Immune-related genes play an important role in the prognosis of patients with testicular germ cell tumor
Introduction
Testicular cancer is a very special type of tumor that occurs more often in men between 14 and 44 years of age. Testicular cancer can be divided into germ cell tumors (TGCT) and non-germ cell tumors according to the pathological type, of which germ cell tumors account for 98%. Germ cell tumors can be further divided into seminoma and non-seminoma. Seminoma are more common in germ cell tumors. The main treatment measures of testicular tumors are surgery and chemoradiation (1). Testicular tumor is a tumor with a high cure rate. Even for patients with metastases, the cure rate is still as high as 80% (2). Therefore, compared to other malignancies, researchers are less interested in exploring the mechanisms of the development of testicular cancer and new treatments. Despite the high cure rate, many questions regarding the treatment and prognosis of testicular tumors remain. Patients with testicular cancer who are not sensitive to existing therapies face an awkward situation. They cannot determine what kind of treatment they should receive and how long they can survive. In addition, patients with recurrent testicular cancer show insensitivity to traditional treatments such as radiotherapy and chemotherapy (3), their survival is also worrying. The issue that cannot be ignored include the long-term survival risk of patients with testicular cancer. It is reported that patients with testicular cancer have a 2–5% chance of contralateral secondary testicular cancer, what’s more, they have a high risk of secondary malignant tumors and a high risk of delayed cardiovascular disease (4-7). Therefore, patients with testicular cancer need a way to accurately predict the prognosis.
Some researchers have pointed out that the prognosis of patients with testicular cancer is closely related to the clinical stage, pathological type and treatment of the tumor. The International Germ Cell Tumor Cooperative Organization (IGCCCG) has confirmed the use of serum tumor markers in more than 5,000 GCT patients for prognosis studies. The prognosis is divided into three levels (8). More and more researches take various clinical indicators as a sign of prognosis (9-11). However, the prognostic accuracy of these clinical indicators is still not very high (12). These indicators cannot help us understand the mechanism and promote the research of new treatment methods.
In recent years, immunotherapy has become a hot research direction of various tumors, which is also closely related to the role of the immune system in the development of tumors (13). The role of immune surveillance in testicular cancer has been extensively studied (14). Siska et al. proposed in 2017 that infiltration of activated T cells is associated with a good prognosis for seminoma (15). In the past two years, it has been proposed that inflammation indicators can be used as predictive factors for testicular cancer (16,17). Fankhauser et al. found that the apparent activation of PD-1/PD-L1 signaling is present in testicular cancer tissue (18). In addition, studies have reported that patients with low PD-L1 expression have better PFS, which means that immune indicators can be used as predictors of prognosis in patients with testicular cancer (19). More excitingly, many cases have reported that PD-L1 inhibitors can be used to treat patients with testicular cancer who are not sensitive to radiotherapy and chemotherapy (17,20,21).
Although many studies have revealed the prognostic value of the immune system in patients with testicular cancer, no one has used high-throughput sequencing results to build a prognostic model based on immune-related genes (IRGs). In other tumors, many prognostic models based on IRGs have been well established and have high prognostic value (22,23). Therefore, our research aims to establish a prognostic model of testicular cancer patients based on IRGs and verify its clinical application value. We present the following article in accordance with the STROBE reporting checklist (available at http://dx.doi.org/10.21037/atm-20-654).
Methods
Acquisition and preparation of data
Transcriptome profiling data and related clinical information of TGCT were downloaded from The Cancer Genome Atlas (TCGA) Data Portal (https://tcga-data.nci.nih.gov/tcga/; accessed December 2019). Transcriptome data for normal tissues were obtained from the Genetype-Tissue Expression (GTEx) Data Portal (https://gtexportal.org/; accessed December 2019). From the ImmPort database (https://www.immport.org/home; accessed December 2019), we obtained 2,498 genes related to immunity. For the obtained transcriptome data, we used R software for standardization and used the “Limma” package for analysis of differentially expressed genes (DEGs). Further, we combined gene expression files with clinical information based on the TCGA id of TCGT patients in the TCGA database. Next, we extracted the expression of 2,498 IRGs in TGCT patients and the clinical information corresponding to the patients. Transcriptome data and corresponding clinical data of TGCT patients for external verification were obtained from GEO database (GSE3218 and GSE10783). The “sva” package in R software was used for batch normalization of gene expression data.
Identification of IRGs with prognostic value
The univariate Cox regression analysis was used to identify genes with prognostic value from differentially expressed IRGs. We used the “survival” package in R software for univariate Cox regression analysis.
Identification differently expressed TFs and construction of a regulatory network between TFs and IRGs
The Cistrome database (http://www.cistrome.org/) is a comprehensive resource for predicted TF (transcription factor) targets and enhancer profiles in cancers. The prediction was from integrative analysis of TCGA expression profiles and public ChIP-seq profiles. Differently expressed TFs were carried out by using “Limma” package in R statistical software between TCGT and non-tumor samples. Correlation analysis between differently expressed TFs and prognostic differently expressed IRGs was performed by the R programming language. Moreover, only correlations that satisfied a correlation coefficient of at least 0.4 and at the same time satisfy a P value of less than 0.001 were considered significant meaningful.
