Approaches to quantify optical coherence tomography angiography metrics
Introduction
The word tomogram originated from the Greek word ‘tomos’, meaning section. Optical coherence tomography (OCT) was developed based on a low-coherence interferometer, an optical imaging modality for resolving the depth-dependent backscattering signals from the biological tissues. By utilizing a scanner or a translational stage, OCT provides cross-sectional tissue structural information. OCT has evolved rapidly since its invention in 1990s (1) and has gained great success in its translation to healthcare. It is nowadays widely applied to multiple clinical fields, including dermatology, dentistry, cardiology, vascular medicine, etc. However, its main field of application is still in ophthalmology because of the layered structural characteristics and semi-transparency of the ocular tissue (2). As an OCT extension, OCT-angiography (OCTA) utilizes faster OCT systems to detect motion contrast of the biological tissue. In general, OCTA can be divided into two categories: phase-resolved Doppler OCT based on the phase difference between adjacent A-scans (3) and repeated B-scans based OCTA. These two categories have different spectra of applications, and in this review, we will only scope the OCTA generated from repeated B-scans, which we will refer to as OCTA for simplicity.
The development of OCTA over the last decade was remarkable. The concept was conceived in 2006 (4) and the first commercial device equipped with OCTA functionalities was approved by the U.S. Food and Drug Administration in 2016. It was welcomed by the rapid acceptance among clinicians due to its similarity to fluorescein angiography (FA) in non-leakage involved applications, a gold standard angiography imaging modality. Moreover, superior to FA, OCTA provides refined three-dimensional visualization of perfused blood vessels down to the capillary level in a dye-free and non-invasive manner. OCTA has provided us a better understanding of various microvascular related ocular diseases, such as diabetic retinopathy (5-10), age-related macular degeneration (11-13), glaucoma (14-17), and retinal vein occlusion (18,19). Qualitative assessment of the OCTA images might be sufficient for diagnosis of some diseases. For example, studies have shown that choroidal neovascularization was successfully diagnosed based on the existence of new pathological vessels revealed by OCTA (20,21). Quantitative OCTA metrics, on the other hand, may reveal additional subtleties about how the values from patients deviate from the healthy population distribution, helping us to better classify diseases into different severities, understand disease progression, and evaluate therapeutic performance. In this review, we will discuss some of the common issues regarding quantitative OCTA metrics, such as how to extract meaningful metrics, select the appropriate metrics and avoid pitfalls from artifacts.
OCTA signal generation from repeated OCT B-scans
The raw OCT signal acquired on the sensor is nonlinearly distributed in the spectral domain, and k-space linearization is applied to interpolate even spaced sampling in k-space. Then, applying Fourier transform converts the raw OCT signal into spatial signal providing the local depth information. The depth-resolved OCT signal is a complex value that can be expressed as:
[1]
at (x,z) positions and a specific time t, with amplitude A (x, z, t) representing the backscattering potential and phase φ (x, z, t) representing the wave propagation.
The basic idea of OCTA is to calculate the decorrelative signal between repeated B-scans (n≥2). A schematic of volumetric OCTA generation is shown in Figure 1. Since the backscattering signal from static tissues has a different temporal distribution as compared to that of moving particles like red blood cells, motion contrast using temporal signal decorrelation can differentiate the dynamic and static tissues. Based on the types of the signal used, OCTA algorithms can be divided into three categories: amplitude-based, phase-based, or phasor-based OCTA.
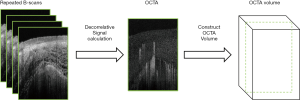
Amplitude-based OCTA
Amplitude based OCTA utilizes the backscattered intensity signal and is insensitive to the phase variation caused by the imaging system itself or the bulk motion from the samples. The amplitude-decorrelation (AD) method calculates the AD between adjacent frames using:
[2]
where An denotes the amplitude of the nth repeated B-scan, and N denotes the number of repeated B-scans at each position (22). Further splitting the full source spectrum into several narrower spectra and calculating the signal decorrelation within each spectrum worsens the axial resolution but demonstrates a better vessel continuity and contrast in angiograms as compared to the full spectrum AD method (23,24). This method is referred as split-spectrum amplitude-decorrelation angiography (SSADA). On the other hand, amplitude variance (AV) OCTA, proposed by Mariampillai et al. in 2008 (25), calculates the inter-frame variance based on the OCT intensity to capture the difference of the Gaussian distribution in “solids” and the Rayleigh distributions in “fluids”. Its capability of capturing capillary size down to 25 µm in an animal chamber window was demonstrated (26). Later, Liu et al. (27) combined spectroscopic OCT (SOCT) and AV OCTA to visualize the oxygen saturation in a chick embryo. SOCT and OCTA contrasts were extracted from the same channel, and SOCT was computed by oxygenated hemoglobin and deoxygenated hemoglobin difference between a longer-wavelength spectral band (880 nm) and a shorter-wavelength one (820 nm). By simply averaging the absolute amplitude difference or squared difference between adjacent tomograms, Huang et al. (28) and Blatter et al. (29) generated a retinal microvasculature map with 10 degrees and 48 degrees field of view (FOV), respectively.
Recently, Ploner and coworkers (30) developed a technique called variable interscan time analysis (VISTA) aiming at quantifying the blood flow from OCTA. A faster 400 kHz swept-source OCT was applied to acquire fast repeated B-scans with 1.5 ms interscan time. By comparing the OCTA signal from 1.5 and 3.0 ms interscan time, the authors could generate a blood flow map in a normalized scale and demonstrated its applicability in patients with various ocular diseases.
Phase-based OCTA
Phase variance (PV) OCTA is similar to AV OCTA. Instead of using the amplitude term in Eq. [2], it calculates the variance of Δφ between repeated scans, where Δφ is the phase difference between adjacent A-scans (22). A review paper has compared these two methods in detail (26). While the AV method suffers less from the system’s phase instability and has a lower computational cost, it is prone to bulk motion and projection artifacts (26). Furthermore, the statistical distribution of the phase decorrelation contains information for predicting the flow velocity. In a phantom setup using intralipid solution in a capillary, Gräfe et al. investigated the relationship between the flow velocity and the statistical properties (based on a maximum likelihood estimator or standard deviation) of the phase distribution, and reported that a normalized flow velocity can be precisely retrieved with repeated phase measurements (31). However, it is not clear what is the minimal number of repeated scans required to predict the flow velocity appropriately and how this algorithm will perform for in-vivo measurements in a clinical setting.
Phasor-based OCTA
Optical microangiography (OMAG) was introduced by Wang et al. (32) and applied to various vascular beds of the body (33-37). The OMAG algorithm migrated from analyzing the frequency components along the A-scan direction (32) to comparing the complex information between repeated B-scans (38). The calculation is achieved by averaging the absolute complex signal difference between consecutive B-scan pairs. Later, a modification combining the geometrical and arithmetic terms was proposed in order to improve the visualization of the choriocapillaris (39). Complex-signal based OMAG showed higher contrast than either amplitude or phase-based OMAG, because both amplitude and phase are sensitive to different flow rates and combining them can therefore increase the overall OCTA signal (39).
Nevertheless, phasor based OCTA is vulnerable to phase noise. For example, in swept-source OCTA, mechanical mirrors in the light source may create jitter artifacts in the spectrum sweep. Several algorithms have been developed for generating phase insensitive OCTA maps (40-43). Akinetic swept sources can sweep the spectrum via electrical tuning, making it immune to mechanical jittering, and therefore have higher phase stability (41,44,45).
