Investigation of hub genes involved in diabetic nephropathy using biological informatics methods
Introduction
Diabetic nephropathy (DN) is a serious impairment of renal function caused by poor blood glucose control in diabetic patients. The clinical incidence of DN is high, treatment is difficult, and prognosis is poor (1). The typical clinical manifestations of DN include a decreased glomerular filtration rate and persistent proteinuria. Patients with severe DN will progress to uremia within 2–3 years of onset, and then many serious complications and death will occur (2). Pathophysiological studies have found that the progression of DN is closely related to genetic factors, metabolic changes, and hemodynamic changes (3).
The microarray technology and bioinformatic analysis allow for the screening of genetic alterations on the genome level. However, independent microarray analysis always lead to false‐positive rates. In this study, we re-analyzed two microarray datasets, GSE30528 and GSE1009, with a different statistical significance detection method which resulted in a dissimilar number of differentially expressed genes (DEGs). Key biomarkers with high specificity and sensitivity were identified by detecting genes with significant differences in expression between DN and normal kidney tissues. The pathogenesis of DN was studied by Gene Ontology (GO), Kyoto Encyclopedia of Genes and Genomes (KEGG) pathway enrichment. and protein-protein interaction (PPI) network analysis. The Nephroseq v5 platform was used to analyse correlations and to perform subgroup analysis among the hub genes and clinical manifestations of DN to further explore the pathogenesis, pathophysiological mechanisms, and key genes involved in DN.
We present the following article in accordance with the MDAR reporting checklist (available at http://dx.doi.org/10.21037/atm-20-5647).
Methods
Microarray data and preprocessing
From the Gene Expression Omnibus (GEO) database, established by Baelde et al., we successfully obtained GSE1009 free microarray data (4). The data in the dataset were derived from three pairs of normally functioning glomerulus and diabetic glomerulus samples that were obtained from autopsy. At the same time, to facilitate data comparisons, we obtained GSE30528 microarray data from this database. These data were first recorded by Woroniecka et al. (5). A total of 22 samples were included, 9 of which were from DN patients, and 13 of which were from normal human kidney tissues. The study was conducted in accordance with the Declaration of Helsinki (as revised in 2013).
Data processing of DEGs
DEGs in kidney tissues of DN and normal subjects were detected by GEO2R online tool (6) with |logFC| >1.5 and P<0.05. GEO2R is an interactive web tool that allows users to compare two or more datasets in a GEO series to identify DEGs across experimental conditions. Subsequently, the original data recorded in the TXT format were validated online by Venn to find significant DEGs in the two datasets; when the test results indicated log FC <0, the expression of the gene was deemed to be downregulated; if log FC >0, gene expression was deemed to be upregulated.
GO and pathway enrichment analysis
The purpose of GO is to unify biological factors while integrating specific definitions, clear structures, and controlled vocabulary into a database (7). Meanwhile, KEGG is a commonly used database for gene research. The KEGG database can conveniently classify all kinds of genes with system path management (8). The Database for Annotation, Visualization, and Integrated Discovery (DAVID) is also a commonly used tool in bioinformatics research. The advantages of DAVID include the detailed analysis and classification of gene and protein functions (9). In our study, we used DAVID to envisage the biological process (BP), molecular function (MF), pathway and cell component (CC), and other DEGs to collect more information in our research.
PPI network analysis and hub gene identification
The PPI network we used was based on Cytoscape software version 3.7.1, which can accurately predict protein-related experimental data and PPI results in the target database (10,11). The results express the interaction between different proteins as a combination fraction. After DEG information was evaluated in the PPI network, if the comprehensive score was more than 0.4, these DEGs were known as an important protein pair. This network system can use the Coexpedia (12) online platform to analyze the hub genes and related gene expression network system. After testing different gene pairs in Clusterone 1.0, PPI network modules with a minimum size of 3 and a minimum density of 0.5 were obtained. A module was considered to have a significant clustering effect when P<0.01.
Statistical analysis
Pearson’s correlation analysis between hub genes, glomerular filtration rate (GFR), and proteinuria in patients with DN was performed using Nephroseq v5 online platform (http://v5.nephroseq.org). Comparisons between two groups were performed using an unpaired Student’s t-test. All tests were two-tailed, with a P value <0.05 considered statistically significant.