Establishment of an independent prognostic index (PI, riskScore) based on IRGs in training cohort
Firstly, we excluded patients with a follow-up time of less than 90 days in the TCGA database. Then, we randomly divided TGCT patients with complete records of clinical information such as age, race, pathology stage, tumor type, Stage of Serum Marker and lymphovascular invasion status into training cohort and testing cohort. From the perspective of the distribution of patients in various clinical features, the patient composition in the training cohort and the patient composition in the testing cohort were similar.
In order to further identify the key prognostic IRGs, the multivariate Cox regression analysis was performed. According to the weight of each gene in multivariate Cox regression analysis, we obtained the correlation coefficient in the model formula for predicting the prognosis of patients. Combined with the expression of various prognosis-related genes, we established an independent prognostic model. The PI was calculated using the following formula: β1 × gene1 expression + β2 × gene2 expression + … + βn × genen expression, where β corresponded to the correlation coefficient.
Evaluation of the prognostic index in the training cohort
Firstly, we used The Human Protein Atlas (HPA) database (https://www.proteinatlas.org/) to verify the protein level of key IRGs in the established prognosis index.
According to our prognostic model, each patient would get a riskScore. We set the median riskScore as the cutoff value for dividing TGCT patients into a high-risk group and a low-risk group. Kaplan-Meier (K-M) method was utilized to plot the survival curves. The log-rank test was performed to assess differences in the survival rates between high-risk group and low-risk group. The receiver operating characteristic curves (ROC) were created by the “survivalROC” package, the area under the curve (AUC) values were calculated to evaluate the specificity and sensitivity of the model. The riskScore distribution of patients, Survival status scatter plots for patients in the prognostic model and the heatmap of prognosis-related IRGs were also displayed.
Verification of the prognostic ability of this model in the testing cohort and the entire TCGA cohort
First of all, to verify the performance of the prognostic model in the testing cohort, we used the “survivalROC” package in the R programming language to make a time-dependent ROC curve. We also used the “survival” package and “survminer” package to draw the survival curve. The riskScore distribution of patients in the prognostic model, survival status scatter plots for patients in the prognostic model and the heatmap of prognosis-related IRGs were also displayed.
We then repeated the verification work done on the testing cohort throughout the entire TCGA cohort. In addition, we also used principal component analysis (PCA) to reduce the dimensions of all expressed genes so that we could explore whether our model can successfully distinguish all TGCT patients in TCGA dataset.
In order to further evaluate the predictive ability of the prognostic index we established in patients with different survival times and different clinical types. We additionally performed time-dependent ROC and the survival curves based on patients with different tumor types, patients with different serum marker levels and patients with different lymphovascular invasion status respectively.
Then, the correlation between the prognostic model (risk genes and riskScore) and each clinical feature was analyzed to illustrate the reliability of the model we built. The correlation analysis between riskScore and tumor immune cell infiltration was also made to explore whether our model can reflect the immune microenvironment of tumor patients.
To further evaluate whether our model could be used as an independent prognostic factor, we included age, stage, race, stage, type, stage of serum marker, T, M, N, lymphovascular invasion status and riskScore as independent variables. And then we did univariate cox regression analysis and multivariate cox regression analysis on the changes of progression-free survival time and progression-free survival outcome.
External validation in the GEO cohort
In order to further verify whether our model can play a good role in predicting prognosis in other TGCT cohorts, we obtained an independent TGCT cohort from the GEO database (GSE3218 and GSE10783). Due to the lack of data about the progress-free survival, we explored the relationship between the model we established and the OS (overall survival) of patients in the cohort. The survival analysis of OS was realized and visualized through the “survival” package and “survminer” package in the R software. The riskScore distribution of patients, Survival status scatter plots and the heatmap of prognosis-related IRGs were also displayed. In addition, we also carried out ROC curve analysis through the “survivalROC” package to evaluate the diagnostic accuracy of the model.
GSEA enrichment analysis
Gene-set enrichment analysis (GSEA) was used to explore the mechanisms that lead to different outcomes between patients in the high-risk group and patients in the low-risk group.
Nomogram development and validation for prognostic risk prediction
By means of “rms” package of R software, a prognostic nomogram was also performed to visualize the relationship between individual predictors and progression-free survival rates in patients with TGCT based on the Cox proportional hazard regression model.
Statistical analysis
Statistical analyses of all data utilized in this article were completed by R software (version 3.5.1, https://www.r-project.org/). When the difference met a joint satisfaction of FDR <0.05 and |log2fold changes (FC)| >1, it was regarded to be statistically significant. The univariate Cox regression analysis and multivariate Cox regression analysis were used to evaluate the relationship between IRGs expression and survival data to establish a prognostic model. “rms” package of R software was used to create the nomogram. The ROC were created by the “survivalROC” package of R software and AUC values were also calculated by this package. If the AUC >0.60, we would consider this model to have a certain predictive ability. If the AUC >0.75, we would consider this prediction model to have excellent predictive value. The rank correlation among the different variables was assessed with the Pearson correlation coefficient test. All statistical tests were two-sided and P<0.05 was considered to be statistically significant.
