Pulmonary vascular enlargement on thoracic CT for diagnosis and differential diagnosis of COVID-19: a systematic review and meta-analysis
Introduction
Over the past 20 years, the world has witnessed three large-scale coronavirus outbreaks, including severe acute respiratory syndrome (SARS), Middle East respiratory syndrome (MERS), and now the 2019 novel coronavirus disease (COVID-19), which is caused by severe acute respiratory syndrome coronavirus 2 (SARS-CoV-2). In December 2020, COVID-19, a viral disorder characterized by fever, dry cough, fatigue, dyspnea, and myalgia, was first identified and officially reported in Wuhan, Hubei Province, China. With strong measures taken by Chinese government and efforts of medical staff, the China’s outbreak centered by Hubei Province has gradually improved (1). But as virus sees no national boundaries, currently, COVID-19 has become a global pandemic, leading to over 9 million confirmed cases and over 400 thousand deaths. According to the World Health Organization (WHO) reports in June, 2020, Americas are the worst-hit places, followed by Europe, Eastern Mediterranean, South-East Asia, Africa and Western Pacific (2). Recently, it was declared a public health emergency of international concern (PHEIC) by the chief of the World Health Organization (WHO), thus ascending to the highest level of global alarm (3). To combat this disease, a united effort is needed now more than ever.
As computed tomography (CT) has features of noninvasiveness, quick speed, high resolution, and easy access, it is recommended by experts for THE first-line screening of suspected COVID-19 patients (4,5). Recently, many descriptive studies, case series, and literature reviews have reported and summarized typical CT manifestations of COVID-19. The common CT features already identified for COVID-19 include multifocal or unifocal patchy and round-shaped ground glass opacity (GGO) or consolidation lesion, along with reticulation or interlobular septal thickening, usually with a bilateral, peripheral, subpleural, lower, and posterior distribution (4,6). Special classic CT signs, including “crazy paving”, “vascular thickening”, “air-bronchogram”, “bronchiectasis or bronchus distortion”, “fibrosis”, “halo” or “reversed halo”, may also be typical, while cavitation, nodules, “tree-in-bud”, pleural effusions, and lymphadenopathy are rare (7). Among these CT signs, “vascular enlargement” sign (VES) (8) is found promising to be a typical early CT feature of COVID-19 as reported by Zhao et al. (9) and Hu et al. (10).
VES, also known as “vascular thickening” (11), “vascular enhancement” (12), “micro-vascular dilation” sign (13,14), “bronchovascular enlarged” (15), or “dandelion fruit” sign (16), is often described as the dilatation of pulmonary vessels around and within the lesions in an unnatural way on CT images (17). The vascular issue is also of great concern for COVID-19 patients from clinical perspective. Elevated D-dimer levels and blood hypercoagulability were found to be common among hospitalized COVID-19 patients (18,19). And some acute exacerbation of COVID-19 was revealed to be related to acute pulmonary embolism (20). Besides, Spagnolo et al. (21) have reported that COVID-19 patients with adverse outcome (death) had higher pulmonary artery diameter. In addition, previous work have examined vascular changes on CT in pulmonary neoplasms (22), vascular malformation (23), pulmonary artery hypertension (24,25), smoke-related diseases (26), or hemorrhagic fever (27) for disease diagnosis, evaluation of disease severity, and even prediction of malignancy, suggesting a possible unique diagnostic role for VES. However, to our knowledge, few studies have reported its connection to SARS or MERS, or other coronavirus pneumonia.
If CT manifestation correlates of actual pathologic findings such as vasculitis (28) can be identified, radiologists may be able to diagnose COVID-19 more accurately. With more attention being paid to pulmonary circulation conditions of COVID-19 patients, some causes of death like acute pulmonary embolism may therefore be reduced. Recently, studies on CT features of COVID-19 have been thriving, but only some of them have examined VES proportions, and the results have been varied among those studies. So far, although several systematic reviews and meta-analyses have been published on CT features of COVID-19, none of the studies systematically reported on VES. Therefore, the purpose of this study was to systematically review the literature and to perform a meta-analysis regarding the CT findings on VES of confirmed COVID-19 patients and corresponding suspected or non-COVID-19 patients. We present the following article in accordance with the preferred reporting items for systematic reviews and meta-analyses (PRISMA) reporting checklist (29) (available at http://dx.doi.org/10.21037/atm-20-4955).
Methods
This meta-analysis was carried out in accordance with the preferred reporting items for systematic reviews and meta-analyses (PRISMA) guidelines (30). We formulated a research question that was based on a modification of the patient, index test, comparator, outcome, and study design (PICOS) criteria as follows: (I) with respect to thoracic CT manifestations, is the VES associated with COVID-19 patients? (II) To what extent is it associated with COVID-19 patients compared to corresponding suspected cases or other non-COVID-19 patients?
Protocol
We conducted a systematic literature search in March 2020 as a protocol to evaluate whether there was an appropriate amount of studies with reliable quality for pooling a convincing result.
Literature search
We systematically searched five English-language databases, including PubMed, Ovid, Embase, Scopus, and Web of Science, and four Chinese-language databases, including WanFang Data, CQVIP Database, SinoMed Database, and China National Knowledge Infrastructure (CNKI) up to March 20, 2020, and continued updating the literature search until April 23, 2020. We also screened the references of included studies to find other eligible studies. We set the following retrieval terms according to the basic patient, index test, comparator, outcome, and study design (PICOS) principle elements: P: “COVID-19”, “2019 novel coronavirus”, “SARS-CoV-2”; I: “comput* AND tomogra*”, “CT”, “imaging”, “radiolog*”; O: “pulmonary vessel enlarge*”, “vascular enlarge*”, “enlarge* subsegmental vessel”, “vascular thicken*”, “vascular changes”. Within each principle element, the logical connector “OR” was used, and “AND” was used between different elements for logical connection. As there is no unified or standard definition of VES, some studies reporting VES might not have identified it as such in the keyword section. Thus, we conducted two rounds of literature search in each database: the first round only included “PI” elements to ensure an overall reliable recall level, and the second round included “PIO” elements to ensure the accuracy and to find any possible omission after the first round literature search. We only use “in the last 1 year” or “year=2019-2020” limit to focus on most recent studies published on COVID-19. No other limits or filters were set.
Inclusion criteria
Qualified studies were included if they satisfied the following patient, index test, comparator, outcome, and study criteria: patients had confirmed diagnosis or exclusion of COVID-19; COVID-19 infection was determined or excluded by real-time reverse transcription polymerase chain reaction (RT-PCR) test, high-throughput nucleic acid gene sequencing, IgM or IgG antibodies detection kit, or some combination of these techniques; the study reported patients’ CT findings including VES or compared CT features between COVID-19 and non-COVID-19 patients including VES; and the publication was an original research article written in English or Chinese.
