Dissociation between airway and systemic autoantibody responses in chronic obstructive pulmonary disease
Introduction
Chronic obstructive pulmonary disease (COPD) is one of the most prevalent inflammatory lung disorders worldwide. The World Health Organization (WHO) predicts that the prevalence of COPD will rise over the next 40 years and there may be over 5.4 million deaths annually from COPD and related conditions by 2060 (1), which will impose a heavy economic and social burden. COPD is associated with local (airway) and systemic inflammation (2) and autoimmune processes may participate in the pathogenesis of the disease (3). Airway/pulmonary inflammation in COPD patients involves both innate (neutrophils, macrophages, eosinophils, and other types of immune cell) and adaptive (T and B lymphocytes) immunity (4). Although the prevailing spillover hypothesis has been challenged (5-7), the overflow of pulmonary inflammatory mediators into the circulation is still considered to be the origin of the systemic inflammation (8) in COPD and to account for many of its systemic manifestations, such as osteoporosis, skeletal muscle weakness, cardiovascular disease and cachexia (8,9).
Autoimmunity has been hypothesized to drive disease progression in COPD (3) and there is increasing evidence that it plays a role in the pathogenesis of this disease (10-13). Previous experimental research has shown a correlation between peripheral T cell function and the severity of COPD (14,15). Moreover, systemic T cell responses against a series of autoantigens in COPD patients have been demonstrated (16-18). Most previous studies focused on the circulating autoantibodies in COPD, and clinical associations of these autoantibodies were widely reported (19-23). However, clinical associations of the sputum autoantibodies were seldom reported, and the relationship between local/airway and systemic/circulation autoantibody responses in COPD patients are not yet clear.
The aim of the current study was to elucidate the relationship between local/airway and systemic/circulating autoantibody responses in COPD by quantifying autoantibodies in sputum and serum obtained simultaneously from the same individual. We also explored the potential associations between sputum/serum autoantibodies and clinical parameters such as lung function, symptom scores, and prior exacerbations. We present the following article in accordance with the STROBE reporting checklist (available at http://dx.doi.org/10.21037/atm-20-944).
Methods
Participants
The current observational, cross-sectional study was performed in the Guangzhou Institute of Respiratory Disease, First Affiliated Hospital of Guangzhou Medical University (Guangzhou, China) between August 2016 and June 2018. Forty-seven patients with stable COPD were recruited for original analysis. A second cohort of 55 patients with stable COPD was recruited for validation, and a group of 59 non-COPD controls and a group of 20 connective-tissue-disease-associated interstitial lung disease (CTD-ILD) patients were also recruited for comparison. The inclusion criteria for COPD patients were as follows: (I) age over 40 years and (II) confirmed diagnosis of COPD with spirometry according to the criteria of Global Initiative for Chronic Obstructive Lung Disease (GOLD) guideline [post-bronchodilator (post-BD) forced expiratory volume in 1 s (FEV1)/forced vital capacity (FVC) ratio <0.7]. Patients with one or more of the following criteria were excluded: (I) a current primary diagnosis of asthma, but patients with a primary diagnosis of COPD who also had asthma or positive bronchodilation tests were included; (II) diagnosis of known respiratory disorders other than COPD except asthma, such as active tuberculosis, idiopathic pulmonary arterial hypertension, and ILD; (III) history of significant inflammatory disease other than COPD, such as inflammatory bowel disease (Crohn’s disease and ulcerative colitis), systematic lupus erythematosus, rheumatoid arthritis, and autoimmune thyroiditis; (IV) COPD exacerbation within 4 weeks of enrolment; (V) history of lung surgery; (VI) diagnosis of cancer; (VII) recipient of a blood transfusion within 4 weeks of enrollment; (VIII) inability to walk, and (IX) current participation in a blinded drug trial.
The inclusion criteria for control subjects were as follows: (I) age over 40 years and (II) without any known respiratory disorder or significant inflammatory disease. Subjects with one or more of the following criteria were excluded: (I) diagnosis of known respiratory disorders; (II) history of significant inflammatory disease; (III) diagnosis of cancer; (IV) recipient of a blood transfusion within 4 weeks of enrollment; (V) inability to walk, and (VI) current participation in a blinded drug trial.
