The value of multiparametric histogram features based on intravoxel incoherent motion diffusion-weighted imaging (IVIM-DWI) for the differential diagnosis of liver lesions
Introduction
Diffusion-weighted imaging (DWI) is a technique that uses the characteristics of the Brownian motion of water molecules for imaging, enabling the study of the human body by magnetic resonance imaging (MRI) at the molecular level. It reflects the microstructure of human tissues and changes in the movement of water molecules inside and outside the cells and thus is valuable for determining the sensitivity of diseases and making differential diagnoses in clinical practice (1). In recent years, with the application of rapid sequences, such as echo-planar imaging, and advances in hardware, DWI has shown promising application prospects in research on liver diseases. Quantitative DWI can provide information that conventional MRI cannot provide. The measurement of the apparent diffusion coefficient (ADC) can help distinguish between benign and malignant lesions and predict and monitor the efficacy of radiotherapy and chemotherapy on malignancies (2). However, the traditional simple exponential model has certain limitations in the quantitative analysis of the diffusive motion of water molecules in tissues. For example, it ignores the effect of the microcirculation (comprised of arterioles, capillaries, and venules) on ADCs in viable tissues. Intravoxel incoherent motion (IVIM) DWI distinguishes the diffusion of water molecules in biological tissues from microcirculatory perfusion (3). There are many reports on the diagnosis of neoplastic lesions of the liver by IVIM-DWI and its quantitative parameters, namely, slow diffusion coefficient (D), pseudo-diffusion coefficient (or fast diffusion coefficient, D*), and perfusion fraction (f) (4).
MR conventional sequence images can only evaluate the uniformity of the general composition structure, and have relatively low specificity and cannot show the smoothness and uniformity of pixel gray scale in the region of interest (ROI) (5). Therefore, it is still difficult to use conventional MRI in the differential diagnosis of liver lesions. The application of texture analysis (TA) provides new ideas for medical image processing (6). Multiparametric histogram features of IVIM-DWI is a region of interest (ROI)—based image processing method, on the basis of routine sequence by extracting the feature value of the ROI in the image, quantifies the image information in a quantitative way, and provides more information about the internal tumor heterogeneity that is difficult for the human eye to distinguish. It can avoid the subjective bias of readers in assessing the characteristics of grayscale images, giving it high reliability and repeatability. In recent years, TA of MR images has been used in the assessment of lesions in the brain, prostate, breast, liver, and other organs. In these studies, TA has been proved to be useful in identifying tumor types and predicting tumor grade and prognosis (5-7).
Histogram analysis is one of the commonly used methods of texture analysis. It mainly describes the number of pixels in a certain gray level of an image, the range of gray level distribution, the degree of brightness and darkness and contrast, and provides parameters related to disease identification and classification. The results show that the histogram method can be used to obtain more detailed characteristic information of lesions, which is of great significance for the differentiation, staging and efficacy evaluation of lesions (8,9). However, there are relatively few studies on the differential diagnosis efficacy of histogram TA in liver space-occupying lesions. The current study analyzed the whole-lesion histogram parameters for IVIM-DWI and explored their capability in differentiating between hepatic hemangioma (HH), hepatic cysts (HC) and hepatocellular carcinoma (HCC). The results provide a reference for the clinical diagnosis of these diseases.
We present the following article in accordance with the STARD reporting checklist (available at http://dx.doi.org/10.21037/atm-20-5109).
Methods
Research subjects
This was a retrospective study. In accordance with the ethical requirements for medical research, 89 patients with space-occupying lesions of the liver in our hospital between June 2016 and October 2019 were selected from the Picture Archiving and Communication Systems (PACS) according to the inclusion and exclusion criteria. In all, this study had 33 cases of HH [19 males and 14 females, aged 28–72 (51.42±13.50) years, Group HH], 22 cases of HC [12 males and 10 females, aged 20–80 (51.3±15.2) years, Group HC], and 34 cases of HCC [31 males and 3 females, aged 33–58 (52.4±10.0) years, Group HCC].