Exclusion criteria
Studies were excluded for the following reasons: the study did not report VES manifestation; the study population included fewer than 10 patients; the publication was not an original research article; researchers only reported other non-COVID-19 coronavirus-related illnesses, such as MERS, SARS; imaging modalities other than CT were used; or the patient population overlapped with that of other studies. If multiple publications had a considerable overlap of study populations, we only included the study that enrolled the highest number of patients.
Data extraction and quality assessment
Two independent investigators (H Lv and T Chen) screened titles, abstracts or full-texts according to the inclusion and exclusion criteria. If there was any disagreement in the process, the final decision was made by a third investigator (H Wang). For the included studies, data were extracted regarding characteristics of study, patient, CT scan, and VES. Study characteristics included origin of study (first author, country, and institution), journal name, year of publication, date of acceptance, total number of enrolled patients, duration of patient recruitment, study design (prospective or retrospective, cross-sectional or case series, multicenter or single center, consecutive or non-consecutive enrollment), and the Joanna Briggs Institute (JBI) quality assessment result. Patient characteristics consisted of number of patients (sorted by SARS-COV-2 confirmation, sex, disease severity, and abnormal CT manifestations), method of pathogen confirmation, source of patients, average age and age range of study population, and comparison characteristics for those studies that included non-COVID-19 patients. CT scan characteristics were image acquisition time, CT scanner model, slice thickness, interval thickness, CT parameters (tube voltage and tube current modulation), use of contrast enhancement, and number of CT readers and their working experience. Imaging characteristics mainly included description of VES and main findings of VES in each of the included studies.
As a greater degree of bias is likely to occur in observational studies, we decided to use both the Joanna Briggs Institute (JBI) Critical Appraisal Checklist for analytical cross-sectional studies (31) and the Quality Assessment of Diagnostic Accuracy Studies-2 (QUADAS-2) tool (32) for detailed methodologic quality assessment. To promote consistent assessments in the usage of QUADAS-2 tool, we developed a rating guideline with operational criteria for each domain (see supplementary QUADAS-2 Quality Assessment Rating Guideline). The JBI evaluation results are listed in tables. The QUADAS-2 evaluation items were interpreted in detail using Review Manager (version 5.3) software, and the results were further exported as graphs from the software. The assessment process was also performed independently by two reviewers (Y Pan and H Wang). Consensus was achieved with the combined use of JBI and QUADAS-2 and through discussion between the two reviewers.
Data synthesis and analysis
For studies that only included COVID-19 patients, data were constructed in a “study, event, n” table. The “event” referred to the number of patients or lesions that had presented with VES in each study. The “n” referred to the total number of patients who had both positive SARS-COV-2 test result and abnormal thoracic CT, or the total number of lesions on thoracic CT of those patients in each study. For studies that included both COVID-19 patients and non-COVID-19 patents, data were reconstructed into a 2×2 contingency table showing the presence or absence of VES in patients with or without COVID-19 infection.
The following statistical processes were all conducted using the ‘meta’ and ‘metafor’ packages of R software (version 3.6.3, R Foundation for Statistical Computing) in R Studio (version 1.2.5042). The random effects model with restricted maximum-likelihood (REML) estimator was used for pooling.
To calculate the pooled VES prevalence and 95% confidence intervals (CIs), logit transformation of the raw proportions was performed in advance to make them conform to a normal distribution. A normal approximation interval based on summary measure (NAsm) method was then used in R software for calculating 95% CIs. The association between the VES and COVID-19 infection was assessed and pooled in the form of an odds ratio (OR) with 95% CIs, also using the random effects model.
For pooled data, statistical heterogeneity between studies was examined with Cochrane’s Q test and the inconsistency index (I2) statistic. For the Q statistic, a P value <0.10 was considered statistically significant for heterogeneity; for I2, a value >50% was considered to show significant heterogeneity (33). Publication bias was evaluated using funnel plot and Egger’s test (34). Asymmetry of the funnel shaped distribution by visual inspection and a P value <0.10 in Egger’s test was considered to indicate statistically significant publication bias.
Potential sources of heterogeneity were first analyzed through leave-one-out analysis i.e., leave one study out at a time, and get the pooling results from the remaining studies to see if there are huge variations after each leave-one-out. Sensitivity analysis, which mainly focused on the variations of the total heterogeneity after leave-one-out, was also conducted. Another adopted method was the influence diagnostic test provided by the ‘metafor’ package, which included calculation of externally standardized residual, DFFITS value, Cook‘s distance, covariance ratio, the leave-one-out amount of (residual) heterogeneity, the leave-one-out test statistic for the test of (residual) heterogeneity, and DFBETAS value. A study may be considered to be statistically influential if at least one of the following is true: the absolute DFFITS value is larger than 3√(p/[k-p]), where p is the number of model coefficients and k the number of studies; the lower tail area of a Chi-square distribution with p degrees of freedom cut off by the Cook’s distance is larger than 50%; the hat value is larger than 3(p/k); any DFBETAS value is larger than one (35,36).
Heterogeneity was further investigated using visual inspection and meta-regression analysis. The covariates selected through visual inspection included average age (over or less than 50-year-old), country (China or Italy), region [Hubei (epicenter) or the rest of the world], sample size (“n” smaller or larger than 50), VES distribution (clearly defined as inside the lesion area or not clearly defined), and slice thickness [no thicker than 1 mm (0–1 mm); greater than 1 mm but no thicker than 3 mm, (1–3 mm); greater than 3 mm; or slice thickness varying within the range of 0.625–5 mm]. Univariate meta-regression analyses were performed to test the individual association of selected covariates with the pooled estimates and to calculate the amount of heterogeneity each covariate accounts for (R2 statistic) (37).
Based on univariate analyses, subgroup analyses were then performed. A multivariate meta-regression model was also developed based on sample size and VES distribution to determine the amount of heterogeneity these two covariates accounted for. Subgroups with no fewer than five studies that provided useful data were considered appropriate for calculating an accurate tau square; otherwise, a common tau square was estimated across subgroups.
Results
Literature search
The search initially identified a total of 3,773 articles, of which 1,934 were duplicates. The remaining 1,839 articles were screened based on title and abstract, and 147 of them eventually underwent full-text review after three main steps were conducted (Figure 1). No additional eligible studies from an extended search of references of included studies were identified for our meta-analysis. There were no disagreements between the two reviewers. Ultimately, a total of 22 studies evaluating 1,638 COVID-19 patients and 331 non-COVID-19 patients (8,9,11,13-15,38-53) were included. Among them, four studies (50-53) had comparisons of VES proportions between COVID-19 and non-COVID-19 patients.