Direct comparison between amplitude, phase, and phasor based OCTA algorithms is complicated, because the hardware and computational time requirements differ in different setups. For prototype systems, Gorczynska et al. (22) used a 100 kHz SSOCT system to compare the performance of AD, AV, and PV OCTA algorithms in imaging retinal and choroidal vessels. Marginal difference in retinal microvasculature was observed among these three methods, but splitting spectrum improved the vessel continuity. For the choroidal vessels, PV OCTA performed slightly better than the amplitude-based methods, while the performance was not significantly different in visualizing choriocapillaris. Poddar et al. (46) utilized a fast megahertz (MHz) Fourier Domain Mode Lock (FDML) laser to acquire OCT images from the posterior pole of human subjects and compared the PV and AV OCTA images. Results showed better delineation of choroidal vessels and choriocapillaris using PV OCTA, while the AV OCTA exhibited less signal strength with fast flow saturating the signal. For commercial systems, agreement of OCTA matrices on the retinal vasculature (47) or neovascularization (48) is weak. One of the possible reasons is that different systems may employ different processing methods in OCTA generation, vessel enhancement, segmentation, binarization, etc. Hence it is difficult to evaluate the performance of OCTA generation algorithms by analyzing the data generated from the commercial machines. More recently, some state-of-the art theories of speckle flowmetry investigated the relationship between OCT temporal decorrelation and flow rate (49). Without considering the phase fluctuation from the system, the author concluded that phasor-based decorrelation can best predict the flow velocity, whereas largest discrepancy was seen when using the amplitude-based method.
Commercial OCTA systems favor different algorithms to generate their OCTA signals. For example, AngioVue (OptoVue, Fremont, California, USA) uses an amplitude-based SSADA algorithm (50), Triton (Topcon, Japan) employs an amplitude-based OCTA ratio analysis (51), and AngioPlex (Zeiss, USA) uses a phasor-based OMAG algorithm (52).
Recently, deep learning (DL) technology has been applied in the biomedical imaging field, and some groups have attempted to generate OCTA images via DL. For example, Lee et al. (53) tried to generate retinal OCTA images from the single acquisition of clinically available OCT images by transferring a trained U-Net, but results were not satisfactory and only large vessels could be successfully reproduced. Liu et al. (54) applied convolutional neural networks to generate OCTA images from four repeated scans, and the ground truth was generated from a phasor based OCTA algorithm (55). They concluded that the OCTA map generated from DL algorithm has a better signal to noise ratio (SNR) and lower speckle noise than the traditional method. With the rapid evolution of new DL networks, such as the generative adversarial network (GAN), there will be more implementation of DL algorithms in OCTA calculation in the near future.
Methods for artifact removal in OCTA
OCTA artifacts originate from multiple sources, such as bulk motion, vessel projections, laser beam blockage due to eyelashes or cataracts, and segmentation errors (56-60). They are common in acquisition and can affect quantification of OCTA metrics as well as clinical diagnosis. Thus, it’s important to develop algorithms that suppress or remove artifacts for improving the performance of OCTA in clinical applications.
Motion artifacts during image acquisition are unavoidable, and it comes from voluntary or involuntary eye motion, cardiac motion or head motion. It leads to vessel breaks, doubling, or stretching, and consequently affects OCTA quantification. Reducing motion artifacts can generally be done by increasing the acquisition speed, implementing a retina tracker, or by redesigning special scanning patterns. MHz OCT with a FDML laser can increase the acquisition speed by a factor of 16× as compared to commercial systems (61,62), so that motion artifacts can be greatly reduced given a short acquisition time. However, FDML lasers are expensive and MHz OCT systems can still be sensitive to fast saccades. Retinal trackers, such as the line-scan ophthalmoscope or scanning laser ophthalmoscope, detect the motion in real-time and send feedback to scanners to adjust the scanning region and suspend scanning during saccades. The drawback is the longer acquisition time especially for patients with fixation problems and high system complexity. Lastly, some special scanning patterns were designed to reconstruct motionless volumes from post-processing, such as x-y scans (63) and Lissajous scans (64), which require longer acquisition time and higher computational cost for image reconstruction. Figure 2A shows an example comparing the enface OCTA images acquired from a 3 mm × 3 mm retinal image with and without the use of the eye tracker.
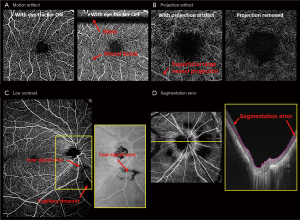
Superficial vessels can project shadows onto deeper vascular plexuses, which are called projection artifacts or tail artifacts. Dynamic scattering potential in blood vessels affects the number of photons and wavefront reaching the deeper tissue, and consequently change the strength of the decorrelative signals from the deeper tissue. This effect is more severe for large vessels with a high flow rate as shown in Figure 2B. Bernucci et al. injected a contrast agent with isotropic scattering (fat emulsion dietary) intravenously to enhance the OCTA signal, and no significant change of the projection artifact was observed (65). This indicated that the projection artifact is partially due to the dumbbell shape of the red blood cell. To overcome the projection artifact, one could use adaptive optics (AO) with better focusing (66,67) or post-processing algorithms: A step-down exponential filter (68) was used to remove projection artifacts from OCTA cross-sectional scans in brains of rats. Moreover, projection artifacts in deeper enface angiograms can be removed by subtracting the signal from the superficial angiograms (69,70), or comparing the OCTA signal from A-scans (17,71). Projection resolved images provided better visualization of the deeper retinal layers and the choroids, as well as the vasculatures in these layers (70,72,73).
Low image contrast is encountered in subjects with cataracts, eyelash blockage or high myopia (Figure 2C). Low contrast can be global or regional, especially for large FOV images. Optical vignetting creates an uneven illumination of the retina, making the center bright and the periphery dark. Moreover, strong curvature of high myopic eyes can result in retina wrapped by the image range (74). Hence, correctly differentiating the low contrast regions from pathological capillary dropout is crucial for diagnosing vascular insufficiency in diseases such as diabetic retinopathy. De Pretto et al. (75). used spatial variance filter and OCT contrast thresholding to differentiate the artifacts from real capillary dropout. The implementation of a DL algorithm was also investigated for the same task from the corresponding structural image (76).
Segmentation error is probably the most important artifact in OCTA (Figure 2D). OCT image quality is easily deteriorated by speckle noise, and retinal layers have limited boundary contrast. Numerous segmentation algorithms, in both automatic and semi-automatic manners, have been developed, including k-nearest mean (77), graph cut (78), graph theory (63,79), Markov boundary model (80), active contour (81,82), texture and shape analysis (83,84), 3-D theoretical representation fitting (85), and sparse high order potentials (86). Dubose et al. (87) modeled statistical intensity distribution of the retinal layers and used Cramèr-Rao lower bound to explain the limitation of traditional retinal layer segmentation. Recently developed retinal layer segmentation algorithms have been heavily influenced by machine learning (ML) concepts. Various ML methods including support vector machine (88), random forest (89), neural network (90), and DL architectures were applied to retinal segmentation in healthy and diseased eyes (91-101). De Fauw and coworkers (102) were able to provide precise segmentation of multiple pathological sites after training on 14,884 scans. Nevertheless, it is still challenging to transfer the ML-based segmentation into OCTA in a clinical setting. It is difficult to transfer trained models from one machine to another, and for some pathologies such as neovascularization, training datasets are simply insufficient.
OCTA metrics algorithms
Numerous methods have been developed and investigated for extracting quantitative metrics from OCTA images. OCTA processing is still in its infant stage and so far there has been no consensus of the standard processing framework, which complicates the comparisons of the reported results. The problem is exacerbated by the interference between different factors, so one cannot simply conclude the performance of one factor without considering the subtle effect of the others. Here, we list out these influences and discuss how they can potentially affect the OCTA quantifications.
