Development and validation of a prognostic and immunotherapeutically relevant model in hepatocellular carcinoma
Introduction
Liver cancer is the fourth leading cause of cancer-associated mortality globally (1,2). Hepatocellular carcinoma (HCC), the most prevalent subtype of liver cancer, is an aggressive chronic malignancy. The prognosis of HCC is poor, which is mainly attributable to metastasis-related recurrence. Most patients with HCC are at an advanced stage at the time of diagnosis and consequently respond poorly to surgical resection or chemotherapy (3). In recent years, immunotherapy has been used in the treatment of HCC, achieving certain therapeutic effects (4). However, the survival benefits of immunotherapy vary widely among HCC patients. Personalized treatment strategies can help to prolong the survival and improve the quality of life of HCC patients. Therefore, an effective model that can accurately predict prognosis and immunotherapeutic response for HCC patients is urgently needed.
A growing amount of evidence has indicated that the tumor immune microenvironment plays a pivotal role in the progression and clinical outcomes of HCC (5,6). For instance, differences in the composition of immune cells, including T cells, macrophages, dendritic cells, and cancer-associated fibroblasts, have been reported to influence the prognosis of HCC in different ways (7). Immune checkpoint-based immunotherapies have emerged as potentially effective treatments for patients with advanced HCC. Immune checkpoint inhibitors, such as the anti-cytotoxic T-lymphocyte-associated antigen 4 (anti-CTLA-4) agent tremelimumab and the anti-programmed cell death protein 1 (anti-PD-1) agent nivolumab, have been used to treat HCC in clinical trials (8). Although immunotherapy has shown promise for some HCC patients, especially as second-line therapy, others have not benefited from this treatment. Recent studies have suggested a close relationship between immune-related genes and prognosis in HCC patients who receive immunotherapy (9-11). However, a model that can predict prognosis and immunotherapeutic response in HCC patients based on immune-related genes has yet to be designed.
In this study, we analyzed immune-related genes and constructed an immune prediction model (IPM) using HCC transcriptome data from The Cancer Genome Atlas (TCGA) database. The prognostic prediction value of our IPM was validated using the GSE14520 dataset from the Gene Expression Omnibus (GEO) database. Further, multivariate Cox regression analysis was carried out to confirm the independent prognostic role of the IPM. A nomogram was also established to predict the prognosis of patients with HCC based on independent clinical risk factors. Gene set enrichment analysis (GSEA) was performed to explore the potential mechanisms. Finally, the model’s potential in guiding the treatment of HCC patients was also evaluated. Overall, our IPM could be used to guide therapeutic strategy in the management of HCC patients, and the genes in the IPM could serve as potential biomarkers for HCC. We present the following article in accordance with the TRIPOD reporting checklist (available at http://dx.doi.org/10.21037/atm-20-6112).
Methods
Data preparation
The mRNA expression data and clinical information of patients with HCC were downloaded from the TCGA database and used to construct the IPM for HCC. The GSE14520 dataset was also downloaded from the GEO database and used to validate the IPM. The alteration status of immune-related genes in HCC was obtained from the cBioPortal for Cancer Genomics database. All data were publicly available and downloaded from online databases; therefore, this study did not require additional ethical approval. The study was conducted in accordance with the Declaration of Helsinki (as revised in 2013).
Identification of differentially expressed immune genes in HCC
A total of 1,534 immune-related genes were downloaded from the ImmPort database (https://immport.niaid.nih.gov), accessed on October 26, 2019 (12). To identify differentially expressed immune genes (DEIGs) in HCC tissues and normal tissues, the raw count data were extracted and analyzed using the R package “edgeR”. DEIGs meeting the criteria of Log2 fold change (FC) >2 and an adjusted P value <0.05 were considered for subsequent analysis.