This study was reviewed and approved by the Ethics Committee of the Panyu Central Hospital (approval number: H20160005) and carried out in strict accordance with the signed research protocol, the clinical trial regulations, and the Declaration of Helsinki (as revised in 2013). The requirement for informed consent was waived because of the retrospective nature of the research.
Inclusion criteria
Patients who met the following criteria were included in this study. (I) They received routine IVIM-DWI MRI scans. (II) All lesions were not treated before MRI examination. (III) All lesions were confirmed by clinical follow-up, biopsy, or postoperative pathology.
Exclusion criteria
Patients who met the following criteria were excluded. (I) The images were of poor quality and could not be used for analysis. (II) The conventional IVIM-DWI MR image data were incomplete. (III) The lesions were too small to be analyzed with the software.
MRI examinations
Patients were scanned in a head-first orientation while in the supine position in a Siemens Avanto 1.5-T superconductive MRI scanner (Magnetom Avanto; Siemens Medical Solutions, Erlangen, Germany) with a 12-channel spine coil and a 6-channel body coil. Before the examination, the patients were required to fast for 4 h and complete the respiratory training, including free breathing at a steady pace and end-inspiratory breath hold. The scan range covered the whole liver. The following MRI sequences were acquired: (I) axial breath-hold T1-weighted imaging (T1WI) double-echo sequences, (II) axial respiratory-triggered fat-suppressed T2-weighted imaging (T2WI) sequences; and (III) axial respiratory-triggered IVIM-DWI sequences. The scan settings were as follows: repetition time =3,000 ms, echo time (TE) =74 ms, field of view =400×296 mm, matrix =128×128, parallel acquisition (parallel acquisition factor =2), slice thickness =5 mm, interslice gap =1.6 mm, spectral adiabatic inversion recovery for fat suppression, and b values =0, 100, 300 and 600 s/mm2.
Image analysis
The data were imported into the Diffusion Imaging application in the Medical Imaging Interaction Toolkit to obtain the parameter maps in the biexponential model, including those of D, D* and f. Referring to fat-suppressed T2WI and multiphase dynamic contrast-enhanced images, the slices with few artifacts and little tumor necrosis were selected on each IVIM image, and the ROI was delineated within the largest solid component of the tumor, avoiding hemorrhage, necrosis, and cystic lesions. The measurements were repeated three times to obtain the mean values of D, D* and f of the lesion. All ROIs were delineated by one person. The three-dimensional (3D) ROIs were automatically generated by the software, the texture features of each lesion were calculated using the software, and the histogram data of each lesion were converted to the corresponding frequency distribution table and then imported into SPSS for analysis. Finally, for each of D, D*, and f, 13 histogram parameters were derived from the IVIM images of the lesions: the minimum value, median, mean, maximum value, kurtosis, entropy, energy, 90th percentile (P90), 10th percentile (P10), standard deviation, uniformity, skewness, and maximum diameter.
Statistical analysis
The measured data were analyzed with SPSS 19.0. The normality was analyzed using the Kolmogorov-Smirnov test. Data with a normal distribution are expressed as mean ± standard deviation, and data with a skewed distribution are expressed as median (upper and lower quartiles). The differences in histogram parameters of D, D*, and f between the three groups were tested using the Kruskal-Wallis H test. According to the diagnosis results, a receiver operating characteristic (ROC) curve was plotted, and the area under the ROC curves (AUCs) of the histogram parameters were compared to evaluate their diagnostic efficacies. The optimal diagnostic threshold for each parameter was determined from the Youden index. P<0.05 was considered statistically significant.
Results
Histogram parameters of D
The maximum diameter, maximum value, minimum value, mean, median, standard deviation, uniformity, skewness, kurtosis, volume, P10, and P90 of D were significantly different between the three groups (all P<0.05). The entropy of D was not significantly different between the three groups (P>0.05). See Table 1 and Figures 1-4.
