ncDRMarker: a computational method for identifying non-coding RNA signatures of drug resistance based on heterogeneous network
Introduction
Chemotherapy is currently the primary treatment strategy for patients with resectable or advanced tumors. However, patients with similar clinical features are usually treated with standard protocols without considering individual responses. Tumor heterogeneity in different patients may lead to significant variations in the effect of chemotherapy, resulting in drug resistance and eventually failure of chemotherapy (1-3). Identifying chemoresistance signatures can help overcome this problem (4,5).
Numerous studies have shown that the cancer genome strongly influences clinical responses to treatment and can be used as molecular signatures to determine which patients are likely to benefit from treatment (4). Analyzing the genomic changes of cancer cell lines with drug treatment is a direct and effective method. Baseline gene expression and in vitro drug sensitivity in cell lines are also widely used to reveal the mechanism of action and to predict clinical drug response (5,6). However, drug effectiveness depends not only on the direct control of its targets, but also on the impact of other regulatory factors, complex biological networks and physiological system activities, which have led to the development and comprehensive analysis of network-based algorithms for predicting drug response. For example, Garnett et al. proposed elastic net (EN) regression, a penalized linear modeling technique, which made full use of cooperative interactions among multiple genes and transcripts across the genome, to predict the signature response of each drug (7). Emad et al. proposed a computational method (ProGENI) to identify genes associated with the variation of drug response by integrating network information (8). Research on the prediction of chemoresistance signatures, along with high-throughput technologies and mature algorithms, has made great progress. Most current research on chemoresistance signatures, however, focuses on protein-coding genes.
Emerging evidence demonstrates that ncRNAs, especially microRNAs (miRNAs) and long non-coding RNAs (lncRNAs), are key regulators of many cancers and play an important role in the drug resistance of tumor cells (9-13). For example, miRNA-195 can increase the sensitivity of colon cancer cells to doxorubicin by targeting BCL2L2 mRNA (14). Wen et al. found that by targeting NRAS and E2F2, miR-26a can enhance the sensitivity of gastric cancer cells to the chemotherapeutic drug cisplatin (15). Moreover, lncRNA TUG1 mediates cell proliferation and chemoresistance in small cell lung cancer by regulating LIMK2b via EZH2 (16). Fang et al. found that lncRNA UCA1 regulates SF1 by acting as a competing endogenous RNA for miR-184 to promote proliferation and modulate the resistance of oral squamous cell carcinoma to cisplatin (17). Briefly, ncRNAs influence drug resistance directly or indirectly and may be new chemoresistance signatures. Thus, the identification of ncRNA signatures of drug resistance would be meaningful to precision medicine.
In this study, we have described ncDRMarker, a computational method for the identification of ncRNA signatures of drug resistance. The method took full consideration of the heterogeneous biological interactions and achieved reasonable efficacy. The role of identified ncRNA signatures in drug resistance were further verified by literature, cancer cell lines and biological function analysis. We also developed a user-friendly platform to identify candidate ncRNAs of drug resistance, which was available at http://bio-bigdata.hrbmu.edu.cn/ncDRMarker/index. The identified ncRNAs may be potential biomarkers, which can be targeted to overcome drug resistance and help to realize individualized treatment. We present the following article in accordance with the MDAR checklist (available at http://dx.doi.org/10.21037/atm-20-603).
Methods
Dataset collection
The known ncRNAs (miRNAs and lncRNAs) related to drug resistance were downloaded from ncDR (http://www.jianglab.cn/ncDR/) (18) and the database of Genomic Elements Associated with drug Resistance (GEAR) (19). The ncDR database is a comprehensive cheminformatics resource that collects drug resistance-related ncRNAs through manual curation from literature before 2016. GEAR is a database of genomic elements associated with drug resistance that aims to provide comprehensive information about chemoresistance biomarkers. Moreover, we queried the PubMed database and manually extracted experimentally validated drug resistance-ncRNA relationships from literature published between Jan 2016, and Mar 2018, to supplement the known ncRNAs related to drug resistance. We further standardized drug names and miRNA symbols via DrugBank (20) and miRBase (21), and finally, we collected 3,414 drug resistance-ncRNA relationships involving 67 drugs and 808 ncRNAs (758 miRNAs and 50 lncRNAs).