Development of IPM for HCC prognosis prediction
Patients with a follow-up time of longer than 1 month were included for survival analysis. Univariate Cox regression analysis was carried out to screen prognostic genes of HCC among the DEIGs with the criterion P<0.05. Multivariate Cox regression analysis was performed by using R package “survival” to construct the IPM for HCC based on the prognostic genes. The risk score of IPM =(βmRNA1 * expression level of mRNA1) + (βmRNA2 * expression level of mRNA2) + (βmRNA3 * expression level of mRNA3) + … + (βmRNAn * expression level of mRNAn). According to the risk score, patients were classified into the high- and low-risk groups. The survival difference between the high- and low-risk groups was evaluated through Kaplan-Meier survival analysis combined with the log-rank test using the “survival” package in R. The predictive potential of the IPM for overall survival (OS) was assessed by time-dependent receiver operating characteristic (ROC) curve analysis using the R package “survivalROC”. Further, the prognostic function of the IPM was validated in the GSE14520 dataset. Risk score was calculated with the same IPM formula from the TCGA dataset. Finally, the Kaplan-Meier method and ROC curves were used to evaluate and validate the predictive value of the IPM in the GSE14520 dataset.
Independent prognostic role of the IPM from traditional clinical factors
The TCGA dataset included HCC patient clinical information including age, gender, tumor grade, tumor stage, and pathological T, pathological_M, and pathological_N. The GSE14520 dataset included HCC patient clinical information including age, gender, alanine aminotransferase (ALT), tumor size, multinodular, liver cirrhosis, alpha fetoprotein (AFP), and TNM stage. To validate whether the IPM was independent of other clinical factors for patients with HCC, univariate and multivariate Cox regression analyses with a forward stepwise procedure were performed.
Construction and evaluation of the nomogram
A nomogram that integrated all of the independent clinical factors identified by multivariate Cox analysis was constructed using the R package “rms”. Discrimination and calibration are the most commonly used methods for evaluating the performance of a nomogram. To assess the discrimination ability of the nomogram, the concordance index (C-index) was calculated by a bootstrap method with 1,000 resamples. The calibration curve was applied to evaluate the similarity between the prediction probabilities and the observed rates. Additionally, ROC curve analysis was performed to compare the nomogram and individual clinical prognostic factors.
Gene set enrichment analysis
To explore the molecular mechanisms potentially underlying our constructed immune signature, GSEA was performed of the Gene Ontology (GO) term in C5, the Kyoto Encyclopedia of Genes and Genomes (KEGG) pathway in C2, the oncogenic signatures of gene sets in C6, and the immunologic signatures of gene sets in C7, respectively (13,14). Gene sets from the Molecular Signatures Database (MSigDB) were used for reference. The number of permutations was set at 1,000. Enrichment results satisfying a nominal P value <0.05 and a false discovery rate (FDR) <0.25 were considered to be statistically significant.
Tumor-infiltrating immune cell analysis
CIBERSORT is an analytical tool that characterizes the cell composition of complex tissues based on their gene expression profiles (15). LM22 is a signature matrix consisting of 547 genes that accurately distinguish immune cell types, including T cell types, naïve and memory B cells, plasma cells, NK cells, and myeloid subsets. To determine immune cell infiltration in the high- and low-risk patient groups, CIBERSORT was used in combination with the LM22 signature matrix to estimate the proportions of immune cell types. For each sample, the sum of all estimated proportions is equal to 1. Cytolytic activity was calculated as the geometric mean of PRF1 and GZMA (16).
Statistical analysis
Statistical analysis was performed using R software v3.5.0 (R Foundation for Statistical Computing, Vienna, Austria) and GraphPad Prism v7.00 (GraphPad Software Inc., USA). Survival analysis was performed using the Kaplan-Meier method and the log-rank test in R package “survival”. A P value <0.05 was considered statistically significant.
Results
Identification of DEIGs
A total of 1,534 immune-related genes were downloaded from the ImmPort database. The expression values of these genes were extracted from the TCGA dataset and analyzed using “edgeR”. Of the 1,534 immune-related genes, 294 were identified as DEIGs between HCC tissues and normal tissues (|log2FC| >1 and P<0.05). A heatmap of the DEIGs is shown in Figure S1. Of these 294 DEIGs, 194 genes were found to be significantly upregulated in HCC compared to normal tissues, and 100 genes were significantly downregulated in HCC relative to normal tissues.