Results
Identification of DEGs in DN
The free NCBI-GEO database was used to search the gene expression profiles of GSE30528 and GSE1009 in kidney tissues of DN patients. Among these datasets, the GSE30528 microarray data package contained 22 samples of human glomerular tissue. The study samples were taken from healthy human transplant donors, diagnostic renal biopsies, and post-nephrectomy specimens. Further analysis of the specific composition showed that it included 9 DN kidney tissue samples and 13 control group kidney tissue samples. The GSE1009 microarray data included 6 human glomerular samples, including DN samples and 3 samples in the control group.
The data obtained from the database were analysed by GEO2R. The cut-off criteria were [log FC] >1.5 and P<0.05. We found 274 and 1133 differentially expressed loci in GSE30528 and GSE1009 gene data. Then, Venn diagram software was used to identify the common DEGs in these two data sets. A total of 110 DEGs were detected, 96 of which were downregulated, and 14 of which were upregulated (log FC <0) (Table 1 and Figure 1).
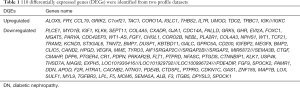
Full table
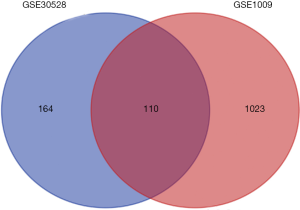
DEG GO analysis in DN
DAVID software and GO analysis were used to further study the 110 DEGs that were identified. These DEGs can be divided into three basic types: molecular structure and function, cell component (CC), and BP. MF analysis showed that the main functions of these genes were the regulation of heparin binding and protein tyrosine phosphatase activity. Genes in CC mainly involved nuclear membrane, collagen IV trimer, lamellipodium, postsynaptic density, actin filament, focal adhesion, extracellular matrix of proteins, extracellular region, extracellular exosome, and extracellular space. In BP, the functions of these genes were mainly involved in regulation of cell differentiation, phosphatidylinositol 3-kinase signaling, prostaglandin metabolic process, osteoblast differentiation, angiogenesis, ERK1 and ERK2 cascade, MAPK activity, tachykinin in receptor signaling pathway, prostatic bud formation, cysteine-type endopeptidase activity involved in the apoptotic process, angiogenesis, epithelial cell proliferation, cell migration, response to prostaglandin E, FGF receptor signaling pathway, angiogenesis, and glomerular basement membrane development (Table 2).
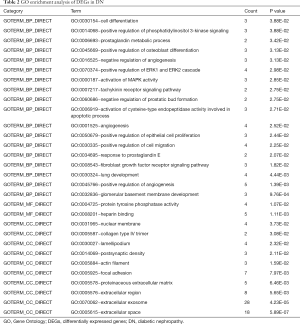
Full table
Signalling pathway enrichment analysis
KEGG pathway analysis was used to verify DEG functions and signalling pathway enrichment. In all, 9 important signalling pathways were identified, including the regulation of actin cytoskeleton, ECM-receptor interaction, dilated cardiomyopathy, hypertrophic cardiomyopathy (HCM), Ras signaling pathway, pathways in cancer, Rap1 signaling pathway, PI3K-Akt signaling pathway, and focal adhesion (Table 3).
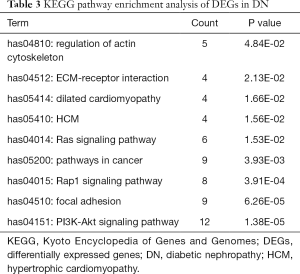
Full table
PPI network analysis
The construction of the PPI network was based on Search Tool for the Retrieval of Interacting Genes/Proteins (STRING) online analysis (Figure 2). A total of 110 DEG data points were imported into the PPI network for analysis, covering 188 edges and 79 key nodes (Figure 2A). Subsequently, 14 central nodes were identified by cell-type steroid optimization analysis: ALB, BMP2, FLT1, CTGF, PDPN, IGF1, GIA1, FGF9, BMP7, FGF1, VEGFA, TGFBR3, WT1, and IGFBP2 (Figure 2B).
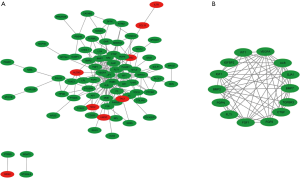
Association between the hub genes and clinical features of DN
Of the common genes identified in this study, 14 were recognized as hub genes (Table 4). In Figure 3, the network of hub genes and related co-expressed genes by Coexpedia is displayed.