In addition, for method comparison, we collected protein-coding genes related to drug resistance. The compilation of relevant information from the dispersed published literature is a monumental challenge, so we extracted chemoresistance genes from Catalogue Of Somatic Mutations In Cancer (COSMIC v86) (22), HerceptinR (23) and GEAR (19). Finally, 1,317 drug resistance-gene relationships, including 54 drugs and 656 genes were used for the performance comparison of our method.
In this study, the NCI-60 cell line panel and its related drug screens were obtained from CellMiner (24), which were widely used to pioneer the approach of linking drug resistance to genomic data. The miRNA expression profiles for the 60 human cancer cell lines and drug activity data in terms of the 50% growth inhibitory concentration (GI50) of 1,429 small molecule drugs were collected and preprocessed. These cancer cell lines were used to verify whether the identified chemoresistance miRNAs can accurately characterize the cell line as either sensitive or resistant to anti-cancer drugs.
Constructed composite network
In this study, we constructed a large-scale composite network by integrating heterogeneous biological interactions, which were all experimentally validated. The protein-protein interactions (PPIs) were from the Human Protein Reference Database (HPRD, HPRD_Release9_041310) (25). All PPIs in HPRD were credible as they were manually extracted from the studies performed by expert biologists. MiRNA-target gene data were downloaded from miRTarBase (Release 7.0), which collects miRNA-target interactions by manually surveying the relevant literature (26). MiRNA-lncRNA interactions were collected from starBase v2.0 (27) and DIANA-LncBase v2.0 (28), which contain experimentally validated miRNA-lncRNA interactions. We further standardized RNA symbols and removed repeating interactions, and acquired 16,710 mRNAs, 2,602 miRNAs, 6,759 lncRNAs and 481,511 interactions. Finally, these RNAs and interactions were used to construct a mRNA-miRNA-lncRNA composite network.
Identifying candidate ncRNAs based on the composite network
The ncDRMarker could identify candidate ncRNAs involved in drug resistance by fully exploiting the heterogeneous biological interactions. With the composite network mentioned above, we extended the random walk with restart (RWR) method to the network. The RWR algorithm was derived from graph theory and simulated a random walker, which started from the seed nodes and repeated an iterative transition until all vertices achieved the steady state probability. At each step, the walker moved from the current nodes to their immediate neighbors with probability 1-r or stayed at the current nodes with probability r, according to the probability transition matrix. In this method, the RWR is defined as:
[1]
In the above-mentioned formula, rϵ(0,1) indicates the restart probability, which was set as 0.2 in this study (Figure S1). The initial probability vector P0 is a normalized unit vector, in which each seed node (known ncRNAs involved in chemoresistance of the drug) has equal probabilities. Pt represents a vector in which the i-th element holds the probability of being at node i at step t. The transition matrix W is a column-normalized adjacency matrix of the composite network, in which Wij is the transition probability from node i to node j. When the difference between Pt and Pt+1 falls below 10-10, it achieves the steady state. The result is a list of ncRNAs ranked by the probability scores, in which top ranked ncRNAs are defined as candidate ncRNA signatures of drug resistance. The flowchart of ncDRMarker is shown in Figure 1.
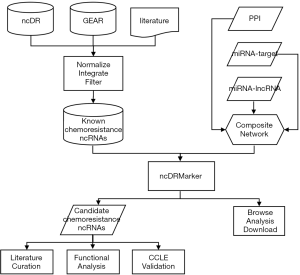
Performance measurement
Cross validation is widely used to evaluate the performance of prediction algorithms. The receiver operating characteristic curve (ROC) plots the true (sensitivity) versus false positive rate (1-specificity) at different cutoffs, and area under curve (AUC) is commonly used to represent the results of cross validation. In this study, we used leave-one-out cross validation (LOOCV) for known drug resistance-ncRNA relations to estimate the performance of ncDRMarker. For every drug, each known drug resistance-ncRNA relation was considered as one test case, the remaining known drug resistance-related ncRNAs were considered as seed nodes, and the held out ncRNA and other ncRNAs in the composite network were considered as candidates. Then, we could obtain a rank list of all candidates by performing the RWR method. Finally, the ROC and AUC intuitively displayed a result of the performance.
