Screening of serum protein biomarkers in hemorrhagic cerebral infarction by surface-enhanced laser desorption/ionization time-of-flight mass spectrometry (SELDI-TOF-MS) technology
Introduction
Cerebrovascular disease is a major disease that endangers the health and life of middle-aged and elderly people in China. It has become the primary cause of death and disability in China, with cerebral infarction (CI) accounting for the majority cases. CI is also known as ischemic stroke resulting from acute thrombosis or other effects of thrombosis metastasis leading to local cerebral vascular occlusion. Hemorrhagic cerebral infarction (HCI) refers to the hemorrhage in the infarct area that occurs after CI. Compared with CI, HCI has a lower diagnostic rate and a higher mortality rate (1).
Currently, HCI is still diagnosed by imaging methods such as computed tomography (CT) and magnetic resonance imaging (MRI) (2,3). However, CT and MRI are invasive examinations that produce radiation which adversely affects cells in the brain tissue during the diagnosis of CI. Furthermore, CT and MRI equipment is expensive and not available in all hospitals, while the inspection appointment cycle is long. Therefore, finding a safe, effective, and specific method for the diagnosis of early HCI is urgently needed in clinical practice.
In recent years, mass spectrometry has been widely used in clinical disease research. In particular, the applications of the surface-enhanced laser desorption/ionization time-of-flight mass spectrometry (SELDI-TOF-MS) has provided a new research platform for the search of disease protein biomarkers.
The SELDI-TOF-MS technology consists of three parts: proteinchip arrays, a time-of-flight mass spectrometer, and protein biological system analysis software. Proteinchips come in chemical and biological types, according to the different properties of the material located on the surface of the chip. The chemical proteinchip arrays incorporate hydrophobic exchanger (H50), weak cationic exchanger (CM10, WCX2), anionic exchanger (SAX2), metal ion exchanger (IMAC30), or hydrophilic exchanger (NP20) spots which are best suited for protein expression profiling studies. The biological proteinchip arrays have pre-activated ‘‘biological surfaces’’ designed for coupling biomolecules to applications in antibody–antigen assays, receptor–ligand interaction studies, and DNA-protein binding experiments.
The SELDI chip has 8–24 sampling spots. When a sample is added to the chip, some proteins in the samples bind to the modified surface, while others are washed off. Then, an energy-absorbing molecule is applied to the surface for crystallization with the sample peptides. In the binding and washing off steps, the surface-bound proteins are left for analyses. Samples spotted on an SELDI chip surface are typically analyzed with time-of-flight mass spectrometry (TOF-MS). The proteins that are bound to the chip undergo desorption and ionization under a certain intensity of laser bombardment. These ions are then accelerated through an electric field and proceed down into the flight tube. Because these ions have different mass-to-charge ratios, they are not in flight for the same amount of time in a vacuum tube. The detector then measures the ions as they reach the end of the tube. The measured proteins appear as a series of peaks, which are plotted as mass spectrograms (4).
SELDI-TOF-MS technology has been widely used in the research of many diseases, including cancers (5), infectious diseases (6), and neurological diseases (7). For instance, Whelan et al. reviewed the diagnosis and detection of various cancers by SELDI-TOF-MS technology and found that a high sensitivity and specificity could be achieved in distinguishing between cancer patients and controls in 17 cancers. SELDI-TOF-MS also has demonstrated ability to predict the chemosensitivity in cancers therapy by testing plasma from pre- and post-treatment patients (8).
The combination of SELDI-TOF-MS and proteinchip technology can analyze a variety of complex biological samples, such as serum and urine. Additionally, samples can be applied in minuscule amounts, and only 0.5 to 5 microliters are required for each analysis. The detectable molecular weight range is wide, and is particularly suitable for proteins with a molecular weight below 20 kDa. Therefore, it is also suitable for the detection of a low abundance of protein or polypeptide. The analysis of one sample can be done in dozens of minutes, and process far more information than two-dimensional electrophoresis. Finally, the analysis software can quickly detect differential proteins with varied disease-specific changes, which can be used to find one or a group of biomarkers related to the disease and provide the best combination index for the diagnosis of the disease (8). However, a major disadvantage is that the sequence of proteins/peptides concerned cannot be given by SELDI-TOF-MS (9).