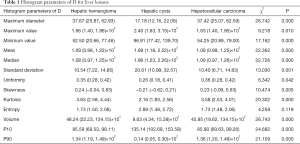
Full table
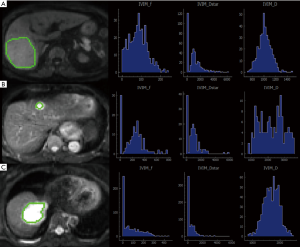
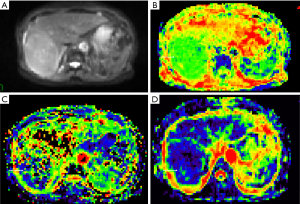
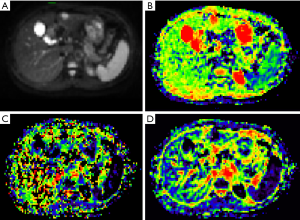
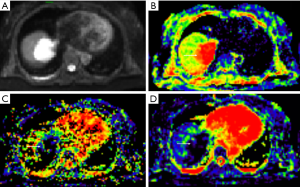
Histogram parameters of D*
The minimum value, entropy, volume and P10 of D* were significantly different between the three groups (all P<0.05). The maximum value, mean, median, standard deviation, uniformity, skewness, kurtosis and P90 of D* were not significantly different between the three groups (all P>0.05). See Table 2 and Figures 1-4.
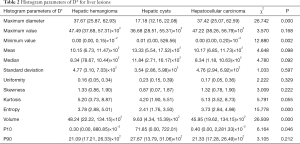
Full table
Histogram parameters of f
The maximum diameter, minimum value, mean, median, skewness, kurtosis, volume, P10, and P90 of f were significantly different between the three groups (all P<0.05). The maximum value, standard deviation, uniformity, and entropy of f were not significantly different between the three groups (all P>0.05). See Table 3 and Figures 1-4.
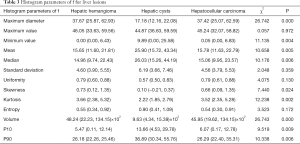
Full table
Efficacies of histogram parameters in differentiating among HH, HC, and HCC
Efficacies of histogram parameters in differentiating between HH and HC
In differentiating between HH and HC, the AUCs for D, D*, and f were 0.64–0.882, 0.612–0.883, and 0.631–0.883, respectively. The largest AUC for both D* and f was that of volume (AUC =0.883 for both). When 1,438.802 was used as the threshold, the sensitivity and specificity of volume for differentiation between HH and HC were 87.88 and 77.27, respectively. See Table 4 and Figure 5.
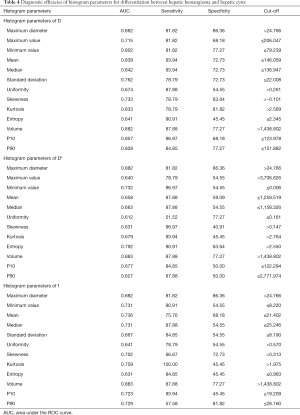
Full table
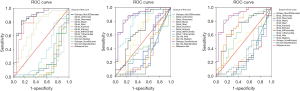
The diagnostic efficacies of histogram parameters in differentiating between HC and HCC
In differentiating between HC and HCC, the AUCs for D, D*, and f were 0.652–0.856, 0.600–0.857, and 0.636–0.857, respectively. The largest AUCs for D* and f were those of volume (AUC =0.883 for both). When 1,438.802 was used as the cut-off volume, the sensitivity and specificity for differentiating between HC and HCC were 77.27 and 85.29, respectively. See Table 5 and Figure 6.
