (p,1,q) model was used for prediction. The distributions of the residual ACF and PACF after the first-order difference are shown in Figure 1 and Figure 2.
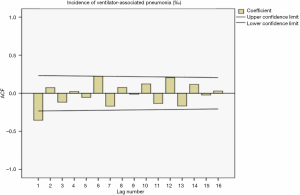
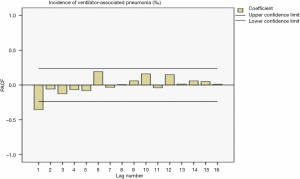
Determination of model parameters
Four models, ARIMA (0,1,0), ARIMA (1,1,0), ARIMA (0,1,1), and ARIMA (1,1,1), were used for time series prediction. The ARIMA (0,1,1) model was selected as the determination equation for the judgment of Ljuing-Box Q test statistical results and BIC value. The model parameters are shown in Table 1.
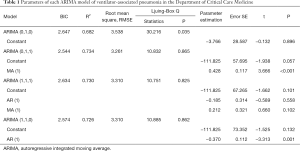
Full table
The results showed R2=0.734 in the ARIMA (0,1,1) model and Q=10.832 (P=0.865) in the residual Ljuing-Box Q test. The ACF and PACF plots of their residuals converge steadily, suggesting it is a white noise sequence (Figure 3).
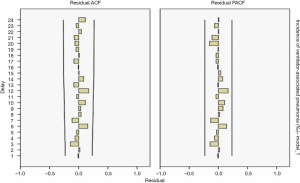
Model fitting
The actual value of the ventilator-associated pneumonia incidence rate matched the predicted value from 2014 to 2019, with a slight difference, as shown in Figure 4.
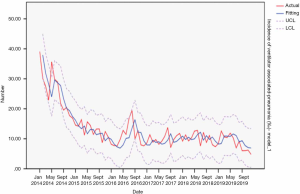
Vascular catheter-related bloodstream infection
Identification of the model
Drawing a sequence diagram of the data, we found the data sequence was stable from 2014 to 2019. After ACF analysis, all data were truncated in one step. The ACF and PACF plots in Figure 5 and Figure 6 showed the seasonality was not apparent, so the ARIMA (p,0,q) model was used for prediction.
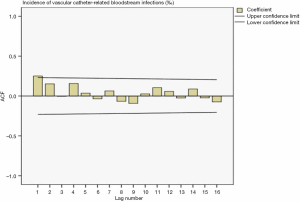
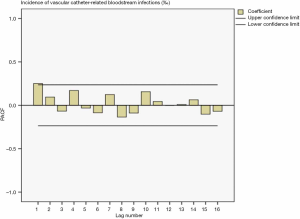
Determination of model parameters
Three models, ARIMA (1,0,0), ARIMA (0,0,1), ARIMA (1,0,1), were used to create the time series prediction. The ARIMA (0,1,1) model was selected as the determination equation by the judgment of Ljuing-Box Q test statistical results and BIC value. The model parameters were shown in Table 2.
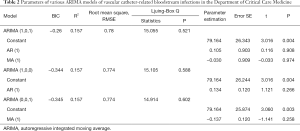
Full table
The results showed R2=0.157 in the ARIMA (0,1,1) model and Q=14.914 (P=0.602) in the residuals Ljuing-Box Q test. The ACF and PACF plots of their residuals converge steadily, suggesting it is a white noise sequence (Figure 7).
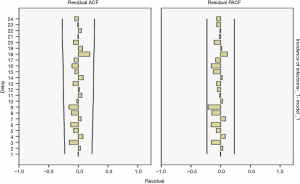
Model fitting
From 2014 to 2019, the actual value of vascular catheter-related bloodstream infections incidence rate matched the predicted value, with a slight difference. The fitting diagram was shown in Figure 8.
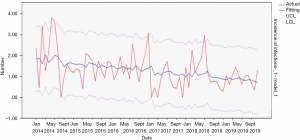
Urinary tract infection related to urinary tract intubation
Model identification
Drawing a sequence diagram of the data, we found the data sequence was stable after logarithmic conversion. The ACF and PACF plots are shown in Figures 9 and 10. Also, the seasonality was not obvious, so the logarithmic transformed ARIMA (p,0,q) model was used.