The inclusion criteria for CTD-ILD patients were as follows: (II) age over 40 years and (II) diagnosis of CTD-ILD by multidisciplinary team (MDT). Patients with one or more of the following criteria were excluded: (I) diagnosis of known respiratory disorders other than CTD-ILD, such as COPD, asthma, active tuberculosis, idiopathic pulmonary arterial hypertension, and ILD; (II) history of significant inflammatory disease other than CTD; (III) infection within 4 weeks of enrolment; (IV) history of lung surgery; (V) diagnosis of cancer; (VI) recipient of a blood transfusion within 4 weeks of enrollment; (VII) inability to walk, and (VIII) current participation in a blinded drug trial. In the current study, we did not exclude chronic comorbidities such as hypertension, diabetes, coronary heart disease, hypercholesterolemia, and benign prostatic hyperplasia for participants of all groups.
Informed consent was obtained from all subjects. The study was conducted in accordance with the Declaration of Helsinki (as revised in 2013) and approved by the Ethics Committee of the First Affiliated Hospital of Guangzhou Medical University (approval No. 2017-22). Clinical trial registered with www.clinicaltrials.gov (NCT 03240315).
Clinical and functional parameters
Data collected at enrollment included patient demographics, pulmonary function, COPD assessment test (CAT), and the Modified Medical Research Council Dyspnea Scale (mMRC) score before sputum induction. Venous blood samples were obtained from all subjects. Spirometry was performed before sputum induction according to the American Thoracic Society (ATS) guidelines (24).
Blood sample collection and processing
Venous blood samples were collected in ethylenediaminetetraacetic acid anticoagulation tubes before sputum induction. A differential white blood cell count was carried out using a Coulter instrument (Sysmex-XE2100, Kobe, Japan). Serum samples were stored at –80 °C for subsequent detection.
Sputum induction and processing
Sputum induction was performed in accordance with the guidelines of the Task Force of the European Respiratory Society (25). A two-step procedure was used to process sputum to avoid the effect of dithiothreitol on subsequent protein analysis. Details of the protocol were reported previously (26). The sputum supernatant and serum samples were stored at –80 °C for further analysis. Pulmonary function test, venous blood sample collection, and sputum induction were performed from 8:30 a.m.–10:00 a.m.
Autoantibody detection
Given that the autoantibody detection in sputum is not performed routinely, we validated the reproducibility of the autoantibody measurements in sputum supernatant from healthy subjects and stable COPD subjects in our recent publication (27). Based on a search of the literature, we selected 10 autoantigens with known or putative links to COPD (19,28-30): Ro/Sjögren syndrome type A antigen (Ro/SSA), La/Sjögren syndrome type B antigen (La/SSB), Smith antigen (Sm), DNA topoisomerase I (Scl-70), ribosomal phosphoprotein P0 (P0), U1 small nuclear ribonucleoprotein (U1-SnRNP), histidyl-tRNA synthetase (Jo-1), thyroid peroxidase (TPO), and myeloperoxidase (MPO), proteinase-3 (PR-3).
The levels of autoantibodies were detected using the Luminex platform, as we described before (31). The selected autoantigens (US Biological, US) were coupled to multiplex magnetic beads (Luminex, US) and incubated with sputum supernatant and serum samples diluted 1:10 and 1:180 at 37 °C for 1 h. The beads were washed using a Bio-Plex ProTM wash station (Bio-Rad, USA), treated with biotin-conjugated anti-human IgG (ThermoFisher, US) at a 1:1,000 dilution and incubated at 37 °C for 1 h. Thereafter, beads were washed and reacted for 15 min at 37 °C with streptavidin-R-phycoerythrin (Bio-Rad, US). After the microspheres were washed and resuspended, the mean fluorescence intensity (MFI) of each encoded microsphere was measured using Bio-Plex 200 (Bio-Rad, US). Bio-Plex ManagerTM 6.0 software was used to generate the result files. All resulting MFI were corrected for their background by subtracting the MFI of the blank control (beads incubated without sputum/serum).