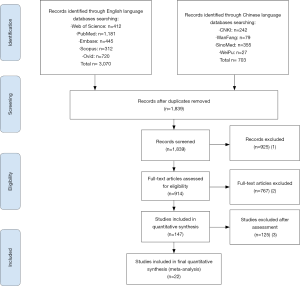
Characteristics of included studies
Study characteristics are shown in Table 1. Overall, 21 studies were retrospective in design, and 1 study was prospective. The sample size of included studies ranged from 10 to 459. Additionally, seven studies were performed at multiple centers. Patient recruitment was consecutive in three studies.
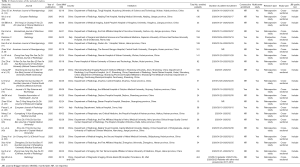
Full table
Patient characteristics are described in Table 2. Patient enrollment took place from January to March in 2020. The source of COVID-19 patients included 11 provinces or municipalities in China and 2 different cities in Italy. Age at diagnosis ranged from 1 to 98 years old. CT acquisition parameters and scanner characteristics are shown in Table 3. Descriptions and main findings on VES of each study are also summarized in Table 4.
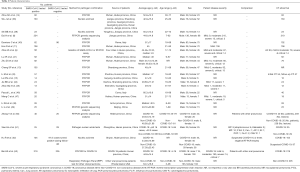
Full table
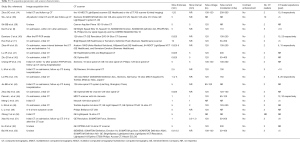
Full table
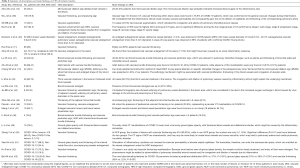
Full table
Quality assessment
Before conducting the quality assessment, a QUADAS-2 quality assessment rating guideline was made according to our study condition and with the consensus of all authors (for more details, readers can read the supplementary online). The quality assessment results according to the JBI checklist are listed in Table 1. All studies met the overall appraisal for inclusion criteria. Quality assessment details using JBI Critical Appraisal Checklist were listed in Table S1. Results of the QUADAS-2 study quality assessment are summarized in Figure 2. There was a certain amount of risk of bias in this meta-analysis, mainly arising from the patient selection and index test domains, as most studies were retrospective and did not clarify a consecutive or random enrollment of patients, or did not describe a blinding method during CT evaluation. Regarding the flow and timing domain, all studies had a low risk of bias.
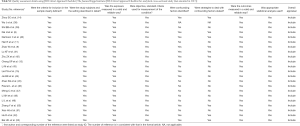
Full table
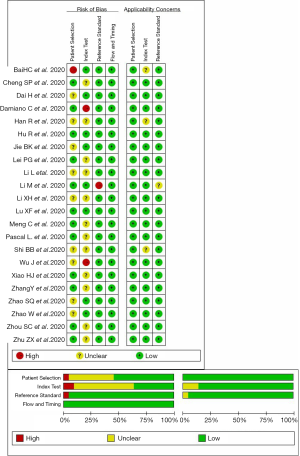
Publication bias was investigated using a funnel plot. A symmetrical distribution of the funnel plot (Figure 3), and the P value >0.10 in Egger’s test (P=0.29) indicated the unlikelihood of publication bias.
Prevalence and OR estimate of VES
VES rates were first calculated for each included study. The results are shown in Table 4. Pooled estimates of VES prevalence were then calculated for all 22 selected studies (8,9,11,13-15,38-53) comprising 1,638 COVID-19 patients. Because the prevalence extracted from those studies ranged from 19.2 to 94.7, logit transformation was performed on the raw prevalence data in advance. The results of the Shapiro–Wilk normality test (W=0.95533, P=0.401) confirmed the normal distribution of the transformed sample data. The overall pooled prevalence of VES in COVID-19 patients was 69.37% (95% CI: 57.40–79.20%) according to the random effects model. The I2 statistic (94%, P<0.01) indicated substantial heterogeneity (Figure 4).
Further meta-analysis of three studies (51-53) after removing an identified outlier (50) showed patients with confirmed COVID-19 infection were more frequently to have VES manifestation on thoracic CT compared with those without COVID-19 infection (OR =6.43, 95% CI: 3.39–12.22, P<0.0001). The I2 statistic (I2=61%, P=0.08) indicated statistically significant heterogeneity (Figure 5).
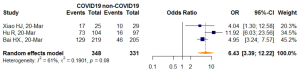
Source of heterogeneity analysis: leave-one-out analysis, influence diagnostic test, and meta-regression
In influential analysis, the leave-one-out results of VES rates were relatively stable (67.04–71.41%) after removing each study (Figure S1), and no statistically significant influence was identified through influence diagnostics among all the 22 included studies for prevalence pooling (Figure S2). However, one significant outlier (50) was identified when only the four studies that compared VESs in COVID-19 and non-COVID-19 patients were involved for pooling ORs (Figure S3); this study was then excluded in the OR calculating process.
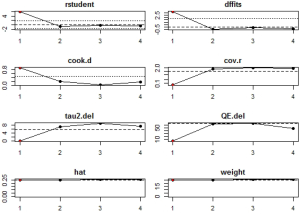
After careful consideration of baseline features of included studies, six categorical covariates were identified as potential sources of heterogeneity. Univariate meta-regression against average age (P=0.665), country (P=0.711), region (P=0.755), VES distribution (P=0.031), sample size (P=0.183), and slice thickness (P=0.963) was conducted. Among them, only the VES distribution was found to have statistically significant effects to the overall pooled result. The R2 (amount of heterogeneity accounted for) for the VES distribution was R2VES=15.33%. Together, the VES, and sample size accounted for 19.73% (R2VES+ sample size=19.73%) of the total amount of heterogeneity.
Variations in VES prevalence: subgroup analysis
Subgroup analyses of all 22 studies according to average age, country, region, VES distribution, sample size, and slice thickness were also conducted. The results are shown in Figures 6,S4-S8.
Studies with a sample size larger than 50 reported a higher VES prevalence of 74.61% (95% CI: 62.68–83.72%), while a rate of 57.24% (95% CI: 32.68–78.68%) was found in studies with sample sizes smaller than 50, but the differences were not statistically significant (P=0.183). When prevalence was stratified by VES distribution, VESs that were explicitly defined as inside the lesion area were shown to have relatively higher prevalence (80.33%; 95% CI: 68.79–88.32%), than VESs that were distributed either inside or outside of the lesion area (59.51%; 95% CI: 42.33–74.64%). The meta-regression (P=0.031) as mentioned before, indicated that this difference was statistically significant, even though a small portion of the two 95%CIs overlapped (Table 5).
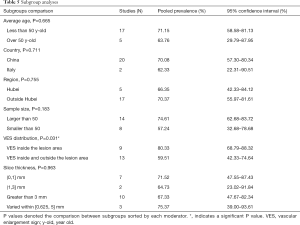
Full table
No significant difference in VES rates was found between patients from different countries (China/Italy), regions (Hubei/outside Hubei), average age groups (over/less than 50-year-old), or among CT images acquired at different slice thicknesses.