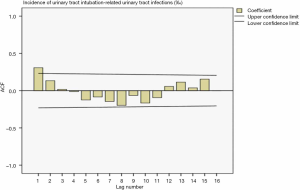
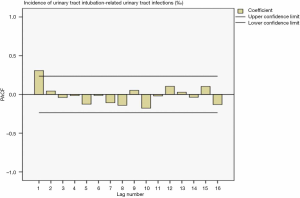
Determination of the parameters in the model
Three models, ARIMA (1,0,0), ARIMA (0,0,1), ARIMA (1,0,1), were used for time series prediction. The ARIMA (1,0,0) model was selected as the best model for the judgment of the Ljuing-Box Q test on the statistical results and BIC values. The model parameters were shown in Table 3.
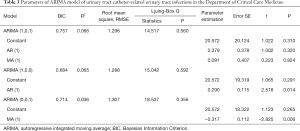
Full table
The ARIMA (1,0,0) model showed R2=0.065, and the Ljuing-Box Q test for residuals showed that Q=15.042 (P=0.592). The ACF and PACF plots of the residuals converge stably, suggesting it is a white noise sequence (Figure 11).
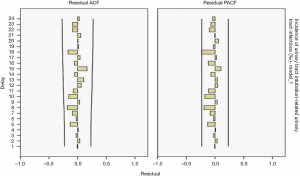
Model fitting
From 2014 to 2019, the actual value of urinary tract intubation-related urinary tract infections incidence rate matched well with the predicted value, with a slight difference. The fitting diagram was shown in Figure 12.
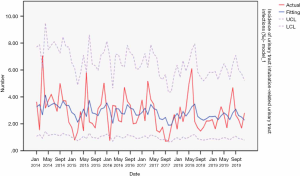
Discussion
The Department of Critical Care Medicine is a high-risk department of hospital infections, and its incidence of hospital infections is significantly higher than ordinary wards, even reaching 2 to 5 times ordinary wards (3). By risk assessment, it has been reported that the department of critical care has the highest incidence of nosocomial infections (1,11). The survey of nosocomial infections in Guizhou Province shows that the prevalence of nosocomial infections in the comprehensive ICU is the highest (12). After a patient develops a hospital infection, the mortality and disease burden of the patient increases significantly. Among the hospital infections, ventilator-associated pneumonia is the most common disease and the leading cause of death from hospital infections (13). Studies on catheter-related bloodstream infections have shown that the rate of central venous catheter-related infections is 20 to 30 times non-catheterized patients, and its hospital mortality rate is as high as 23.0% (14). Also, urinary tract intubation-related urinary tract infection incidence rate is high because of the substantial number and prolonged time required for urinary catheters in the Department of Intensive Medicine. Therefore, it is of great significance for the control of nosocomial infections to reduce the occurrence of ventilator-associated pneumonia effectively, catheter-related bloodstream infections, and urinary tract catheter-related urinary tract infections in the Department of Critical Care Medicine.
Time series forecasting is also called time-series, historical complex, or dynamic series, to sort the values of a certain statistical index into the formed sequence in chronological order. The time series forecasting method is to compile and analyze the time series, and according to the reflection of the development process, direction and trend in the time series, then the level that may be reached in the next period or several years in the future can be predicted by analogy or extension. Among them, the ARIMA model has become one of the most widely used time series prediction models, because it can synthesize the stationarity, seasonality, and randomness of the sequence, and continuously modify the model until the optimal model is selected for disease research (15,16). This study used the ARIMA models to simulate ventilator-associated pneumonia incidence, catheter-related bloodstream infections, and urinary tract intubation-related urinary tract infections to provide a basis for the development of hospital infection control policies, aiming to improve the efficiency of hospital infections and reduce the happening of infections.