Statistical analysis
Data are here expressed as the mean ± standard deviation (SD) for continuous variables. Variables with skewed distribution were expressed as the median [interquartile range (P25–P75)]. For categorical variables, the number of observations and percentages in each category are reported. All statistical analyses were performed using SPSS software (version 19.0). Unbiased/unsupervised agglomerative (“bottoms-up”) hierarchical clustering was performed on the Spearman’s correlation matrix by using the uncentered correlation as the similarity metric (Cluster 3.0, available at http://bonsai.hgc.jp/~mdehoon/software/cluster/software.htm) to identify the autoantibody modules. The dendrogram and resulting heatmap were visualized using TreeView (version 1.1.6r4, available at http://jtreeview.sourceforge.net/) based on Java (available at https://www.java.com/zh_CN/). An autoantibody network was then established using Gephi software (version 0.9.1, available at http://www.gephi.org/). Network clustering was conducted using the “fast unfolding” algorithm included in the Gephi software.
Results
Clinical and molecular characteristics of the patients
The demographics and clinical characteristics of the four study groups are shown in Table 1. The sputum and serum autoantibodies levels of the four groups are shown in Table 2, and their post-hoc comparisons are shown in Figure 1. In COPD groups, sputum anti-Ro/SSA and serum anti-U1-SnRNP were significantly higher than in the control group.
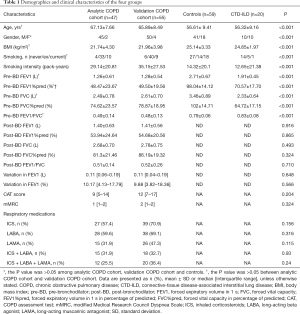
Full table
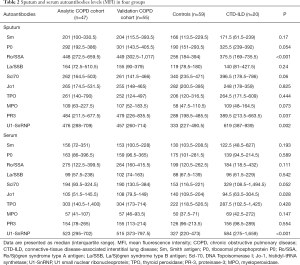
Full table
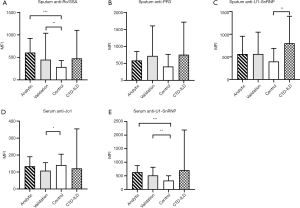
Relationship between autoantibody profiles and clinical parameters in COPD
Of all detected autoantibodies, with adjustment for age, FEV1 in percentage of predicted (FEV1%pred) correlated negatively with the sputum anti-PR3 level, but there was no significant correlation with serum autoantibody levels. The CAT score was positively correlated with sputum anti-P0, anti-Ro/SSA, anti-MPO, and anti-U1-SnRNP levels but weakly and negatively correlated with the serum anti-TPO level. The number of exacerbation events in the previous year was found to be positively correlated with the sputum anti-P0, anti-Ro/SSA, anti-PR3, and anti-U1-SnRNP levels but negatively correlated with the serum anti-TPO level (Tables 3,4).
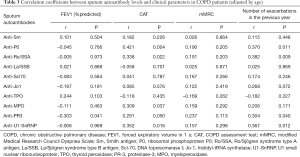
Full table
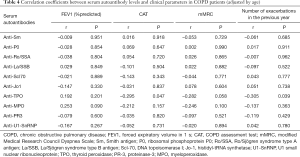
Full table
Identification of autoantibody clusters by using hierarchical clustering
To identify the autoantibody clusters, hierarchical clustering was performed on Spearman’s correlation matrix using the uncentered correlation as the similarity metric. In COPD cohorts, hierarchical clustering of the correlation matrix showed that sputum and serum autoantibodies could be distinguished perfectly in both the analysis cohort and validation cohort (Figure 2A,B).
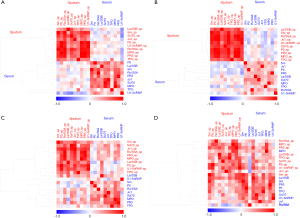
In those controls and CTD-ILD patients, hierarchical clustering could not separate the autoantibodies into airway vs. circulating compartments (Figure 2C,D), suggesting an association between sputum and serum autoantibody responses.