Vessel enhancement algorithms
Retinal vessel network in healthy human eyes can usually be divided into several plexuses, each of them almost parallel to the retinal surface. Vertical anastomoses link the entrances and exits of each plexus to form a closed-loop network. Therefore, enface angiograms in each plexus can be analyzed individually. Prior to the quantification, some vessel enhancement filters are usually applied to increase the angiogram SNR. The Frangi filter approach (103) is widely applied to enhance the tube-like properties in images by using second-order derivative of the image intensity. However, its performance is strongly dependent on the presence of vessels of different sizes (scales) in the same image. Gabor filter is a vessel enhancement algorithm based on the determination of orientations within the image. It detects the local image orientation by convoluting the image with Gabor kernels and Hendargo et al. (104) used it to increase the efficiency of angiogram mosaic stitching. Alternative filters include weighted symmetry filter (105), active shape models (106), vessel enhancement diffusion (107) and local phase-based filter (108). Wavelet decomposition (109) and modified Bayesian residual transform (MBRT) (110) decompose the image into multiple residual images, where each residual image represents information at a unique scale. Both methods could simultaneously reduce speckle noise and enhance the contrast of vessels. Figure 3 shows examples of applying representative vessel enhancement filters (Frangi, Gaussian, Gabor, Bayesian and superpixel) to two 3 mm × 3 mm macular scans, one from a healthy eye and the other from an eye with moderate diabetic retinopathy. Vessels appeared significantly different with various filters, and consequently perfusion density results range from 40.4% to 62.0% in the healthy eye and 38.1% to 61.8% in the diabetic retinopathy eye, using mean grayscale intensity as a threshold for binarization. There is as yet no agreement on which filter best suits OCTA analysis. Hong et al. tested the repeatability of OCTA metrics with multiple filters (Gaussian, Gabor, Frangi, and MBRT) in an aged population. The performance of different filters varies in 3 mm × 3 mm and 12 mm × 12 mm protocols: Gabor and MBRT filters yielded the best performances in 3 mm × 3 mm and 12 mm × 12 mm protocols, respectively (111).
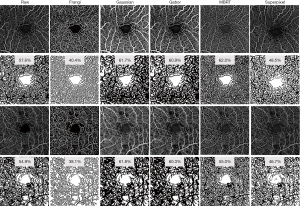
Averaging over multiple scans might be the most straightforward method to reduce image speckle and increase SNR. Pulsatile flow and pericyte constriction can cause a heterogeneous blood vessel lumen appearance and averaging multiple frames can reduce this unevenness. Although averaging phasor images lead to better results than averaging amplitude image (112), it is computationally expensive and such post-processing is impractical to deal with images from commercial systems because of the limited access to the raw data. Uji et al. (113) showed that averaging nine scans greatly improves the visualization of retinal vessels and better delineates the boundary of the fovea avascular zone (FAZ). Mo et al. (114) observed a better correlation between AO scanning laser ophthalmoscope FA and OCTA when multiple enface images were registered and averaged. OCTA images of choriocapillaris also benefit from averaging. High lateral resolution and sampling are required to visualize choriocapillaris, and averaging multiple frames can substantially increase their visibility (113,115). However, the drawback is the longer acquisition time which leads to the low tolerability for patients with poor fixation or physical difficulties. One study examined the relationship between the number of averaged frames and quantification of retinal vessel metrics using a commercial system and concluded that five scans were optimal to balance the acquisition time and image robustness (116). Using another machine (PlexElite 9000, Zeiss), we performed ten repeated measurements and examined the performance of averaging (unpublished data, Figure 4). Here, averaging three images seemed visually comparable to averaging ten images to balance the retinal vessel unevenness and generate an anatomically reasonable choriocapillaris pattern. Moreover, it is worth noting that scan quality consistency and precise registration algorithms are critical to avoid signal degradation.
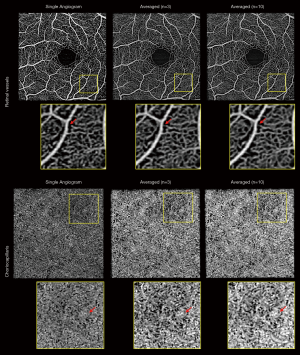
Selection of thresholding method is also critical for correct OCTA interpretation. The thresholding methods can be divided into two categories: global methods and local or regional methods. Global thresholding includes Otsu’s histogram adaptive thresholding (117) and mean/standard deviation thresholding. Otsu methods try to separate the foreground and background by adaptively selecting a local minimum in the histogram to be the threshold, and the mean/standard deviation method sets a fixed value, e.g., mean + standard deviation, to be the threshold. Local or regional thresholding methods such as Phansalkar (118), Niblack (119), and Sauvola (120), use different types of local information to binarize images. Both types of methods have their advantages and disadvantages. For instance, local thresholding methods tackle the uneven illuminance in images, but the drawback is the need to tune the kernel size. One example is over-segmenting the noise in the FAZ (121) with a small kernel radius. Furthermore, noise present in the OCTA images, especially in the low signal regions such as choriocapillaris can easily cause misinterpretation if thresholding is not done properly. For careful interpretation of the perfusion readings, the binarized images must be assessed alongside the OCTA images (122).
Vessel selectivity algorithms allow for the analysis of large vessels and capillaries separately. For several diseases such as glaucoma (123), diabetic retinopathy (6,124), ischemic optic neuropathy (125,126) and Alzheimer’s disease (127) where capillaries are more affected, separating the large vessels from capillaries could bring extra diagnostic power as compared to combining them. Vessel selectivity can be simply done by thresholding the angiograms and applying some morphology operators, and some aforementioned vessel enhancement filters can also be applied prior to the thresholding. However, the vessel selectivity algorithm is not perfect yet, and the selection standard usually differs from the anatomical definitions, such as vessel branch order.
Quantification
Traditional quantitative metrics for OCTA analysis are perfusion density and vessel density. The perfusion density is calculated as the ratio of the binarized perfusion area to the entire imaged area. Vessel density is based on the skeletonized vessel map, using a one-pixel line to represent the vessel, as such excluding the effect of the vessel diameter. It is calculated as the ratio of vessel area to the entire imaged area.
There are also some other proposed metrics such as fractal dimension (8,128) and complexity index (129), but they are less intuitive and more computationally costly. Because of the heterogeneous nature of the parafoveal vessels (130), perfusion and vessel densities were sometimes calculated separately in four quadrants. For example, as shown in Figure 5, an annulus with inner ring diameter 1 mm and outer ring diameter 2.5 mm was evenly separated into superior, inferior, temporal and nasal quadrants.
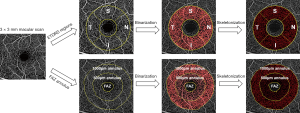
For choriocapillaris, instead of calculating the area of perfusion, researchers used the non-perfused area for analysis with some binarization approaches. These non-perfused regions, based on individual preference, are called flow voids, flow deficits or signal voids. The total non-perfused region usually reflects the global change in the imaged area due to systemic diseases, such as hypertension (73), and sizes of individual non-perfused regions can represent the local change due to more focal pathology, such as drusen (131).