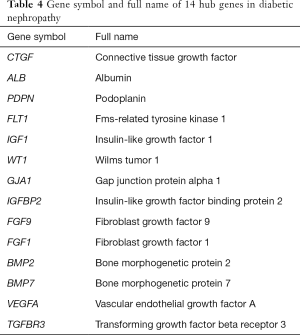
Full table
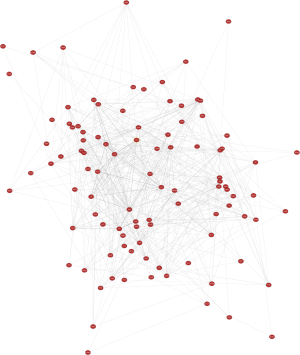
Subsequently, we focused on the changes in the hub genes in kidney tissue samples from DN patients. With the help of Nephroseq v5, the correlation between the unknown hub genes and GFR was determined. The expression of BRMP 2, CTGF, FGF9, and iGFBP2 in kidney tissue samples of DN patients was negatively correlated with GFR. Thus, the expression changes of these four genes directly affect the occurrence and development of DN. The expression of the ALB, BMP7, TGFBR3, and VEGFA genes was positively correlated with GFR. Thus, the expression of these four genes contributes to the maintenance and repair of renal function (Figure 4).
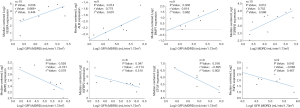
The Nephroseq v5 results indicated that the expression of the GJA1 gene was positively correlated with proteinuria in DN patients (Figure 5), indicating that the high expression of GJA1 promoted the progression and deterioration of DN. However, the level of proteinuria in DN patients decreased significantly with the increase of the expression of genes such as VEGFA, CTGF, FLT1, IGF1, and TGFBR 3. There was a negative correlation between proteinuria and the expression of these genes, which indicated that the expression of these genes contributed to the maintenance and repair of renal function.
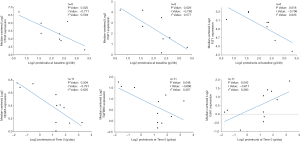
Discussion
In developed countries, the incidence of type 2 diabetes mellitus has risen dramatically, with renal dysfunction and failure having become the main cause of diabetes-related death (13). The occurrence of diabetes mellitus and the occurrence of the later stages of renal disease are influenced by both genetic and environmental factors (14). In our research, we successfully analyzed the expression pattern of target genes from the GEO database and further identified 110 genes with significant differences between DN and normal populations. Bioinformatics tools such as GO and KEGG helped us to examine the interaction between DEGs. The results showed that genes with significant expression differences were mainly involved in cell maturation and differentiation, cell migration, extracellular area, extracellular matrix components, exons, and other processes (15).
KEGG enrichment analysis of DEGs showed that many of these genes were related to regulation of actin cytoskeleton, ECM-receptor interaction, Ras signaling pathway, focal adhesion, and PI3K-Akt signaling pathway. From a pathological point of view, glomerular hypertrophy and proliferation of the renal basement membrane are often the beginning of glomerular injury in patients with DN. Extracellular bodies also play an important role in the progression of DN. The appearance of extracellular vesicles in renal parenchymal cells can be used as a marker of DN (16). The deposition of a large number of proteins in the basement membrane will eventually lead to glomerulosclerosis. The collagen binding and the interaction between ECM receptors are closely related to the degree and progression of glomerulosclerosis.