Development of an IPM based on the TCGA dataset
The 294 DEIGs were used to construct the IPM for HCC based on the TCGA dataset. First, 80 of the 294 DEIGs were identified as being significantly associated with OS by univariate Cox regression analysis (P<0.05). Then, multivariate Cox regression analysis was performed to determine the genes with the greatest prognostic value. Eight genes with relative regression coefficients were identified and used to construct the IPM. The eight genes identified were matrix metalloproteinase-12 (MMP12), tyrosine kinase 2 (TYK2), heme oxygenase 1 (HMOX1), chemokine receptor type 6 (CXCR6), hepatoma-derived growth factor (HDGF), placental growth factor (PGF), retinoid X receptor beta (RXRB), and cyclin-dependent kinase 4 (CDK4). Survival risk score was calculated as follows: risk score = (0.0864* expression of MMP12) + (−0.3658* expression of TYK2) + (0.2206* expression of HMOX1) + (−0.3100* expression of CXCR6) + (0.5089* expression of HDGF) + (0.1430* expression of PGF) + (−0.5244* expression of RXRB) + (0.3323* expression of CDK4). Next, the risk score was calculated for each HCC patient and the patients were ranked (Figure 1A). As shown in the heatmap in Figure 1A, patients in the high-risk group tended to have increased levels of MMP12, HMOX1, CXCR6, HDGF, PGF, and CDK4 expression, as well as reduced TYK2 and RXRB expression. The Kaplan-Meier curve analysis and log-rank test suggested that the high-risk patients had significantly worse OS than patients in the low-risk group (P<0.001, Figure 1B). Figure 1C shows the predictive potential of the IPM using time-dependent ROC curves. The area under the ROC curve (AUC) of the prognostic model for HCC was 0.768, 0.739, and 0.757 at 1, 3, and 5 years, respectively.
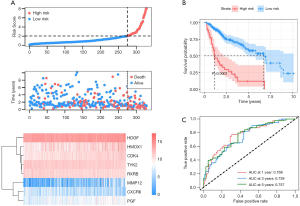
Validation and evaluation of the IPM in the GEO dataset
To validate whether the IPM had similar prognostic value among different HCC populations, we calculated the risk score for each patient in the GSE14520 dataset using the formula described above. The patients were divided into high- and low-risk groups with a risk score of 1.455 as the optimal cutoff point. Figure 2A shows the distribution of risk scores and survival times with the IPM, along with the gene expression data, in the GSE14520 dataset. Consistent with our previous findings, the Kaplan-Meier curve suggested that patients in the high-risk group showed significantly worse OS than patients in the low-risk group (P<0.001, Figure 2B). The AUCs for 1-, 3-, and 5-year OS were 0.669, 0.703, and 0.701, respectively (Figure 2C). Furthermore, the percentage of alteration of the eight genes in HCC patients was investigated using the cBioPortal website (Figure S2). The alteration percentages varied from 0.6–10% (HDGF, 10%; RXRB, 3%; and CDK4, 1.1%; TYK2, 0.8%; CXCR6, 0.6%; HMOX1, 0.6%; MMP12, 0.6%; and PGF, 0.6%). Overall, these results suggested that our IPM had a good performance in predicting the survival of patients with HCC.
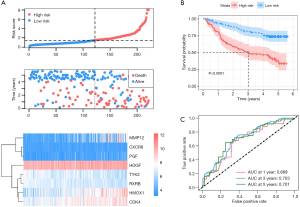
Independent prognostic ability of the IPM
Univariate and multivariate Cox regression analyses were performed to determine whether the IPM was independent of other clinical parameters. In the TCGA dataset, univariate Cox analysis showed that TNM stage, pathological_T, pathological_M, and risk score were significantly associated with OS. Furthermore, the results of multivariate Cox analysis showed that pathological_T, pathological_N, and risk score were independent prognostic factors for OS (Table 1). The same result was observed in the GSE14520 dataset after adjusting for other clinical features, including age, gender, ALT, tumor size, multinodular, cirrhosis, AFP, TNM stage, and risk score. Univariate and multivariate Cox regression analyses indicated that TNM stage and risk score were independent prognostic factors for OS (Table 1). Therefore, the IPM had independent prognostic significance for OS in HCC patients.