Results
Differentially expressed prognostic IRGs
The flow diagram for this study was displayed in Figure S1. Through the online TCGA database, we obtained the RNA sequences and clinical information of 156 TGCT samples. Gene expression files for the other 165 normal testicular samples were obtained from the GTEx database. Table 1 shows the clinical information of included patients. After standardizing the data, we obtained the DEG profile between the TGCT group and the normal group through the “limma” package of the R software. Then, we extracted the information on the differential expression of 2,498 IRGs obtained from the ImmPort database. The filtration conditions (|log2FC| >1, FDR <0.05) were set. Next, 333 differentially expressed IRGs were identified, including 256 up-regulated genes and 77 down-regulated genes (Figure 1). Based on the obtained differentially expressed IRGs, we carried out the univariate Cox regression analysis to identify IRGs related to PFS. Finally, a total of 31 genes were considered to be prognostic IRGs (Table 2).
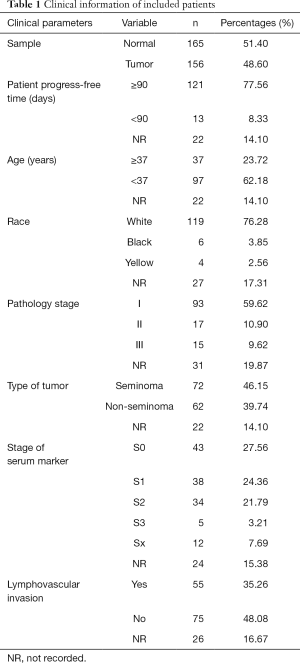
Full table
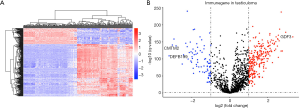
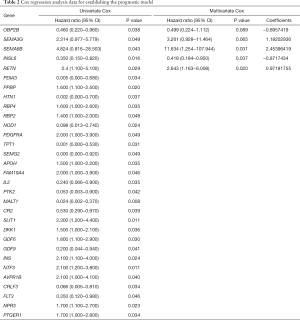
Full table
Differentially expressed TFs
By comparing gene expression data with TFs from Cistrome, we finally obtained the expression of 315 relevant TFs. In order to further screen out valuable differentially expressed TFs, we set the joint satisfaction of FDR <0.05 and |log2FC| >1 to the filtration condition. Heatmap of differently expressed TFs was presented in Figure 2A. A volcano map showing 5 down-regulated and 35 up-regulated differentially expressed TFs was presented in Figure 2B. Next, we analyzed the correlation between the differentially expressed TFs and the differentially expressed prognostic IRGs. The cut-off values (correlation coefficient >0.4 and P value <0.001) were set to clarify the interacting TFs and IRGs. Networks between TFs and IRGs were detailed in Figure 2C.
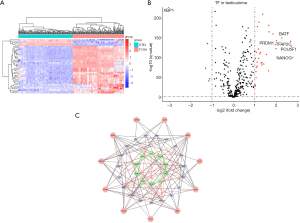
Construction a prognostic model index based on IRGs
After excluding patients with a follow-up survival time of less than 90 days (n=34) and excluding patients with incomplete clinical information (n=11), we divided the remaining patients into two groups with similar composition ratios (79 patients for training cohort, 32 patients for testing cohort).
Immediately afterwards, we performed a multivariate Cox regression analysis on the 31 prognostic-related IRGs by using the information of patients in training cohort, identified the 5 optimal risk genes (Table 2). Among these five risk genes, SEMA6B, SEMA3G, and RETN were considered as predictors of poor prognosis. The higher the expression of these three genes, the worse the prognosis of patients. Two other genes, INSL6 and OBP2B, were protective factors. According to the results of multivariate Cox regression analysis, we obtained the risk coefficient of each differentially expressed IRGs and then constructed a prognostic model index to predict the prognosis of patients with TGCT. The 5 prognostic IRGs related PI formula was as follows: (OBP2B expression) ×(−0.6957419)+ (SEMA3G expression) ×1.18202936+ (SEMA6B expression) ×2.45396419+ (INSL6 expression) ×(−0.8717434)+ (RETN expression) ×0.97181755. The cutoff value was 20.7880594.
Evaluation of the prognostic model index based on IRGs in training cohort and testing cohort
After obtaining the prognostic model index based on IRGs for predicting the prognosis of TGCT patients, we took a series of measures to evaluate the model.
Firstly, we searched The Human Protein Atlas (HPA) database (https://www.proteinatlas.org/) for risk genes and found that at the protein level, the expression of SEMA6B, SEMA3G, and INSL6 was consistent with the results of the mRNA differential analysis we obtained (Figure 3). This indicated that the model we built is to some extent credible.
