Identification of hub genes in diabetic kidney disease via multiple-microarray analysis
Introduction
Diabetic kidney disease (DKD) is considered to be one of the most common microvascular complications and affects approximately 30% of the global population. It is also the main cause of end-stage renal disease in many populations and is associated with high mortality and increased medical care costs (1-3). The key pathogenesis of DKD is renal fibrosis, which was induced at least by renal hemodynamic changes, inflammatory processes and overactive renin-angiotensin-aldosterone system (RAAS), ischemia and over-reactive oxidative stress (4). Recent molecular and cellular researches explored new fields of pathophysiology of DKD, such as mitochondria dysfunction (5), podocyte autophagy (6), and genetic and epigenetic regulation (7). The interventional managements for DKD included intensive control of blood glucose, blood pressure and lipid, and smoking cessation, which could significantly improve the prognosis of cardiovascular events and help to slow down the progression of micro-albuminuria to macro-albuminuria in DKD (8). Besides, more and more novel treatment based on molecular changes have been developed such as protein kinase C inhibitor (9), endothelium A receptor antagonists (10), vitamin D analogs (11), JAK inhibitor (12), non-steroidal mineralocorticoid antagonist (13) and so on. Thus, tracking the biological changes in DKD at the genomic level should be a valuable strategy both for pathogenesis and treatment of DKD.
In recent years, gene sequencing technology combined with intensive bioinformatic analysis has been conducted to identify multiple disease-related genes, which might be considered therapeutic targets in the future. Within an extremely short time, bioinformatic analysis could process large amounts of samples and provide useful information about diseases, and several genes closely associated with DKD have been found in previous years and driven research innovations. For example, Tang et al. analyzed gene expression profiles in a microarray including 22 microdissected human renal glomerular and 22 tubule samples from healthy patients and patients with DKD, and identified 10 novel potential therapeutic targets for DKD, including ETS proto-oncogene 1, transcription factor, lipopolysaccha-ride induced TNF factor, nuclear factor, erythroid-derived 2, retinoic acid receptor, γ and signal transducer, and activator of transcription 5A (14). Cui et al. reported three potential microRNA (miRNA) biomarkers including miR-17-5p, miR-20a, and miR-106a, with the predicted targets of NR4A3, PTPRO, and KLF9 being involved in the pathogenesis of DKD (15). Furthermore, Kiyanpour et al. re-analyzed two mRNA microarray datasets related to glomerular and tubulointerstitial compartments of human diabetic kidneys, and found two novel miRNAs, miR-208a-3p and miR-496a-3p, that were overexpressed in the cortex of diabetic kidneys (16). Also, Yang et al. found BMP7, CD55, CSF1R, INHBC, and F5 playing crucial roles in the pathogenesis of DKD (17). Additionally, Tang et al. identified Nertin G1 and hepatocyte growth factor as reliable biomarkers for DKD (18). However, the results of one or two microarray analyses seemed to be disputable due to the false-positive rates, and thus more integrated analysis of different kinds of DKD datasets should be considered and unearthed from different perspectives.
Single-cell RNA sequencing (scRNA-seq) is a well-established and powerful method for investigating transcriptomic variation, and can be used to provide insights into physiological and pathological processes in various cell types. In addition, after assigning cell types, probable interactions between each cell type based on gene expression profiles can be quantified, which aids in understanding the interactions between different cellular components (CCs). To provide novel insight into the pathogenesis and therapeutic biomarkers of DKD, we re-analyzed scRNA microarray data, and integrated the information with two other microarray datasets downloaded from the Gene Expression Omnibus (GEO) and as far as we know this was a first bio-informative analysis integrated with scRNA-seq datasets in DKD.
We present the following article in accordance with the MDAR reporting checklist (available at http://dx.doi.org/10.21037/atm-20-5171).
Methods
Microarray data
Microarray data were downloaded from the GEO database (http://www.ncbi.nlm.nih.gov/geo). The GSE131882 dataset included data on kidney samples from patients with DKD (n=3) and healthy controls (n=3) on the GPL24676 (Illumina NovaSeq 6000) platform. The GSE30122 dataset included data on kidney samples from patients with DKD (n=19) and healthy controls (n=50) on the GPL571 (Affymetrix Human Genome U133A 2.0 Array) platform. The GSE30529 dataset included data on kidney samples from patients with DKD (n=41) and controls (n=20) based on the GPL17586 (Affymetrix Human Transcriptome Array 2.0) platform.