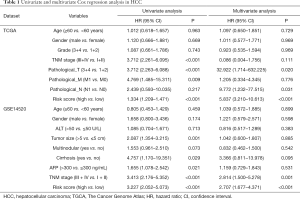
Full table
Construction and validation of a nomogram based on the IPM
The prognostic nomogram was developed by integrating the IPM and independent clinical risk factors from the TCGA and GSE14520 datasets, respectively. In the TCGA dataset, the risk score was found to contribute more risk points (ranging from 0 to 100) than the other clinical factors, which was consistent with the results of our Cox multivariate regression analysis (Figure 3A). The calibration plot showed good performance between the outcomes predicted by the nomogram and actual observations (Figure 3B). The C-index for the nomogram was 0.696 with 1,000 bootstrap replicates (95% CI: 0.624–0.769). The AUCs for 1-, 3-, and 5-year OS were: 0.743, 0.703, and 0.820, respectively, for the TNM model; 0.678, 0.693, and 0.771, respectively, for the IPM; and 0.677, 0.692, and 0.783, respectively, for the combined model (Figure 3C). Furthermore, we applied the same method to build a nomogram based on the IPM and TNM stage in the GSE14520 dataset (Figure 3D,E,F). As expected, compared with the TNM model and the IPM, the combined model had the largest AUCs for 1-, 3-, and 5-year OS. In summary, these findings demonstrate that our nomogram could be used to predict OS in HCC patients more accurately than individual prognostic factors.
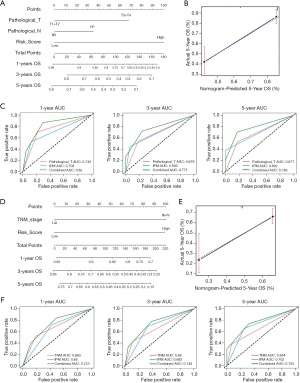
Gene set enrichment analysis
To explore the underlying mechanisms of the genes used to construct our IPM in HCC, we applied the GSEA method to investigate the potential biological processes and pathways in the high- and low-risk groups. GO enrichment analysis identified 142 enriched GO terms, including cell cycle G2 M phase transition, mitotic cell cycle checkpoint, and regulation of chromosome segregation, in the high-risk group (Figure 4A). The enrichment of c2 suggested that 3 KEGG pathways (regulation of fatty acid metabolism, the peroxisome proliferator-activated receptor (PPAR) signaling pathway, and peroxisome) were enriched in the low-risk group, and that 5 KEGG pathways (cell cycle, Fc gamma receptor mediated phagocytosis, DNA replication) were enriched in the high-risk group (Figure 4B). Furthermore, 20 oncological signatures [including E2F transcription factor 1 (E2F1), myelocytomatosis oncogene (MYC), and vascular endothelial growth factor (VEGF)] were significantly enriched in the high-risk group, while no oncological signatures were significantly enriched in the low-risk group (Figure 4C). In terms of immunologic signatures, B cells, CD4+ T cells, NKT cells, and PD-1 ligation were the most enriched pathways in the high-risk group (Figure 4D).
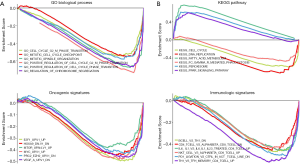
Correlation of clinicopathological characteristics with the IPM
The relationship between the clinicopathological characteristics of HCC patients and the IPM was analyzed in the TCGA and GSE14520 datasets. As shown in Figure 5A-P, risk score was negatively associated with survival time. In terms of AJCC-TNM stage, in the TCGA dataset, stage III and IV patients had higher risk scores than stage II and I patients, which was consistent with our findings from the GSE14520 dataset. Similarly, in terms of T stage, grade, Barcelona Clinic Liver Cancer (BCLC) classification, and AFP, patients with advanced HCC had a higher risk score than early-stage patients. Further, to explore the clinical significance of the eight genes described above, we analyzed the correlation between their expression levels and tumor stage. The results showed that the mRNA expression levels of MMP12, PGF, and CDK4 were significantly increased in advanced HCC patients. In contrast, CXCR6 expression in these patients was significantly decreased compared to that in early-stage patients.