Validation of Identified ncRNAs of drug resistance
NCI-60 cancer cell lines and their related drug screens from CellMiner database were used to validate the identified chemoresistance ncRNAs. Due to the scarce lncRNA expression profiles relevant to pharmacogenomics, we only tested whether the identified miRNAs could accurately classify the cancer cell lines into sensitive or resistant to drug according to drug concentrations. For each identified miRNA-drug resistance relation, we firstly extracted two groups of cell lines from 60 cancer cell lines according to the expression of the miRNA: the top 25% cancer cell lines were group 1, and the bottom 25% cancer cell lines were group 2. Next, we collected the drug concentrations (normalized GI50 values) across the cancer cell lines in two groups, then the comparisons were performed by using Wilcoxon rank-sum test on two groups. We deemed that the identified miRNAs could significantly classify the cancer cell lines into drug sensitive or resistant when there was significant difference between two groups (P value <0.01).
Enrichment analysis was implemented to characterize the biological function of some identified ncRNAs involved in multiple drug resistance. Since most of the ncRNA functions remain unclear, we studied the biological function of ncRNAs based on the protein-coding genes related to ncRNAs. We downloaded target genes of miRNAs from miRTarBase for enrichment analysis. Meanwhile, we collected 28 human RNA-seq datasets generated under different experimental conditions from the NCBI Sequence Read Archive (SRA) database (29). Then, we calculated the Pearson correlation coefficients (PCC) between lncRNA and mRNA expression and obtained the co-expressed protein-coding genes of lncRNA with a strict threshold (P value <0.01 and PCC values ranked in the top or bottom 0.1%). Then, we implemented KEGG (Kyoto Encyclopedia of Genes and Genomes) (30) pathway enrichment analysis for each identified ncRNA using Metascape with default parameters (31).
Framework and web interface
We provided a web tool for users to identify candidate ncRNAs involved in drug resistance. The website was developed using JavaEE platform and operated on Tomcat 6.0 web server. All the data were stored in a MySQL 5.6 relationship database management system. The server-side was implemented with Java 1.7 scripts, and the web server was written in JSP. ncDRMarker is freely available at http://bio-bigdata.hrbmu.edu.cn/ncDRMarker/index.
Results
Evaluating the performance of ncDRMarker
To assess ncDRMarker’s performance, we assessed its ability to identify known ncRNAs involved in drug resistance. We chose drugs with at least two known chemoresistance ncRNAs in the composite network and obtained 56 anti-cancer drugs. For each drug, the LOOCV method was applied on known drug resistance-ncRNA relations. We performed ROC by plotting the true positive rate (sensitivity) versus the false positive rate (1-specificity) at various threshold settings to evaluate the overall performance. We found that all drugs achieved high predictive performances, the AUC values ranged from 0.816 to 0.998 (Table S1). Furthermore, the drugs that had at least five known chemoresistance ncRNAs in the composite network achieved a higher performance, and the AUC values ranged from 0.881 to 0.998. We selected ten common clinical anti-cancer drugs (imatinib, erlotinib, docetaxel, gemcitabine, sorafenib, 5-fluorouracil, doxorubicin, paclitaxel, cisplatin, and tamoxifen) for later analysis. Figure 2 shows the ROC curves of these drugs for identifying chemoresistance ncRNA signatures, with the AUC values ranging from 0.881 to 0.951. The ncDRMarker’s high predictive power indicates that the approach utilizing multi-omics data interactions from the composite network has high efficiency in identifying candidate ncRNA signatures involved in drug resistance.
In addition, we adopted different types of seed nodes to compare the method and evaluate the performance of ncDRMarker. Because of the scarcity of ncRNA signatures involved in chemoresistance, we collected experimentally verified protein-coding genes related to drug resistance to supplement the seed nodes. Further, based on the type of seed nodes, we divided the test case into three groups: known ncRNAs related to drug resistance were defined as group 1 (ncRNA); known experiment verified RNAs related to drug resistance were defined as group 2 (ncRNA+mRNA); and known experiment verified protein-coding genes related to drug resistance were defined as group 3 (mRNA). We implemented ncDRMarker with different groups and obtained results as expected (Figure 3A). The results showed that when only ncRNAs were used as seed nodes, the algorithm had the highest efficiency, and the AUC values were between 0.881 and 0.951. Secondly, when ncRNAs+mRNAs were used as seed nodes, the algorithm also had a high performance, and the AUC values were between 0.799 and 0.921. The research on ncRNAs involved in drug resistance has been relatively limited and scattered, and highly credible and large-scale drug resistant-ncRNA data are still scarce. Therefore, if the number of known ncRNAs involved in drug resistance is small (the number of nodes is less than five), the model of mRNA+ncRNA can be used as the complementary algorithm in this study. Finally, when we used mRNAs as seed nodes, the AUC values were between 0.640 and 0.881, which were lower than other groups. This suggested that the efficiency of the algorithm was higher when prior knowledge of ncRNAs related to drug resistance was used.