Magnification is an important factor to consider. The actual FOV Sactual can be modeled as a function of axial eye length:
Sactual=p · q · Sprotocol[3]
where Sprotocol is the FOV of scanning protocol, p is the magnification factor of the machine optics, and q is the magnification factor of the eye which has been expressed as 0.01306 · (AL−1.82) with AL representing the axial eye length and 1.82 is the distance between the corneal apex and the second principal plane in mm (132). Whether this formula is, however, truly correcting all magnification errors remains unproven. Magnification can affect how much FAZ is included in the calculation and also perfusion density because perfusion decreases along with eccentricity (133), which can further affect the quantification of OCTA images especially in highly myopic eyes with long axial eye length (134,135). A systematic review revealed only 8% of 509 articles correctly rescale the imaging area (136). A lack of axial eye measurement might be the reason and, in this case, the FAZ annulus method might be a more appropriate approach to mitigate the influence of FAZ. An example is shown in Figure 5, where the FAZ was manually delineated and its 500 µm and 1,000 µm thick annulus were defined for regional OCTA quantification. Nevertheless, this method could be labor-intensive due to the manual delineation of FAZ.
OCTA metrics can be significantly affected by many other factors, such as lateral resolution, sampling rate, system speed and scan direction. Different lateral resolutions and sampling rates from clinical machines gave dissimilar qualitative and quantitative OCTA results (48). AO OCTA is a new technology to correct the ocular aberration and achieve diffraction limited lateral resolution by utilizing deformable mirrors, and capillaries in AO-OCTA images appeared to be narrower and consequently yielded lower perfusion density compared to that imaged without AO (66,114,137,138). In addition, increasing the sampling density has a substantial effect on the OCTA visualization and quantification (139), changing the sampling rate post-processing can significantly alter the choriocapillaris size (140) and retinal perfusion density (141). Different sampling densities using the same machine (PlexElite 9000, Zeiss, USA) resulted in disagreement of perfusion and vessel density calculation and performed differently in diagnosing diabetic retinopathy (142). Imaging speed, including A-scan and B-scan speed, also contribute to the OCTA signal. The time interval between repeated B-scans is sensitive to the blood flow in a specific range (30,143). Due to the large variation of blood flow rate in retinal and choroidal vessels, different systems may advance in detecting either one of them: faster systems, in general, have shorter interscan time interval, which are more sensitive to faster flow and the converse is also true. For example, a 1.7-MHz FDML laser is 17× faster than most lasers that are used in commercial machines. Using such a laser as light source could substantially reduce the interscan time and consequently improve the visualization of the fast flow such as choriocapillaris and choroid, but conversely compromised the detection of slow flow velocities as, for example in foveal capillaries adjacent to the FAZ (144). Last but not least, the scan direction is another factor influencing the OCTA signal that is not sufficiently taken into account. First, the OCTA signal is most sensitive to the flow perpendicular to the fast scanning direction. Changing the scan direction will result in being sensitive the flow from a different angle (145). Second, the motion artifacts are usually along the slow scan axis, which might consequently alter the OCTA image appearance. Generally speaking, quantification of OCTA metrics can be affected by a myriad of factors, which can influence the outcome and the results will need to be reported alongside detailed method description. Comparisons are complicated among commercial machines using different parameters and methods.
In summary, OCTA can reveal the three-dimensional retinal and choroidal vascular network with unprecedented resolution and speed. Although OCTA machines have been commercialized by several companies and the technology has been translated to clinics, research is still ongoing to find optimized solutions for interpreting and quantifying OCTA images. Novel approaches are directed towards the quantification of blood flow rate from OCTA, which is important for understanding local or global perfusion changes as well as vascular dysregulation prior to the morphological change in microvasculature, but no solution for this problem has been found as yet. Whereas OCTA has been extensively studies for ocular disease, future studies will also explore the use of ocular OCTA as a window to link ocular vasculature to the function of other organs such as the kidney and brain. To find clinically relevant association selection of an optimized quantification algorithm is crucial.
Acknowledgments
Funding: The study is funded by National Medical Research Council (grants CG/C010A/2017, OFIRG/0048/2017, OFLCG/004c/2018, and TA/MOH-000249-00/2018), the Duke-NUS Medical School [Duke-NUS-KP(Coll)/2018/0009A], Singapore and the Austrian Science Fund (KLIF529). The sponsor or funding organization had no role in the design or conduct of this research.
Footnote
Provenance and Peer Review: This article was commissioned by the Guest Editor (Dr. Gabor Hollo) for the series “OCT Angiography in Glaucoma” published in Annals of Translational Medicine. The article was sent for external peer review organized by the Guest Editor and the editorial office.
Conflicts of Interest: All authors have completed the ICMJE uniform disclosure form (available at http://dx.doi.org/10.21037/atm-20-3246). The series “OCT Angiography in Glaucoma” was commissioned by the editorial office without any funding or sponsorship. The authors have no other conflicts of interest to declare.
Ethical Statement: The authors are accountable for all aspects of the work in ensuring that questions related to the accuracy or integrity of any part of the work are appropriately investigated and resolved.
Open Access Statement: This is an Open Access article distributed in accordance with the Creative Commons Attribution-NonCommercial-NoDerivs 4.0 International License (CC BY-NC-ND 4.0), which permits the non-commercial replication and distribution of the article with the strict proviso that no changes or edits are made and the original work is properly cited (including links to both the formal publication through the relevant DOI and the license). See: https://creativecommons.org/licenses/by-nc-nd/4.0/.
References
- Huang D, Swanson EA, Lin CP, et al. Optical Coherence Tomography HHS Public Access. Science 1991;254:1178-81. [Crossref] [PubMed]
- Drexler W, Fujimoto JG. Optical coherence tomography: technology and applications. Springer Science & Business Media, 2008.
- Leitgeb RA, Werkmeister RM, Blatter C, et al. Doppler optical coherence tomography. Prog Retin Eye Res 2014;41:26-43. [Crossref] [PubMed]
- Makita S, Hong Y, Yamanari M, et al. Optical coherence angiography. Opt Express 2006;14:7821. [Crossref] [PubMed]
- Couturier A, Mané V, Bonnin S, et al. Capillary plexus anomalies in diabetic retinopathy on optical coherence tomography angiography. Retina 2015;35:2384-91. [Crossref] [PubMed]
- Tan B, Chua J, Lin E, et al. Quantitative Microvascular Analysis With Wide-Field Optical Coherence Tomography Angiography in Eyes With Diabetic Retinopathy. JAMA Netw Open 2020. [Crossref] [PubMed]
- Tey KY, Teo K, Tan ACS, et al. Optical coherence tomography angiography in diabetic retinopathy: a review of current applications. Eye Vis (Lond) 2019;6:37. [Crossref] [PubMed]
- Ting DSW, Tan GSW, Agrawal R, et al. Optical coherence tomographic angiography in type 2 diabetes and diabetic retinopathy. JAMA Ophthalmol 2017;135:306-12. [Crossref] [PubMed]
- Hirano T, Kakihara S, Toriyama Y, et al. Area-based algorithm for quantifying diabetes-related capillary dropout using optical coherence tomography. Ophthalmology 2018;135:1199-203.