Microarray data processing
The R software package was applied to process the microarray data and to normalize the unqualified files. For the dataset in GSE131882, data preprocessing included conversion from probes into gene symbols, data quality control, batch normalization, principal component (PC) analysis, and cluster analysis. DropletUtils package (19) in R software was used to detect the expression of each cell, and the scater package (20) was subsequently used to count the expression of genes in cells. Cells were filtered according to the proportion of mitochondrial genes (≤5%) and ribosomal genes (≥10%). Seurat package (21) in R software was further utilized to standardize the expression of filtered samples and find the top 2,000 genes with the most obvious difference between cells. ScaleData in the Seurat package was used to scale the expression data linearly, while the RunPCA in Seurat package was used for PC analysis. The PCs with larger standard deviation (cumulative standard deviation higher than 70%) were selected, and the FindNeighbors and FindClusters in the Seurat package were used for cell cluster analysis. The cells were labeled and clustered according to the existing notes utilizing CellMarker (22) in the Seurat package.
For the datasets in GSE30122 and GSE30529, raw data in the format of CEL was obtained, and the Affy package (23) in R software was used to perform background correction and normalization.
Identification of differentially expressed genes (DEGs)
DEGs from the datasets in GSE30122 and GSE30529 were identified using the limma package in R software (24). DEGs from the dataset in GSE131882 were identified by the FindMarkers in the Seurat package. Samples with an absolute value of log fold change greater than 1.5 and a P value less than 0.05 were considered DEGs. Probe sets without corresponding gene symbols or genes with more than 1 probe set were removed or averaged, respectively. If some genes were upregulated in one data set and downregulated in another data set, they were removed in the subsequent analysis.
Enrichment analysis
All identified DEGs in GSE30122 and GSE30529 were used for subsequent analysis as the inadequate numbers of DEGs in the intersection of the two data sets. Based on the database of gene ontology (GO) (25) and Kyoto Encyclopedia of Genes and Genomes (KEGG) pathway database (26) via DAVID online tools, the DEGs were analyzed by functional enrichment analysis. GO analysis consists of biological processes (BP), cellular component (CC), and molecular function (MF) analyses. The Fisher’s exact test was used to find out which specific functional items were most related to a group of DEGs. Each item in the analysis results corresponded to a statistical value p-value to express the significance, and FDR was calculated.
Protein-protein interaction (PPI) network creation and hub gene identification
To establish a PPI network of DEGs, Search Tool for the Retrieval of Interacting Genes/Proteins (STRING) (27) was used to retrieve interacting genes. Interaction with a combined score >0.7 was set as the cutoff point. The PPI network was drawn by Cytoscape software (28). A node was defined as the protein product of a DEG in the network, and it was required that all nodes in the network were DEGs. Significant modules and hub genes in the PPI network were identified by molecular complex detection (MCODE) (29). The parameters of DEG clustering and scoring were set as follows: MCODE score ≥3, degree cutoff =2, node score cutoff =0.2, max depth =100, and k-score =2.
Clinicopathological correlation analysis
The Pearson’s correlation analysis between hub genes and glomerular filtration rate (GFR) (30,31) and urine albumin to creatinine ratio (ACR) (30) in patients with DKD were performed using Nephroseq v5 online database. The statistical analyses were carried out using GraphPad prism 7.0 (GraphPad Software Inc. La Jolla, CA, USA).
Ethical statement
All data were obtained from an open-access database, and not directly from patients or animals directly. Thus, acquiring ethical approval was not necessary.
Results
Identification of DEGs
For the GSE131882 dataset which was a scRNA-seq microarray, we firstly performed a series of filtrations and standardizations and selected the top 2,000 genes with high intercellular differences. The top 10 genes with the largest standard deviation are shown in Figure S1. We then used a PC analysis to select the PC with the larger standard deviation for subsequent clustering analysis. In both healthy and DKD kidney samples, 10 clusters were identified including nephron epithelial cells, epithelial cells, B cells, regulatory T (Treg) cells, mast cells, T helper 9 (Th9) cells, plasmacytoid dendritic cells, mesangial cells, neutrophils, and endothelial cells (Figure 1A). We selected the genes based on the classification of cell groups, and the top 10 marker gene expressions were listed as a cluster heap map (Figure 1B). The DEGs were then selected accordingly in each cell clusters (Figure 1C).
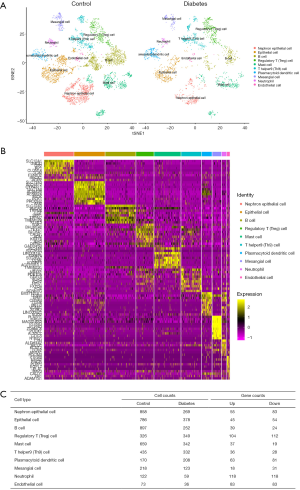
For the microarray datasets GSE30122 and GSE96804, we used genes with significant differences in mean (P value ≤0.05) to do PC analysis (Figure S2) and subsequently created the correlation heat map (Figure 2A,B). After standardization of the microarray results, 472 DEGs were identified from the GSE30122 dataset, including 286 upregulated genes and 186 downregulated genes (Figure 2C). Similarly, 1,851 DEGs were screened from the GSE96804 dataset, including 988 upregulated genes and 863 downregulated genes (Figure 2D). The cluster heatmaps of the DEGs are shown in Figure 2E,F.