In addition to this, SELDI-TOF-MS is also fast and high-throughput-capable, and can simultaneously detect a variety of proteins, which is optimal for screening disease biomarkers. Previous studies on markers of proteins related to HCI have mainly focused on single proteins. However, few studies have attempted to screen serum protein markers for HCI by using mass spectral techniques of proteomic methods. Therefore, the purpose of this study was to analyze the serum protein profile of HCI and controls by using SELDI-TOF-MS technology, so as to screen potential serum protein biomarkers for patients with HCI. We present the following article in accordance with the STARD reporting checklist (available at http://dx.doi.org/10.21037/atm-20-6071).
Methods
Patient characteristics
All procedures performed in this study involving human participants were performed in accordance with the Declaration of Helsinki (as revised in 2013). The research scheme was approved by the ethics committee of Beijing Xiaotangshan Hospital. All subjects signed informed consent before the study. Data from 48 patients with HCI, 53 patients with CI, and 48 healthy controls (HCs) were collected in this study (Table 1). The collection time was from November 2018 to October 2019. All cases were diagnosed according to their clinical symptoms, physical examination, and head CT or MRI scan, which were in accordance with the “Diagnostic criteria of cerebrovascular diseases in China (version 2019)” (10). CI cases caused by tumor, brain trauma, cardiac disease, and blood disease were excluded. The HCs group consisted of individuals who visited our hospital for physical examination during the collection period outlined above. Those with tumor, autoimmune disease, genetic disease, blood disease, liver and kidney disease, pregnancy, or cerebrovascular disease history were excluded.

Full table
Serum sample collection and preservation
All samples were collected by drawing fasting blood in the morning into a 5 mL BD Vacutainer (Becton Dickinson Vacutainer Systems, Franklin Lakes, NJ, USA) without anticoagulant. The samples were left to clot at room temperature for 1 h, centrifuged at 3,500 rpm for 10 min, and stored at –80 °C for further analysis.
Instruments and reagents
A SELDI-TOF-MS spectrometer (PBSII-c) was purchased from Ciphergen Biosystems company (Austin, TX, USA), and CM10 chips were purchased from Bio-Rad Laboratories, Inc. (Hercules, CA, USA). Urea, 3- [3-(cholamide propyl) dimethylamino] propyl sulfonate, dithiothreitol, sodium acetate, water for high-performance liquid chromatography (HPLC), and sinapinic acid were purchased from Sigma-Aldrich (St. Louis, MO, USA).
Sample pretreatment
The serum samples were thawed and centrifuged at 4 °C for 10,000 rpm for 5min. The supernatant was removed, and 3 µL of each sample was mixed with 6 µL 0.5% U9 buffer (9M urea, 0.2% 3- [3- (cholamide propyl) dimethylamino] propyl sulfonate, 0.1% dithiothreitol). Samples were then incubated for 30 min at 4 °C on a platform shaker set at 600 rpm. Next, 108 µL of binding buffer (50 mM sodiumacetate pH4.0) was added to the above-mentioned 9 µL sample. The CM10 chip was removed and loaded into the bioprocessor. Then, 200 µL of sodium acetate buffer (50 mM, pH4.0) was added to each well and incubated for 5 min at 4 °C and shaken at 600 rpm. The liquid was removed and retained, and the procedure was repeated. After removing the buffer, 200 µL of sodium acetate buffer was added into each well and incubated for 5 min at 4°C and shaken at 600 rpm. Samples retained from different patients were added to separate wells (100 µL/well), incubated for 1 h at 4 °C, and shaken at 600 rpm. They were then washed twice with 200 µL of sodium acetate buffer for 5 min at 4 °C, shaken at 600 rpm, and rinsed twice with 200 µL of HPLC water. Prior to SELDI-TOF-MS, the chip was removed from the bioprocessor, and 0.5 µL of a saturated solution of sinapinic acid in 50% acetonitrile and 0.5% trifluoroacetic acid was added to each chip array and air dried at room temperature.