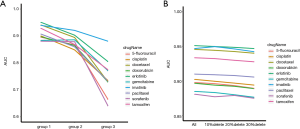
We evaluated the robustness of ncDRMarker by randomly deleting the regulatory relationship (edge) of the composite network. We deleted 10%, 20% and 30% regulatory relationships in the network at random and repeated five times for each deletion. Then, we applied the algorithm to the new incomplete network and evaluated the prediction results using the LOOCV. The mean AUC value from five repetitions is as shown in Figure 3B. The results showed that some relationships in the network had been discarded randomly, and the AUC of the method did not change significantly, suggesting that the ncDRMarker approach is robust to changes in the network.
Identifying chemoresistance ncRNA signatures
We constructed an mRNA-miRNA-lncRNA composite network that included 26,071 nodes (16,710 mRNAs, 2,602 miRNAs and 6,759 lncRNAs) and 481,511 edges. The RWR algorithm, which makes full use of the network topology, was applied to identify candidate ncRNAs involved in drug resistance on the composite network. This algorithm was used for ten common clinical anti-cancer drugs to identify chemoresistance ncRNA biomarkers. The resulting list of the top 50 candidate chemoresistance ncRNAs for each drug is shown inTable S2, and some of the identified candidate ncRNAs were manually confirmed as being related to chemoresistance or drug indications by newly published literature (Table 1). For example, cisplatin is a platinum-based chemotherapy drug and has been widely used in clinics to treat various types of cancers. Some top ranked ncRNAs in the resulting list have been confirmed to be related to the resistance of cisplatin, such as the lncRNA NEAT1. NEAT1 ranked second in the resulting list, and Yan et al. demonstrated this NEAT1-cisplatin resistance relationship in anaplastic thyroid carcinoma (ATC) cells (32). They found miR-9-5p (seed node of cisplatin) overexpression sensitized ATC cells to cisplatin. NEAT1 suppressed miR-9-5p expression by binding to miR-9-5p, and SPAG9 was a direct target of miR-9-5p. NEAT1 silencing exerted its inhibitory effect on cisplatin-resistance of ATC via the miR-9-5p/SPAG9 axis in vitro and in vivo. Their results demonstrated that lncRNA NEAT1 enhances the resistance of ATC cells to cisplatin by sponging miR-9-5p and regulating SPAG9 expression. The overexpression of another top ranked ncRNA, miR-186, can reverse cisplatin resistance and the formation of the glioblastoma-initiating cell phenotype in glioblastoma cells (33). Gemcitabine is prevalently used to treat a variety of cancers, such as advanced ovarian, metastatic non-small cell lung cancer (NSCLC), and metastatic adenocarcinoma of the pancreas. Yan et al. found that hsa_circ_0035483 sponges hsa-miR-335, one of the top ranked ncRNAs, can promote gemcitabine resistance in renal clear cells (34). Linc-ROR modulated the miR-124-3p, a candidate ncRNA, conferred gemcitabine resistance to pancreatic cancer cells (35). Our results suggested that ncDRMarker was an effective method for identifying ncRNA signatures of drug resistance, and the identified ncRNAs may be used as biomarkers for targeted cancer therapies.