Discussion
Our study presents a comprehensive meta-analysis of pulmonary vascular enlargement manifestations on thoracic CT of COVID-19 patients. To the best of our knowledge, this is the first meta-analysis to focus on vascular changes caused by SARS-Cov-2 on thoracic CT. With the accumulation of clinical experience and the proliferation of medical studies, the evidence clearly indicates that COVID-19 patients are at a higher risk of pulmonary vascular damage and blood coagulation dysfunction.
Elevated D-dimer levels and blood hypercoagulability were found to be common among hospitalized COVID-19 patients (18,19), and prominent elevation of D-dimmer and comorbidities relating to blood circulation, such as hypertension, could predict poorer prognosis of COVID-19 (54). With regard to pathologic findings, Dr. Menter et al. published a 21-case post-mortem multi-organ autopsy study in Switzerland (55), reporting exudative diffuse alveolar damage (DAD) with massive capillary congestion in most of the cases and accompanying microthrombi of alveolar capillaries despite anticoagulation in 45% of all cases. Besides this, pulmonary embolisms, alveolar hemorrhage, vasculitis, and signs of disseminated intravascular coagulation (DIC) with small fibrin thrombi in glomerular capillaries were also found. Yao and colleagues have also reported the presence of congested, edematous and widened blood vessels of the alveolar septum, and hyaline thrombi in microvessels in both the lung and kidney (56). Importantly, another pathology study consisting of five cases from the USA by Magro et al. (57) revealed that, apart from DAD with edema, hyaline membranes, inflammation, and type II pneumocyte hyperplasia, which were reported by preliminary studies as features characteristic of typical ARDS, the pulmonary abnormalities in their patients appeared largely restricted to the alveolar capillaries, which is more characteristic of a thrombotic microvascular injury with few signs of viral cytopathic or fibroproliferative changes. Cases of pulmonary embolism and symmetric cutaneous vasculitis in COVID-19 patients were also reported by Dr. Rotzinger (20) and Dr. Castelnovo (58), respectively.
On thoracic CT, VES was usually defined as blood vessels seen thickening and passing through or passing by the ground glass opacity (GGO), the probable pathological basis of which might be congestion of alveolar septal capillaries (59). Our meta-analysis across 22 studies included a total of 1,969 patients from China, the United States (U.S.), and Italy in regions inside or outside of Hubei (the epicenter) who had undergone non-contrast thoracic CT scans. Herein, we paid special attention to vascular features in those studies in order to ascertain the vascular changes observed by CT and to explore their role in diagnosis. Before synthesis, we strictly followed the QUADAS-2 critical appraisal tool and JBI checklist to define the methodologic quality of each study. We strictly applied inclusion and exclusion criteria and up-to-date estimates using a random effects model with logit transformed values. We found that the overall pooled prevalence of VES among COVID-19 patients was 69.37% (95% CI: 57.40–79.20%). Because the number (four) of studies that reported VES proportions in non-COVID-19 patients was considered small and the enrolled non-COVID-19 patient characteristics of each study varied, the VES prevalence was not pooled in the non-COVID-19 group. After exclusion of one outlier, which had a different, yet much larger sample size (No. of lesions) and reported a much greater VES rate than the other three, the OR pooling results showed that VES manifestation was more frequently observed in COVID-19 patients than in non-COVID-19 patients, (OR =6.43, 95% CI: 3.39–12.22). The main descriptions and findings of VES are systematically summarized in Table 4.
After pooling, heterogeneity was detected and analyzed using a meta-regression model and subgroup analysis. Interestingly, VES distribution was found as a source of heterogeneity (R2=15.33%). Studies that explicitly defined VES in the lesion area pooled a significantly higher prevalence (80.33%, 95% CI: 68.79–88.32%) than studies without a clear definition of VES distribution (59.51%, 95% CI: 42.33–74.64%) (P=0.03), which might indicate a possible underestimation of VES prevalence when lacking an established standard.
Subgroup analyses also revealed a relatively higher VES rate in studies with a sample size larger than 50, but the difference was not statistically significant. No significant difference in VES rates was found between patients from different countries (China/Italy), regions (Hubei/outside Hubei), average age groups (over/less than 50-year-old), or among images acquired at different CT slice thicknesses. The above non-significant moderators also suggested that VES prevalence was relatively stable regardless of patient age, sample size, country, region, CT scan slice thicknesses.
VES findings in COVID-19 patients with different clinical severities or at different disease stages were not analyzed due to limited study materials. However, as reported by Zhou et al. (14) and Zhao et al. (9) no significant differences of VES rates were found among patients at the early phase (no more than 7 days after symptom onset) or advanced phase (8–14 days after symptom onset) of COVID-19, or among non-emergency groups (mild and common clinical types) and emergency groups (severe and fatal clinical types) of COVID-19.
Although the specific physiopathologic mechanisms of VES remain unclear, previous evidence has shown that SARS-CoV-2 had a much stronger ability to combine with angiotensin-converting enzyme 2 (ACE2) receptor compared with SARS-CoV-1 (60), which indicates a higher chance of immunoreaction in the vessels. Reduced expression of ACE2 in the vasculature may also promote endothelial dysfunction and inflammation and exacerbate existing atherosclerosis and diabetes (61-65). As reported by Magro et al., extensive deposition of complement components within the lung septal microvasculature might result in membrane attack complex-mediated microvascular endothelial cell injury and subsequent activation of the clotting pathway (57). Although the term “vascular enlargement” for chest CT might be non-specific and was interchangeably reported by different studies, as mentioned by Salehi et al. (66), our results suggest that considering VES along with other specific CT manifestations of COVID-19 would be very helpful for the diagnosis and differential diagnosis of COVID-19.
Some limitations were identified in this meta-analysis. First, a great degree of heterogeneity was identified across most estimates (I2>80%), with only about 20% of the heterogeneity being attributable to VES distribution, and sample size. This leaves the other factors that contributed to large remaining portion of heterogeneity unidentified, making it difficult to obtain valid and stable meta-analysis results despite the use of a standardized analysis process. Second, quality assessment showed that many involved studies were retrospective. Thus, a consecutive enrollment of patients or a blind method in CT evaluation during the study period was not always applied. Third, we did not compare VES findings in patients with different clinical severities or at different disease stages of COVID-19 infection due to the insufficient figures found in the studies. Fourth, even though we tried our best to search for all eligible studies available online without any nationality restriction, we only got study populations coming from China, U.S., or Italy. With COVID-19 becoming a global pandemic, future studies are encouraged to involve patient population from more different countries. Furthermore, to our knowledge, vascular enlargement sign (VES) has not been uniformly described in the widely read glossaries of thoracic imaging (67), and thus requires a standard definition in the near future. Future meta-analysis should include more prospective cohort studies to control the possible bias during evaluation and lower the heterogeneity across studies.