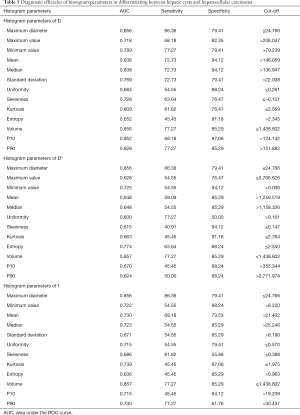
Full table
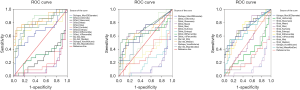
The diagnostic efficacies of histogram parameters in differentiating between HH and HCC
In differentiating between HH and HCC, the AUCs for D, D*, and f were all less than 0.6, and the clinical diagnosis accuracies were extremely low (Figure 7).
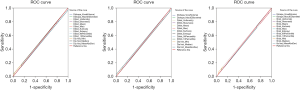
Discussion
HH and HC are the most common benign liver lesions. Cavernous hemangioma, the most common HH, is rich in sinusoids and blood. The water content of the blood exceeds 80%. HC are also rich in water content. Both HH and HC have a long T1 and a long T2 on MRI, it is difficult to distinguish them by conventional MRI scanning sequence. HCC is the most common malignancy of the liver. Patients with typical HCC symptoms can be qualitatively diagnosed by imaging and clinical evidence. However, it is difficult to qualitatively diagnose patients who have no specific imaging features or patients who cannot receive contrast-enhanced imaging due to adverse reactions to contrast agents.
DWI is effective for the detection and differential diagnosis of liver lesions. It has been widely implemented in routine clinical MRI programs (10,11). Low-b-value DWI has a high sensitivity for lesion detection (12,13), and the ADC is an important parameter reflecting the characteristics of a lesion. However, due to the influence of other types of incoherent motion [such as perfusion (14)], the simple exponential model may not satisfy the need for further exploration. Le Bihan et al. (15) proposed the concept of IVIM and used the biexponential model, which can obtain tissue perfusion and diffusion information at the same time without using contrast agents (14). The water molecules in the human body are divided into two parts, one is the water molecules located in the intracellular and intercellular substance, and the extracellular space, and the other is the water molecules in the microcirculation. This assumption is made in this model. The distribution of the vascular system in the body is random and disordered, and the water molecules in the blood are also doing relatively random and disorderly movement, but its macroscopic diffusion rate is usually significantly faster than the conventional water molecular diffusion, so it is usually called pseudo random movement, this part of perfusion can be solved by the familiar diffusion model. Based on this principle, the IVIM model can separate two different diffusion components, and its double-e exponent model can calculate two diffusion coefficients, one is the true diffusion coefficient D, also called the slow diffusion coefficient, the true diffusion coefficient of water molecules in tissues after microcirculation perfusion is removed, representing the mobility of water molecules in tissues, which depends on the number of cells, the curvature of the extracellular space, the integrity of the cell membrane and the viscosity of the liquid (11,12,16,17); one is the pseudo diffusion coefficient D*, also known as the fast diffusion coefficient, which corresponds to the perfusion signal and depends on the blood flow velocity and the length of the microvessel segment (14,15); in addition, there is the proportional coefficient f corresponding to the fast diffusion, that is, the perfusion fraction, which reflects the relative contribution of the microvascular blood flow to the DWI signal (18). Histogram analysis based on pixel distribution is a new technology for analyzing parameter mapping. It predicts the mean signal of the ROI, the number of pixels, and the range of interpixel signal variation by analyzing the signal values in the entire lesion, thereby providing quantitative information on tumor heterogeneity (19). Contrast-enhanced scanning is of great significance for the differential diagnosis of HH, HC, and HCC. However, some patients are not suitable for contrast-enhanced scanning due to certain kidney diseases and allergies. This study aims to clarify the application value of histogram parameters in the differential diagnosis of HH, HC, and HCC and to provide a reference for diagnosis of patients who have contraindications for contrast-enhanced scans.