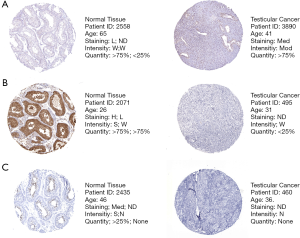
Figure 4A shows the time-dependent ROC curves of riskScore in predicting the prognosis of training cohort patients. In the training cohort, the AUC of the prognostic model at 1, 3 and 5 years were 0.863, 0.803 and 0.798 respectively. Then, we created Kaplan-Meier curves based on the log-rank test to visualize the prognostic value of our established prognostic model in training cohort (Figure 4B). Figure 4C shows the result of risk classification of patients in training cohort according to riskScore. From Figure 4D we found that as the riskScore increases, the number of patients with tumor progression increases. The expression patterns of the risk genes in the high-risk group and the low-risk group were shown in the form of a heat map (Figure 4E), from which we found that in the training cohort, SEMA6B, SEMA3G and RETN were up-regulated in the high-risk group, down-regulated in the low-risk group. The patterns of INSL6 and OBP2B were the opposite. Figure 5A shows the time-dependent ROC curves of riskScore in predicting the prognosis of testing cohort patients. In the testing cohort, the AUC of the prognostic model at 1, 3 and 5 years were 0.728, 0.768 and 0.736 respectively. Figure 5B shows Kaplan-Meier curves based on the log-rank test in testing cohort. Figure 5C shows the result of risk classification of patients in testing cohort according to riskScore. From Figure 5D we found that in testing cohort, as the riskScore increases, the number of patients with tumor progression increases. The expression patterns of the risk genes in the high-risk group and the low-risk group were shown in the form of a heat map (Figure 5E), from which we found that in the testing cohort, SEMA6B, SEMA3G and RETN were up-regulated in the high-risk group, down-regulated in the low-risk group. The patterns of INSL6 and OBP2B were the opposite. From the survival curves, we could see that whether in the training cohort or in the testing cohort, patients in the high-risk group (41 in training cohort and 14 in testing cohort) have worse prognosis than those in the low-risk group (38 in training cohort and 18 in testing cohort) (HR =1.3, 95% CI, 1.1−1.5, P=0.001; HR =1.6, 95% CI, 1.1−2.3, P=0.035).
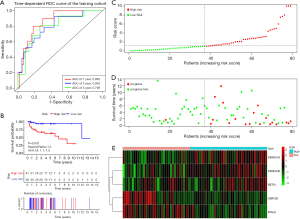
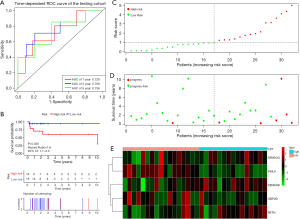
Evaluation of the prognostic model index based on IRGs in entire TCGA cohort
Figure 6 shows the preliminary validation results of the performance of the prognostic model in all TCGA patients. According to the riskScore, 60 patients were included into the high-risk group, 61 patients were included into the low-risk group. A Kaplan-Meier curve based on the log-rank test and the ROC curve of multiple prognostic indicators were created to visualize the prognostic value of our established prognostic model in all TCGA patients (Figure 6A,B). The AUC values of riskScore and stage based on serum marker were 0.815 and 0.736 respectively. It was worth mentioning that the predictive ability of the prognostic model we established was better than the predictive ability of the stage based on serum marker. To further demonstrate the correctness of the discrimination between the high-risk group and the low-risk group which was divided by riskScore, we used PCA to reduce the dimensions of all genes. We found interestingly that the high-risk group and the low-risk group clearly distinguished (Figure 6C). Figure 6D shows the result of risk classification of patients according to riskScore. From Figure 6E we could found that as the riskScore increases, more patients with tumor progression. The expression patterns of the risk genes in the high-risk group and the low-risk group were shown in the form of a heat map (Figure 6F), from which we found that SEMA6B, SEMA3G, and RETN were up-regulated in the high-risk group, down-regulated in the low-risk group. The expression patterns of INSL6 and OBP2B were the opposite.
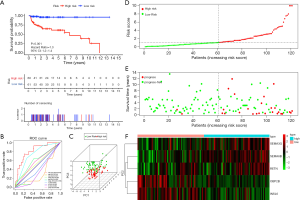
Further, in order to better illustrate the universality of the application of our prognostic model, we did a time-dependent ROC curve analysis of the entire TCGA cohort (Figure 7A,B,C). The AUCs at 1, 3 and 5 years were 0.855, 0.843 and 0.843 respectively. At the same time, we stratified all TGCT patients according to tumor type, stage based on serum markers, lymphovascular invasion status. Then, Kaplan-Meier curve analysis was performed respectively (Figure 7D,E,F,G,H,I). Figure 7D,G plot the survival curves of patients with seminoma and patients with non-seminoma, respectively. Figure 7E,H plot survival curves for patients with normal serum markers (S0) and patients with abnormal serum markers (S1-3), respectively. Figure 7F,I, respectively, plot survival curves of patients without lymphovascular invasion and patients with lymphovascular invasion.