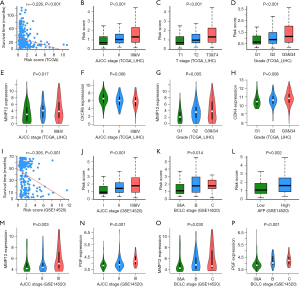
Correlation of tumor-infiltrating immune cells with the IPM
To determine the possible differences of tumor-infiltrating immune cells in the HCC microenvironment, we applied the CIBERSORT algorithm to estimate the relative proportions of immune cells between low- and high-risk patients. The association between risk score and immune cells was analyzed by Pearson’s correlation coefficient. As shown in Figure 6A-F, naïve B cells, activated dendritic cells, regulatory T cells (Tregs), M2 macrophages, activated NK cells, and CD8+ T cells were significantly negatively correlated with risk score. In contrast, monocytes, M0 macrophages, and neutrophils were significantly positively correlated with risk score (Figure 6G-I). However, no significant correlations were observed between resting mast cells, T follicular helper cells, or activated memory CD4 T cells (Figures 6J-L). Moreover, the proportions of different subpopulations of tumor-infiltrating immune cells were weakly to moderately correlated (Figure S3). Therefore, variation in the proportions of tumor-infiltrating immune cells might represent an intrinsic heterogeneity that could characterize differences between individual patients.
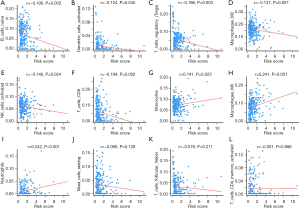
The IPM could identify the HCC patients most likely to benefit from immunotherapy
Immune checkpoints have emerged as important therapeutic targets in cancer immunotherapy. Therefore, we investigated the expression levels of PDCD1 (encoded protein PD-1), CD274 (encoded protein PD-L1), and CTLA4 (encoded protein CTLA-4) in the high- and low-risk groups. As shown in Figure 7A-L, in the high-risk group, the expression levels of PDCD1 and CTLA-4 were significantly increased while CD274 expression was significantly decreased compared to the low-risk group. Furthermore, the expression of PDCD1, CD274, and CTLA-4 was significantly positively correlated with CD8+ T cells, but negatively correlated with NK cells. No significant correlation existed between the expression of CD274 and NK cells. Cytolytic activity, which is associated with clinical responses to anti-CTLA-4 and anti-PD-L1 immunotherapies, was calculated. The results showed a significant positive correlation with PDCD1, CD274, and CTLA-4 expression, suggesting a potentially enhanced effect of PD-1, PD-L1, and CTLA-4 antibodies in HCC patients with a high-risk score.
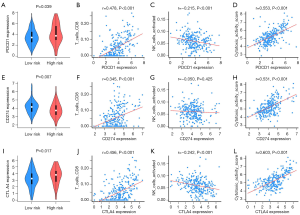
Discussion
In this study, we constructed and validated an IPM based on eight genes (MMP12, TYK2, HMOX1, CXCR6, HDGF, PGF, RXRB, and CDK4), which was able to predict the prognosis and immunotherapeutic response of HCC patients. MMP12, HMOX1, HDGF, PGF, and CDK4 were found to be negative prognostic genes, while TYK2, CXCR6, and RXRB were found to be positive prognostic genes. Our IPM had independent prognostic significance for HCC, with patients in the high-risk group showing significantly poorer survival than those in the low-risk group. Calibration and ROC curve analysis demonstrated that the nomogram that integrated the IPM with AJCC-TNM stage gave the best performance in predicting the survival of HCC patients. Furthermore, the correlation of the IPM with tumor-infiltrating immune cells revealed that T cells and macrophages played a crucial role in the IPM. The patients with a high-risk score had increased expression levels of PD-L1 and CTLA-4, indicating a potentially high response rate to immunotherapy. Lastly, GSEA revealed several significantly enriched oncological signatures and various immunologic signatures, which might help to explain the underlying molecular mechanisms of the genes used to construct the IPM.