Few studies have used the IVIM histogram to analyze liver lesions. Li et al. (20) investigated the value of histogram analysis of IVIM based on whole-tumor volume in the prediction of microvascular invasion (MVI) in single HCC. They found that histogram analysis of IVIM based on whole-tumor volume could be applied in the prediction of MVI and that the 5th percentile of D was most useful to predict MVI in HCC. Zheng et al. (21) believed that traditional DWI had limited worth in the quantitative evaluation of liver fibrosis and that whole-liver ADC histogram analysis could be helpful for the diagnosis and staging of liver fibrosis. They found that kurtosis, entropy, skewness, mode, and the 90th and 75th percentiles of ADC may be helpful for the diagnosis and staging of liver fibrosis and that among these parameters, kurtosis was the most useful.
In this study, we found that histogram parameters can help differentiate between HH, HC, and HCC. The histogram features of the image are the one-dimensional statistics reflecting the distribution of gray values. According to IVIM theory, D is a parameter that simply reflects the diffusive motion of water molecules, that is, the dispersion of water molecules in tissues. D mainly depends on the cell density and extracellular matrix components in tissues and is negatively correlated with the nucleocytoplasmic ratio in tissues. Therefore, the higher the cell density is, the higher the nucleocytoplasmic ratio and the smaller the extracellular space are, so the more limited the diffusion of water molecules will be (22-25), and the smaller the D value is. Studies on benign and malignant nodules of the lung, liver cancer, prostate cancer, and breast cancer (22-26) found that the D values of tumors with high malignancy were significantly lower than those of benign lesions and normal surrounding tissues. This study showed that the maximum value, minimum value, and standard deviation of D in HCC were smaller than those of HH and HC, which to some extent indicates that the water molecule diffusion was more restricted in hepatic malignancies than in benign liver lesions. The mean, median, uniformity, entropy, and P10 of D in HCC were close to or equal to those of HH, which may be related to the presence of some atypical hemangiomas. Histology showed that the unfilled gaps and internal septa in the center of giant HHs were cystic degeneration, liquefaction, or fibrous tissue (27,28), and mucoid degeneration, bleeding, and necrosis were sometimes seen. In addition, some hemangiomas may have a fluid-liquid level (29), which is a common sign in malignant liver lesions, to some extent making the differentiation between HH and HCC difficult.
The D* value is positively proportional to the average blood flow velocity and the average capillary segment length, indicating that D* can reflect the vascular and microcirculatory perfusion of the tumor. The higher the D*, the higher the microcirculatory perfusion in the tissue (30,31). Like D*, f is a perfusion-related parameter. The f value reflects the microvessel density and to some extent the rate of microangiogenesis (31). Ichikawa et al. (32) compared benign and malignant focal liver lesions using IVIM-DWI. The results showed that the D and D* values of the malignant group were significantly lower than those of the benign group and that the f values were not significantly different between the two groups. Luo et al. (33) found that ADC, D, and f in the malignant group were significantly lower than those in the benign group and that among the three parameters, D had the highest efficacy in differentiating between benign and malignant lesions, whereas, D* was not significantly different between the two groups. In the present study, the maximum diameter, minimum value, entropy, volume and P10 of D* were significantly different between the three groups (P<0.05); the maximum diameter, minimum value, mean, median, skewness, kurtosis, volume and P90 of f were significantly different between the three groups (P<0.05); and the volume of D* and the volume of f had high diagnostic efficacy in differentiating between HH and HC and between HC and HCC.
D* and f are controversial in the differential diagnosis of liver lesions. The controversy might be related to differences in the lesion type, the sample size, the b value used for scanning, and the blood supply of tumors of different types. Hence, the diagnostic values of D* and f need to be further studied. In addition, the measurement of f largely depends on the MRI scanning scheme and the relevant parameters of the magnetic field—different TEs and T2 relaxation times can lead to different f values (34). Therefore, it is necessary to scientifically standardize the settings of TE and T2 for scanning.
Entropy refers to the statistical measure of the irregularity in the histogram, which describes the changes in the parameter distribution in the ROI and can reflect the complexity of the internal signal of the tumor to a certain extent. The greater the entropy, the more uneven the tumor signal. The entropy values of D* were significantly different between the three groups. The entropy values were highest for HH, followed by HCC and then by HC, indicating that HH has greater heterogeneity than HC and HCC. This may be related to the types of hemangioma included in this study. Maximum diameter was significantly different between the three groups (P<0.05), and the maximum diameter of HH was largest. Its large maximum diameter might have increased the heterogeneity of HH to some extent and given it a high entropy.