- Schottenhamml J, Moult EM, Ploner S, et al. An automatic, intercapillary area-based algorithm for quantifying diabetes-related capillary dropout using optical coherence tomography angiography. Retina 2016;36:S93-101. [Crossref] [PubMed]
- Borrelli E, Sarraf D, Freund KB, et al. OCT angiography and evaluation of the choroid and choroidal vascular disorders. Prog Retin Eye Res 2018;67:30-55. [Crossref] [PubMed]
- Borrelli E, Uji A, Sarraf D, et al. Alterations in the choriocapillaris in intermediate age-related macular degeneration. Invest Ophthalmol Vis Sci 2017;58:4792-8. [Crossref] [PubMed]
- Nesper PL, Soetikno BTBT, Fawzi AAA. Choriocapillaris Non-Perfusion is Associated with Poor Visual Acuity in Eyes with Reticular Pseudodrusen. Am J Ophthalmol 2017;174:42-55. [Crossref] [PubMed]
- Richter GM. The Promise of Optical Coherence Tomography Angiography in Glaucoma. Ophthalmology 2017;124:1577-8. [Crossref] [PubMed]
- Liu L, Jia Y, Takusagawa HL, et al. Optical Coherence Tomography Angiography of the Peripapillary Retina in Glaucoma. JAMA Ophthalmol 2015;133:1045-52. [Crossref] [PubMed]
- Bojikian KD, Chen PP, Wen JC. Optical coherence tomography angiography in glaucoma. Curr Opin Ophthalmol 2019;30:110-6. [Crossref] [PubMed]
- Takusagawa HL, Liu L, Ma KN, et al. Projection-Resolved Optical Coherence Tomography Angiography of Macular Retinal Circulation in Glaucoma. Ophthalmology 2017;124:1589-99. [Crossref] [PubMed]
- Nobre Cardoso J, Keane PA, Sim DA, et al. Systematic Evaluation of Optical Coherence Tomography Angiography in Retinal Vein Occlusion. Am J Ophthalmol 2016;163:93-107.e6. [Crossref] [PubMed]
- Adhi M, Bonini Filho MA, Louzada RN, et al. Retinal capillary network and foveal avascular zone in eyes with vein occlusion and fellow eyes analyzed with optical coherence tomography angiography. Invest Ophthalmol Vis Sci 2016;57:OCT486-94. [Crossref] [PubMed]
- Bailey ST, Thaware O, Wang J, et al. Detection of Nonexudative Choroidal Neovascularization and Progression to Exudative Choroidal Neovascularization Using OCT Angiography. Ophthalmol Retina 2019;3:629-36. [Crossref] [PubMed]
- Kuehlewein L, Bansal M, Lenis TL, et al. Optical Coherence Tomography Angiography of Type 1 Neovascularization in Age-Related Macular Degeneration. Am J Ophthalmol 2015;160:739-48.e2. [Crossref] [PubMed]
- Gorczynska I, Migacz JV, Zawadzki RJ, et al. Comparison of amplitude-decorrelation, speckle-variance and phase-variance OCT angiography methods for imaging the human retina and choroid. Biomed Opt Express 2016;7:911. [Crossref] [PubMed]
- Jia Y, Bailey ST, Hwang TS, et al. Quantitative optical coherence tomography angiography of vascular abnormalities in the living human eye. Proc Natl Acad Sci U S A 2015;112:E2395-402. [Crossref] [PubMed]
- Jia Y, Tan O, Tokayer J, et al. Split-spectrum amplitude-decorrelation angiography with optical coherence tomography. Opt Express 2012;20:4710. [Crossref] [PubMed]
- Mariampillai A, Standish BA, Moriyama EH, et al. Speckle variance detection of microvasculature using swept-source optical coherence tomography. Opt Lett 2008;33:1530-2. [Crossref] [PubMed]
- Mahmud MS, Cadotte DW, Vuong B, et al. Review of speckle and phase variance optical coherence tomography to visualize microvascular networks. J Biomed Opt 2013;18:50901. [Crossref] [PubMed]
- Liu X, Zhang K, Huang Y, et al. Spectroscopic-speckle variance OCT for microvasculature detection and analysis. Biomed Opt Express 2011;2:2995. [Crossref] [PubMed]
- Huang Y, Zhang Q, Thorell MR, et al. Swept-source OCT angiography of the retinal vasculature using intensity differentiation-based optical microangiography algorithms. Ophthalmic Surg Lasers Imaging Retina 2014;45:382-9. [Crossref] [PubMed]
- Blatter C, Klein T, Grajciar B, et al. Ultrahigh-speed non-invasive widefield angiography. J Biomed Opt 2012;17:070505. [Crossref] [PubMed]
- Ploner SB, Moult EM, Choi W, et al. Toward Quantitative Optical Coherence Tomography Angiography. Retina 2016;36:S118-26. [Crossref] [PubMed]
- Gräfe MGO, Gondre M, de Boer JF. Precision analysis and optimization in phase decorrelation OCT velocimetry. Biomed Opt Express 2019;10:1297. [Crossref] [PubMed]
- Wang RK, Jacques SL, Ma Z, et al. Three dimensional optical angiography. Opt Express 2007;15:4083-97. [Crossref] [PubMed]
- Zhi Z, Cepurna W, Johnson E, et al. Volumetric and quantitative imaging of retinal blood flow in rats with optical microangiography. Biomed Opt Express 2011;2:579-91. [PubMed]
- An L, Qin J, Wang RK. Ultrahigh sensitive optical microangiography for in vivo imaging of microcirculations within human skin tissue beds. Opt Express 2010;18:8220-8. [PubMed]
- Yousefi S, Qin J, Wang RK. Super-resolution spectral estimation of optical micro-angiography for quantifying blood flow within microcirculatory tissue beds in vivo. Biomed Opt Express 2013;4:1214-28. [Crossref] [PubMed]
- Bizheva K, Tan B, Fisher CJ, et al. In-vivo imaging of the morphology and blood perfusion of brain tumours in rats with UHR-OCT (Conference Presentation). Neural Imaging Sens 2017;10051:1005107. [Crossref]
- Tan B, MacLellan B, Mason E, et al. Structural, functional and blood perfusion changes in the rat retina associated with elevated intraocular pressure, measured simultaneously with a combined OCT+ ERG system. PLoS One 2018;13:e0193592. [Crossref] [PubMed]
- An L, Wang RK. In vivo volumetric imaging of vascular perfusion within human retina and choroids with optical micro-angiography. Opt Express 2008;16:11438-52. [Crossref] [PubMed]
- Chu Z, Chen CL, Zhang Q, et al. Complex signal-based optical coherence tomography angiography enables in vivo visualization of choriocapillaris in human choroid. J Biomed Opt 2017;22:1-10. [Crossref] [PubMed]
- Song S, Xu J, Men S, et al. Robust numerical phase stabilization for long-range swept-source optical coherence tomography. J Biophotonics 2017;10:1398-410. [Crossref] [PubMed]
- Xu J, Song S, Wei W, et al. Wide field and highly sensitive angiography based on optical coherence tomography with akinetic swept source. Biomed Opt Express 2016;8:420. [PubMed]
- Nam AS, Chico-Calero I, Vakoc BJ. Complex differential variance algorithm for optical coherence tomography angiography. Biomed Opt Express 2014;5:3822-32. [PubMed]
- Braaf B, Donner S, Nam AS, et al. Complex differential variance angiography with noise-bias correction for optical coherence tomography of the retina. Biomed Opt Express 2018;9:486-506. [Crossref] [PubMed]
- Preisser S, Rohringer W, Liu M, et al. All-optical highly sensitive akinetic sensor for ultrasound detection and photoacoustic imaging. Biomed Opt Express 2016;7:4171-86. [Crossref] [PubMed]
- Chen Z, Liu M, Minneman M, et al. Phase-stable swept source OCT angiography in human skin using an akinetic source. Biomed Opt Express 2016;7:3032-48. [Crossref] [PubMed]
- Poddar R, Werner JS. Implementations of three OCT angiography (OCTA) methods with 1.7 MHz A-scan rate OCT system on imaging of human retinal and choroidal vasculature. Opt Laser Technol 2018;102:130-9. [Crossref]
- Li XX, Wu W, Zhou H, et al. A quantitative comparison of five optical coherence tomography angiography systems in clinical performance. Int J Ophthalmol 2018;11:1784-95. [PubMed]
- Corvi F, Cozzi M, Barbolini E, et al. Comparison between several optical coherence tomography angiography devices and indocyanine green angiography of choroidal neovascularization. Retina 2020;40:873-80. [Crossref] [PubMed]
- Gräfe MGO, Nadiarnykh O, De Boer JF. Optical coherence tomography velocimetry based on decorrelation estimation of phasor pair ratios (DEPPAIR). Biomed Opt Express 2019;10:5470. [Crossref] [PubMed]