Most of the eight genes in our IPM have been reported to be involved in cancer progression. Matrix metalloproteinases (MMPs) are a large family of zinc-dependent proteases that have been demonstrated to be implicated in cancer progression and metastasis by degradation of matrix proteins (17). Recent research has shown that overexpression of MMP-12 is correlated with poor prognosis in HCC (18,19). Nevertheless, the role and mechanism of MMP-12 in HCC remain unclear. TYK2, a member of the Janus kinases (JAKs) protein family, plays a key role in immune responses and inflammation through cytokine receptors (20). In this study, we found that the expression of TYK2 was associated with OS. However, to date, the role of TYK2 in HCC has not been illuminated. HMOX1, a core enzyme in heme catabolism, is associated with tumor growth and metastasis (21). Dysregulated expression of CXC chemokines and CXC chemokine receptors can serve as a useful biomarker in various cancers. In particular, CXCR6, which was found to be overexpressed in HCC, is associated with invasive growth, inflammatory recruitment, and angiogenic activities in HCC (22,23). Recent studies have suggested that HDGF may be an independent prognostic factor for predicting the disease-free survival and OS of patients with various cancers, including HCC. Furthermore, the proliferation of HCC cells is promoted by HDGF overexpression, but inhibited by reduced HDGF expression (24). PGF, a member of the VEGF family, is closely associated with tumor angiogenesis. Cell cycle dysregulation is a hallmark of cancer that results in the uncontrolled proliferation of cancer cells. CDK4 is involved cell cycle regulation, and is frequently overexpressed or mutated in cancer (25). Recently, a study reported that high expression of CDK4 was a significant prognostic factor for OS of HCC (26).
Immune cells are key participants of the tumor immune microenvironment, and can promote or inhibit tumor formation and development. To explore the association of immune cells with our IPM, the relative proportions of immune cells were investigated. The risk score was significantly negatively correlated with CD8+ T cells, NK cells, Tregs, dendritic cells, and M2 macrophages. The HCC patients with a low risk score had high proportions of dendritic cells and CD8+ T cells, which cooperate to achieve an antitumor effect. Moreover, we found that high-risk HCC patients generally had higher proportions of M0 macrophages and neutrophils than low-risk patients. M0 macrophages are well known to contribute to tumor progression at different levels, including by promoting genetic instability, paving the way to metastasis, and taming protective adaptive immunity (27). Recent research has shown that neutrophils recruit M0 macrophages and Tregs to promote HCC progression (28,29). All of these results suggest that these differences in tumor-infiltrating immune cells promote HCC growth, progression, invasion, and angiogenesis, resulting in poor prognosis of high-risk patients.
Immune checkpoint-based immunotherapies that target the PD-1 or CTLA-4 pathways have achieved some success in the treatment of liver cancers, including HCC and cholangiocarcinoma (30). However, only a proportion of patients benefit from immunotherapy. Thus, a deeper understanding of the mechanisms underlying the varied therapeutic responses to immunotherapy is critical to improving individual diagnoses and precision medicine. PD-1 is a potent inhibitor of T-cell immune response, and is expressed by activated CD8+ T cells and NK cells (4). The binding of PD-L1 to PD-1 receptors blocks T-cell antigen receptor (TCR) signaling and inhibits T cells activation. Importantly, PD-L1 expression is an important biomarker that can predict the response to anti-PD-1/PD-L1 therapies (31). CTLA-4 is critical for the control of CD4+ T cell function and is primarily involved in the priming phase of the immune response (4). CTLA-4 further promotes immunosuppression by inducing Treg activity and differentiation (32). In our analysis, the expression levels of PD-1, PD-L1, and CTLA-4 were significantly increased in the high-risk group. Furthermore, the expression levels of PD-1, PD-L1, and CTLA-4 were significantly correlated with CD8+ T cells, NK cells, and cytolytic activity, which provides a potential basis for PD-1/PD-L1, and CTLA-4 treatment. Similarly, the IPM we constructed also indicated that high expression levels of PD-1, PD-L1, and CTLA-4 were correlated with a high-risk score. Therefore, patients with a high-risk score could derive more benefit from immunotherapy than patients with a low risk score.
However, our study had some limitations that need to be recognized. First, the sample size in our study was relatively small, and research on larger sample sizes is needed to increase the reliability of our findings. Second, the IPM and prognostic nomogram need to be validated with more independent datasets. Third, functional experiments are needed to clarify the underlying mechanism of the IPM. Therefore, future research is still needed to address these issues. Because of the small sample size and incomplete clinical information in the current study, further well-designed studies with larger sample sizes are necessary to validate our results.