SELDI-TOF-MS analysis
The prepared CM10 chips were placed on Protein Biological System II-c mass spectrometer reader (PBSII-c; Ciphergen Biosystems), the automatic baseline correction was applied, and the peak of molecular weight between 1 kDa and 50 kDa was automatically detected. Data were collected by averaging 80 laser shots collected on each spot at a laser intensity of 175 and a detector sensitivity of 8. The quality range was from 1,000 to 50,000 mass-to-charge ratio (m/z) and the highest m/z to 50,000. External calibration of the instrument used the all-in-one peptide molecular mass standards and NP20 chip (Ciphergen Biosystems).
Data processing
Ciphergen ProteinChip 3.2.1, Biomarker Wizard 3.1.0, and BPS 5.0 software are all available data analysis support software provided by Ciphergen Biosystems and were used in this study. Ciphergen ProteinChip 3.2.1software was used to analyze the protein peaks with a m/z between 1,000 and 50,000. To avoid interference from polymers, energy molecules, and other possible chemical contaminants, protein peaks with an m/z between 0 and 1,000 were excluded. Protein peak detection involved baseline correction, quality accuracy calibration, and automatic peak detection. Biomarkers were generated using Biomarker Wizard 3.1.0 software. Differential protein peaks were selected according to the differences in protein peak intensities between groups using Biomarker Wizard 3.1.0 software. A decision tree classification algorithm was constructed by using Biomarker Patterns 5.0 software (BPS, Ciphergen Biosystems). BPS is an implementation of the classification regression tree (CART) decision tree system, which uses the peak information generated by the training set of a known sample to construct a binary decision tree algorithm (11). The algorithm allocates each sample in the data set to one of two nodes and makes rules according to the specific peak or strength of the allocator.
Statistical analysis
The spectra of 149 serum samples were randomly divided into a training group (a total of 93 patients, including 30 HCI, 31 HCs, and 32 CI patients) and a blind test group (a total of 56 patients, including 18 HCI, 17 HCs, and 21 CI patients). Our aim was to build a decision tree classification model by using the training group. The data of the clinical diagnosis and proteomic spectrum of each sample are available. The decision tree classification model was applied to the blind test data to estimate the accuracy of the model. All results are expressed as mean ± standard deviation. Independent samples t-test was used for comparison between groups, and a one-sample Kolmogorov-Smirnov test was used for the normality hypothesis test. P values <0.05 were considered statistically significant. Sensitivity was defined as the possibility of predicting the diagnosis of HCI, and specificity was defined as the possibility of predicting the diagnosis of CI or HCs. Accuracy was considered to be the ratio of correct classification.
Results
Instrument calibration and repeatability
SELDI-TOF-MS was calibrated with the all-in-one peptide molecular mass standard before the beginning of the experiment, which included five polypeptides, including 1,084.247 (arginine 8-vasopressin), 1,637.903 (growth hormone inhibitor), 3,495.941 (bovine insulin B chain), 5,807.653 (human insulin), and 7,033.614 (hirudin) (Figure 1). The mass accuracy of SELDI-TOF-MS reached 0.09%.
The standard quality control serum was measured eight times according to the sample processing procedure. The coefficient of variation of the mass-to-charge ratio ranged from 0.02 to 0.04, while the protein peak strength ranged from 0.30 to 0.68. The mean variance coefficient based on the standard quality control serum was lower than 17% (Figure 2). Therefore, the diurnal variation from samples, instruments, and chips could be ignored.