- Agemy SA, Scripsema NK, Shah CM, et al. Retinal vascular perfusion density mapping using optical coherence tomography angiography in normals and diabetic retinopathy patients. Retina 2015;35:2353-63. [Crossref] [PubMed]
- Stanga PE, Tsamis E, Papayannis A, et al. Swept-Source Optical Coherence Tomography AngioTM (Topcon Corp, Japan): Technology Review. Dev Ophthalmol 2016;56:13-7. [Crossref] [PubMed]
- Rosenfeld PJ, Durbin MK, Roisman L, et al. ZEISS Angioplex™ Spectral Domain Optical Coherence Tomography Angiography: Technical Aspects. Dev Ophthalmol 2016;56:18-29. [Crossref] [PubMed]
- Lee CS, Tyring AJ, Wu Y, et al. Generating retinal flow maps from structural optical coherence tomography with artificial intelligence. Sci Rep 2019;9:5694. [Crossref] [PubMed]
- Liu X, Huang Z, Wang Z, et al. A deep learning based pipeline for optical coherence tomography angiography. J Biophotonics 2019;12:e201900008. [Crossref] [PubMed]
- Liu G, Jia Y, Pechauer AD, et al. Split-spectrum phase-gradient optical coherence tomography angiography. Biomed Opt Express 2016;7:2943-54. [Crossref] [PubMed]
- Say EAT, Ferenczy S, Magrath GN, et al. Image quality and artifacts on optical coherence tomography angiography: Comparison of Pathologic and Paired Fellow Eyes in 65 Patients With Unilateral Choroidal Melanoma Treated With Plaque Radiotherapy. Retina 2017;37:1660-73. [Crossref] [PubMed]
- Holmen IC, Konda MS, Pak JW, et al. Prevalence and Severity of Artifacts in Optical Coherence Tomographic Angiograms. JAMA Ophthalmol 2019;138:119-26. [Crossref] [PubMed]
- Enders C, Lang GE, Dreyhaupt J, et al. Quantity and quality of image artifacts in optical coherence tomography angiography. PLoS One 2019;14:e0210505. [Crossref] [PubMed]
- Bernucci MT, Merkle CW, Srinivasan VJ. Investigation of artifacts in retinal and choroidal OCT angiography with a contrast agent. Biomed Opt Express 2018;9:1020. [Crossref] [PubMed]
- Spaide RF, Fujimoto JG, Waheed NK. Image artifacts in Optical coherence tomography angiography. Retina 2015;35:2163-80. [Crossref] [PubMed]
- Kolb JP, Klein T, Kufner CL, et al. Ultra-widefield retinal MHz-OCT imaging with up to 100 degrees viewing angle. Biomed Opt Express 2015;6:1534-52. [Crossref] [PubMed]
- Klein T, Wieser W, Reznicek L, et al. Multi-MHz retinal OCT. Biomed Opt Express 2013;4:1890-908. [Crossref] [PubMed]
- Larocca F, Chiu SJ, McNabb RP, et al. Robust automatic segmentation of corneal layer boundaries in SDOCT images using graph theory and dynamic programming. Biomed Opt Express 2011;2:1524-38. [Crossref] [PubMed]
- Chen Y, Hong YJ, Makita S, et al. Three-dimensional eye motion correction by Lissajous scan optical coherence tomography. Biomed Opt Express 2017;8:1783-802. [PubMed]
- Bernucci MT, Merkle CW, Srinivasan VJ. Investigation of artifacts in retinal and choroidal OCT angiography with a contrast agent. Biomed Opt Express 2018;9:1020-40. [Crossref] [PubMed]
- Salas M, Augustin M, Ginner L, et al. Visualization of micro-capillaries using optical coherence tomography angiography with and without adaptive optics. Biomed Opt Express 2016;8:207-22. [Crossref] [PubMed]
- Salas M, Augustin M, Ginner L, et al. Investigation of the benefit of adaptive optics optical coherence tomography angiography for the human retina (Conference Presentation), 2017.
- Vakoc BJ, Lanning RM, Tyrrell JA, et al. Three-dimensional microscopy of the tumor microenvironment in vivo using optical frequency domain imaging. Nat Med 2009;15:1219-23. [Crossref] [PubMed]
- Zhang A, Zhang Q, Wang RK. Minimizing projection artifacts for accurate presentation of choroidal neovascularization in OCT micro-angiography. Biomed Opt Express 2015;6:4130-43. [Crossref] [PubMed]
- Zhang Q, Zhang A, Lee CS, et al. Projection Artifact Removal Improves Visualization and Quantitation of Macular Neovascularization Imaged by Optical Coherence Tomography Angiography. Ophthalmol Retina 2017;1:124-36. [Crossref] [PubMed]
- Wang J, Zhang M, Hwang TS, et al. Reflectance-based projection-resolved optical coherence tomography angiography Biomed Opt Express 2017;8:1536-48. [Invited]. [Crossref] [PubMed]
- Hagag AM, Wang J, Lu K, et al. Projection-Resolved Optical Coherence Tomographic Angiography of Retinal Plexuses in Retinitis Pigmentosa. Am J Ophthalmol 2019;204:70-9. [Crossref] [PubMed]
- Chua J, Chin CWL, Tan B, et al. Impact of systemic vascular risk factors on the choriocapillaris using optical coherence tomography angiography in patients with systemic hypertension. Sci Rep 2019;9:5819. [Crossref] [PubMed]
- Ang M, Wong CW, Hoang Q V, et al. Imaging in myopia: potential biomarkers, current challenges and future developments. Br J Ophthalmol 2019;103:855-62. [Crossref] [PubMed]
- De Pretto LR, Moult EM, Alibhai AY, et al. Controlling for Artifacts in Widefield Optical Coherence Tomography Angiography Measurements of Non-Perfusion Area. Sci Rep 2019;9:9096. [Crossref] [PubMed]
- Zhang M, Hwang TS, Dongye C, et al. Automated quantification of nonperfusion in three retinal plexuses using projection-resolved optical coherence tomography angiography in diabetic retinopathy. Invest Ophthalmol Vis Sci 2016;57:5101-6. [PubMed]
- Fu H, Xu Y, Lin S, et al. Segmentation and quantification for angle-closure glaucoma assessment in anterior segment OCT. IEEE Trans Med Imaging 2017;36:1930-8. [Crossref] [PubMed]
- Chen X, Niemeijer M, Zhang L, et al. Three-dimensional segmentation of fluid-associated abnormalities in retinal OCT: Probability constrained graph-search-graph-cut. IEEE Trans Med Imaging 2012;31:1521-31. [Crossref] [PubMed]
- Srinivasan PP, Heflin SJ, Izatt JA, et al. Automatic segmentation of up to ten layer boundaries in SD-OCT images of the mouse retina with and without missing layers due to pathology. Biomed Opt Express 2014;5:348-65. [Crossref] [PubMed]
- Koozekanani D, Boyer K, Roberts C. Retinal thickness measurements from optical coherence tomography using a Markov boundary model. IEEE Trans Med Imaging 2001;20:900-16. [Crossref] [PubMed]
- Niu S, de Sisternes L, Chen Q, et al. Automated geographic atrophy segmentation for SD-OCT images using region-based C-V model via local similarity factor. Biomed Opt Express 2016;7:581-600. [Crossref] [PubMed]
- Mujat M, Chan RC, Cense B, et al. Retinal nerve fiber layer thickness map determined from optical coherence tomography images. Opt Express 2005;13:9480-91. [Crossref] [PubMed]
- Gossage KW, Tkaczyk TS, Rodriguez JJ, et al. Texture analysis of optical coherence tomography images: feasibility for tissue classification. J Biomed Opt 2003;8:570-5. [Crossref] [PubMed]
- Baroni M, Diciotti S, Evangelisti A, et al. Texture classification of retinal layers in optical coherence tomography. IFMBE Proc. 2007. [Crossref]
- Zhu W, Zhang L, Shi F, et al. Automated framework for intraretinal cystoid macular edema segmentation in three-dimensional optical coherence tomography images with macular hole. J Biomed Opt 2017;22:76014. [Crossref] [PubMed]
- Oliveira J, Pereira S, Gonçalves L, et al. Multi-surface segmentation of OCT images with AMD using sparse high order potentials. Biomed Opt Express 2016;8:281-97. [Crossref] [PubMed]
- Dubose TB, Cunefare D, Cole E, et al. Statistical Models of Signal and Noise and Fundamental Limits of Segmentation Accuracy in Retinal Optical Coherence Tomography. IEEE Trans Med Imaging 2018;37:1978-88. [Crossref] [PubMed]
- Zawadzki RJ, Fuller AR, Wiley DF, et al. Adaptation of a support vector machine algorithm for segmentation and visualization of retinal structures in volumetric optical coherence tomography data sets. J Biomed Opt 2007;12:041206. [Crossref] [PubMed]
- Lang A, Carass A, Sotirchos E, et al. Segmentation of retinal OCT images using a random forest classifier. Med. Imaging 2013 Image Process, 2013.