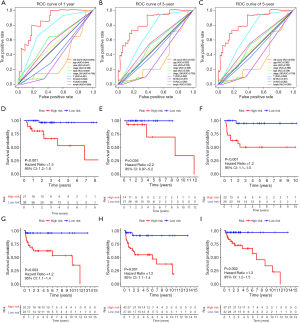
Evaluation of the prognostic model index based on IRGs in external cohort
We obtained an independent TGCT cohort containing the gene expression data of 108 patients and corresponding survival data from the GEO database. Figure 8A,B,C show the prognostic value of riskScore in GEO cohort. According to the model we established in TCGA cohort, every patient in GEO cohort got a corresponding risk score. Finally, 71 patients were included into the high-risk group, 37 patients were included into the low-risk group. The Kaplan-Meier curve (Figure 8A) shows that riskScore was significantly associated with prognosis (P=0.003). Throughout the follow-up period, the survival rate of patients in the high-risk group was lower than in the low-risk group. The riskScore distribution, survival status and risk gene expression in GEO cohort are displayed in Figure 8B. Similar to the results obtained in TCGA cohort, SEMA3G, SEMA6B, RETN were up-regulated in the high-risk group, down-regulated in the low-risk group. The patterns of INSL6 and OBP2B were the opposite. Figure 8C shows the result of ROC analysis. The AUC was 0.702. In addition, we further investigated whether each risk gene is related to the prognosis of TGCT. Figure 8D,E,F,G,H show that four of the five risk genes are significantly related to prognosis.
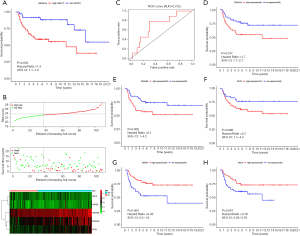
Clinical correlation and immune correlation analysis
Based on all testicular tumor patients in the TCGA database, the correlation analysis between risk factors in the prognostic model we constructed (the riskScore and each component gene) and clinical characteristics such as tumor types, stage based on serum markers, stage, lymphovascular invasion status, TMN was performed. Figure 9 showed the results of the analysis. We found that both the riskScore and SEMA3G expression were significantly correlated with the stage based on serum markers (Figure 9A,B). SEMA3G and INSL6 were significantly related to tumor types (Figure 9D).
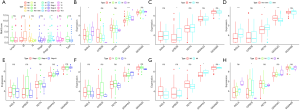
In addition, in order to assess whether our prediction model could indicate the status of the tumor’s immune microenvironment, we performed a correlation analysis between the riskScore and immune cell content of testicular tumor patients in TCGA (Figure 10). As the riskScore increased, both the number of infiltrating B cells and the number of CD8 + T-cells in testicular tumor tissue gradually decreased (Figure 10A,C).
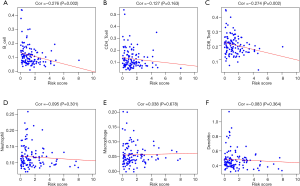
Independent prognostic factor evaluation and GSEA enrichment analysis
To further evaluate whether our model could be used as an independent prognostic factor, we included some key clinical characteristics containing age, race, stage, histological type, stage based on serum markers, T, M, N, lymphovascular invasion status and riskScore as independent variables. By means of univariate and multivariate Cox regression analysis, our established PI (riskScore) remained significant (both P<0.001, Figure 11A,B). At the same time, the results of stage based on serum markers in univariate Cox regression analysis and multivariate Cox regression analysis also showed that it could be used as an independent prognostic indicator (both P<0.05, Figure 11A,B). Looking only at the results of multivariate Cox regression analysis, we could find that riskScore, stage based on serum markers and lymphovascular invasion status were related to prognosis (P<0.001, P=0.005, P=0.039 respectively; Figure 11B).
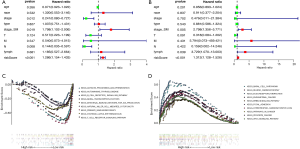
In addition, in order to further explore the possible mechanisms that caused different outcomes in the high-risk group and the low-risk group, we performed GSEA on the gene expression profiles of the two groups of patients. Figure 11C plots enriched pathways in the low-risk group, while Figure 11D plots enriched pathways in the high-risk group. The results of GSEA suggested that most of the DEGs in the high-risk group patients were enriched in cancer pathways and cardiovascular disease-related pathways, and many of the DEGs in the low-risk group were genes related to immune pathways and transcription factors.
Nomogram development and validation
Finally, to better predict the 1-year PFS, 3-year PFS and 5-year PFS of TCGT patients, we constructed a new Nomogram based on the results of the multivariate Cox regression analysis of independent prognostic factors (Figure 12A). Figure 12B shows the Calibration curves of the nomogram for the probability of PFS at 1, 3 and 5 years. The C-index of the nomogram for PFS prediction was 0.780 (95% CI, 0.70–0.86), while the C-index of riskScore for PFS prediction was 0.763 (95% CI, 0.67–0.86).