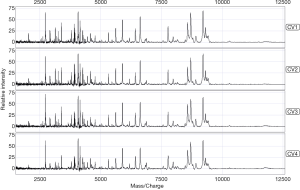
Comparison of serum protein profiles of the HCI group and the HCs group (model 1)
In a comparison of the mass spectra of 30 patients with HCI and 31 with HCs, it was found that 122 protein peaks were detected in the m/z range of 1,000 to 50,000. After statistical analysis, nine differential protein peaks were screened out, including m/z 1,258.3, 2,000.1, 2,016.4, 2,034.7, 3,495.2, 4,426.9, and 8,941.0, which were higher in the HCI group than in the HCs group. The expressions of m/z 4,161.7 and 15,890.4 in the HCI group were lower than those in the HCs group (Table 2). To develop biomarker patterns for the diagnosis of HCI, three differential protein peaks (m/z 3,495.2, 8,941.0 and 15,890.4) were selected to establish the decision tree classification model (Figure 3). Figure 4 shows the tree structure and sample distribution. The sensitivity, specificity, accuracy, and area under the receiver operating characteristic (ROC) curve of model 1 for the diagnosis of HCI were 86.8%, 87.1%, 86.9%, and 0.899, respectively (Table 3 and Figure 5). The sensitivity, specificity, and accuracy of the blind test validation of model 1 were 88.9%, 94.1%, and 91.4%, respectively (Table 3).
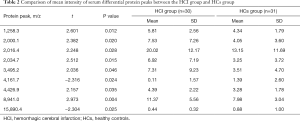
Full table
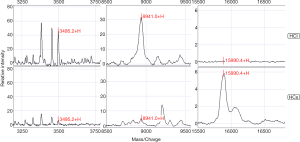
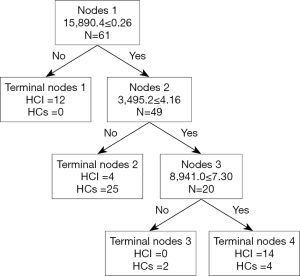
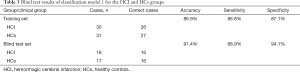
Full table
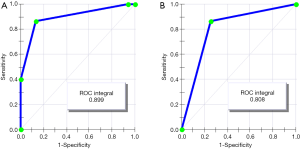
Comparison of serum protein profiles of the HCI group and CI group (model 2)
In a comparison of the mass spectra of 30 patients with HCI and 32 with CI, it was found that 122 protein peaks were detected in the m/z range of 1,000 to 50,000. After statistical analysis, 14 differential protein peaks were screened, which were m/z 1,135.3, 1,258.3, 1,479.2, 1,505.1, 2,941.3, 3,379.0, 3,452.7, 4,233.9, 4,277.6, 4,426.9, 4,481.6, 8,941.0, 9,152.1, and 17,417.2. The expressions of m/z 1,135.3, 1,479.2 and 2,941.3 in the HCI group were lower than those in the CI group, while the expressions of other m/z values were higher than those in the CI group (Table 4). m/z 2,941.3 was selected to establish the decision tree classification model between the HCI group and CI group (Figure 6). Figure 7 shows the tree structure and sample distribution. The sensitivity, specificity, accuracy, and area under ROC curve of the model 2 were 86.7%, 75.0%, 80.6%, and 0.808 respectively (Table 5, Figure 5). The sensitivity, specificity, and accuracy of the blind test validation model were 83.3%, 90.4%, and 87.2%, respectively (Table 5).