Percentiles can reflect the voxels that underlie histograms (35) and quantify the internal heterogeneity of tumors. In the present study, P10 and P90 of D and f were significantly different between the three groups (P<0.05), indicating that the voxel values were significantly different between the three types of hepatic lesions. Therefore, P10 and P90 of D and f are useful in differential diagnosis.
This study has some inadequacies: (I) it was a retrospective analysis that assumed that MRI acquisition, processing, and analysis were performed under the same conditions. (II) The small sample size of the three types of liver lesions may have led to biased results, which may also be the reason why some characteristic histogram parameters had poor clinical diagnostic efficacies for the three types of liver lesions. (III) Only three types of liver lesions were studied, and the interobserver reliability was not analyzed but needs further in-depth study.
In summary, the IVIM-DWI MRI histogram can reflect the signal distribution inside the HH, HC, and HCC and can provide more information for the differentiation between the three. Some of the histogram parameters of D, D*, and f have high diagnostic values. Among them, the AUCs of the volume of D* and the volume of f were the largest. Therefore, the volume of D* and volume of f had better diagnostic efficacy than other histogram parameters and could provide valuable reference information for clinical differential diagnosis.
Acknowledgments
Funding: This research was supported by grants from National Natural Science Foundation of China (No. 81671853), Natural Science Foundation of Guangdong (No. 2015A030313753), the Science and Technology Program of Guangzhou (No. 201903010032) and the Panyu Science and Technology Program of Guangzhou (No. 2017-Z04-12; 2019-Z04-01; 2019-Z04-23).
Footnote
Reporting Checklist: The authors have completed the STARD reporting checklist. Available at http://dx.doi.org/10.21037/atm-20-5109
Data Sharing Statement: Available at http://dx.doi.org/10.21037/atm-20-5109
Conflicts of Interest: All authors have completed the ICMJE uniform disclosure form (available at http://dx.doi.org/10.21037/atm-20-5109). The authors have no conflicts of interest to declare.
Ethical Statement: The authors are accountable for all aspects of the work in ensuring that questions related to the accuracy or integrity of any part of the work are appropriately investigated and resolved. This study was reviewed and approved by the Ethics Committee of the Panyu Central Hospital (approval number: H20160005) and carried out in strict accordance with the signed research protocol, the clinical trial regulations, and the Declaration of Helsinki (as revised in 2013). The requirement for informed consent was waived because of the retrospective nature of the research.
Open Access Statement: This is an Open Access article distributed in accordance with the Creative Commons Attribution-NonCommercial-NoDerivs 4.0 International License (CC BY-NC-ND 4.0), which permits the non-commercial replication and distribution of the article with the strict proviso that no changes or edits are made and the original work is properly cited (including links to both the formal publication through the relevant DOI and the license). See: https://creativecommons.org/licenses/by-nc-nd/4.0/.
References
- Koh DM, Collins DJ. Diffusion-weighted MRI in the body: applications and challenges in oncology. AJR Am J Roentgenol 2007;188:1622-35. [Crossref] [PubMed]
- Grasparil ADI, Gupta H, Sheybani E, et al. Low b-value (50-100) diffusion-weighted images detect significantly more hyperintense liver lesions in children than T2-weighted images. Pediatr Radiol 2019;49:1299-305. [Crossref] [PubMed]
- Le Bihan D, Breton E, Lallemand D, et al. Separation of diffusion and perfusion in intravoxel incoherent motion MR imaging. Radiology 1988;168:497-505. [Crossref] [PubMed]
- Wang QQ, Liu XJ. Advances in application of IVIM-DWI in liver focal lesions. Chinese Journal of Magnetic Resonance Imaging 2018;9:312-5.