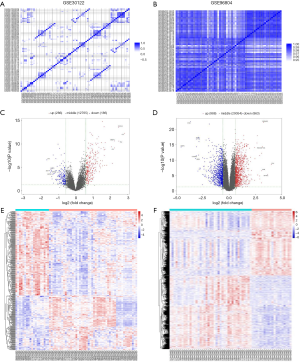
Functional enrichment analysis of DEGs
All identified DEGs in the GSE30122 and GSE96804 datasets were used for subsequent analysis as the limited numbers of DEGs in the intersection of the two datasets. We then used an intersection between DEGs in the scRNA dataset and the union DEGs in GSE30528 and GSE30529 for further analysis. A total of 84 upregulated (Figure 3A, Table S1) and 49 downregulated (Figure 3B, Table S2) DEGs were identified. On the basis of the GO biological process, the top 10 most significantly enriched GO terms are presented. The upregulated genes in GO terms were primarily associated with multicellular organism development, neurogenesis, nervous system development, generation of neurons, and epithelial cell differentiation (Figure 3C). The downregulated genes in GO terms were primarily associated with negative regulation of cell population proliferation, kidney development, chloride ion homeostasis, metanephric nephron tubule development, and renal system development (Figure 3D). The top 10 most significantly enriched GO terms in MF and CC analysis are shown in Figure S3.
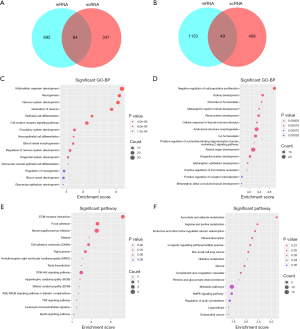
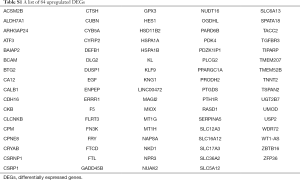
Full table
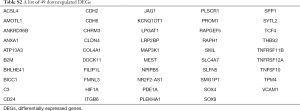
Full table
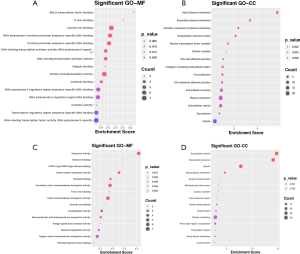
To explore enriched pathways of DEGs, KEGG pathway analysis was done using the Database for Annotation, Visualization and Integrated Discovery (DAVID) online tools. Results of this analysis revealed that upregulated DEGs were mainly enriched in extracellular matrix (ECM) receptor interaction, focal adhesion, human papillomavirus infection, malaria, and cell adhesion molecules (Figure 3E). Meanwhile, the downregulated DEGs were mainly enriched in ascorbate and aldarate metabolism, arginine and proline metabolism, endocrine– and other factor–regulated calcium reabsorption, mineral absorption, and longevity regulating pathway multiple species (Figure 3F).
PPI network analysis and hub gene recognition
To identify most significant clusters of DEGs, a PPI network of DEGs was constituted by STRING and visualized by Cytoscape (Figure 4A). The three most significant modules were recognized by the MCODE plug-in of Cytoscape. Among these modules, a total of 17 hub genes were identified including DUSP1, GADD45B, ATF3, and BTG2 (Figure 4B); EGF, B2M, CDH2, CLDN4, and SPP1 (Figure 4C); CD24, JAG1, PROM1, VCAM1, F5, C3, SOX9, and KNG1(Figure 4D). Furthermore, to better understand the hub genes’ role in cell-cell interaction and regulation, we listed the distributions of hub genes in each cell clusters using the data from scRNA microarray (Table 1).
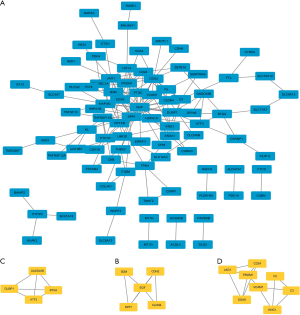
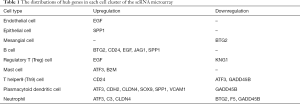
Full table
The association between hub genes and clinical features of DKD
To verify the potential roles of hub genes in DKD, correlation analysis and subgroup analysis between hub genes and clinical features were conducted using the Nephroseq v5 online tool. First, the results showed that mRNA expression of EGF, KNG1, GADD45B, and CDH2 positively correlated with in DKD patients (Figure 5), suggesting that these genes may have reno-protective roles in DKD. Meanwhile, the mRNA expression of ATF3, B2M, VCAM1, CLDN4, SPP1, SOX9, JAG1, C3, and CD24 negatively correlated with GFR in DKD patients (Figure 6), indicating that these hub genes might promote the progression of DKD. Moreover, the mRNA expression of ATF3 and BTG2 positively correlated with ACR, also suggesting that these two genes might contribute to the progression of DKD (Figure 7).