Fourteen genes, ALB, BMP2, FLT1, CTGF, PDPN, IGF1, GIA1, FGF9, BMP7, FGF1, VEGFA, TGFBR3, WT1, and IGFBP2, showed significant differences in expression and were identified as key genes. Among these genes, the albumin (ALB) gene encodes serum ALB. Serum ALB, known as the “intravascular porter”, can combine with various ions, hormone molecules, and drug molecules. Serum ALB also has many physiological functions, such as stabilizing the colloidal osmotic pressure, and anti-inflammatory and anti-oxidation functions (17). The results of this study showed that the increase in ALB expression led to a significant increase in GFR expression. A study conducted by Zhao et al. showed that serum ALB levels were negatively correlated with cholesterol histopathological changes and urinary protein content (18). Baelde et al. used bioinformatics tools to measure the expression of the VEGF-A gene in glomeruli of type 2 diabetes mellitus patients and found that the expression of this gene was significantly decreased (4). Some studies have shown that this gene is also inhibited in the renal tubules of glomerular interstitial tissue, and the decrease in renal vessels, cortical atrophy, and urinary protein is further aggravated in patients with decreased expression of the VEGFA gene (19,20). Podoplanin (PDPN) can be detected mainly on the glomerular podocyte membrane. The physiological functions of PDPN include the stability of normal cell morphology. The expression of PDPN in the kidney tissue of streptozotocin-induced diabetic mice was found to be severely inhibited during the progression of DN (21). Soluble Flt1 fms-related tyrosine kinase 1 (sFlt1) is an alternatively spliced soluble form of VEGF receptor 1 (VEGFR-1)/Flt-1, and works as a decoy ligand to kinase insert domain receptor (KDR). With the decrease in FLT1 expression, glomerular podocytes change the cytoskeletal structure of glomerular parenchymal cells, destroying the filtration barrier, and then producing a large number of urinary proteins, leading to renal function damage and failure (22). There results showed that there was a positive correlation between the expression of GFR and the expression of vascular endothelial growth factor receptor (VEGFRA) in DN patients, and there was a negative correlation between the expression of GFR and proteinuria. The expression of FIT1 was negatively correlated with proteinuria. The results showed that the expression of VEGFA and FIT1 played an important role in the maintenance and repair of renal function and had a renal protective effect (23). In another study, an Affymetrix microarray was used to analyse the expression of IGF-1 in the kidneys of DN patients. The results showed that the increased expression of IGF-1 in the kidneys of DN patients accompanied the decrease in proteinuria, suggesting that IGF-1 has a protective effect on renal function.
IGFBP-2 expression was detected in glomerular cells, the loop of Henle, and the medullary collecting ducts. This gene could have a role in the growth and maturation of podocytes (24). A study on the IGFBP-2 gene by Narayana et al. showed that the high expression of the IGFBP-2 gene was accompanied by the inhibition of GRF expression and an increase of proteinuria (25). CTGF, a 38-kDa secretory protein that is rich in cysteine, was initially found in the umbilical vein endothelial cells of patients with diabetic complications (26). Further studies showed that protein levels of CTGF were increased in glomeruli, tubules, and interstitial cells of diabetic kidneys and were negatively correlated with the expression of GFR. Previous studies have suggested that WT-1 is very important for the development of the kidneys and is a typical protective factor for renal function (27). When kidney tissue is damaged or renal function is damaged, the expression of BMP-7 is absent. Wang et al.’s research confirmed that the increased expression of BMP-7 can damage renal function in patients with DN (28). At the same time, the microarray analysis indicated that BMP-7 expression was positively correlated with GRF. The main functions of fibroblast growth factor-1 include the regulation of mitosis and insulin sensitization. We also observed a significant reduction in the renal expression of FGF1 in patients with DN by microarray analysis. Wu et al. found that when fibroblast growth factor-1 expression increased, pathological phenomena such as the overexpression of alpha-SMA and the accumulation of collagen were reversed, improving renal function (29). Therefore, fibroblast growth factor-1 also potentially has a protective effect on renal function.
Thus far, few if any studies have mentioned the interaction of GJA-1, BMP-2, TGFBR-3, and FGF-9 hub genes. In fact, the change in BMP-2 expression in the kidney tissue of DN patients is significantly and negatively correlated with the expression of GFR. Some scholars have reported that a BMP-2 binding site (30) could be detected in renal tubular epithelial tissue. Presently, there is abundant evidence that BMP-2 expression is closely related to renal lesions and that BMP-2 expression is negatively correlated with GFR in DN patients. Fibroblast growth factor-9 and BMP-7 can be found in nasopharyngeal carcinoma tissues (31). The expression of fibroblast growth factor-9 was negatively correlated with GFR in the kidney tissue samples of DN patients. Gap junction protein-alpha 1 (GJA-1) is one of components in cell junction structure. Some molecular biology studies have found that these proteins play an important role in the transport of small molecules between cells (32). There was a positive correlation between GJA-1 and proteinuria in DN patients. We speculate that GJA-1, BMP-2, and FGF-9 are closely related to the progression of renal injury in DN patients. In some studies, TGFBR-3 had effects on both tumorigenesis and tumor suppression (33). The results showed that TGFBR-3 in the renal tissue of DN patients was positively correlated with GFR and was negatively correlated with proteinuria. Therefore, TGFBR-3 may also be an effective protective factor for renal function.
Conclusions
A total of 110 DEGs and 14 hub genes were identified as biomarkers for the clinical diagnosis of DN and as potential targets for new therapies. However, the specific molecular mechanism and biological functions of these genes still require further study.