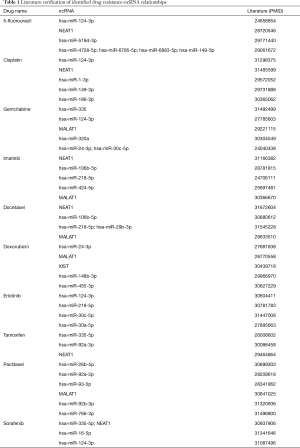
Full table
Validating identified chemoresistance ncRNA in cancer cell lines
Cancer cell lines and drug activity scores have been widely used to identify chemoresistance biomarkers. In this research, NCI-60 cancer cell lines were used to check the chemoresistance of the ncRNA signature, to elucidate whether the identified ncRNA could further classify the cancer cell lines into either sensitive or resistant to drug treatment. Due to the lack of pharmacogenomics-related lncRNA expression profiles, only the identified chemoresistance miRNA markers were validated using cancer cell lines. For each identified miRNA involved in drug resistance, we divided the cancer cell lines into group 1 and group 2 according to the expression values of this miRNA. Then, we performed Wilcoxon rank-sum test on these two groups by using the drug concentrations (normalized GI50 scores) across cancer cell lines in two groups (see Validation of Identified ncRNAs of Drug Resistance in Methods). From the results, we found that miR-92a-3p, a candidate ncRNA of tamoxifen and paclitaxel resistance, can significantly classify cancer cell lines into sensitive or resistant by using tamoxifen (or paclitaxel) activity z scores. Tamoxifen is a common clinical anti-cancer drug that has been used to treat estrogen receptor-positive metastatic breast cancer in adults, and miR-92a-3p was ranked as a top candidate in the tamoxifen ncRNA list. In this study, the tamoxifen concentration distribution was significantly different between two groups, which was divided by miR-92a-3p (Wilcoxon rank-sum test, P=0.0014; Figure 4A left). Cun et al. reported this tamoxifen-miR-92a-3p relation in their study. They found that miR-92a-3p expression was higher in breast cancer serum or tissue, and high expression of miR-92a-3p could predict poor prognosis of breast cancer patients. They validated the point by their qRT-PCR experiment, that miR-92a-3p was upregulated in tamoxifen-resistant cells. Their results demonstrated that the relationship between miR-92a-3p and some key genes were related to tamoxifen resistance (36). We further dissected the PPI network, and found that targets of tamoxifen were closely connected to target genes of miR-92a-3p (Figure 4A right), which indirectly explained why miR-92a-3p was involved in tamoxifen resistance. Paclitaxel is a chemotherapeutic agent that has been widely used in the treatment of lung, ovarian, and breast cancer, and miR-92a-3p was top ranked in its candidate ncRNA list. Cancer cell lines were also significantly classified into sensitive or resistant by miR-92a-3p according to paclitaxel activity z scores (Wilcoxon rank-sum test, P=0.0032; Figure 4B left). Chen et al. identified that a STAT3-miR-92a-DKK1 pathway was involved in the generation of cancer stem-like cells in ovarian tumors. They observed that this pathway might be related to paclitaxel resistance by blocking its progression (37). Further, targets of paclitaxel were also closely connected to target genes of miR-92a-3p in the PPI network (Figure 4B right). In addition, to characterize the biological function of miR-92a-3p, we applied KEGG pathway enrichment on target protein-coding genes of miR-92a-3p. Significantly enriched pathways (P value <0.01) are displayed in Figure 4C, among which, cell cycle (hsa04110), ribosome (hsa03010), and thyroid hormone signaling pathway (hsa04919) were significantly related to cancers, which indicated that miR-92a-3p might be involved in multiple drugs resistance. The above results further illustrated the effectiveness of ncDRMarker and showed that the identified ncRNAs can be used as chemoresistance ncRNA signatures.