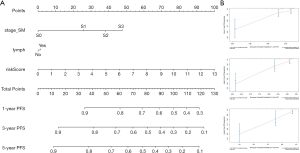
Discussion
As the role of the immune system in tumorigenesis continues to be explored, the phenomenon that the immune system is activated in tumors and then further mediates immune cells to kill tumor cells has been widely recognized (24). The expression of IRGs in tumors directly reflects the state of the immune system in tumors. Therefore, the use of IRGs as a prognostic factor for tumors is convincing. Our study is the first research to establish a prognostic model based on IRGs in patients with TGCT. With the development and wide application of high-throughput sequencing technology, many other genetic prognostic models have also been established in testicular cancer, such as prognostic models based on microRNA expression patterns and prognostic models based on genes from altered genomic regions (25,26). Unlike other studies, we mainly focused on the changes in the expression of IRGs. In addition to the outstanding prognostic value, our research could guide the clinical exploration of immunotherapy for testicular cancer to a certain extent.
In order to obtain a reliable prognostic model, our research used a scientific and rigorous method to establish a prognostic model. Then we carried out comprehensive verifications of the established model. Firstly, due to the particularity of testicular tumor treatment, the TCGA database only contains gene expression data for testicular tumor tissues. In order to solve this problem, we combined the gene expression data of normal testicular tissues in GTEx database and the tumor tissues data in TCGA database to conduct a difference analysis. Secondly, we divided the TCGT patients in the TCGA database into training cohort and testing cohort based on the prognostic clinical indicators indicated in the EAU guidelines (27). Among them, the proportion of patients in the training cohort for each clinical indicator is similar to the testing cohort. The prognostic risk genes were trained from the training cohort and verified in both the testing cohort and the entire TCGA cohort. In the end, we obtained five risk genes (SEMA6B, SEMA3G, OBP2B, INSL6 and RETN). we also successfully used immunohistochemistry to verify the expression differences of three of the risk genes at the protein expression level. Regardless of whether it was in the training cohort, testing cohort or entire TCGA cohort, for predicting the PFS of TGCT patients, the ROC curve analysis and survival analysis of our model showed excellent results. Furthermore, we also performed PCA analysis, time-dependent multi-index ROC curve analysis and survival curve analysis in patients with different clinical characteristics in entire TCGA cohort. In addition, we also conducted external validation of the model. The results of the above verifications showed that our model was reliable and widely applicable. It was worth noting that from the analysis of the ROC curve, in addition to the prognosis model, the stage based on the levels of the serum marker also had a higher prognosis value, while other clinical indicators had lower prognostic value. However, the AUC of our prognostic model was higher than the AUC of the stage based on the serum marker levels. Thirdly, we further evaluated the correlation between prognostic models (including risk genes and riskScore) and clinical variables. We found interestingly that the riskScore and SEMA3G were significantly related to stage based on the serum markers, INSL6 and SEMA3G were significantly related to tumor types. Therefore, the model we established showed high clinical applicability. Correlation analysis between prognostic model and immune cells was also implemented. The analysis results showed that there was a significant negative correlation between riskScore and immune cells (B cell and CD8+ T-cell). Which also proved that the infiltration of immune cells could affect the prognosis of patients with testicular cancer, suggesting that the immune system plays an important role in the development of testicular cancer.
Finally, univariate Cox regression analysis and multivariate Cox regression analysis identified riskScore, stage based on the serum marker levels and lymphovascular invasion status as independent prognostic factors. We created a new Nomogram to better predict the 1-year PFS, 3-year PFS and 5-year PFS of TCGT patients based on riskScore, stage based on the serum marker levels and lymphovascular invasion status. Consistency analysis shows that this new Nomogram has a higher prognostic value than the riskScore.
For the five risk genes we obtained (SEMA6B, SEMA3G, OBP2B, INSL6 and RETN), we made further exploration. SEMA6B is related to the development of the nervous system. Some studies have reported that SEMA6B is a gene related to macrophages and has a high prognostic value in glioma (28). A recent article mentioned that SEMA3G has a higher prognostic value in clear cell renal cell carcinoma and is a protective factor (22). Liu et al. proposed in 2015 that SEMA3G can inhibit the migration and invasion of tumor cells through the action of PPAR-gamma, which explains to some extent why SEMA3G can be used as a protective prognostic factor for renal clear cell carcinoma (29). However, in our prognostic model, SEMA3G seems to play the opposite role, its mechanism needs to be further studied. OBP2B, most studies believe that it is related to immunoglobulin E-related allergic reactions, acting as the binding site of some small molecules (30). As to whether OBP2B can be used as a prognostic factor for tumors, there are no related reports. INSL6, in addition to OBP2B, is the other protective factor in our prognostic model. INSL6 is highly expressed in the testis, it interacts with MAEA and can promote macrophage maturation (31). The immune effect of INSL6 was confirmed in mouse experiments (32). RETN is the popular IRG in recent years. It is associated with poor prognosis in diseases such as glioma and type 2 diabetes (33,34).