Acknowledgments
Funding: None.
Footnote
Reporting Checklist: The authors have completed the MDAR reporting checklist. Available at http://dx.doi.org/10.21037/atm-20-5647
Conflicts of Interest: All authors have completed the ICMJE uniform disclosure form (available at http://dx.doi.org/10.21037/atm-20-5647). The authors have no conflicts of interest to declare.
Ethical Statement: The authors are accountable for all aspects of the work in ensuring that questions related to the accuracy or integrity of any part of the work are appropriately investigated and resolved. The study was conducted in accordance with the Declaration of Helsinki (as revised in 2013).
Open Access Statement: This is an Open Access article distributed in accordance with the Creative Commons Attribution-NonCommercial-NoDerivs 4.0 International License (CC BY-NC-ND 4.0), which permits the non-commercial replication and distribution of the article with the strict proviso that no changes or edits are made and the original work is properly cited (including links to both the formal publication through the relevant DOI and the license). See: https://creativecommons.org/licenses/by-nc-nd/4.0/.
References
- Thomas B. The Global Burden of Diabetic Kidney Disease: Time Trends and Gender Gaps. Curr Diab Rep 2019;19:18. [Crossref] [PubMed]
- Fei X, Xing M, Wo M, et al. Thyroid stimulating hormone and free triiodothyronine are valuable predictors for diabetic nephropathy in patient with type 2 diabetes mellitus. Ann Transl Med 2018;6:305. [Crossref] [PubMed]
- Mafi A, Namazi G, Soleimani A, et al. Metabolic and genetic response to probiotics supplementation in patients with diabetic nephropathy: a randomized, double-blind, placebo-controlled trial. Food Funct 2018;9:4763-70. [Crossref] [PubMed]
- Baelde HJ, Eikmans M, Doran PP, et al. Gene expression profiling in glomeruli from human kidneys with diabetic nephropathy. Am J Kidney Dis 2004;43:636-50. [Crossref] [PubMed]
- Woroniecka KI, Park AS, Mohtat D, et al. Transcriptome analysis of human diabetic kidney disease. Diabetes 2011;60:2354-69. [Crossref] [PubMed]
- Davis S, Meltzer PS. GEOquery: a bridge between the Gene Expression Omnibus (GEO) and BioConductor. Bioinformatics 2007;23:1846-7. [Crossref] [PubMed]
- Ashburner M, Ball CA, Blake JA, et al. Gene ontology: tool for the unification of biology. The Gene Ontology Consortium. Nat Genet 2000;25:25-9. [Crossref] [PubMed]
- Altermann E, Klaenhammer TR. PathwayVoyager: pathway mapping using the Kyoto Encyclopedia of Genes and Genomes (KEGG) database. BMC Genomics 2005;6:60. [Crossref] [PubMed]
- Huang da W. Sherman BT, Lempicki RA. Systematic and integrative analysis of large gene lists using DAVID bioinformatics resources. Nat Protoc 2009;4:44-57. [Crossref] [PubMed]
- Szklarczyk D, Franceschini A, Kuhn M, et al. The STRING database in 2011: functional interaction networks of proteins, globally integrated and scored. Nucleic Acids Res 2011;39:D561-8. [Crossref] [PubMed]
- Shannon P, Markiel A, Ozier O, et al. Cytoscape: a software environment for integrated models of biomolecular interaction networks. Genome Res 2003;13:2498-504. [Crossref] [PubMed]
- Yang S, Kim CY, Hwang S, et al. COEXPEDIA: exploring biomedical hypotheses via co-expressions associated with medical subject headings (MeSH). Nucleic Acids Res 2017;45:D389-96. [Crossref] [PubMed]
- Lou J, Jing L, Yang H, et al. Risk factors for diabetic nephropathy complications in community patients with type 2 diabetes mellitus in Shanghai: Logistic regression and classification tree model analysis. Int J Health Plann Manage 2019;34:1013-24. [Crossref] [PubMed]
- Soler MJ, Batlle D. Single-cell RNA profiling of glomerular cells in diabetic kidney: a step forward for understanding diabetic nephropathy. Ann Transl Med 2019;7:S340. [Crossref] [PubMed]