- Zhao Q, Xie T, Fu C, et al. Differentiation between idiopathic granulomatous mastitis and invasive breast carcinoma, both presenting with non-mass enhancement without rim-enhanced masses: The value of whole-lesion histogram and texture analysis using apparent diffusion coefficient. Eur J Radiol 2020;123:108782. [Crossref] [PubMed]
- Ye Z, Jiang H, Chen J, et al. Texture analysis on gadoxetic acid enhanced-MRI for predicting Ki-67 status in hepatocellular carcinoma: A prospective study. Chin J Cancer Res 2019;31:806-17. [Crossref] [PubMed]
- Nardone V, Reginelli A, Scala F, et al. Magnetic-Resonance-Imaging Texture Analysis Predicts Early Progression in Rectal Cancer Patients Undergoing Neoadjuvant Chemoradiation. Gastroenterol Res Pract 2019;2019:8505798. [Crossref] [PubMed]
- He W, Xiao X, Li X, et al. Whole-tumor histogram analysis of apparent diffusion coefficient in differentiating intracranial solitary fibrous tumor/hemangiopericytoma from angiomatous meningioma. Eur J Radiol 2019;112:186-91. [Crossref] [PubMed]
- Li H, Li A, Zhu H, et al. Whole-Tumor Quantitative Apparent Diffusion Coefficient Histogram and Texture Analysis to Differentiation of Minimal Fat Angiomyolipoma from Clear Cell Renal Cell Carcinoma. Acad Radiol 2019;26:632-9. [Crossref] [PubMed]
- Chang N, Wang XH, Cui LB, et al. Diagnostic performance of diffusion-weighted magnetic resonance imaging in pulmonary malignant lesions: a meta-analysis. Transl Lung Cancer Res 2019;8:738-47. [Crossref] [PubMed]
- Swinburne NC, Schefflein J, Sakai Y, et al. Machine learning for semiautomated classification of glioblastoma, brain metastasis and central nervous system lymphoma using magnetic resonance advanced imaging. Ann Transl Med 2019;7:232. [Crossref] [PubMed]
- Takahara T, Kwee TC. Low b-value diffusion-weighted imaging: emerging applications in the body. J Magn Reson Imaging 2012;35:1266-73. [Crossref] [PubMed]
- Kwee TC, Takahara T. Diffusion-weighted MRI for detecting liver metastases: importance of the b-value. Eur Radiol 2011;21:150. [Crossref] [PubMed]
- Koh DM, Collins DJ, Orton MR. Intravoxel incoherent motion in body diffusion-weighted MRI: reality and challenges. AJR Am J Roentgenol 2011;196:1351-61. [Crossref] [PubMed]
- Le Bihan D, Breton E, Lallemand D, et al. Separation of diffusion and perfusion in intravoxel incoherent motion MR imaging. Radiology 1988;168:497-505. [Crossref] [PubMed]
- Padhani A R, Liu G, Mu-Koh D, et al. Diffusion-Weighted Magnetic Resonance Imaging as a Cancer Biomarker: Consensus and Recommendations. Neoplasia 2009;11:102-25. [Crossref] [PubMed]
- Aoyagi T, Shuto K, Okazumi S, et al. Apparent diffusion coefficient correlation with oesophageal tumour stroma and angiogenesis. Eur Radiol 2012;22:1172-7. [Crossref] [PubMed]
- Luo M, Zhang L, Jiang XH, et al. Intravoxel incoherent motion: application in differentiation of hepatocellular carcinoma and focal nodular hyperplasia. Diagn Interv Radiol 2017;23:263-71. [Crossref] [PubMed]
- Xu XQ, Hu H, Su GY, et al. Utility of histogram analysis of ADC maps for differentiating orbital tumors. Diagn Interv Radiol 2016;22:161-7. [Crossref] [PubMed]