In order to better understand the mechanism of this prognostic model, we not only constructed a transcription factor regulatory network, but also made the difference analysis and GSEA analysis between the gene expression profile of patients in the high-risk group and the gene expression profile of patients in the low-risk group. From the results of the GSEA analysis, we were surprised to find that the poor prognosis of patients in the high-risk group may be related to some cancer signaling pathways and cardiovascular disease signaling pathways. Which also explained why patients with testicular cancer have a long-term risk of secondary malignant tumors and secondary cardiovascular disease (5-7). On the other hand, the better prognosis of patients in the low-risk group may be caused by changes in immune-related pathways. Which further illustrated the high clinical value of our prognostic model.
However, our study still has some limitations: The results of our study were only validated in the TCGA database and GEO database. Retrospective data analysis made our prediction model valuable in the training set. Whether it had real clinical application value, the model requires more data support from clinical patients. In addition, the mechanism by which immunity affects the prognosis of testicular cancer patients needs to be further explored through in vivo and in vitro experiments.
Conclusions
All in all, we have successfully established a risk model (riskScore) based on 5 IRGs, which could accurately predict the prognosis of patients with TGCT. A nomogram combining clinical variables and riskScore was also drawn to improve the accuracy of the prediction. We found it interesting that different immune status in tumor microenvironment may be responsible for the different survival outcomes among TGCT patients. Our research shed new light to the prospects for the application of immunotherapy in patients with testicular cancer. However, further experiments are also required to validate our findings.
Acknowledgments
We would like to thank the researchers and study participants for their contributions.
Funding: This article was funded by the National Natural Science Foundation of China (grant number: 81871151).
Footnote
Reporting Checklist: The authors have completed the STROBE reporting checklist. Available at http://dx.doi.org/10.21037/atm-20-654
Conflicts of Interest: All authors have completed the ICMJE uniform disclosure form (available at http://dx.doi.org/10.21037/atm-20-654). The authors have no conflicts of interest to declare.
Ethical Statement: The authors are accountable for all aspects of the works in ensuring that questions related to the accuracy and integrity of any part of the work are appropriately investigated and resolved.
Open Access Statement: This is an Open Access article distributed in accordance with the Creative Commons Attribution-NonCommercial-NoDerivs 4.0 International License (CC BY-NC-ND 4.0), which permits the non-commercial replication and distribution of the article with the strict proviso that no changes or edits are made and the original work is properly cited (including links to both the formal publication through the relevant DOI and the license). See: https://creativecommons.org/licenses/by-nc-nd/4.0/.
References
- Cheng L, Albers P, Berney DM, et al. Testicular cancer. Nat Rev Dis Primers 2018;4:29. [Crossref] [PubMed]
- Albany C, Adra N, Snavely AC, et al. Multidisciplinary clinic approach improves overall survival outcomes of patients with metastatic germ-cell tumors. Ann Oncol 2018;29:341-6. [Crossref] [PubMed]
- Adra N, Abonour R, Althouse SK, et al. High-Dose Chemotherapy and Autologous Peripheral-Blood Stem-Cell Transplantation for Relapsed Metastatic Germ Cell Tumors: The Indiana University Experience. J Clin Oncol 2017;35:1096-102. [Crossref] [PubMed]
- Capocaccia R, Gatta G, Dal Maso L. Life expectancy of colon, breast, and testicular cancer patients: an analysis of US-SEER population-based data. Ann Oncol 2015;26:1263-8. [Crossref] [PubMed]
- Travis LB, Fossa SD, Schonfeld SJ, et al. Second cancers among 40,576 testicular cancer patients: focus on long-term survivors. J Natl Cancer Inst 2005;97:1354-65. [Crossref] [PubMed]
- Ross DM, Arthur C, Burbury K, et al. Chronic myeloid leukaemia and tyrosine kinase inhibitor therapy: assessment and management of cardiovascular risk factors. Intern Med J 2018;48 Suppl 2:5-13. [Crossref] [PubMed]
- Lauritsen J, Hansen MK, Bandak M, et al. Cardiovascular Risk Factors and Disease After Male Germ Cell Cancer. J Clin Oncol 2020;38:584-92. [Crossref] [PubMed]
- Bosl GJ, Motzer RJ. Testicular germ-cell cancer. N Engl J Med 1997;337:242-53. [Crossref] [PubMed]
- Adra N, Althouse SK, Liu H, et al. Prognostic factors in patients with poor-risk germ-cell tumors: a retrospective analysis of the Indiana University experience from 1990 to 2014. Ann Oncol 2016;27:875-9. [Crossref] [PubMed]