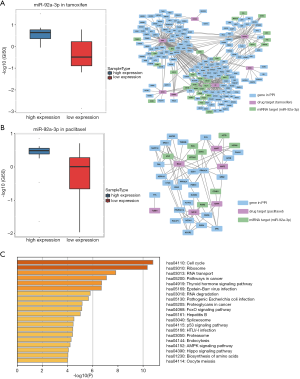
Functional characterization of identified chemoresistance ncRNAs
We found that some ncRNA signatures, such as miR-335-5p, miR-124-3p, miR-92a-3p, LINC00657, etc., were top ranked in the candidate ncRNA lists of many drugs. For example, miR-124-3p was identified to be involved in the resistance to erlotinib, 5-fluorouracil, gemcitabine, cisplatin and sorafenib (ranked top 50 in candidate ncRNA list). We investigated the composite network and found that miR-124-3p was the hub node in the network. It was closely connected to some significant genes, which were immediate neighbors of some drug resistance seed nodes (see Figure 5). EPHA2, for instance, was an immediate neighbor of miR-200a-3p, miR-34a-5p and miR-141-3p (seed nodes of erlotinib), and studies showed that miR-124-3p mediates erlotinib resistance in K-RAS mutated pancreatic cancer by targeting EPHA2 (38). EZH2, another key gene, was closely connected to miR-193b-3p and miR-126-3p (seed nodes of sorafenib), and Wang et al. proved that the sorafenib-resistant cells regained sensitivity for sorafenib by EZH2 intervention with miR-124/506 overexpression or EZH2 inhibitor treatment in vitro and in vivo. In their research, miR-124-3p and miR-506-3p (miR-124/506) were remarkably reduced in the serum from sorafenib resistant patients. EZH2, the target gene of miR-124 and miR-506, was modulated by them in sorafenib-resistant cells. Targeting EZH2 by overexpression of miR-124/506 or EPZ-6438 treatment inhibited the proliferation ability of sorafenib-resistant thyroid tumor cells via epigenetic regulation, and the combination of sorafenib with miR-124/506 overexpression or EZH2 inhibitor improved the survival in a mouse model (39). The results indicated that ncRNAs as hub nodes in the heterogeneous network, which receives high probability in the transfer process, might be involved in multiple drug resistance.
To further investigate the regulatory effects of these ncRNAs on drug resistance, we studied the biological functions of the identified ncRNAs. Since the biological functions of most ncRNAs remained unclear, we applied KEGG pathway enrichment to protein-coding genes related to ncRNAs (see 2.5. Validation of identified ncRNAs involved in drug resistance). Pathways with a strict threshold of P value <0.01 were defined as significantly enriched pathways. In this study, most of the chemoresistance ncRNAs were enriched in the PI3K-Akt signaling pathway (hsa04151), p53 signaling pathway (hsa04115) and DNA replication (hsa03030), which are important pathways closely related to cancer. Thus, the candidate chemoresistance ncRNAs in our study were involved in a wide range of biological regulation process, which indicated that they were related to multiple drug resistance indirectly (Table S3).
Web interface of ncDRMarker
ncDRMarker provides a convenient, user-friendly interface that enables users to identify candidate ncRNA signatures involved in drug resistance. First, users can study the drug indicated in this study, their corresponding known chemoresistance ncRNAs can be browsed by clicking on the drug name, then the prediction process can be quickly achieved by clicking “Analysis using ncRNAs below”, and the results will be displayed as a ranked list of ncRNAs involved in drug resistance. Second, users can prioritize the drug chemoresistance ncRNAs by inputting their own data, then the known ncRNAs/genes related to drug resistance are suggested. Further, the results also provide a ranked list of ncRNAs involved in the drug resistance. All the results can be downloaded by clicking “Export Result”. Finally, a “SUBMIT” page is also included in the website, on which users can provide the new drug resistance-ncRNA relationships confirmed by new literature, and the relationships will be integrated to the seed vector once verified.
Discussion
Chemoresistance is the major cause of chemotherapy failure, and the identification of chemoresistance biomarkers can help to overcome this problem and realize individualized treatment (40,41). Protein-coding genes have been widely studied as signatures of drug response, while ncRNA signatures have been scarce. In this study, due to the fact drug efficacy depends on the influence of complex biological interactions, but not just the regulation of its targets, we provided a computational method, ncDRMarker, for the identification of chemoresistance ncRNA signatures based on a heterogeneous network.
Initially, we constructed a composite network by integrating PPI, miRNA-mRNA and miRNA-lncRNA relationships. Then, we applied RWR on this composite network and prioritized candidate ncRNAs related to chemoresistance by fully exploiting the global biological interactions. We have shown that ncDRMarker is an efficient computational technique for identifying ncRNAs that play a role in drug resistance. Using ncDRMarker, we studied ten common clinical anti-cancer drugs, and acquired a decent ROC by performing LOOCV. The AUC values were between 0.881 and 0.951, indicating the high predictive power. Protein-coding genes related to drug resistance may be used as complementary seed nodes to perform ncDRMarker when there are few known ncRNAs involved in drug resistance (less than five). Moreover, the identified candidate ncRNAs were confirmed to be related to chemoresistance or drug indications by the literature. The results that the identified ncRNAs can classify the cancer cell lines into sensitive or resistant according to drug concentrations were further verified using cancer cell lines. In addition, some meaningful ncRNAs, were found to be involved in multiple drug resistance. To study these ncRNAs, we dissected the mRNA-miRNA-lncRNA composite network and found that they are hub ncRNAs that can receive high probability in the transfer process. We further applied functional analysis on these ncRNAs, and the results showed that they involved in a wide range of biological regulation process. Finally, we provided the ncDRMarker website for users to identify chemoresistance ncRNA signatures.