- McDonough K, Kolmanovsky I, Glybina IV. A neural network approach to retinal layer boundary identification from optical coherence tomography images. Conference: 2015 IEEE Conference on Computational Intelligence in Bioinformatics and Computational Biology (CIBCB). 2015.
- Shah A, Zhou L, Abrámoff MD, et al. Multiple surface segmentation using convolution neural nets: application to retinal layer segmentation in OCT images. Biomed Opt Express 2018;9:4509. [Crossref] [PubMed]
- Hamwood J, Alonso-Caneiro D, Read SA, et al. Effect of patch size and network architecture on a convolutional neural network approach for automatic segmentation of OCT retinal layers. Biomed Opt Express 2018;9:3049. [Crossref] [PubMed]
- Seebock P, Orlando JI, Schlegl T, et al. Exploiting Epistemic Uncertainty of Anatomy Segmentation for Anomaly Detection in Retinal OCT. IEEE Trans Med Imaging 2020;39:87-98. [Crossref] [PubMed]
- He Y, Carass A, Liu Y, et al. Deep learning based topology guaranteed surface and MME segmentation of multiple sclerosis subjects from retinal OCT. Biomed Opt Express 2019;10:5042. [Crossref] [PubMed]
- Xu R, Niu S, Chen Q, et al. Automated geographic atrophy segmentation for SD-OCT images based on two-stage learning model. Comput Biol Med 2019;105:102-11. [Crossref] [PubMed]
- Masood S, Fang R, Li P, et al. Automatic Choroid Layer Segmentation from Optical Coherence Tomography Images Using Deep Learning. Sci Rep 2019;9:3058. [Crossref] [PubMed]
- Tsuji S, Sekiryu T, Sugano Y, et al. Semantic Segmentation of the Choroid in Swept Source Optical Coherence Tomography Images for Volumetrics. Sci Rep 2020;10:1088. [Crossref] [PubMed]
- Gao K, Niu S, Ji Z, et al. Double-branched and area-constraint fully convolutional networks for automated serous retinal detachment segmentation in SD-OCT images. Comput Methods Programs Biomed 2019;176:69-80. [Crossref] [PubMed]
- Roy AG, Conjeti S, Karri SPK, et al. ReLayNet: retinal layer and fluid segmentation of macular optical coherence tomography using fully convolutional networks. Biomed Opt Express 2017;8:3627. [Crossref] [PubMed]
- Ngo L, Cha J, Han JH. Deep Neural Network Regression for Automated Retinal Layer Segmentation in Optical Coherence Tomography Images. IEEE Trans Image Process 2019;29:303-12. [Crossref] [PubMed]
- Lu D, Heisler M, Lee S, et al. Deep-learning based multiclass retinal fluid segmentation and detection in optical coherence tomography images using a fully convolutional neural network. Med Image Anal 2019;54:100-10. [Crossref] [PubMed]
- De Fauw J, Ledsam JR, Romera-Paredes B, et al. Clinically applicable deep learning for diagnosis and referral in retinal disease. Nat Med 2018;24:1342-50. [Crossref] [PubMed]
- Frangi AF, Niessen WJ, Vincken KL. Multiscale vessel enhancement filtering. Med Image Comput Comput Assist Interv 1998;1496:130-7.
- Hendargo HC, Estrada R, Chiu SJ, et al. Automated non-rigid registration and mosaicing for robust imaging of distinct retinal capillary beds using speckle variance optical coherence tomography. Biomed Opt Express 2013;4:803. [Crossref] [PubMed]
- Zhao Y, Zheng Y, Liu Y, et al. Automatic 2-D/3-D Vessel Enhancement in Multiple Modality Images Using a Weighted Symmetry Filter. IEEE Trans Med Imaging 2018;37:438-50. [Crossref] [PubMed]
- de Bruijne M, Van Ginneken B, Viergever MA, et al. Adapting active shape models for 3D segmentation of tubular structures in medical images. Inf Process Med Imaging 2003;18:136-47. [Crossref] [PubMed]
- Manniesing R, Viergever MA, Niessen WJ. Vessel enhancing diffusion. A scale space representation of vessel structures. Med Image Anal 2006;10:815-25. [Crossref] [PubMed]
- Läthén G, Jonasson J, Borga M. Blood vessel segmentation using multi-scale quadrature filtering. Pattern Recognit Lett 2010;31:762-7. [Crossref]
- Mallat SG. A Theory for Multiresolution Signal Decomposition: The Wavelet Representation. IEEE Trans Pattern Anal Mach Intell 1989;11:674-93. [Crossref]
- Tan B, Wong A, Bizheva K. Enhancement of morphological and vascular features in OCT images using a modified Bayesian residual transform. Biomed Opt Express 2018;9:2394. [Crossref] [PubMed]
- Hong J, Tan B, Quang ND, et al. Intra-session Repeatability of Quantitative Metrics Using Widefield Optical Coherence Tomography Angiography (OCTA) in Elderly Subjects. Acta Ophthalmol 2019. [Crossref] [PubMed]
- Baumann B, Merkle CW, Leitgeb RA, et al. Signal averaging improves signal-to-noise in OCT images: But which approach works best, and when? Biomed Opt Express 2019;10:5755. [Crossref] [PubMed]
- Uji A, Balasubramanian S, Lei J, et al. Choriocapillaris Imaging Using Multiple En Face Optical Coherence Tomography Angiography Image Averaging. JAMA Ophthalmol 2017;135:1197-204. [Crossref] [PubMed]
- Mo S, Krawitz B, Efstathiadis E, et al. Imaging foveal microvasculature: Optical coherence tomography angiography versus adaptive optics scanning light ophthalmoscope fluorescein angiography. Invest Ophthalmol Vis Sci 2016;57:130-40. [Crossref] [PubMed]
- Chu Z, Zhou H, Cheng Y, et al. Improving visualization and quantitative assessment of choriocapillaris with swept source OCTA through registration and averaging applicable to clinical systems. Sci Rep 2018;8:16826. [Crossref] [PubMed]
- Schmidt TG, Linderman RE, Strampe MR, et al. The utility of frame averaging for automated algorithms in analyzing retinal vascular biomarkers in angiovue OCTA. Transl Vis Sci Technol 2019;8:10. [Crossref] [PubMed]
- Otsu N. A Threshold Selection Method from Gray-Level Histograms. IEEE Trans Syst Man Cybern 1979;9:62-6. [Crossref]
- Phansalkar N, More S, Sabale A, et al. Adaptive local thresholding for detection of nuclei in diversity stained cytology images. ICCSP 2011 - International Conference on Communications and Signal Processing. 2011.