Conclusions
In this study, we developed and validated an IPM to help predict OS and immunotherapeutic response in patients with HCC. Our IPM may help to guide clinical decision-making for HCC patients.
Acknowledgments
Funding: None.
Footnote
Reporting Checklist: The authors have completed the TRIPOD reporting checklist. Available at http://dx.doi.org/10.21037/atm-20-6112
Conflicts of Interest: All authors have completed the ICMJE uniform disclosure form (available at http://dx.doi.org/10.21037/atm-20-6112). The authors have no conflicts of interest to declare.
Ethical Statement: The authors are accountable for all aspects of the work in ensuring that questions related to the accuracy or integrity of any part of the work are appropriately investigated and resolved. All data were publicly available and downloaded from online databases; therefore, this study did not require additional ethical approval. The study was conducted in accordance with the Declaration of Helsinki (as revised in 2013).
Open Access Statement: This is an Open Access article distributed in accordance with the Creative Commons Attribution-NonCommercial-NoDerivs 4.0 International License (CC BY-NC-ND 4.0), which permits the non-commercial replication and distribution of the article with the strict proviso that no changes or edits are made and the original work is properly cited (including links to both the formal publication through the relevant DOI and the license). See: https://creativecommons.org/licenses/by-nc-nd/4.0/.
References
- Bray F, Ferlay J, Soerjomataram I, et al. Global cancer statistics 2018: GLOBOCAN esti-mates of incidence and mortality worldwide for 36 cancers in 185 countries. CA Cancer J Clin 2018;68:394-424. [Crossref] [PubMed]
- Yang JD, Hainaut P, Gores GJ, et al. A global view of hepatocellular carcinoma: trends, risk, prevention and management. Nat Rev Gastroenterol Hepatol 2019. [Crossref] [PubMed]
- Chawla A, Ferrone C. Hepatocellular carcinoma surgical therapy: perspectives on the current limits to resection. Chin Clin Oncol 2018;7:48. [Crossref] [PubMed]
- Prieto J, Melero I, Sangro B. Immunological landscape and immunotherapy of hepato-cellular carcinoma. Nat Rev Gastroenterol Hepatol 2015;12:681-700. [Crossref] [PubMed]
- Kurebayashi Y, Ojima H, Tsujikawa H, et al. Landscape of immune microenvironment in hepatocellular carcinoma and its additional impact on histological and molecular clas-sification. Hepatology 2018;68:1025-41. [Crossref] [PubMed]
- Nishida N, Kudo M. Immunological Microenvironment of Hepatocellular Carcinoma and Its Clinical Implication. Oncology 2017;92 Suppl 1:40-9. [Crossref] [PubMed]
- Tang X, Shu Z, Zhang W, et al. Clinical significance of the immune cell landscape in hepatocellular carcinoma patients with different degrees of fibrosis. Ann Transl Med 2019;7:528. [Crossref] [PubMed]
- Hato T, Goyal L, Greten TF, et al. Immune checkpoint blockade in hepatocellular carci-noma: current progress and future directions. Hepatology 2014;60:1776-82. [Crossref] [PubMed]
- Carone C, Olivani A, Dalla Valle R, et al. Immune Gene Expression Profile in Hepato-cellular Carcinoma and Surrounding Tissue Predicts Time to Tumor Recurrence. Liver Cancer 2018;7:277-94. [Crossref] [PubMed]
- Wang Z, Zhu J, Liu Y, et al. Development and validation of a novel immune-related prognostic model in hepatocellular carcinoma. J Transl Med 2020;18:67. [Crossref] [PubMed]
- Siu EH, Chan AW, Chong CC, et al. Treatment of advanced hepatocellular carcinoma: immunotherapy from checkpoint blockade to potential of cellular treatment. Transl Gastroenterol Hepatol 2018;3:89. [Crossref] [PubMed]