Conclusions
Pulmonary VES on thoracic CT was found in almost two-thirds of the COVID-19 patients and was more prevalent in COVID-19 patients than in non-COVID-19 patients. While the physiopathologic mechanisms remain unclear, the current findings suggest a promising role of VES for identifying pneumonia caused by coronavirus and indicate that more attention should be paid to pulmonary vascular changes in thoracic CT–based diagnosis.
Supplementary
QUADAS-2 Quality Assessment Rating Guideline (32,68)
Domain 1: patient selection
Signaling questions and answering guidelines
Was a consecutive or a random sample of persons enrolled?
Since CT examination is usually taken as a recommendation rather than a must-do test for all/consecutive COVID-19 patients, it is acceptable that original studies focusing on CT manifestations will not have to enroll all/consecutive COVID-19 patients, but patients with a CT scan. Therefore, it will not be considered a high risk of bias when the study excludes patients without available CT results.
Answer ‘yes’ if one of the following conditions is met.
- It is explicitly stated in the study report that enrolment was consecutive (or random).
- It is reported that all eligible, screened, or potential study participants with a CT scan were included, and that enrollment took place at all hours on any day during the enrolment period.
Answer ‘no’ if neither of the conditions is met.
Answer ‘unclear’ if insufficient information is available to answer ‘yes’ or ‘no’.
Was a case-control design avoided?
This question is irrelevant because studies with case-control design are excluded from the review.
Did the study avoid inappropriate exclusions?
Answer ‘yes’ if both of the following conditions are met.
- The appropriate exclusion criteria are explicitly explained in the study.
- No exclusions that are unrelated to execution of the index test (e.g. fear of radiation exposure, inability to be positioned, sex or age restriction).
Answer ‘no’ if neither of the conditions is met.
Answer ‘unclear’ if insufficient information is available to answer ‘yes’ or ‘no’.
Guidelines for assessing risk of bias
Risk of bias from patient selection will be assessed as ‘low’ when signaling question 1 and 3 are answered ‘yes’.
Risk will be assessed as ‘high’ when signaling question 1 or 3 is answered ‘no’.
Risk will be assessed as ‘unclear’ when insufficient information is reported to answer signaling question 1 or 3.
Guidelines for assessing concern regarding applicability
Is there concern that the included patients do not match the review question?
Concern regarding applicability in relation to patient selection will be assessed as ‘low’ when the study population represents an unselected sample of patients with suspected COVID-19. Because the study question concerns the CT manifestation for diagnosing COVID-19 in the general population, exclusion of children or persons with diabetes etc. will be considered inappropriate. By contrast, we do not consider it inappropriate if persons with extreme a priori probabilities of COVID-19 or non-COVID-19 are excluded. As stated in the background section, it is probably in persons with intermediate a priori probability that CT has the greatest role in guiding decisions on management. Finally, exclusion of severely or acutely ill persons and persons with mental incapacities is not considered inappropriate. If inappropriate exclusions account for 5% or less of the number of included persons, the potential impact of inappropriate exclusions will be considered negligible.
Concern will be assessed as ‘high’ when the study population does not represent an unselected sample of adults with suspected COVID-19.
Concern will be assessed as ‘unclear’ when insufficient information is available.
Domain 2: index test
Signaling questions and answering guidelines
Were the index test results interpreted without knowledge of the results of the reference standard?
For practical reasons, COVID-19 is highly contagious and a CT-scan must take place with communication and good cooperation between doctors and patients. Hence, it is often necessary for doctors to be aware of the potential infectious status of the patients before CT scan. However, a third person who is involved only in the evaluation scenario and not in the diagnosis procedure is considered to have low risk of bias.
Answer ‘yes‘ if one of the following conditions is met.
- The CT evaluations used in the analyses were performed before the patient had laboratory confirmation of certain pathogens.
- The CT evaluations used in the analyses were postponed evaluations or reevaluations, and the radiologists were kept unaware of laboratory findings and of whether persons had a certain lung infection.
Answer ‘no’ if neither of the conditions is met.
Answer ‘unclear’ if insufficient information is available to answer ‘yes’ or ‘no’.
If a threshold was used, was it pre-specified?
Answer ‘yes’ if the following two conditions are met.
- The components (e.g., distribution, size, shape of lung lesion; characteristics of lung lesion, esp. the VES sign) included in the evaluation of the CT-scan are explicitly reported in the study report.
- The hierarchy and logical combination of components are explicitly reported in the study report.
Answer ‘no’ if one or more of the conditions above are not met.
Answer ‘unclear’ if insufficient information is available to answer ‘yes’ or ‘no’.
Guidelines for assessing risk of bias
Risk of bias from index test execution will be assessed as ‘low’ when signaling questions 1 and 2 are answered ‘yes’.
Risk will be assessed as ‘high’ when signaling question 1 or 2 is answered ‘no’.
Risk will be assessed as ‘unclear’ when insufficient information is reported to answer signaling questions 1 or 2.
Guidelines for assessing concern regarding applicability
Two issues will influence our assessment concerning applicability in relation to execution of the index test.
Is the index test described in sufficient detail to permit its replication?
Answer ‘yes’ when the following details are reported.
- Number of slices of the CT device.
- Use of multi-planar reformations (assumed not used if the number of slices of the CT device is lower than 16, unless stated otherwise).
- Taken in the supine position and at full-inhalation.
- Region included in the scan (involve entire lung, from the inlet of thoracic to costophrenic angles).
- Slice thickness, slice interval, tube voltage (kilovolt, kv), and tube current modulation (milliampere second, mAs).
Answer ‘no’ if one or more of the details listed above (I to V) are not described.
Answer ‘unclear’ if insufficient information is available to answer ‘yes’ or ‘no’.
Was the report of CT signs (e.g., VES sign) accurate?
Answer ‘yes’ if following two conditions are met.
- CT signs are clearly defined and explicitly illustrated in the study.
- CT readers have no fewer than 5 years of experience.
Answer ‘no’ if the analysis is based on a reassessment of the CT-scan by a senior radiologist or a consensus panel.
Answer ‘unclear’ if insufficient information is available to answer ‘yes’ or ‘no’.
Concern regarding applicability in relation to index test execution will be assessed as ‘low’ when questions 1 and 2 are answered ‘yes’.
Concern will be assessed as ‘high’ when question 1 or 2 is answered ‘no’.
Concern will be assessed as ‘unclear’ when insufficient information is reported to answer questions 1 and 2.
Domain 3: reference standard
Signaling questions and answering guidelines
Is the reference standard likely to correctly classify the target condition?
Answer ‘yes’ if the following conditions are met.
- The diagnosis of COVID-19 infection is based on the pathogen gene sequencing or RT-PCR test. Also classify as ‘yes’ if the diagnosis of COVID-19 is based on IgG or IgM kit for SARS-COV-2 specific antibody examination.