- Niblack W. An introduction to digital image processing. An Introd to Digit Image Process 1986. doi: 10.1201/9781315123905-1.. [Crossref]
- Sauvola JJ, Pietikäinen M. Adaptive document image binarization. Pattern Recognit 2000;33:225-36. [Crossref]
- Mehta N, Liu K, Alibhai AY, et al. Impact of Binarization Thresholding and Brightness/Contrast Adjustment Methodology on Optical Coherence Tomography Angiography Image Quantification. Am J Ophthalmol 2019;205:54-65. [Crossref] [PubMed]
- Cole ED, Moult EM, Dang S, et al. The Definition, Rationale, and Effects of Thresholding in OCT Angiography. Ophthalmol Retina 2017;1:435-47. [Crossref] [PubMed]
- Geyman LS, Garg RA, Suwan Y, et al. Peripapillary perfused capillary density in primary open - Angle glaucoma across disease stage: An optical coherence tomography angiography study. Br J Ophthalmol 2017;101:1261-8. [Crossref] [PubMed]
- Xu X, Chen C, Ding W, et al. Automated quantification of superficial retinal capillaries and large vessels for diabetic retinopathy on optical coherence tomographic angiography. J Biophotonics 2019;12:e201900103. [Crossref] [PubMed]
- Balducci N, Morara M, Veronese C, et al. Optical coherence tomography angiography in acute arteritic and non-arteritic anterior ischemic optic neuropathy. Graefes Arch Clin Exp Ophthalmol 2017;255:2255-61. [Crossref] [PubMed]
- Ho JK, Stanford MP, Shariati MA, et al. Optical Coherence Tomography Study of Experimental Anterior Ischemic Optic Neuropathy and Histologic Confirmation. Invest Ophthalmol Vis Sci 2013;54:5981. [Crossref] [PubMed]
- Zhang YS, Zhou N, Knoll BM, et al. Parafoveal vessel loss and correlation between peripapillary vessel density and cognitive performance in amnestic mild cognitive impairment and early Alzheimer’s Disease on optical coherence tomography angiography. PLoS One 2019;14:e0214685. [Crossref] [PubMed]
- Corvi F, Pellegrini M, Erba S, et al. Reproducibility of Vessel Density, Fractal Dimension, and Foveal Avascular Zone Using 7 Different Optical Coherence Tomography Angiography Devices. Am J Ophthalmol 2018;186:25-31. [Crossref] [PubMed]
- Chu Z, Lin J, Gao C, et al. Quantitative assessment of the retinal microvasculature using optical coherence tomography angiography. J Biomed Opt 2016;21:66008. [Crossref] [PubMed]
- Yu DY, Cringle SJ, Yu PK, et al. Retinal capillary perfusion: Spatial and temporal heterogeneity. Prog Retin Eye Res 2019;70:23-54. [Crossref] [PubMed]
- Zhang Q, Zheng F, Motulsky EH, et al. A novel strategy for quantifying choriocapillaris flow voids using swept-source OCT angiography. Invest. Ophthalmol Vis Sci 2018;59:203-11. [Crossref] [PubMed]
- Bennett AG, Rudnicka AR, Edgar DF. Improvements on Littmann’s method of determining the size of retinal features by fundus photography. Graefes Arch Clin Exp Ophthalmol 1994;232:361-7. [Crossref] [PubMed]
- Lavia C, Mecê P, Nassisi M, et al. Retinal Capillary Plexus Pattern and Density from Fovea to Periphery Measured in Healthy Eyes with Swept-Source Optical Coherence Tomography Angiography. Sci Rep 2020;10:1474. [Crossref] [PubMed]
- Yang Y, Wang J, Jiang H, et al. Retinal microvasculature alteration in high Myopia. Invest Ophthalmol Vis Sci 2016;57:6020-30. [Crossref] [PubMed]
- Sampson DM, Gong P, An D, et al. Axial length variation impacts on superficial retinal vessel density and foveal avascular zone area measurements using optical coherence tomography angiography. Invest Ophthalmol Vis Sci 2017;58:3065-72. [Crossref] [PubMed]
- Llanas S, Linderman RE, Chen FK, et al. Assessing the Use of Incorrectly Scaled Optical Coherence Tomography Angiography Images in Peer-Reviewed Studies: A Systematic Review. JAMA Ophthalmol 2019. [Crossref] [PubMed]
- Kurokawa K, Liu Z, Miller DT. Adaptive optics optical coherence tomography angiography for morphometric analysis of choriocapillaris Biomed Opt Express 2017;8:1803. [Invited]. [Crossref] [PubMed]
- Polans J, Cunefare D, Cole E, et al. Enhanced visualization of peripheral retinal vasculature with wavefront sensorless adaptive optics optical coherence tomography angiography in diabetic patients. Opt Lett 2017;42:17-20. [Crossref] [PubMed]
- Poddar R, Migacz JV, Schwartz DM, et al. Challenges and advantages in wide-field optical coherence tomography angiography imaging of the human retinal and choroidal vasculature at 1.7-MHz A-scan rate. J Biomed Opt 2017;22:1-14. [PubMed]
- Tan B, Chua J, Barathi VA, et al. Quantitative analysis of choriocapillaris in non-human primates using swept-source optical coherence tomography angiography (SS-OCTA). Biomed Opt Express 2019;10:356-71. [Crossref] [PubMed]
- Zhou T, Zhou K, Yang J, et al. Digital resolution enhancement in low transverse sampling optical coherence tomography angiography using deep learning. arXiv 2019:1910.01344.
- Hirano T, Kitahara J, Toriyama Y, et al. Quantifying vascular density and morphology using different swept-source optical coherence tomography angiographic scan patterns in diabetic retinopathy. Br J Ophthalmol 2019;103:216-21. [Crossref] [PubMed]
- Choi W, Moult EM, Waheed NK, et al. Ultrahigh-speed, swept-source optical coherence tomography angiography in nonexudative age-related macular degeneration with geographic atrophy. Ophthalmology 2015;122:2532-44. [Crossref] [PubMed]
- Migacz JV, Gorczynska I, Azimipour M, et al. Megahertz-rate optical coherence tomography angiography improves the contrast of the choriocapillaris and choroid in human retinal imaging. Biomed Opt Express 2018;10:50. [Crossref] [PubMed]
- Braaf B, Gräfe MGO, Uribe-Patarroyo N, et al. OCT-Based Velocimetry for Blood Flow Quantification. In: Bille JF. editor. High Resolution Imaging in Microscopy and Ophthalmology. New Frontiers in Biomedical Optics, Cham: Springer International Publishing, 2019:161-79.