- Fizazi K, Culine S, Kramar A, et al. Early predicted time to normalization of tumor markers predicts outcome in poor-prognosis nonseminomatous germ cell tumors. J Clin Oncol 2004;22:3868-76. [Crossref] [PubMed]
- Loriot Y, Pagliaro L, Flechon A, et al. Patterns of relapse in poor-prognosis germ-cell tumours in the GETUG 13 trial: Implications for assessment of brain metastases. Eur J Cancer 2017;87:140-6. [Crossref] [PubMed]
- Boormans JL, Mayor de Castro J, Marconi L, et al. Testicular Tumour Size and Rete Testis Invasion as Prognostic Factors for the Risk of Relapse of Clinical Stage I Seminoma Testis Patients Under Surveillance: a Systematic Review by the Testicular Cancer Guidelines Panel. Eur Urol 2018;73:394-405. [Crossref] [PubMed]
- Sharma P, Allison JP. The future of immune checkpoint therapy. Science 2015;348:56-61. [Crossref] [PubMed]
- Chovanec M, Mardiak J, Mego M. Immune mechanisms and possible immune therapy in testicular germ cell tumours. Andrology 2019;7:479-86. [PubMed]
- Siska PJ, Johnpulle RAN, Zhou A, et al. Deep exploration of the immune infiltrate and outcome prediction in testicular cancer by quantitative multiplexed immunohistochemistry and gene expression profiling. Oncoimmunology 2017;6:e1305535. [Crossref] [PubMed]
- Fankhauser CD, Sander S, Roth L, et al. Systemic inflammatory markers have independent prognostic value in patients with metastatic testicular germ cell tumours undergoing first-line chemotherapy. Br J Cancer 2018;118:825-30. [Crossref] [PubMed]
- Chovanec M, Cierna Z, Miskovska V, et al. Systemic immune-inflammation index in germ-cell tumours. Br J Cancer 2018;118:831-8. [Crossref] [PubMed]
- Fankhauser CD, Curioni-Fontecedro A, Allmann V, et al. Frequent PD-L1 expression in testicular germ cell tumors. Br J Cancer 2015;113:411-3. [Crossref] [PubMed]
- Cierna Z, Mego M, Miskovska V, et al. Prognostic value of programmed-death-1 receptor (PD-1) and its ligand 1 (PD-L1) in testicular germ cell tumors. Ann Oncol 2016;27:300-5. [Crossref] [PubMed]
- Zschäbitz S, Lasitschka F, Hadaschik B, et al. Response to anti-programmed cell death protein-1 antibodies in men treated for platinum refractory germ cell cancer relapsed after high-dose chemotherapy and stem cell transplantation. Eur J Cancer 2017;76:1-7. [Crossref] [PubMed]
- Zschäbitz S, Lasitschka F, Jager D, et al. Activity of immune checkpoint inhibition in platinum refractory germ-cell tumors. Ann Oncol 2016;27:1356-60. [Crossref] [PubMed]
- Wan B, Liu B, Huang Y, et al. Prognostic value of immune-related genes in clear cell renal cell carcinoma. Aging (Albany NY) 2019;11:11474-89. [Crossref] [PubMed]
- Bai S, Yan YB, Chen W, et al. Bioinformatic Analysis Reveals an Immune/Inflammatory-Related Risk Signature for Oral Cavity Squamous Cell Carcinoma. J Oncol 2019;2019:3865279. [Crossref] [PubMed]
- Gentles AJ, Newman AM, Liu CL, et al. The prognostic landscape of genes and infiltrating immune cells across human cancers. Nat Med 2015;21:938-45. [Crossref] [PubMed]
- Korkola JE, Heck S, Olshen AB, et al. Development and Validation of a Gene-Based Model for Outcome Prediction in Germ Cell Tumors Using a Combined Genomic and Expression Profiling Approach. PLoS One 2015;10:e0142846. [Crossref] [PubMed]
- Ling H, Krassnig L, Bullock MD, et al. MicroRNAs in Testicular Cancer Diagnosis and Prognosis. Urol Clin North Am 2016;43:127-34. [Crossref] [PubMed]
- Albers P, Albrecht W, Algaba F, et al. Guidelines on Testicular Cancer: 2015 Update. Eur Urol 2015;68:1054-68. [Crossref] [PubMed]
- Sun X, Liu X, Xia M, et al. Multicellular gene network analysis identifies a macrophage-related gene signature predictive of therapeutic response and prognosis of gliomas. J Transl Med 2019;17:159. [Crossref] [PubMed]
- Liu W, Li J, Liu M, et al. PPAR-gamma promotes endothelial cell migration by inducing the expression of Sema3g. J Cell Biochem 2015;116:514-23. [Crossref] [PubMed]
- Curin M, Weber M, Hofer G, et al. Clustering of conformational IgE epitopes on the major dog allergen Can f 1. Sci Rep 2017;7:12135. [Crossref] [PubMed]
- Lampert F, Stafa D, Goga A, et al. The multi-subunit GID/CTLH E3 ubiquitin ligase promotes cell proliferation and targets the transcription factor Hbp1 for degradation. Elife 2018;7:e35528. [Crossref] [PubMed]
- Brailoiu GC, Dun SL, Yin D, et al. Insulin-like 6 immunoreactivity in the mouse brain and testis. Brain Res 2005;1040:187-90. [Crossref] [PubMed]
- Kapłon-Cieślicka A, Tyminska A, Rosiak M, et al. Resistin is a prognostic factor for death in type 2 diabetes. Diabetes Metab Res Rev 2019;35:e3098. [PubMed]
- Vachher M, Arora K, Burman A, et al. NAMPT, GRN, and SERPINE1 signature as predictor of disease progression and survival in gliomas. J Cell Biochem 2020;121:3010-23. [Crossref] [PubMed]