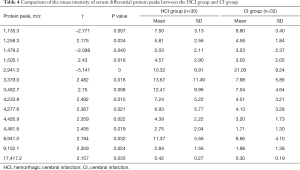
Full table
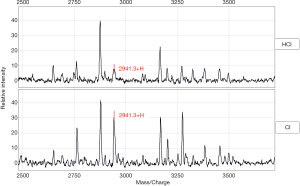
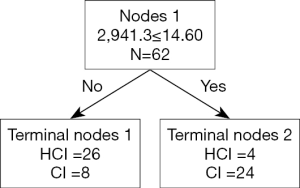
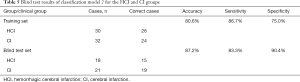
Full table
Discussion
Stroke is the primary cause of death and disability in Chinese adults, and has a high incidence, disability, mortality, and recurrence rate (1). CI is also known as ischemic stroke. In 2016, the incidence rate of CI in China was 276.25/10 million, the prevalence rate was 1,762.77/10 million, the recurrence rate was 17.1%, and the mortality rate was 56.9/10 million (1). HCI, also known as post-CI hemorrhage transformation, refers to a hemorrhage caused by blood flow reperfusion after CI (4). Compared with CI, HCI is more elusive and has a worse prognosis (12). There are four types of mechanism proposed for HCI. The first type is ischemic injury. After cerebral infarction, ischemia leads to endothelial cell damage and basement membrane degradation, resulting in the destruction of neurovascular unit function and structure, and the gradual exudation of blood components (including blood cells), resulting in hemorrhagic transformation (13). The second type is reperfusion injury which includes cerebral vascular embolization, embolus rupture, dissolution, or distal vascular paralysis after dilation of the embolus with blood flow to the distal end of the vessel, under the action of blood pressure rupture bleeding and the formation of hemorrhagic transformation (14). The third type is coagulation function disorder. The use of thrombolytic, anticoagulant, anti-platelet drugs can lead to coagulation factor dysfunction or thrombocytopenia and increase the risk of hemorrhagic transformation (15). The fourth type is blood-brain barrier breakdown. The activation of oxidative stress reaction, inflammatory reaction, and vascular reaction after cerebral infarction releases a series of inflammatory factors, oxygen-free radicals, and cytokines which destroy the blood-brain barrier and lead to hemorrhagic transformation (16,17). After ischemia, the activation of extracellular matrix proteolytic enzymes (mainly matrix metalloproteinases) degrades the extracellular matrix (including collagen and laminin), which destroys the tight connections between endothelial cells, leading to the destruction of the blood-brain barrier and ultimately to the occurrence of hemorrhagic transformation (18-20).
In addition to the above differences, the treatment methods between CI and HCI also differ. For patients with a definite diagnosis of CI, intravenous thrombolysis, anticoagulation, and defibrillation should be applied (21), while for patients with HCI, thrombolysis, anticoagulation, and coagulation factor supplementation should be stopped (21). At present, the imaging mainstays of MRI (2) and CT (3) are the gold standard for the diagnosis of HCI. These require that patients visit a hospital equipped with MRI and CT equipment, and several days are needed to perform the relevant examinations, which increases the corresponding treatment time (22). Therefore, the early and accurate diagnosis of HCI would a valuable tool in the treatment of HCI. In this study, SELDI-TOF-MS and CM10 ProteinChip were used to search for potential protein markers in the serum of HCI, and a decision tree classification model was established to identify new indicators for early clinical diagnosis.
In this experiment, CI, which is closely related to HCI in clinic, was selected as the differential diagnosis disease while a healthy physical examination group was used as the control group, with serum samples being collected from all groups for the experiment. Previous studies on markers of proteins related to HCI have mainly focused on single proteins, such as matrix metalloprotein-9, S100B, glial fibrillary acidic protein, neural specific enolase, high-sensitivity C-reactive protein, vascular adhesion protein, semicarbazide-sensitive amine oxidase, ferritin, and cell junction protein (23). However, few studies have attempted to identify the screening serum protein markers for HCI or to establish diagnostic models by mass spectral techniques of proteomic methods. Among these methods, SELDI-TOF-MS combined with protein chip is a very useful technology in screening biomarkers, as it can directly analyze complex samples. It can detect proteins lower than 20 kDa, and these proteins with a small molecular weight may have biological significance, as they might have been cut or shed due to disease. For example, Schlichtemeier et al. screened four proteins with SELDI-TOF-MS, using a method which could distinguish hepatocellular carcinoma from adjacent non-neoplastic liver tissues (24). Meanwhile, Allard et al. used SELDI-TOF-MS and SAX chip (strong anion) technology to analyze the serum of patients with ischemic stroke and hemorrhagic stroke, and found four differential protein peaks. Among these, apolipoprotein CI (APOC-I) and apolipoprotein CIII (APOC-III) could ably distinguish patients with ischemic stroke from those with hemorrhagic stroke (25).