- The diagnosis of COVID-19 in patients who did not prove initially positive for the above tests is based on clinical follow-up and repeated lab pathogen tests.
Answer ‘no’ if the diagnosis of COVID-19 (or its absence; i.e., non-COVID-19) is not based on the conditions stated above.
Answer ‘unclear’ if insufficient information is available to answer ‘yes’ or ‘no’.
Were the reference standard results interpreted without knowledge of the results of the index test?
Answer ‘yes’ if the laboratory technicians performing the RT-PCR or the pathogen gene sequencing work in different departments from the radiologists and are kept unaware of the results of the CT-scan.
Answer ‘no’ if one of the relevant conditions stated above is not met.
Answer ‘unclear’ if insufficient information is available to answer ‘yes’ or ‘no’.
Guidelines for assessing risk of bias
Risk of bias related to the reference standard will be assessed as ‘low’ when signaling questions 1 or 2 is answered ‘yes’.
Risk will be assessed as ‘high’ when both signaling question 1 and 2 are answered ‘no’.
Risk will be assessed as ‘unclear’ when insufficient information is reported to answer signaling questions 1 and 2.
Guidelines for assessing concern regarding applicability
Is there concern that the target condition as defined by the reference standard does not match the review question?
Sometimes, due to the quick spread of the pandemic, there may be a shortage of lab diagnostic kits, and the quality control of kits may be lax.
Concern regarding applicability in relation to patient selection will be assessed as ‘low’ when the lab diagnostic procedure or the production of diagnostic kit is clearly reported.
Concern will be assessed as ‘unclear’ when insufficient information is available.
Concern will be assessed as ‘high’ when neither the lab diagnostic procedure nor the production of diagnostic kit is reported in the study.
Domain 4: flow and timing
Signaling questions and answering guidelines
Did all persons receive a reference standard?
Answer ‘yes’ if at least 95% of included persons had pathogen gene sequencing, RT-PCR test, IgG or IgM kit for SARS-COV-2 specific antibody examination, or clinical follow-up.
Answer ‘no’ if fewer than 95% of included persons had pathogen gene sequencing, RT-PCR test, IgG or IgM kit for SARS-COV-2 specific antibody examination, or clinical follow-up.
Answer ‘unclear’ if insufficient information is available to answer ‘yes’ or ‘no’.
Did all persons receive the same reference standard?
Answer ‘yes’ if one of the following conditions is met.
- 90% of included persons had pathogen gene sequencing, RT-PCR test, or IgG or IgM kit examination.
- 90% of included persons were managed by clinical follow-up.
Answer ‘no’ if neither of the conditions is met.
Answer ‘unclear’ if insufficient information is available to answer ‘yes’ or ‘no’.
Was there an appropriate interval between the index test and reference standard?
The appropriate time interval between the CT-scan and laboratory tests is unclear. To our knowledge, CT is usually more sensitive to detecting signs of infection than laboratory tests. Even though CT is not considered as the golden standard of COVID-19, it is advised that a CT scan should be performed in timely fashion. After careful consideration, we generally consider both the CT scan conducted on admission and in follow-up as acceptable regardless of the time period from symptom onset to admission.
Were all patients included in the analysis?
Answer ‘yes’ if the analyses encompassed all included persons. Also, answer ‘yes’ if 5% or fewer were excluded from the analysis because no reference standard assessment was available (to accommodate signaling question 1).
Answer ‘no’ if the requirement stated above is not met.
Answer ‘unclear’ if insufficient information is available to answer ‘yes’ or ‘no’.
Guidelines for assessing risk of bias
Risk of bias related to patient flow and timing will be assessed as ‘low’ when three of above signaling questions are answered ‘yes’.
Risk will be assessed as ‘high’ when signaling question 1, 2, or 4 is answered ‘no’.
Risk will be assessed as ‘unclear’ when insufficient information is reported to answer signaling questions 1, 2, or 4.
Acknowledgments
Funding: This work was supported by Shanghai Jiao Tong University (2020RK66), the Action Plan of Major Diseases Prevention and Treatment (2017ZX01001-S12), and the Shanghai Municipal Health Commission (ZHYY-ZXYJHZX-201901).
Footnote
Reporting Checklist: The authors have completed the PRISMA reporting checklist. Available at http://dx.doi.org/10.21037/atm-20-4955
Conflicts of Interest: All authors have completed the ICMJE uniform disclosure form (available at http://dx.doi.org/10.21037/atm-20-4955). The authors have no conflicts of interest to declare.
Ethical Statement: The authors are accountable for all aspects of the work in ensuring that questions related to the accuracy or integrity of any part of the work are appropriately investigated and resolved.
Open Access Statement: This is an Open Access article distributed in accordance with the Creative Commons Attribution-NonCommercial-NoDerivs 4.0 International License (CC BY-NC-ND 4.0), which permits the non-commercial replication and distribution of the article with the strict proviso that no changes or edits are made and the original work is properly cited (including links to both the formal publication through the relevant DOI and the license). See: https://creativecommons.org/licenses/by-nc-nd/4.0/.
References
- Ye Q, Wang B, Mao J, et al. Epidemiological analysis of COVID-19 and practical experience from China. J Med Virol 2020;92:755-69. [Crossref] [PubMed]
- WHO. Coronavirus disease (COVID-19) outbreak situation. Available online: https://www.who.int/emergencies/diseases/novel-coronavirus-2019. Accessed June 24, 2020.
- WHO. Statement on the second meeting of the International Health Regulations (2005) Emergency Committee regarding the outbreak of novel coronavirus (2019-nCoV). Available online: https://www.who.int/news-room/detail/30-01-2020-statement-on-the-second-meeting-of-the-international-health-regulations-(2005)-emergency-committee-regarding-the-outbreak-of-novel-coronavirus-(2019-ncov). Accessed May 16, 2020.
- Jin YH, Cai L, Cheng ZS, et al. A rapid advice guideline for the diagnosis and treatment of 2019 novel coronavirus (2019-nCoV) infected pneumonia (standard version). Mil Med Res 2020;7:4. [PubMed]
- Shi HS, Yu J, Zheng CS, et al. Radiological Diagnosis of New Coronavirus Infected Pneumonitis: Expert Recommendation from the Chinese Society of Radiology (First edition). Zhonghua Fang She Xue Za Zhi 2020:279-85.