Identification of autoantibody modules by using network analysis
A second approach to the identification of the autoantibody clusters (modules in the network) involved clustering applied to the autoantibody network, which was here used to identify two modules (Figure 3A). Module 1 (yellow) was composed of 11 nodes that included all of the sputum autoantibodies and one serum autoantibody (anti-TPO). Module 2 (green) was composed of nine nodes comprising all serum autoantibodies. In this way, only one autoantibody (serum anti-TPO) was misclassified from one module (serum) to the other (sputum), showing that network clustering can adequately distinguish sputum from serum autoantibodies.
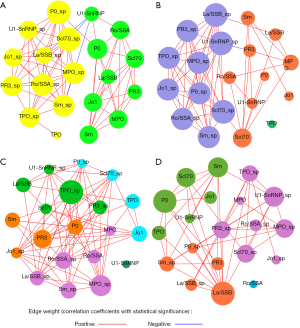
In the COPD cohort used for validation, network analysis perfectly separated the autoantibodies into two compartments (airway and circulating) (Figure 3B). In the control group, network clustering identified four autoantibody modules, but it did not separate the autoantibodies into two compartments (Figure 3C). In CTD-ILD patients, network clustering separated the autoantibodies into the three modules, but it did not separate the autoantibodies into two compartments (Figure 3D).
Discussion
This is the first study to use a sensitive detection method to simultaneously investigate autoantibody levels in two compartments (airway and circulation) in COPD patients. In addition, two unbiased approaches were used to identify the autoantibody modules. The main finding of our study was the dissociation between airway and circulating autoantibody levels in patients with stable COPD. We then validated this result using a second COPD cohort and compared the results to those from healthy controls and CTD-ILD patients. We also found that sputum but not serum autoantibody levels to be positively associated with the risk of disease exacerbation.
In the current study, the selected autoantibodies used were against three types of autoantigens (including 10 self-antigens) and were chosen for exploratory purposes based on a search of the literature: (I) ubiquitous self-antigens: Sm, P0, Ro/SSA, La/SSB, Scl-70, Jo-1, and U1-SnRNP. These self-antigens were present in all nucleated cells. Noxious particles or gases stimulate an inflammatory response in the lung that damages the lung tissue. The consequent cell damage releases nucleoprotein complexes and exposes the self-antigens to the immune system, thus triggering autoimmunity (28). In addition, oxidative stress may lead to chemical modification of the above proteins, resulting in the generation of neo-antigens and the induction of an autoantibody response. (II) The organ-specific antigen TPO, which may not be involved in the pathogenesis of COPD. TPO served as an exploratory antigen in our study. Packard et al. reported that serum anti-TPO levels were lower in patients with emphysematous than non-emphysematous COPD (19). (III) The cytoplasmic neutrophil antigens MPO and PR3. There is abundant evidence that neutrophil degranulation releases a range of bioactive substances that contribute significantly to the tissue damage in COPD (29). Under the effect of neutrophil extracellular traps (30), neutrophil degranulation products such as MPO and PR3 may also serve as self-antigens, triggering autoimmunity and tissue damage in the airways.
Previous studies evaluated the relationships between inflammatory markers in the airway and circulation in COPD patients by simultaneously monitoring the same mediators in the two compartments (5-7,32). These studies showed a lack of correlation between mediators in the two compartments. However, they did not examine the relationship between airway and circulating autoantibodies. We found a correlation between the presence of the same autoantibodies in the airway and circulation of patients with stable COPD and used an unbiased approach to discriminate the autoantibodies in one compartment vs. the other.
While it is widely accepted that systemic inflammation contributes to the systemic manifestations of COPD, little is known about the relationship between local and systemic autoimmune responses. Therefore, in the current study, we used two unbiased approaches, hierarchical clustering based on the uncentered correlation similarity metric and network clustering based on a “fast unfolding” algorithm, to identify the autoantibody modules in sputum and serum and the possible relationship among them. Hierarchical clustering discriminated between serum and sputum autoantibodies, although network clustering was also effective. The results showed that, in patients with stable COPD, the autoantibody profiles in these two biological compartments are significantly different.