Through comparison with the diagnostic model established in this experiment, it was found that there was a marked difference in the serum proteome between the HCI, CI, and HCs groups. The accuracy of the diagnosis model1 established by the HCI and HCs group was 86.9%, and the accuracy of the diagnosis model2 established by the HCI and CI group was 80.6%. Furthermore, by combining the ROC curve and the area under the curve (AUC) value, the established diagnostic model could ably distinguish the HCI group from the CI and HCs groups. Meanwhile, the expression of m/z 8,941.0 in the HCI group was higher than that in the CI group and HCs group. In a review of the relevant literature, it was found that Shi et al. combined SELDI-TOF-MS and WCX chip technology to analyze the differential protein peak, m/z 8,937, and identified acute lymphoblastic leukemia as a complement C3a (26). m/z 8,941.0 is the closest to m/z 8,937, and it is likely that they are the same protein. Complement components play an important role as mediators of inflammatory and immune responses. Complement 3 is composed of α and β chains, and is the most abundant complement component in serum (27). Studies have shown that tissue fibrinogen activator can promote the cleavage of complement 3 into complement 3a and complement 3b, thereby leading to enhanced permeability of endothelial cells related to the complement 3a receptor and increased hemorrhagic transformation in patients with ischemic stroke (28).
The next step in research will be to identify the differential protein peaks by secondary mass spectrometry methods like liquid chromatography-mass spectrometry, and to verify them using immunological techniques. The confirmation of differential protein can provide a sound basis for studying the pathogenesis of HCI. The reason for the small sample size included in this study is that reports on SELDI-TOF-MS being used to study HCI are rare. We referred to the literature on acute cerebral infarction using the same method, which included 32 patients with acute cerebral infarction and 60 healthy controls (29).
In conclusion, SELDI-TOF-MS combined with proteinchip technology, which is mature and common in clinic, was used in this study to analyze the differential protein peaks in HCI, CI, and HCs serum samples, and to establish the corresponding diagnosis model for the early diagnosis of HCI. Compared with the previous studies which focused on a single protein, the construction of this model type has the advantages of simple operation, high throughput, and high accuracy; it can also aptly address the challenges in HCI diagnosis, which include the difficulty of acquiring early imaging findings. Furthermore, we believe the study of differential protein in the serum of HCI patients and differential diagnosis of disease for HCI can be more useful for the early diagnosis of HCI, and has important reference significance for the study of other diseases.
Acknowledgments
Funding: This work was supported by grants from the Health Science and Technology Development Project of Changping District, Beijing Municipality (Changweike 2018-3-01); Beijing Municipal Administration of Hospitals Incubating Program (Code: PX2020078).
Footnote
Reporting Checklist: The authors have completed the STARD reporting checklist. Available at http://dx.doi.org/10.21037/atm-20-6071
Data Sharing Statement: Available at http://dx.doi.org/10.21037/atm-20-6071
Conflicts of Interest: All authors have completed the ICMJE uniform disclosure form (available at http://dx.doi.org/10.21037/atm-20-6071). The authors have no conflicts of interest to declare.