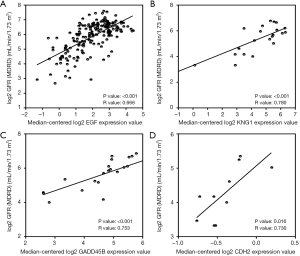
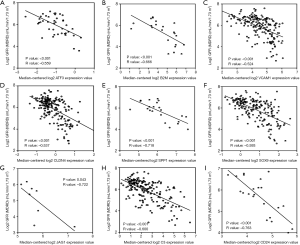
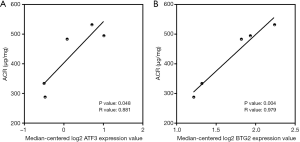
Discussion
DKD is the result of multiple gene interactions; thus, by using bioinformatics analysis, an extensive application of gene expression can offer the possibility to study the pathogenesis of DKD. In our study, a set of 2,178 DEGs from the GSE30122 and GSE30529 datasets were identified, including 1,202 upregulated genes and 976 downregulated genes. Among them, the expression of 133 DEGs (84 upregulated genes and 39 downregulated genes) were validated by an ScRNA-seq dataset GSE131882. According to the functional enrichment analysis, we found a set of upregulated DEGs were most significantly enriched in ECM receptor interaction including COL4A1, ITGB6, SPP1, and THBS2. In fact, the structural features of DKD was characterized by increased amounts of ECM which may be pathologically essential as it could lead to glomerulosclerosis and tubulointerstitial fibrosis accompanied by subsequent nephron loss. Thus, it was not surprising that ECM receptor interaction pathway was one of the most active pathways in the KEGG analysis. Furthermore, a series of downregulated DEGs were discovered to be most significantly enriched in the pathway of ascorbate and aldarate metabolism, and included ALDH7A1, MIOX, and UGT2B7. In a previous report, genes in ascorbate and aldarate metabolism had been found to be involved in the pathogenesis of DKD by a genome-wide association analysis (32). Also, Wang et al. found that ascorbate-aldarate metabolism played key roles in the development of diabetic retinopathy, which was another important predictor of DKD (33).
In the 133 DEGs, we further selected 17 DEGs as hub genes. Among these hub genes, DUSP1, GADD45B, and BTG2 showed the highest MCODE score of 4; EGF, B2M, CDH2, CLDN4, and SPP1 showed an MCODE score of 3.5; CD24, JAG1, PROM1, VCAM1, F5, C3, SOX9, and KNG1 showed an MCODE score of 3.429. There have been many studies about the relationships between the identified hub genes and DKD, such as EGF (34,35), F5 (36,37), and KNG1 (38,39). Some genes such as DUSP1 and B2M have been found dysregulated under hyperglycemia associated with changed renal function, although the inner mechanism still remained unknown. The DUSP1 encoded a threonine-tyrosine dual-specificity phosphatase which dephosphorylated and inactivated extracellular signal-regulated kinase, p38, and c-Jun N-terminal kinase in a context-dependent manner. Sheng et al. reported that DUSP1 was downregulated by chronic hyperglycemia stimulus, while overexpression of DUSP1 interrupted mitochondrial fission, reducing hyperglycemia-mediated mitochondrial damage and subsequently improving renal function (40). B2M was found to encode the β-chain of the major histocompatibility complex (MHC) class I molecules and to be upregulated in inflammatory and tumor cells. Monteiro et al. reported that mRNA expression of B2M in cells of the urinary sediment was higher in type 1 diabetic patients with kidney diseases (41).
The pathophysiology of DKD included thickening of the glomerular basement membrane, mesangial expansion, segmental glomerulosclerosis or global glomerulosclerosis, tubulointerstitial fibrosis, and afferent and efferent arteriole hyalinosis (42), in which renal fibrosis is the key process. Our study identified several hub genes that were directly associated with renal fibrosis, including SPP1, VCAM-1, SOX9, and JAG1. SPP1 encodes osteopontin which has been shown to cause glomerular damage (43) and interstitial fibrosis (44) in DKD. Cai et al. also found the epigenetic regulation of SPP1 was also very important in DKD, and, with the targeting of histone markers such as H3K9ac, H3K4me1, H3K4me3, and H3K27me3, might provide an new method to protect kidneys from deleterious effects of glucose (45). VCAM-1, an adhesion molecule which predominantly promotes the adhesion of lymphocytes, monocytes, eosinophils, and basophils, has been reported to be significantly upregulated in the tubulointerstitium of DKD (46). Also, serum levels of VCAM-1 have been found to be significantly elevated in patients with type 2 diabetes and correlated with the extent of albuminuria (47,48). SOX9 is a cartilage-specific transcription factor, which has been found to be mainly involved in the deposition of ECM (49). Kishi et al. reported that SOX9 protein induced a chondrogenic phenotype of mesangial cells and contributed to advancement of DKD (50). Meanwhile, JAG1 was revealed to encode one of five cell surface proteins that interact with four receptors in the Notch signaling pathway. Morrissey et al. reported a TGFβ1-mediated upregulation of JAG1 in kidney tubules utilizing a mouse model of renal fibrosis (51).