In this study, we analyzed the infection data of the Department of Critical Medicine covering six prefecture-level cities and three tertiary A comprehensive treatment hospitals in Guizhou Province. The data were collected from January 2014 to December 2019 lasting for a total of 6 years, and through the ARIMA model, the optimal model was selected according to the BIC criterion. Results showed that the ARIMA (0,1,1) model was the best predictive model for ventilator-associated pneumonia, with an R2 as high as 0.734. The residual Ljuing-Box Q test result showed its Q=10.832 (P=0.865), and the ACF and PACF plots of the residuals converge steadily, suggesting it is a white noise sequence. And the predicted value matched the actual value well. The optimal model of vascular catheter-related bloodstream infection occurrence is the ARIMA (0,0,1) model, with an R2 of 0.157. The Ljuing-Box Q test of the residuals showed Q=14.914 (P=0.602), and the ACF and PACF plots of the residuals converge steadily, suggesting it is a white noise sequence. The predicted values had an excellent match to the actual value. The optimal model of urinary catheterization-related urinary tract infections is the ARIMA (1,0,0) model, with an R2=0.065. The residual Ljuing-Box Q test showed its Q=15.042 (P=0.592), and the ACF and PACF plots of its residuals converged steadily, suggesting it is a white noise sequence. And the predicted value and the actual value matched well.
In China, many researchers use the ARIMA model to predict the time series of hospital infection data. It is suitable to predict the incidence of brucellosis in Jinzhou City of China using the ARIMA (1,1,1), (0,1,1) models (8,17). Li et al.’s research shows that the ARIMA (0,1,1) model is superior to some ARIMA models in predicting the short-term TB incidence in the Chinese population (18). The different time series may have different ARIMA models as for the same infectious diseases and the ARIMA (0,1,1) and ARIMA (1,1,0) models were used to echinococcosis in China from 2007 to 2015 (19).
In this paper, the ARIMA model is used to fit ventilator-associated pneumonia, vascular catheter-related bloodstream infections, and urinary catheter-related urinary tract infections in the Department of Critical Care Medicine of Guizhou Province, which shows a beneficial effect. However, the ARIMA model cannot be simply applied. The model used in this study needs to be used with caution in other provinces with different economic levels, geographical factors, or other clinical departments with distinctive characteristics. Also, the results of this study do not show seasonal changes, which may be because the close environment of the hospital’s intensive care department avoids the changes caused by seasonality changes effectively.
In summary, the ARIMA model was used to fit nosocomial infections incidence rate in the Department of Critical Care Medicine of Guizhou Province, including ventilator-associated pneumonia, vascular catheter-related bloodstream infections, and urinary catheterization-related urinary tract infections, and the optimal models are the ARIMA (0,1,1) model, the ARIMA (0,0,1) model and the ARIMA (1,0,0) model for fitting, respectively. The simulation and prediction effect of these ARIMA models are acceptable.
Acknowledgments
Funding: Guizhou Provincial Science and Technology Plan Project [a grant no. LH (2017)7217].
Footnote
Reporting Checklist: The authors have completed the TRIPOD reporting checklist. Available at http://dx.doi.org/10.21037/atm-20-4171
Data Sharing Statement: Available at http://dx.doi.org/10.21037/atm-20-4171
Conflicts of Interest: All authors have completed the ICMJE uniform disclosure form (available at http://dx.doi.org/10.21037/atm-20-4171). The authors have no conflicts of interest to declare.
(English Language Editor: J. Chapnick)
Ethical Statement: The authors are accountable for all aspects of the work in ensuring that questions related to the accuracy or integrity of any part of the work are appropriately investigated and resolved. The study was conducted in accordance with the Declaration of Helsinki (as revised in 2013).
Open Access Statement: This is an Open Access article distributed in accordance with the Creative Commons Attribution-NonCommercial-NoDerivs 4.0 International License (CC BY-NC-ND 4.0), which permits the non-commercial replication and distribution of the article with the strict proviso that no changes or edits are made and the original work is properly cited (including links to both the formal publication through the relevant DOI and the license). See: https://creativecommons.org/licenses/by-nc-nd/4.0/.
References
- Zhu SJ, Ni XY, Wu QZ, et al. Surveillance of nosocomial infection and analysis of risk factors in an intensive care unit. Chin j Crit Care Med 2019;5:9-14.