We also found that while the presence of many sputum autoantibodies is correlated with clinical parameters, in serum, this was the case only for anti-TPO. These results suggested that sputum autoantibodies are more clinically relevant than serum autoantibodies. Airflow limitation (FEV1%pred) was found to be negatively correlated with sputum anti-PR3 level, whereas the CAT score was positively correlated with sputum anti-P0, sputum anti-Ro/SSA, sputum anti-MPO, and sputum anti-U1-SnRNP levels, but negatively correlated with serum anti-TPO. Importantly, the risk of exacerbation of COPD (number of exacerbation events in the previous year) was found to be positively correlated with sputum anti-P0, sputum anti-Ro/SSA, anti-PR3 levels, and sputum anti-U1-SnRNP. Whereas the serum anti-ENA autoantibody profiles did not correlate with clinical parameters, in agreement with a previous study showing that serum ANA titers were not related to lung function (20). The positive correlation between serum anti-TPO levels and FEV1%pred was consistent with the results reported by Packard et al., who showed that serum anti-TPO levels were lower in patients with emphysematous than non-emphysematous COPD (19).
The current study has several main strengths. First, we detected autoantibody profiles in two compartments (airway and circulation) simultaneously and evaluated the relationships between serum and sputum autoantibodies by using two unbiased approaches: (I) hierarchical clustering; (II) network-based clustering, a novel approach recommended by many researchers (33-36). Second, we added a second cohort with a larger sample size to validate the relationships between sputum and serum autoantibodies in COPD, and we also compared the results to tests performed under other conditions i.e., non-COPD controls and CTD-ILD patients. Third, we evaluated this relationship using clinical parameters.
Our study also has several limitations. First, as an observational study, conclusions regarding the causal relationship between sputum autoantibody levels and the risk of COPD exacerbation could not be drawn. Second, the autoantibodies in the current study are not specific to COPD, which could be a limiting factor in identification of pathological autoantibodies involved in the mechanism underlying COPD. Third, we detected the IgM autoantibodies against the selected autoantigens, but most values were around the lower detection limits and similar to those of healthy controls, so we do not think the data are reliable enough and did not show them here. Fourth, most COPD patients were male. This may be because of the uneven sex distribution of the most important COPD risk factor: According to the Global Adults Tobacco Survey of 2018, 50.5% of males but only 2.1% of females in China were smokers (37). Because many more males than females smoke, there was a corresponding sexual bias among our study participants. Fifth, due to different risk factors and prognoses between groups, we could not match all the characteristics, such as gender, age, smoking status, and chronic comorbidities, between COPD groups and the other groups. However, the goal of the current study was to elucidate the relationship between airway and systemic autoantibody responses in COPD patients. In this way, this limitation may not affect the main conclusions of our study.
In conclusion, we observed a dissociation between sputum autoantibodies and serum autoantibodies in the context of their correlation patterns in patients with stable COPD, suggesting different immunopathogenic mechanisms. Sputum autoantibodies are more clinically relevant than serum autoantibodies. Therefore, focusing on airway autoimmunity may help improve understanding of the immunopathological mechanism of COPD.
Acknowledgments
We thank LetPub (www.letpub.com) for its linguistic assistance during the preparation of this manuscript.
Funding: This study was supported by National Key R&D Program of China (2017YFC1310600, 2018YFC1311900, 2016YFC0903700); the Guangzhou Healthcare Collaborative Innovation Major Project (201604020012); Medical Scientific Research Foundation of Guangdong Province (C2017050); and Project 111 (D18010).
Footnote
Reporting Checklist: The authors have completed the STROBE reporting checklist. Available at http://dx.doi.org/10.21037/atm-20-944
Data Sharing Statement: Available at http://dx.doi.org/10.21037/atm-20-944
Peer Review File: Available at http://dx.doi.org/10.21037/atm-20-944
Conflicts of Interest: All authors have completed the ICMJE uniform disclosure form (available at http://dx.doi.org/10.21037/atm-20-944). All authors report grants from National Key R&D Program of China (2017YFC1310600, 2018YFC1311900, 2016YFC0903700), grants from Guangzhou Healthcare Collaborative Innovation Major Project (201604020012), grants from Medical Scientific Research Foundation of Guangdong Province (C2017050), grants from Project 111 (D18010), during the conduct of the study.