Usually, the expression of genes under one constant pathological condition maintains the same regulation pattern. In our study, we found the hub gene ATF3 to be upregulated in mast cells, plasmacytoid dendritic cells, and neutrophils, but downregulated in Th9 cells, a phenomenon which has hitherto not been reported. The ATF3, a member of the ATF/CREB family of transcription factors, which was originally isolated from a serum-induced HeLa cell cDNA library (52), has been implicated in cell cycle progression (53), immune response pathways (54), and endoplasmic reticulum stress response (55). However, because the functions of ATF3 depend on its transcriptional milieu, ATF3 could have the opposite effects on different types of cells (56). In an animal model of DKD, Zhang et al. reported that ATF3 expression was elevated and this overexpression of ATF3 increased podocyte apoptosis and decreased expression of podocin, the cell marker of podocyte; in contrast, ATF3-small interfering RNA knockdown was shown to be reduce podocyte apoptosis and increase podocin expression (57).
Although the majority of the hub genes selected have been reported in DKD, some hub genes, including BTG2, CDH2, GADD45B, and CLDN4, have not been studied in detail. By using the Nephroseq v5 online tool, we found that the mRNA expression of GADD45B of CDH2 was positively correlated with GFR, the mRNA expression of CLDN4 was negatively correlated with GFR, and the mRNA expression of BTG2 was positively correlated with ACR. These findings, taken together, offered new potential targets in future DKD research.
In our study, we also screened gene expression across all cell types present in the DKD microenvironment by using the cluster analysis in single-cell dataset. We identified four major cellular compartments on the basis of canonical marker expression: endothelial cell, immune cell, mesangial cell, and epithelium cell. Within the immune cell clusters, various cell types were found, including Th9 cells, B cells, Treg cells, neutrophils, plasmacytoid dendritic cells, and mast cells. We also found the hub genes we selected were most differently expressed in the immune cell clusters, indicating the importance of immune regulation in DKD. Recently, increasing evidence from clinical and experimental studies has shown that both systemic and local renal inflammation have crucial roles in the development and progression of DKD. Actually, in the glomeruli and interstitium of renal biopsy samples across all stages of DKD, the infiltration of immune cells appears to be common. Moon et al. reported that the number of activated T cells was increased in the kidneys of type 2 diabetic patients, and the number of T cells such as CD4+ and CD20+ cells was correlated with the degree of proteinuria in these patients (58). Lim et al. reported that under diabetic conditions, Rag1−/− mice who lacked mature T and B lymphocytes had milder albuminuria when compared to wild-type controls, suggesting that T or B cells promote the development of albuminuria (59). Similar to previous reports, our study also found the cell marker CD24 expression was upregulated both in B cells and Th9 cells, which provided further evidence of immune cell proliferation in DKD (60,61).
The implications of these findings should be considered alongside some limitations in our study. First, these predictions were not confirmed by experiments, and the number of samples used for analysis was still small. In further studies, more samples will be combined, and the predictions will be verified by experimental in DKD animal models and DKD cohort.
Conclusions
A total of 84 upregulated and 49 downregulated DEGs were identified from 3 microarray datasets, including a scRNA-seq dataset. The upregulated DEGs were most significantly enriched in ECM receptor interaction while the downregulated DEGs were most significantly enriched in the pathway of ascorbate and aldarate metabolism. We further selected 17 hub genes, among which BTG2, CDH2, GADD45B, and CLDN4, have rarely been reported in relation to DKD, indicating the potential new targets for this condition. Moreover, using scRNA-seq, we found most of the hub genes to be dysregulated in immune cells, showing the importance of inflammation in DKD. Altogether, these findings may provide significant insight into the etiology and underlying molecular events of DKD.
Acknowledgments
Funding: This study was supported by the Natural Science Foundation of Jiangsu Province (no. BK20170700) and the National Natural Science Foundation of China (no. 81900773).
Footnote
Reporting Checklist: The authors have completed the MDAR reporting checklist. Available at http://dx.doi.org/10.21037/atm-20-5171
Conflicts of Interest: All authors have completed the ICMJE uniform disclosure form (available at http://dx.doi.org/10.21037/atm-20-5171). The authors have no conflicts of interest to declare.