Ethical Statement: The authors are accountable for all aspects of the work in ensuring that questions related to the accuracy or integrity of any part of the work are appropriately investigated and resolved. All procedures performed in this study involving human participants were in accordance with the Declaration of Helsinki (as revised in 2013). The research scheme was approved by the ethics committee of Beijing Xiaotangshan Hospital (No. 2018-31). All subjects signed the informed consent before the study.
Open Access Statement: This is an Open Access article distributed in accordance with the Creative Commons Attribution-NonCommercial-NoDerivs 4.0 International License (CC BY-NC-ND 4.0), which permits the non-commercial replication and distribution of the article with the strict proviso that no changes or edits are made and the original work is properly cited (including links to both the formal publication through the relevant DOI and the license). See: https://creativecommons.org/licenses/by-nc-nd/4.0/.
References
- Gu HQ, Yang X, Rao ZZ, et al. Disparities in outcomes associated with rural-urban insurance status in China among inpatient women with stroke: a registry-based cohort study. Ann Transl Med 2019;7:426. [Crossref] [PubMed]
- Suh CH, Jung SC, Cho SJ, et al. MRI for prediction of hemorrhagic transformation in acute ischemic stroke: a systematic review and meta-analysis. Acta Radiol 2020;61:964-72. [Crossref] [PubMed]
- Suh CH, Jung SC, Cho SJ, et al. Perfusion CT for prediction of hemorrhagic transformation in acute ischemic stroke: a systematic review and meta-analysis. Eur Radiol 2019;29:4077-87. [Crossref] [PubMed]
- Seibert V, Wiesner A, Buschmann T, et al. Surface-enhanced laser desorption ionization time-of-flight mass spectrometry (SELDI TOF-MS) and ProteinChip technology in proteomics research. Pathol Res Pract 2004;200:83-94. [Crossref] [PubMed]
- Zhang J, Guo F, Wang L, et al. Screening and identification of non-inflammatory specific protein markers in Wilms' tumor tissues. Arch Biochem Biophys 2019;676:108112. [Crossref] [PubMed]
- Golizeh M, Melendez-Pena CE, Ward BJ, et al. Proteomic fingerprinting in HIV/HCV co-infection reveals serum biomarkers for the diagnosis of fibrosis staging. PLoS One 2018;13:e0195148. [Crossref] [PubMed]
- Watt AD, Perez KA, Hung LW. Elucidating the role of metals in Alzheimer's disease through the use of Surface-Enhanced Laser Desorption/Ionisation time-of-flight mass spectrometry. Methods Mol Biol 2011;752:229-40. [Crossref] [PubMed]
- Whelan LC, Power KA, McDowell DT, et al. Applications of SELDI-MS technology in oncology. J Cell Mol Med 2008;12:1535-47. [Crossref] [PubMed]
- Van Gorp T, Cadron I, Daemen A, et al. Proteomic biomarkers predicting lymph node involvement in serum of cervical cancer patients. Limitations of SELDI-TOF MS. Proteome Sci 2012;10:41. [PubMed]
- Chinese Society of Neurology, Chinese Stroke Society. Diagnostic criteria of cerebrovascular diseases in China (version 2019). Chin J Neurol 2019;52:710-5.