- Salehi S, Abedi A, Balakrishnan S, et al. Coronavirus Disease 2019 (COVID-19): A Systematic Review of Imaging Findings in 919 Patients. AJR Am J Roentgenol 2020;215:87-93. [Crossref] [PubMed]
- Li M. Chest CT features and their role in COVID-19. Radiol Infect Dis 2020. [Epub ahead of print]. [PubMed]
- Dai H, Zhang X, Xia J, et al. High-resolution Chest CT Features and Clinical Characteristics of Patients Infected with COVID-19 in Jiangsu, China. Int J Infect Dis 2020;95:106-12. [Crossref] [PubMed]
- Zhao W, Zhong Z, Xie X, et al. Relation Between Chest CT Findings and Clinical Conditions of Coronavirus Disease (COVID-19) Pneumonia: A Multicenter Study. AJR Am J Roentgenol 2020;214:1072-7. [Crossref] [PubMed]
- Hu Q, Guan H, Sun Z, et al. Early CT features and temporal lung changes in COVID-19 pneumonia in Wuhan, China. Eur J Radiol 2020;128:109017. [Crossref] [PubMed]
- Han R, Huang L, Jiang H, et al. Early Clinical and CT Manifestations of Coronavirus Disease 2019 (COVID-19) Pneumonia. AJR Am J Roentgenol 2020.1-6. [Crossref] [PubMed]
- Qanadli SD, Beigelman-Aubry C, Rotzinger DC. Vascular Changes Detected With Thoracic CT in Coronavirus Disease (COVID-19) Might Be Significant Determinants for Accurate Diagnosis and Optimal Patient Management. AJR Am J Roentgenol 2020;215:W15. [PubMed]
- Cheng SP, Duan YH, Yao JK, et al. Chest CT features of 105 patients with COVID-19: a multicenter retrospective study in Shandong province. Shandong Da Xue Xue Bao (Yi Xue Ban) 2020;58:38-45.
- Zhou S, Wang Y, Zhu T, et al. CT Features of Coronavirus Disease 2019 (COVID-19) Pneumonia in 62 Patients in Wuhan, China. AJR Am J Roentgenol 2020;214:1287-94. [Crossref] [PubMed]
- Lei P, Huang Z, Liu G, et al. Clinical and computed tomographic (CT) images characteristics in the patients with COVID-19 infection: What should radiologists need to know? J Xray Sci Technol 2020;28:369-81. [Crossref] [PubMed]
- Fu XB, Li JS, Huang Z, et al. Dandelion clock-like sign on CT for diagnose of COVID-19. Nan Fang Yi Ke Da Xue Xue Bao 2020;40:159-63. [PubMed]
- Ye Z, Zhang Y, Wang Y, et al. Chest CT manifestations of new coronavirus disease 2019 (COVID-19): a pictorial review. Eur Radiol 2020. [Epub ahead of print]. [Crossref] [PubMed]
- Han H, Yang L, Liu R, et al. Prominent changes in blood coagulation of patients with SARS-CoV-2 infection. Clin Chem Lab Med 2020;58:1116-20. [Crossref] [PubMed]
- Terpos E, Ntanasis-Stathopoulos I, Elalamy I, et al. Hematological findings and complications of COVID-19. Am J Hematol 2020;95:834-47. [Crossref] [PubMed]
- Rotzinger DC, Beigelman-Aubry C, von Garnier C, et al. Pulmonary embolism in patients with COVID-19: Time to change the paradigm of computed tomography. Thromb Res 2020;190:58-9. [Crossref] [PubMed]
- Spagnolo P, Cozzi A, Foà RA, et al. CT-derived pulmonary vascular metrics and clinical outcome in COVID-19 patients. Quant Imaging Med Surg 2020;10:1325-33. [Crossref] [PubMed]
- Gao F, Li M, Ge X, et al. Multi-detector spiral CT study of the relationships between pulmonary ground-glass nodules and blood vessels. Eur Radiol 2013;23:3271-7. [Crossref] [PubMed]
- Saboo SS, Chamarthy M, Bhalla S, et al. Pulmonary arteriovenous malformations: diagnosis. Cardiovasc Diagn Ther 2018;8:325-37. [Crossref] [PubMed]
- Grosse C, Grosse A. CT findings in diseases associated with pulmonary hypertension: a current review. Radiographics 2010;30:1753-77. [Crossref] [PubMed]
- Wells JM, Washko GR, Han MK, et al. Pulmonary arterial enlargement and acute exacerbations of COPD. N Engl J Med 2012;367:913-21. [Crossref] [PubMed]
- Iyer KS, Newell JD Jr, Jin D, et al. Quantitative Dual-Energy Computed Tomography Supports a Vascular Etiology of Smoking-induced Inflammatory Lung Disease. Am J Respir Crit Care Med 2016;193:652-61. [Crossref] [PubMed]
- Aktaş T, Aktaş F, Özmen Z, et al. Does Crimean-Congo Hemorrhagic Fever Cause a Vasculitic Reaction with Pulmonary Artery Enlargement and Acute Pulmonary Hypertension? Lung 2016;194:807-12. [Crossref] [PubMed]
- Boraschi P. COVID-19 Pulmonary Involvement: Is Really an Interstitial Pneumonia? Acad Radiol 2020. [Epub ahead of print]. [Crossref] [PubMed]
- Moher D, Liberati A, Tetzlaff J, et al. Preferred Reporting Items for Systematic Reviews and Meta-Analyses: The PRISMA Statement. PLoS Med 2009;6:e1000097. [Crossref] [PubMed]
- Liberati A, Altman DG, Tetzlaff J, et al. The PRISMA statement for reporting systematic reviews and meta-analyses of studies that evaluate health care interventions: explanation and elaboration. PLoS Med 2009;6:e1000100. [Crossref] [PubMed]
- Moola S, Munn Z, Tufanaru C, et al. Joanna Briggs Institute Reviewer’s Manual. Chapter 7: Systematic reviews of etiology and risk. The Joanna Briggs Institute; 2017. Available online: https://reviewersmanual.joannabriggs.org/
- Whiting PF, Rutjes AW, Westwood ME, et al. QUADAS-2: a revised tool for the quality assessment of diagnostic accuracy studies. Ann Intern Med 2011;155:529-36. [Crossref] [PubMed]
- Higgins JP, Thompson SG. Quantifying heterogeneity in a meta-analysis. Stat Med 2002;21:1539-58. [Crossref] [PubMed]
- Page M, Higgins J, Sterne J. Cochrane Handbook for Systematic Reviews of Interventions version 6.0 (updated July 2019). Chapter 13: Assessing risk of bias due to missing results in a synthesis. Cochrane; 2019. Available online: https://training.cochrane.org/handbook
- Viechtbauer W. Conducting Meta-Analyses in R with the metafor Package. J Stat Softw 2010;36:1-48. [Crossref]
- Viechtbauer W, Cheung MW. Outlier and influence diagnostics for meta-analysis. Res Synth Methods 2010;1:112-25. [Crossref] [PubMed]
- Raudenbush SW. Analyzing effect sizes: Random effects models. In: Harris C, Larry VH, Jeffrey CV. editors. The handbook of research synthesis and meta-analysis. 2nd ed. New York: Russell Sage Foundation, 2009:295-315.