There were also some limitations to our current study. First, compared to the tens of thousands of ncRNAs that have been found, the number of known ncRNAs (especially lncRNAs) involved in drug resistance in our study is quite small. This is because known chemoresistance ncRNAs in our analysis were mainly manually collected from the literature, and although accurate, may be insufficient at the same time. An authoritative and highly credible database of chemoresistance-related ncRNAs will extend and improve the modeling techniques we employed in this study. Another aspect that should be further explored in future studies is integrating genomics data and clinical profiles, which would be an abundant resource in pharmacogenomics.
Conclusions
Here, we have provided a computational method, ncDRMarker, to identify ncRNA signatures of chemoresistance based on a heterogeneous network. Our analysis and results suggest that ncDRMarker is an efficient computational technique for identifying candidate ncRNAs involved in drug resistance, and the identified ncRNAs may be targeted to overcome drug resistance and help realize individualized treatments.
Acknowledgments
We thank International Science Editing for editing the English text of a draft of the manuscript.
Funding: This work was supported by National Key R&D Program of China (2018YFC2000100), the National Natural Science Foundation of China (Grant Nos. 61873075, 61902095, 31801107).
Footnote
Reporting Checklist: The authors have completed the MDAR checklist. Available at http://dx.doi.org/10.21037/atm-20-603
Conflicts of Interest: All authors have completed the ICMJE uniform disclosure form (available at http://dx.doi.org/10.21037/atm-20-603). The authors have no conflicts of interest to declare.
Ethical Statement: The authors are accountable for all aspects of the work in ensuring that questions related to the accuracy or integrity of any part of the work are appropriately investigated and resolved. The study was conducted in accordance with the Declaration of Helsinki (as revised in 2013).
Open Access Statement: This is an Open Access article distributed in accordance with the Creative Commons Attribution-NonCommercial-NoDerivs 4.0 International License (CC BY-NC-ND 4.0), which permits the non-commercial replication and distribution of the article with the strict proviso that no changes or edits are made and the original work is properly cited (including links to both the formal publication through the relevant DOI and the license). See: https://creativecommons.org/licenses/by-nc-nd/4.0/.
References
- Shekhar MP. Drug resistance: challenges to effective therapy. Curr Cancer Drug Targets 2011;11:613-23. [Crossref] [PubMed]
- Holohan C, Van Schaeybroeck S, Longley DB, et al. Cancer drug resistance: an evolving paradigm. Nat Rev Cancer 2013;13:714-26. [Crossref] [PubMed]
- Yan Y, Chen X, Wang X, et al. The effects and the mechanisms of autophagy on the cancer-associated fibroblasts in cancer. J Exp Clin Cancer Res 2019;38:171. [Crossref] [PubMed]
- Yang W, Soares J, Greninger P, et al. Genomics of Drug Sensitivity in Cancer (GDSC): a resource for therapeutic biomarker discovery in cancer cells. Nucleic Acids Res 2013;41:D955-61. [Crossref] [PubMed]
- Geeleher P, Cox NJ, Huang RS. Clinical drug response can be predicted using baseline gene expression levels and in vitro drug sensitivity in cell lines. Genome Biol 2014;15:R47. [Crossref] [PubMed]
- Rees MG, Seashore-Ludlow B, Cheah JH, et al. Correlating chemical sensitivity and basal gene expression reveals mechanism of action. Nat Chem Biol 2016;12:109-16. [Crossref] [PubMed]