- Zhang Z, Bokhari F, Guo Y, et al. Prolonged length of stay in the emergency department and increased risk of hospital mortality in patients with sepsis requiring ICU admission. Emerg Med J 2019;36:82-7. [PubMed]
- Lima Serrano M, González Méndez MI, Carrasco Cebollero FM, et al. Risk factors for pressure ulcer development in Intensive Care Units: A systematic review. Factores de riesgo asociados al desarrollo de úlceras por presión en unidades de cuidados intensivos de adultos: revisión sistemática. Med Intensiva 2017;41:339-46. [Crossref] [PubMed]
- Luyt CE, Hékimian G, Koulenti D, et al. Microbial cause of ICU-acquired pneumonia: hospital-acquired pneumonia versus ventilator-associated pneumonia. Curr Opin Crit Care 2018;24:332-8. [Crossref] [PubMed]
- Wu ZH, Xu Y, Hu QQ, et al. Reducing the incidence of healthcare-associated infection in department of critical care medicine by risk assessment method. Chinese Journal of Infection Control 2019;18:238-42.
- Gao LL, Zhang XL, Duan ZQ, et al. Forcasting Analysis of Health Resources Demand of Medical Institutions in Sichuan Province Based on ARIMA Model. Journal of Preventive Medicine Information 2020;36:197-202.
- Application of ARIMA model in prediction of incidence of surgical site infection. Chinese Journal of Nosocomiology 2020;30:141-5.
- Feng DY, Zhou YQ, Zhou M, et al. Risk Factors for Mortality Due to Ventilator-Associated Pneumonia in a Chinese Hospital: A Retrospective Study. Med Sci Monit 2019;25:7660-5. [Crossref] [PubMed]
- Kalanuria AA, Ziai W, Mirski M. Ventilator-associated pneumonia in the ICU. Crit Care 2016;20:29. [Crossref] [PubMed]
- Monitoring and prevention of multi-drug resistant organism nosocomial infection from 2015 to 2017. Chinese Journal of Nosocomiology 2019.465-8.
- Pan H, Lou Y, Zeng L, et al. Infections Caused by Carbapenemase-Producing Klebsiella pneumoniae: Microbiological Characteristics and Risk Factors. Microb Drug Resist 2019;25:287-96. [Crossref] [PubMed]
- Zhang M, Yang H, Mou X, et al. An interactive nomogram to predict healthcare-associated infections in ICU patients: A multicenter study in GuiZhou Province, China. PLoS One 2019;14:e0219456. [Crossref] [PubMed]
- Johnstone J, Heels-Ansdell D, Thabane L, et al. Evaluating probiotics for the prevention of ventilator-associated pneumonia: a randomised placebo-controlled multicentre trial protocol and statistical analysis plan for PROSPECT. BMJ Open 2019;9:e025228. [Crossref] [PubMed]
- Rupp ME, Karnatak R. Intravascular Catheter-Related Bloodstream Infections. Infect Dis Clin North Am 2018;32:765-87. [Crossref] [PubMed]
- Sato RC. Disease management with ARIMA model in time series. Einstein (Sao Paulo) 2013;11:128-31. [Crossref] [PubMed]
- Liu Q, Li Z, Ji Y, et al. Forecasting the seasonality and trend of pulmonary tuberculosis in Jiangsu Province of China using advanced statistical time-series analyses. Infect Drug Resist 2019;12:2311-22. [Crossref] [PubMed]
- Wang L, Liang C, Wu W, et al. Epidemic Situation of Brucellosis in Jinzhou City of China and Prediction Using the ARIMA Model. Can J Infect Dis Med Microbiol 2019;2019:1429462.
- Li Z, Wang Z, Song H, et al. Application of a hybrid model in predicting the incidence of tuberculosis in a Chinese population. Infect Drug Resist 2019;12:1011-20. [Crossref] [PubMed]
- En-Li T, Zheng-Feng W, Wen-Ce Z, et al. Zhongguo Xue Xi Chong Bing Fang Zhi Za Zhi 2018;30:47-53. [PubMed]
(English Language Editor: J. Chapnick)