- Li H, Zhang J, Zheng Z, et al. Preoperative histogram analysis of intravoxel incoherent motion (IVIM) for predicting microvascular invasion in patients with single hepatocellular carcinoma. Eur J Radiol 2018;105:65-71. [Crossref] [PubMed]
- Zheng Y, Xu YS, Liu Z, et al. Whole-Liver Apparent Diffusion Coefficient Histogram Analysis for the Diagnosis and Staging of Liver Fibrosis. J Magn Reson Imaging 2020;51:1745-54. [Crossref] [PubMed]
- Woo S, Lee JM, Yoon JH, et al. Intravoxel Incoherent Motion Diffusion-weighted MR Imaging of Hepatocellular Carcinoma: Correlation with Enhancement Degree and Histologic Grade. Radiology 2014;270:758-67. [Crossref] [PubMed]
- Yuan M, Pu XH, Xu XQ, et al. Lung adenocarcinoma: Assessment of epidermal growth factor receptor mutation status based on extended models of diffusion-weighted image. J Magn Reson Imaging 2017;46:281-9. [Crossref] [PubMed]
- Yuan M, Zhong Y, Zhang YD, et al. Volumetric analysis of intravoxel incoherent motion imaging for assessment of solitary pulmonary lesions. Acta Radiol 2017;58:1448-56. [Crossref] [PubMed]
- Lee YJ, Kim SH, Kang BJ, et al. Intravoxel incoherent motion (IVIM)-derived parameters in diffusion-weighted MRI: Associations with prognostic factors in invasive ductal carcinoma. J Magn Reson Imaging 2017;45:1394-406. [Crossref] [PubMed]
- Zhang YD, Wang Q, Wu CJ, et al. The histogram analysis of diffusion-weighted intravoxel incoherent motion (IVIM) imaging for differentiating the gleason grade of prostate cancer. Eur Radiol 2015;25:994-1004. [Crossref] [PubMed]
- Jang HJ, Kim TK, Lim HK, et al. Hepatic hemangioma: atypical appearances on CT, MR imaging, and sonography. AJR Am J Roentgenol 2003;180:135-41. [Crossref] [PubMed]
- Coumbaras M, Wendum D, Monnier-Cholley L, et al. CT and MR imaging features of pathologically proven atypical giant hemangiomas of the liver. AJR Am J Roentgenol 2002;179:1457-63. [Crossref] [PubMed]
- Ghai S, Dill-Macky M, Wilson S, et al. Fluid-fluid levels in cavernous hemangiomas of the liver: baffled? AJR Am J Roentgenol 2005;184:S82-5. [Crossref] [PubMed]
- Guo W, Luo D, Lin M, et al. Pretreatment Intra-Voxel Incoherent Motion Diffusion-Weighted Imaging (IVIM-DWI) in Predicting Induction Chemotherapy Response in Locally Advanced Hypopharyngeal Carcinoma. Medicine 2016;95:e3039. [Crossref] [PubMed]
- Le Bihan D, Turner R. The capillary network: a link between IVIM and classical perfusion. Magn Reson Med 1992;27:171-8. [Crossref] [PubMed]
- Ichikawa S, Motosugi U, Ichikawa T, et al. Intravoxel incoherent motion imaging of focal hepatic lesions. J Magn Reson Imaging 2013;37:1371-6. [Crossref] [PubMed]
- Luo M, Zhang L, Jiang XH, et al. Intravoxel Incoherent Motion Diffusion-weighted Imaging: Evaluation of the Differentiation of Solid Hepatic Lesions. Transl Oncol 2017;10:831-8. [Crossref] [PubMed]
- Lemke A, Laun FB, Simon D, et al. An in vivo verification of the intravoxel incoherent motion effect in diffusion-weighted imaging of the abdomen. Magn Reson Med 2010;64:1580-5. [Crossref] [PubMed]
- Lu SS, Kim SJ, Kim N, et al. Histogram analysis of apparent diffusion coefficient maps for differentiating primary CNS lymphomas from tumefactive demyelinating lesions. AJR Am J Roentgenol 2015;204:827-34. [Crossref] [PubMed]