- Bhattacharya S, Dunn P, Thomas CG, et al. ImmPort, toward repurposing of open access immunological assay data for translational and clinical research. Sci Data 2018;5:180015. [PubMed]
- Subramanian A, Tamayo P, Mootha VK, et al. Gene set enrichment analysis: a knowledge-based approach for interpreting genome-wide expression profiles. Proc Natl Acad Sci U S A 2005;102:15545-50. [PubMed]
- Liberzon A, Subramanian A, Pinchback R, et al. Molecular signatures database (MSigDB) 3.0. Bioinformatics 2011;27:1739-40. [Crossref] [PubMed]
- Newman AM, Liu CL, Green MR, et al. Robust enumeration of cell subsets from tissue expression profiles. Nat Methods 2015;12:453-7. [Crossref] [PubMed]
- Rooney MS, Shukla SA, Wu CJ, et al. Molecular and genetic properties of tumors asso-ciated with local immune cytolytic activity. Cell 2015;160:48-61. [Crossref] [PubMed]
- Kessenbrock K, Plaks V, Werb Z. Matrix metalloproteinases: regulators of the tumor microenvironment. Cell 2010;141:52-67. [Crossref] [PubMed]
- Ng KT, Qi X, Kong KL, et al. Overexpression of matrix metalloproteinase-12 (MMP-12) correlates with poor prognosis of hepatocellular carcinoma. Eur J Cancer 2011;47:2299-305. [Crossref] [PubMed]
- Gao H, Zhou X, Li H, et al. Role of Matrix Metallopeptidase 12 in the Development of Hepatocellular Carcinoma. J Invest Surg 2019.1-7. [Crossref] [PubMed]
- Woss K, Simonovic N, Strobl B, et al. TYK2: An Upstream Kinase of STATs in Cancer. Cancers (Basel) 2019;11:1728. [Crossref] [PubMed]
- Chiang SK, Chen SE, Chang LC. A Dual Role of Heme Oxygenase-1 in Cancer Cells. Int J Mol Sci 2018.20. [Crossref] [PubMed]
- Mossanen JC, Kohlhepp M, Wehr A, et al. CXCR6 Inhibits Hepatocarcinogenesis by Promoting Natural Killer T- and CD4(+) T-Cell-Dependent Control of Senescence. Gastroenterology 2019;156:1877-89.e4. [Crossref] [PubMed]
- Gao Q, Zhao YJ, Wang XY, et al. CXCR6 upregulation contributes to a proinflammatory tumor microenvironment that drives metastasis and poor patient outcomes in hepa-tocellular carcinoma. Cancer Res 2012;72:3546-56. [Crossref] [PubMed]
- Min X, Wen J, Zhao L, et al. Role of hepatoma-derived growth factor in promoting de novo lipogenesis and tumorigenesis in hepatocellular carcinoma. Mol Oncol 2018;12:1480-97. [Crossref] [PubMed]
- Hamilton E, Infante JR. Targeting CDK4/6 in patients with cancer. Cancer Treat Rev 2016;45:129-38. [Crossref] [PubMed]
- Lu JW, Lin YM, Chang JG, et al. Clinical implications of deregulated CDK4 and Cyclin D1 expression in patients with human hepatocellular carcinoma. Med Oncol 2013;30:379. [Crossref] [PubMed]
- Mantovani A, Marchesi F, Malesci A, et al. Tumour-associated macrophages as treat-ment targets in oncology. Nat Rev Clin Oncol 2017;14:399-416. [Crossref] [PubMed]
- Galdiero MR, Bonavita E, Barajon I, et al. Tumor associated macrophages and neutro-phils in cancer. Immunobiology 2013;218:1402-10. [Crossref] [PubMed]
- Zhou SL, Zhou ZJ, Hu ZQ, et al. Tumor-Associated Neutrophils Recruit Macrophages and T-Regulatory Cells to Promote Progression of Hepatocellular Carcinoma and Re-sistance to Sorafenib. Gastroenterology 2016;150:1646-58. e17.
- Pardoll DM. The blockade of immune checkpoints in cancer immunotherapy. Nat Rev Cancer 2012;12:252-64. [Crossref] [PubMed]
- Havel JJ, Chowell D, Chan TA. The evolving landscape of biomarkers for checkpoint inhibitor immunotherapy. Nat Rev Cancer 2019;19:133-50. [Crossref] [PubMed]
- Wei SC, Duffy CR, Allison JP. Fundamental Mechanisms of Immune Checkpoint Blockade Therapy. Cancer Discov 2018;8:1069-86. [Crossref] [PubMed]
(English Language Editor: J. Reynolds)