Ethical Statement: The authors are accountable for all aspects of the work in ensuring that questions related to the accuracy or integrity of any part of the work are appropriately investigated and resolved. All data were obtained from an open-access database, and not directly from patients or animals directly. Thus, acquiring ethical approval was not necessary.
Open Access Statement: This is an Open Access article distributed in accordance with the Creative Commons Attribution-NonCommercial-NoDerivs 4.0 International License (CC BY-NC-ND 4.0), which permits the non-commercial replication and distribution of the article with the strict proviso that no changes or edits are made and the original work is properly cited (including links to both the formal publication through the relevant DOI and the license). See: https://creativecommons.org/licenses/by-nc-nd/4.0/.
References
- Chadban SJ, Briganti EM, Kerr PG, et al. Prevalence of kidney damage in Australian adults: The AusDiab kidney study. J Am Soc Nephrol 2003;14:S131-8. [Crossref] [PubMed]
- Nichols GA, Vupputuri S, Lau H. Medical care costs associated with progression of diabetic nephropathy. Diabetes Care 2011;34:2374-8. [Crossref] [PubMed]
- Reutens AT. Epidemiology of diabetic kidney disease. Med Clin North Am 2013;97:1-18. [Crossref] [PubMed]
- Cahn A, Cernea S, Raz I. The SONAR study—is there a future for endothelin receptor antagonists in diabetic kidney disease? Ann Transl Med 2019;7:S330. [Crossref] [PubMed]
- Gordon JW, Dolinsky VW, Mughal W, et al. Targeting skeletal muscle mitochondria to prevent type 2 diabetes in youth. Biochem Cell Biol 2015;93:452-65. [Crossref] [PubMed]
- Zhu J, Wang KZQ, Chu CT. After the banquet: mitochondrial biogenesis, mitophagy, and cell survival. Autophagy 2013;9:1663-76. [Crossref] [PubMed]
- Bell CG, Teschendorff AE, Rakyan VK, et al. Genome-wide DNA methylation analysis for diabetic nephropathy in type 1 diabetes mellitus. BMC Med Genomics 2010;3:33. [Crossref] [PubMed]
- Thomas MC, Brownlee M, Susztak K, et al. Diabetic kidney disease. Nat Rev Dis Primers 2015;1:15018. [Crossref] [PubMed]
- Tuttle KR, Bakris GL, Toto RD, et al. The effect of ruboxistaurin on nephropathy in type 2 diabetes. Diabetes Care 2005;28:2686-90. [Crossref] [PubMed]
- de Zeeuw D, Coll B, Andress D, et al. The endothelin antagonist atrasentan lowers residual albuminuria in patients with type 2 diabetic nephropathy. J Am Soc Nephrol 2014;25:1083-93. [Crossref] [PubMed]
- de Zeeuw D, Agarwal R, Amdahl M, et al. Selective vitamin D receptor activation with paricalcitol for reduction of albuminuria in patients with type 2 diabetes (VITAL study): a randomised controlled trial. Lancet 2010;376:1543-51. [Crossref] [PubMed]
- Brosius FC, Tuttle KR, Kretzler M. JAK inhibition in the treatment of diabetic kidney disease. Diabetologia 2016;59:1624-7. [Crossref] [PubMed]
- Bakris GL, Agarwal R, Chan JC, et al. Effect of Finerenone on Albuminuria in Patients With Diabetic Nephropathy: A Randomized Clinical Trial. JAMA 2015;314:884-94. [Crossref] [PubMed]
- Tang W, Gao Y, Li Y, et al. Gene networks implicated in diabetic kidney disease. Eur Rev Med Pharmacol Sci 2012;16:1967-73. [PubMed]
- Cui C, Cui Y, Fu Y, et al. Microarray analysis reveals gene and microRNA signatures in diabetic kidney disease. Mol Med Rep 2018;17:2161-8. [PubMed]
- Kiyanpour F, Abedi M, Gheisari Y. A systematic integrative approach reveals novel microRNAs in diabetic nephropathy. J Res Med Sci 2020;25:1. [Crossref] [PubMed]
- Yang H, Lian D, Zhang X, et al. Key Genes and Signaling Pathways Contribute to the Pathogensis of Diabetic Nephropathy. Iran J Kidney Dis 2019;13:87-97. [PubMed]