Ethical Statement: The authors are accountable for all aspects of the work in ensuring that questions related to the accuracy or integrity of any part of the work are appropriately investigated and resolved. The study was conducted in accordance with the Declaration of Helsinki (as revised in 2013) and approved by the Ethics Committee of the First Affiliated Hospital of Guangzhou Medical University (approval No. 2017-22). Clinical trial registered with www.clinicaltrials.gov (NCT 03240315). Informed consent was taken from all the patients.
Open Access Statement: This is an Open Access article distributed in accordance with the Creative Commons Attribution-NonCommercial-NoDerivs 4.0 International License (CC BY-NC-ND 4.0), which permits the non-commercial replication and distribution of the article with the strict proviso that no changes or edits are made and the original work is properly cited (including links to both the formal publication through the relevant DOI and the license). See: https://creativecommons.org/licenses/by-nc-nd/4.0/.
References
- World Health Organization. Projections of mortality and causes of death, 2016 to 2060. Available online: (accessed 14 October, 2019).http://www.who.int/healthinfo/global_burden_disease/projections/en/
- 2020 Global strategy for prevention, diagnosis and management of COPD. Available online: https://goldcopd.org/gold-reports/
- Agustí A, MacNee W, Donaldson K, et al. Hypothesis: does COPD have an autoimmune component? Thorax 2003;58:832-4. [Crossref] [PubMed]
- Barnes PJ. Inflammatory mechanisms in patients with chronic obstructive pulmonary disease. J Allergy Clin Immunol 2016;138:16-27. [Crossref] [PubMed]
- Núñez B, Sauleda J, Garcia-Aymerich J, et al. Lack of correlation between pulmonary and systemic inflammation markers in patients with chronic obstructive pulmonary disease: a simultaneous, two-compartmental analysis. Arch Bronconeumol 2016;52:361-7. [Crossref] [PubMed]
- Donaldson GC, Belchamber KBR, Singh R, et al. Dissociation between airway and systemic inflammatory changes at exacerbation in COPD. Am J Respir Crit Care Med 2016;193:A1020.
- Vernooy JH, Kucukaycan M, Jacobs JA, et al. Local and systemic inflammation in patients with chronic obstructive pulmonary disease: soluble tumor necrosis factor receptors are increased in sputum. Am J Respir Crit Care Med 2002;166:1218-24. [Crossref] [PubMed]
- Barnes PJ, Celli BR. Systemic manifestations and comorbidities of COPD. Eur Respir J 2009;33:1165-85. [Crossref] [PubMed]
- Decramer M, Janssens W. Chronic obstructive pulmonary disease and comorbidities. Lancet Respir Med 2013;1:73-83. [Crossref] [PubMed]
- Caramori G, Ruggeri P, Di Stefano A, et al. Autoimmunity and COPD: clinical implications. Chest 2018;153:1424-31. [Crossref] [PubMed]
- Brusselle GG, Joos GF, Bracke KR. New insights into the immunology of chronic obstructive pulmonary disease. Lancet 2011;378:1015-26. [Crossref] [PubMed]
- Cosio MG, Saetta M, Agusti A. Immunologic aspects of chronic obstructive pulmonary disease. N Engl J Med 2009;360:2445-54. [Crossref] [PubMed]
- Ji X, Niu X, Qian J, et al. A phenome-wide association study uncovers a role for autoimmunity in the development of chronic obstructive pulmonary disease. Am J Respir Cell Mol Biol 2018;58:777-9. [Crossref] [PubMed]
- Domagała-Kulawik J, Hoser G, Dąbrowska M, et al. CD4+/CD25+ cells in systemic inflammation in COPD. Scand J Immunol 2011;73:59-65. [Crossref] [PubMed]
- Zhu X, Gadgil AS, Givelber R, et al. Peripheral T cell functions correlate with the severity of chronic obstructive pulmonary disease. J Immunol 2009;182:3270-7. [Crossref] [PubMed]
- Bon J, Kahloon R, Zhang Y, et al. Autoreactivity to glucose regulated protein 78 links emphysema and osteoporosis in smokers. PLoS One 2014;9:e105066. [Crossref] [PubMed]