- Wu J, Pan J, Teng D, et al. Interpretation of CT signs of 2019 novel coronavirus (COVID-19) pneumonia. Eur Radiol 2020. [Epub ahead of print]. [Crossref] [PubMed]
- Shi BB, Fu JX, Sun J, et al. CT imaging analysis of 23 patients with novel coronavirus pneumonia in Yangzhou area. Shi Yong Lin Chuang Yi Yao Za Zhi 2020;24:10-2.
- Caruso D, Zerunian M, Polici M, et al. Chest CT Features of COVID-19 in Rome, Italy. Radiology 2020. [Epub ahead of print].
- Lu XF, Gong W, Wang L, et al. Clinical features and high resolution CT imaging findings of preliminary COVID-19. Zhong Hua Fang She Xue Za Zhi 2020;54:296-9.
- Zhu ZX, Zhang Q, Xu W, et al. Analysis of CT Findings of 82 Cases of Novel Coronavirus Pneumonia in Wuhan. Xi Nan Da Xue Xue Bao 2020. [Epub ahead of print].
- Li M, Peng WX, Chen MM, et al. CT imaging features and image evolution characteristics of coronavirus disease 2019. Zhong Nan Da Xue Xue Bao (Yi Xue Ban) 2020;45:243-9. [PubMed]
- Jie B, Liu X, Suo H, et al. Clinical and Dynamic Computed Tomography Features of 24 Patients With Coronavirus Disease 2019. Can Assoc Radiol J 2020. [Epub ahead of print]. [Crossref] [PubMed]
- Zhao SQ, Zhou YS, Yin L, et al. Clinical characteristics and CT findings of COVID-19. Fen Zi Ying Xiang Xue Za Zhi 2020;43:59-63.
- Lomoro P, Verde F, Zerboni F, et al. COVID-19 pneumonia manifestations at the admission on chest ultrasound, radiographs, and CT: single-center study and comprehensive radiologic literature review. Eur J Radiol Open 2020;7:100231. [Crossref] [PubMed]
- Meng C, Chen YX, Liu K. CT features of COVID-2019: a study of 20 cases. Guangdong Yi Xue 2020;41:791-6.
- Li XH, Qiu XH, Zhang JP, et al. Clinical presentations and CT features of COVID-19. Shou Du Yi Ke Da Xue Xue Bao 2020;41:257-60.
- Li L, Wang K, Ren MJ, et al. Early chest CT manifestations of COVID-19. Shou Du Yi Ke Da Xue Xue Bao 2020;41:174-7.
- Zhang Y, Nan CR, Liu HX, et al. Re-discussion about CT signs of novel coronavirus pneumonia. Lin Chuang Hui Cui 2020;35:106-12.
- Xiao HJ, Gu YB, Liang P, et al. Value of CT signs and dynamic changes in the differential diagnosis of suspected COVID-19 cases. Zhengzhou Da Xue Xue Bao (Yi Xue Ban) 2020. [Epub ahead of print].
- Hu R, Huang N, Chen W, et al. Comparison of chest CT images between confirmed and suspected cases of COVID-19. Zhong Hua Fang She Xue Za Zhi 2020;54:440-4.
- Bai HX, Hsieh B, Xiong Z, et al. Performance of radiologists in differentiating COVID-19 from viral pneumonia on chest CT. Radiology 2020;296:E46-E54. [PubMed]
- Zhou F, Yu T, Du R, et al. Clinical course and risk factors for mortality of adult inpatients with COVID-19 in Wuhan, China: a retrospective cohort study. Lancet 2020;395:1054-62. [Crossref] [PubMed]
- Menter T, Haslbauer JD, Nienhold R, et al. Post-mortem examination of COVID19 patients reveals diffuse alveolar damage with severe capillary congestion and variegated findings of lungs and other organs suggesting vascular dysfunction. Histopathology 2020. [Epub ahead of print]. [Crossref] [PubMed]
- Yao XH, Li TY, He ZC, et al. A pathological report of three COVID-19 cases by minimal invasive autopsies. Zhonghua Bing Li Xue Za Zhi 2020;49:411-7. [PubMed]
- Magro C, Mulvey JJ, Berlin D, et al. Complement associated microvascular injury and thrombosis in the pathogenesis of severe COVID-19 infection: a report of five cases. Transl Res 2020. [Epub ahead of print]. [Crossref] [PubMed]
- Castelnovo L, Capelli F, Tamburello A, et al. Symmetric cutaneous vasculitis in COVID-19 pneumonia. J Eur Acad Dermatol Venereol 2020. [Epub ahead of print]. [Crossref] [PubMed]
- Li M, Lei P, Zeng B, et al. Coronavirus Disease (COVID-19): Spectrum of CT Findings and Temporal Progression of the Disease. Acad Radiol 2020;27:603-8. [Crossref] [PubMed]
- Yan R, Zhang Y, Li Y, et al. Structural basis for the recognition of SARS-CoV-2 by full-length human ACE2. Science 2020;367:1444-8. [Crossref] [PubMed]
- Zhang C, Zhao YX, Zhang YH, et al. Angiotensin-converting enzyme 2 attenuates atherosclerotic lesions by targeting vascular cells. Proc Natl Acad Sci U S A 2010;107:15886-91. [Crossref] [PubMed]
- Yousif MH, Dhaunsi GS, Makki BM, et al. Characterization of Angiotensin-(1-7) effects on the cardiovascular system in an experimental model of type-1 diabetes. Pharmacol Res 2012;66:269-75. [Crossref] [PubMed]
- Sahara M, Ikutomi M, Morita T, et al. Deletion of angiotensin-converting enzyme 2 promotes the development of atherosclerosis and arterial neointima formation. Cardiovasc Res 2014;101:236-46. [Crossref] [PubMed]
- Lovren F, Pan Y, Quan A, et al. Angiotensin converting enzyme-2 confers endothelial protection and attenuates atherosclerosis. Am J Physiol Heart Circ Physiol 2008;295:H1377-84. [Crossref] [PubMed]
- Dong B, Zhang C, Feng JB, et al. Overexpression of ACE2 enhances plaque stability in a rabbit model of atherosclerosis. Arterioscler Thromb Vasc Biol 2008;28:1270-6. [Crossref] [PubMed]
- Salehi S, Abedi A, Gholamrezanezhad A. Reply to “Vascular Changes Detected With Thoracic CT in Coronavirus Disease (COVID-19) Might Be Significant Determinants for Accurate Diagnosis and Optimal Patient Management”. AJR Am J Roentgenol 2020;215:W16. [Crossref] [PubMed]
- Hansell DM, Bankier AA, MacMahon H, et al. Fleischner Society: glossary of terms for thoracic imaging. Radiology 2008;246:697-722. [Crossref] [PubMed]
- Rud B, Vejborg TS, Rappeport ED, et al. Computed tomography for diagnosis of acute appendicitis in adults. Cochrane Database Syst Rev 2019;2019.