- Margari N, Mastorakis E, Pouliakis A, et al. Classification and regression trees for the evaluation of thyroid cytomorphological characteristics: A study based on liquid based cytology specimens from thyroid fine needle aspirations. Diagn Cytopathol 2018;46:670-81. [Crossref] [PubMed]
- van Kranendonk KR, Treurniet KM, Boers AMM, et al. Hemorrhagic transformation is associated with poor functional outcome in patients with acute ischemic stroke due to a large vessel occlusion. J Neurointerv Surg 2019;11:464-8. [Crossref] [PubMed]
- Yaghi S, Willey JZ, Cucchiara B, et al. Treatment and Outcome of Hemorrhagic Transformation After Intravenous Alteplase in Acute Ischemic Stroke: A Scientific Statement for Healthcare Professionals From the American Heart Association/American Stroke Association. Stroke 2017;48:e343-61. [Crossref] [PubMed]
- Ogata J, Yutani C, Imakita M, et al. Hemorrhagic infarct of the brain without a reopening of the occluded arteries in cardioembolic stroke. Stroke 1989;20:876-83. [Crossref] [PubMed]
- Vandelli L, Marietta M, Gambini M, et al. Fibrinogen decrease after intravenous thrombolysis in ischemic stroke patients is a risk factor for intracerebral hemorrhage. J Stroke Cerebrovasc Dis 2015;24:394-400. [Crossref] [PubMed]
- Jin R, Yang G, Li G. Molecular insights and therapeutic targets for blood-brain barrier disruption in ischemic stroke: critical role of matrix metalloproteinases and tissue-type plasminogen activator. Neurobiol Dis 2010;38:376-85. [Crossref] [PubMed]
- Kim EY, Na DG, Kim SS, et al. Prediction of hemorrhagic transformation in acute ischemic stroke: role of diffusion-weighted imaging and early parenchymal enhancement. AJNR Am J Neuroradiol 2005;26:1050-5. [PubMed]
- Jickling GC, Liu D, Stamova B, et al. Hemorrhagic transformation after ischemic stroke in animals and humans. J Cereb Blood Flow Metab 2014;34:185-99. [Crossref] [PubMed]
- Yaghi S, Eisenberger A, Willey JZ. Symptomatic intracerebral hemorrhage in acute ischemic stroke after thrombolysis with intravenous recombinant tissue plasminogen activator: a review of natural history and treatment. JAMA Neurol 2014;71:1181-5. [Crossref] [PubMed]
- Wang W, Li M, Chen Q, et al. Hemorrhagic Transformation after Tissue Plasminogen Activator Reperfusion Therapy for Ischemic Stroke: Mechanisms, Models, and Biomarkers. Mol Neurobiol 2015;52:1572-9. [Crossref] [PubMed]
- Chinese Society of Neurology, Chinese Stroke Society. Chinese guideline for diagnosis and treatment of acute ischemic stroke 2018. Chin J Neurol 2018;51:666-82.
- Behnke S. Time is brain: Time management in acute stroke treatment. Radiologe 2019;59:590-5. [Crossref] [PubMed]
- Lu G, He Q, Shen Y, et al. Potential biomarkers for predicting hemorrhagic transformation of ischemic stroke. Int J Neurosci 2018;128:79-89. [Crossref] [PubMed]
- Schlichtemeier SM, Nahm CB, Xue A, et al. SELDI-TOF MS Analysis of Hepatocellular Carcinoma in an Australian Cohort. J Surg Res 2019;238:127-36. [Crossref] [PubMed]
- Allard L, Lescuyer P, Burgess J, et al. ApoC-I and ApoC-III as potential plasmatic markers to distinguish between ischemic and hemorrhagic stroke. Proteomics 2004;4:2242-51. [Crossref] [PubMed]
- Shi L, Zhang J, Wu P, et al. Discovery and identification of potential biomarkers of pediatric acute lymphoblastic leukemia. Proteome Sci 2009;7:7. [Crossref] [PubMed]
- Lin Z, Lin H, Li W, et al. Complement Component C3 Promotes Cerebral Ischemia/Reperfusion Injury Mediated by TLR2/NFκB Activation in Diabetic Mice. Neurochem Res 2018;43:1599-607. [Crossref] [PubMed]
- Zhao XJ, Larkin TM, Lauver MA, et al. Tissue plasminogen activator mediates deleterious complement cascade activation in stroke. PLoS One 2017;12:e0180822. [Crossref] [PubMed]
- Zhang X, Guo T, Wang H, et al. Potential biomarkers of acute cerebral infarction detected by SELDI-TOF-MS. Am J Clin Pathol 2008;130:299-304. [Crossref] [PubMed]
(English Language Editor: J. Gray)