- Rinaldi M, Lehouck A, Heulens N, et al. Antielastin B-cell and T-cell immunity in patients with chronic obstructive pulmonary disease. Thorax 2012;67:694-700. [Crossref] [PubMed]
- Lee SH, Goswami S, Grudo A, et al. Antielastin autoimmunity in tobacco smoking-induced emphysema. Nat Med 2007;13:567-9. [Crossref] [PubMed]
- Packard TA, Li QZ, Cosgrove GP, et al. COPD is associated with production of autoantibodies to a broad spectrum of self-antigens, correlative with disease phenotype. Immunol Res 2013;55:48-57. [Crossref] [PubMed]
- Núñez B, Sauleda J, Anto JM, et al. Anti-tissue antibodies are related to lung function in chronic obstructive pulmonary disease. Am J Respir Crit Care Med 2011;183:1025-31. [Crossref] [PubMed]
- Wen L, Krauss-Etschmann S, Petersen F, et al. Autoantibodies in chronic obstructive pulmonary disease. Front Immunol 2018;9:66. [Crossref] [PubMed]
- Ma A, Wen L, Yin J, et al. Serum levels of autoantibodies against extracellular antigens and neutrophil granule proteins increase in patients with COPD compared to Non-COPD smokers. Int J Chron Obstruct Pulmon Dis 2020;15:189-200. [Crossref] [PubMed]
- Byrne R, Todd I, Tighe PJ, et al. Autoantibodies in chronic obstructive pulmonary disease: a systematic review. Immunol Lett 2019;214:8-15. [Crossref] [PubMed]
- Miller MR, Hankinson J, Brusasco V, et al. Standardisation of spirometry. Eur Respir J 2005;26:319-38. [Crossref] [PubMed]
- Djukanović R, Sterk PJ, Fahy JV, et al. Standardised methodology of sputum induction and processing. Eur Respir J Suppl 2002;37:1s-2s. [Crossref] [PubMed]
- Bafadhel M, McCormick M, Saha S, et al. Profiling of sputum inflammatory mediators in asthma and chronic obstructive pulmonary disease. Respiration 2012;83:36-44. [Crossref] [PubMed]
- Wang F, Liang Z, Yang Y, et al. Reproducibility of fluid-phase measurements in PBS-treated sputum supernatant of healthy and stable COPD subjects. Int J Chron Obstruct Pulmon Dis 2019;14:835-52. [Crossref] [PubMed]
- Mackay IR, Leskovsek NV, Rose NR. Cell damage and autoimmunity: a critical appraisal. J Autoimmun 2008;30:5-11. [Crossref] [PubMed]
- Hoenderdos K, Condliffe A. The neutrophil in chronic obstructive pulmonary disease. Am J Respir Cell Mol Biol 2013;48:531-9. [Crossref] [PubMed]
- Sangaletti S, Tripodo C, Chiodoni C, et al. Neutrophil extracellular traps mediate transfer of cytoplasmic neutrophil antigens to myeloid dendritic cells toward ANCA induction and associated autoimmunity. Blood 2012;120:3007-18. [Crossref] [PubMed]
- Qin R, Long F, Xiao X, et al. Sputum autoantibodies are more relevant in autoimmune responses in asthma than are serum autoantibodies. Allergy Asthma Immunol Res 2019;11:406-21. [Crossref] [PubMed]
- Hurst JR, Perera WR, Wilkinson TM, et al. Systemic and upper and lower airway inflammation at exacerbation of chronic obstructive pulmonary disease. Am J Respir Crit Care Med 2006;173:71-8. [Crossref] [PubMed]
- Barabási AL, Gulbahce N, Loscalzo J. Network medicine: a network-based approach to human disease. Nat Rev Genet 2011;12:56-68. [Crossref] [PubMed]
- Faner R, Agusti A. Network analysis: a way forward for understanding COPD multimorbidity. Eur Respir J 2015;46:591-2. [Crossref] [PubMed]
- Faner R, Cruz T, Lopez-Giraldo A, et al. Network medicine, multimorbidity and the lung in the elderly. Eur Respir J 2014;44:775-88. [Crossref] [PubMed]
- Diez D, Agusti A, Wheelock CE. Network analysis in the investigation of chronic respiratory diseases. From basics to application. Am J Respir Crit Care Med 2014;190:981-8. [Crossref] [PubMed]
- World Health Organization. Global Adult Tobacco Survey (GATS) (China 2018). 2019.