- Tang YL, Dong XY, Zeng ZG, et al. Gene expression-based analysis identified NTNG1 and HGF as biomarkers for diabetic kidney disease. Medicine (Baltimore) 2020;99:e18596. [Crossref] [PubMed]
- Lun ATL, Riesenfeld S, Andrews T, et al. EmptyDrops: distinguishing cells from empty droplets in droplet-based single-cell RNA sequencing data. Genome Biol 2019;20:63. [Crossref] [PubMed]
- McCarthy DJ, Campbell KR, Lun AT, et al. Scater: pre-processing, quality control, normalization and visualization of single-cell RNA-seq data in R. Bioinformatics 2017;33:1179-86. [PubMed]
- Butler A, Hoffman P, Smibert P, et al. Integrating single-cell transcriptomic data across different conditions, technologies, and species. Nat Biotechnol 2018;36:411-20. [Crossref] [PubMed]
- Zhang X, Lan Y, Xu J, et al. CellMarker: a manually curated resource of cell markers in human and mouse. Nucleic Acids Res 2019;47:D721-8. [Crossref] [PubMed]
- Kauffmann A, Gentleman R, Huber W. arrayQualityMetrics--a bioconductor package for quality assessment of microarray data. Bioinformatics 2009;25:415-6. [Crossref] [PubMed]
- Ritchie ME, Phipson B, Wu D, et al. limma powers differential expression analyses for RNA-sequencing and microarray studies. Nucleic Acids Res 2015;43:e47. [Crossref] [PubMed]
- Ashburner M, Ball CA, Blake JA, et al. Gene ontology: tool for the unification of biology. The Gene Ontology Consortium. Nat Genet 2000;25:25-9. [Crossref] [PubMed]
- Kanehisa M, Goto S. KEGG: kyoto encyclopedia of genes and genomes. Nucleic Acids Res 2000;28:27-30. [Crossref] [PubMed]
- Franceschini A, Szklarczyk D, Frankild S, et al. STRING v9.1: protein-protein interaction networks, with increased coverage and integration. Nucleic Acids Res 2013;41:D808-15. [Crossref] [PubMed]
- Shannon P, Markiel A, Ozier O, et al. Cytoscape: a software environment for integrated models of biomolecular interaction networks. Genome Res 2003;13:2498-504. [Crossref] [PubMed]
- Bandettini WP, Kellman P, Mancini C, et al. MultiContrast Delayed Enhancement (MCODE) improves detection of subendocardial myocardial infarction by late gadolinium enhancement cardiovascular magnetic resonance: a clinical validation study. J Cardiovasc Magn Reson 2012;14:83. [Crossref] [PubMed]
- Ju W, Nair V, Smith S, et al. Tissue transcriptome-driven identification of epidermal growth factor as a chronic kidney disease biomarker. Sci Transl Med 2015;7:316ra193. [Crossref] [PubMed]
- Woroniecka KI, Park AS, Mohtat D, et al. Transcriptome analysis of human diabetic kidney disease. Diabetes 2011;60:2354-69. [Crossref] [PubMed]
- Sandholm N, Van Zuydam N, Ahlqvist E, et al. The Genetic Landscape of Renal Complications in Type 1 Diabetes. J Am Soc Nephrol 2017;28:557-74. [Crossref] [PubMed]
- Wang H, Fang J, Chen F, et al. Metabolomic profile of diabetic retinopathy: a GC-TOFMS-based approach using vitreous and aqueous humor. Acta Diabetol 2020;57:41-51. [Crossref] [PubMed]
- Klein J, Bascands JL, Buffin-Meyer B, et al. Epidermal growth factor and kidney disease: a long-lasting story. Kidney Int 2016;89:985-7. [Crossref] [PubMed]
- Wu L, Li XQ, Chang DY, et al. Associations of urinary epidermal growth factor and monocyte chemotactic protein-1 with kidney involvement in patients with diabetic kidney disease. Nephrol Dial Transplant 2020;35:291-7. [PubMed]
- Wang H, Madhusudhan T, He T, et al. Low but sustained coagulation activation ameliorates glucose-induced podocyte apoptosis: protective effect of factor V Leiden in diabetic nephropathy. Blood 2011;117:5231-42. [Crossref] [PubMed]
- Peter A, Fritsche A, Machicao F, et al. Lower plasma creatinine and urine albumin in individuals at increased risk of type 2 diabetes with factor v leiden mutation. ISRN Endocrinol 2014;2014:530830.