- Kanwar YS, Sun L, Xie P, et al. A glimpse of various pathogenetic mechanisms of diabetic nephropathy. Annu Rev Pathol 2011;6:395-423. [Crossref] [PubMed]
- Musante L, Tataruch DE, Holthofer H. Use and isolation of urinary exosomes as biomarkers for diabetic nephropathy. Front Endocrinol (Lausanne) 2014;5:149. [Crossref] [PubMed]
- Arques S, Ambrosi P. Human serum albumin in the clinical syndrome of heart failure. J Card Fail 2011;17:451-8. [Crossref] [PubMed]
- Zhao XM, Zhang Y, He XH, et al. Chinese herbal medicine Shenzhuo Formula treatment in patients with macroalbuminuria secondary to diabetic kidney disease: study protocol for a randomized controlled trial. Trials 2018;19:200. [Crossref] [PubMed]
- Bortoloso E, Del Prete D, Dalla Vestra M, et al. Quantitave and qualitative changes in vascular endothelial growth factor gene expression in glomeruli of patients with type 2 diabetes. Eur J Endocrinol 2004;150:799-807. [Crossref] [PubMed]
- Lindenmeyer MT, Kretzler M, Boucherot A, et al. Interstitial vascular rarefaction and reduced VEGF-A expression in human diabetic nephropathy. J Am Soc Nephrol 2007;18:1765-76. [Crossref] [PubMed]
- Yu J, Zong GN, Wu H, et al. Podoplanin mediates the renoprotective effect of berberine on diabetic kidney disease in mice. Acta Pharmacol Sin 2019;40:1544-54. [Crossref] [PubMed]
- Maezawa Y, Takemoto M, Yokote K. Cell biology of diabetic nephropathy: Roles of endothelial cells, tubulointerstitial cells and podocytes. J Diabetes Investig 2015;6:3-15. [Crossref] [PubMed]
- Yang S, Deng H, Zhang Q, et al. Amelioration of Diabetic Mouse Nephropathy by Catalpol Correlates with Down-Regulation of Grb10 Expression and Activation of Insulin-Like Growth Factor 1 / Insulin-Like Growth Factor 1 Receptor Signaling. PLoS One 2016;11:e0151857. [Crossref] [PubMed]
- Fujinaka H, Katsuyama K, Yamamoto K, et al. Expression and localization of insulin-like growth factor binding proteins in normal and proteinuric kidney glomeruli. Nephrology (Carlton) 2010;15:700-9. [Crossref] [PubMed]
- Narayanan RP, Fu B, Heald AH, et al. IGFBP2 is a biomarker for predicting longitudinal deterioration in renal function in type 2 diabetes. Endocr Connect 2012;1:95-102. [Crossref] [PubMed]
- Guha M, Xu ZG, Tung D, et al. Specific down-regulation of connective tissue growth factor attenuates progression of nephropathy in mouse models of type 1 and type 2 diabetes. FASEB J 2007;21:3355-68. [Crossref] [PubMed]
- Wan J, Hou X, Zhou Z, et al. WT1 ameliorates podocyte injury via repression of EZH2/beta-catenin pathway in diabetic nephropathy. Free Radic Biol Med 2017;108:280-99. [Crossref] [PubMed]
- Wang S, Chen Q, Simon TC, et al. Bone morphogenic protein-7 (BMP-7), a novel therapy for diabetic nephropathy. Kidney Int 2003;63:2037-49. [Crossref] [PubMed]
- Wu Y, Li Y, Jiang T, et al. Reduction of cellular stress is essential for Fibroblast growth factor 1 treatment for diabetic nephropathy. J Cell Mol Med 2018;22:6294-303. [Crossref] [PubMed]
- Yang YL, Liu YS, Chuang LY, et al. Bone morphogenetic protein-2 antagonizes renal interstitial fibrosis by promoting catabolism of type I transforming growth factor-beta receptors. Endocrinology 2009;150:727-40. [Crossref] [PubMed]
- Muthukrishnan SD, Yang X, Friesel R, et al. Concurrent BMP7 and FGF9 signalling governs AP-1 function to promote self-renewal of nephron progenitor cells. Nat Commun 2015;6:10027. [Crossref] [PubMed]
- Choi J, Yang A, Song A, et al. Oculodentodigital Dysplasia with a Novel Mutation in GJA1 Diagnosed by Targeted Gene Panel Sequencing: A Case Report and Literature Review. Ann Clin Lab Sci 2018;48:776-81. [PubMed]
- Bernabeu C, Lopez-Novoa JM, Quintanilla M. The emerging role of TGF-beta superfamily coreceptors in cancer. Biochim Biophys Acta 2009;1792:954-73. [Crossref] [PubMed]
(English Language Editor: J. Gray)