- Vionnet N, Tregouet D, Kazeem G, et al. Analysis of 14 candidate genes for diabetic nephropathy on chromosome 3q in European populations: strongest evidence for association with a variant in the promoter region of the adiponectin gene. Diabetes 2006;55:3166-74. [Crossref] [PubMed]
- Härma MA, Dahlström EH, Sandholm N, et al. Decreased plasma kallikrein activity is associated with reduced kidney function in individuals with type 1 diabetes. Diabetologia 2020;63:1349-54. [Crossref] [PubMed]
- Sheng J, Li H, Dai Q, et al. DUSP1 recuses diabetic nephropathy via repressing JNK-Mff-mitochondrial fission pathways. J Cell Physiol 2019;234:3043-57. [Crossref] [PubMed]
- Monteiro MB, Thieme K, Santos-Bezerra DP, et al. Beta-2-microglobulin (B2M) expression in the urinary sediment correlates with clinical markers of kidney disease in patients with type 1 diabetes. Metabolism 2016;65:816-24. [Crossref] [PubMed]
- Najafian B, Fogo AB, Lusco MA, et al. AJKD Atlas of Renal Pathology: diabetic nephropathy. Am J Kidney Dis 2015;66:e37-8. [Crossref] [PubMed]
- Nicholas SB, Liu J, Kim J, et al. Critical role for osteopontin in diabetic nephropathy. Kidney Int 2010;77:588-600. [Crossref] [PubMed]
- Nagao T, Okura T, Irita J, et al. Osteopontin plays a critical role in interstitial fibrosis but not glomerular sclerosis in diabetic nephropathy. Nephron Extra 2012;2:87-103. [Crossref] [PubMed]
- Cai M, Bompada P, Atac D, et al. Epigenetic regulation of glucose-stimulated osteopontin (OPN) expression in diabetic kidney. Biochem Biophys Res Commun 2016;469:108-13. [Crossref] [PubMed]
- de Boer IH, Rue TC, Hall YN, et al. Temporal trends in the prevalence of diabetic kidney disease in the United States. JAMA 2011;305:2532-9. [Crossref] [PubMed]
- Rubio-Guerra AF, Vargas-Robles H, Lozano Nuevo JJ, et al. Correlation between circulating adhesion molecule levels and albuminuria in type-2 diabetic hypertensive patients. Kidney Blood Press Res 2009;32:106-9. [Crossref] [PubMed]
- Stehouwer CDA, Gall M-A, Twisk JWR, et al. Increased urinary albumin excretion, endothelial dysfunction, and chronic low-grade inflammation in type 2 diabetes: progressive, interrelated, and independently associated with risk of death. Diabetes 2002;51:1157-65. [Crossref] [PubMed]
- Akiyama H, Chaboissier MC, Martin JF, et al. The transcription factor Sox9 has essential roles in successive steps of the chondrocyte differentiation pathway and is required for expression of Sox5 and Sox6. Genes Dev 2002;16:2813-28. [Crossref] [PubMed]
- Kishi S, Abe H, Akiyama H, et al. SOX9 protein induces a chondrogenic phenotype of mesangial cells and contributes to advanced diabetic nephropathy. J Biol Chem 2011;286:32162-9. [Crossref] [PubMed]
- Morrissey J, Guo G, Moridaira K, et al. Transforming growth factor-beta induces renal epithelial jagged-1 expression in fibrotic disease. J Am Soc Nephrol 2002;13:1499-508. [Crossref] [PubMed]
- Hai TW, Liu F, Coukos WJ, et al. Transcription factor ATF cDNA clones: an extensive family of leucine zipper proteins able to selectively form DNA-binding heterodimers. Genes Dev 1989;3:2083-90. [Crossref] [PubMed]
- Yin X, Dewille JW, Hai T. A potential dichotomous role of ATF3, an adaptive-response gene, in cancer development. Oncogene 2008;27:2118-27. [Crossref] [PubMed]
- Gilchrist M, Thorsson V, Li B, et al. Systems biology approaches identify ATF3 as a negative regulator of Toll-like receptor 4. Nature 2006;441:173-8. [Crossref] [PubMed]
- Mungrue IN, Pagnon J, Kohannim O, et al. CHAC1/MGC4504 is a novel proapoptotic component of the unfolded protein response, downstream of the ATF4-ATF3-CHOP cascade. J Immunol 2009;182:466-76. [Crossref] [PubMed]
- Lin H, Cheng CF. Activating transcription factor 3, an early cellular adaptive responder in ischemia/reperfusion-induced injury. Ci Ji Yi Xue Za Zhi 2018;30:61-5. [PubMed]
- Zhang H, Liang S, Du Y, et al. Inducible ATF3-NFAT axis aggravates podocyte injury. J Mol Med (Berl) 2018;96:53-64. [Crossref] [PubMed]
- Moon JY, Jeong KH, Lee TW, et al. Aberrant recruitment and activation of T cells in diabetic nephropathy. Am J Nephrol 2012;35:164-74. [Crossref] [PubMed]
- Lim AK, Ma FY, Nikolic-Paterson DJ, et al. Lymphocytes promote albuminuria, but not renal dysfunction or histological damage in a mouse model of diabetic renal injury. Diabetologia 2010;53:1772-82. [Crossref] [PubMed]
- Li O, Zheng P, Liu Y. CD24 expression on T cells is required for optimal T cell proliferation in lymphopenic host. J Exp Med 2004;200:1083-9. [Crossref] [PubMed]
- Luna-Antonio BI, Rodriguez-Muñoz R, Namorado-Tonix C, et al. Gas1 expression in parietal cells of Bowman's capsule in experimental diabetic nephropathy. Histochem Cell Biol 2017;148:33-47. [Crossref] [PubMed]
(English Language Editor: J. Gray)