- Garnett MJ, Edelman EJ, Heidorn SJ, et al. Systematic identification of genomic markers of drug sensitivity in cancer cells. Nature 2012;483:570-5. [Crossref] [PubMed]
- Emad A, Cairns J, Kalari KR, et al. Knowledge-guided gene prioritization reveals new insights into the mechanisms of chemoresistance. Genome Biol 2017;18:153. [Crossref] [PubMed]
- Ayers D, Vandesompele J. Influence of microRNAs and Long Non-Coding RNAs in Cancer Chemoresistance. Genes (Basel) 2017;8:95. [Crossref] [PubMed]
- Deng H, Zhang J, Shi J, et al. Role of long non-coding RNA in tumor drug resistance. Tumour Biol 2016;37:11623-31. [Crossref] [PubMed]
- Li H, Yang BB. Friend or foe: the role of microRNA in chemotherapy resistance. Acta Pharmacol Sin 2013;34:870-9. [Crossref] [PubMed]
- Yan Y, Xu Z, Li Z, et al. An Insight into the Increasing Role of LncRNAs in the Pathogenesis of Gliomas. Front Mol Neurosci 2017;10:53. [Crossref] [PubMed]
- Xu Z, Yan Y, Qian L, et al. Long non-coding RNAs act as regulators of cell autophagy in diseases Oncol Rep 2017;37:1359-66. (Review). [Crossref] [PubMed]
- Qu J, Zhao L, Zhang P, et al. MicroRNA-195 chemosensitizes colon cancer cells to the chemotherapeutic drug doxorubicin by targeting the first binding site of BCL2L2 mRNA. J Cell Physiol 2015;230:535-45. [Crossref] [PubMed]
- Wen L, Cheng F, Zhou Y, et al. MiR-26a enhances the sensitivity of gastric cancer cells to cisplatin by targeting NRAS and E2F2. Saudi J Gastroenterol 2015;21:313-9. [Crossref] [PubMed]
- Niu Y, Ma F, Huang W, et al. Long non-coding RNA TUG1 is involved in cell growth and chemoresistance of small cell lung cancer by regulating LIMK2b via EZH2. Mol Cancer 2017;16:5. [Crossref] [PubMed]
- Fang Z, Zhao J, Xie W, et al. LncRNA UCA1 promotes proliferation and cisplatin resistance of oral squamous cell carcinoma by sunppressing miR-184 expression. Cancer Med 2017;6:2897-908. [Crossref] [PubMed]
- Dai E, Yang F, Wang J, et al. ncDR: a comprehensive resource of non-coding RNAs involved in drug resistance. Bioinformatics 2017;33:4010-1. [Crossref] [PubMed]
- Wang YY, Chen WH, Xiao PP, et al. GEAR: A database of Genomic Elements Associated with drug Resistance. Sci Rep 2017;7:44085. [Crossref] [PubMed]
- Law V, Knox C, Djoumbou Y, et al. DrugBank 4.0: shedding new light on drug metabolism. Nucleic Acids Res 2014;42:D1091-7. [Crossref] [PubMed]
- Kozomara A, Griffiths-Jones S. miRBase: annotating high confidence microRNAs using deep sequencing data. Nucleic Acids Res 2014;42:D68-73. [Crossref] [PubMed]
- Tate JG, Bamford S, Jubb HC, et al. COSMIC: the Catalogue Of Somatic Mutations In Cancer. Nucleic Acids Res 2019;47:D941-7. [Crossref] [PubMed]
- Ahmad S, Gupta S, Kumar R, et al. Herceptin resistance database for understanding mechanism of resistance in breast cancer patients. Sci Rep 2014;4:4483. [Crossref] [PubMed]
- Reinhold WC, Sunshine M, Liu H, et al. CellMiner: a web-based suite of genomic and pharmacologic tools to explore transcript and drug patterns in the NCI-60 cell line set. Cancer Res 2012;72:3499-511. [Crossref] [PubMed]
- Keshava Prasad TS, Goel R, Kandasamy K, et al. Human Protein Reference Database--2009 update. Nucleic Acids Res 2009;37:D767-72. [Crossref] [PubMed]
- Chou CH, Shrestha S, Yang CD, et al. miRTarBase update 2018: a resource for experimentally validated microRNA-target interactions. Nucleic Acids Res 2018;46:D296-D302. [Crossref] [PubMed]
- Li JH, Liu S, Zhou H, et al. starBase v2.0: decoding miRNA-ceRNA, miRNA-ncRNA and protein-RNA interaction networks from large-scale CLIP-Seq data. Nucleic Acids Res 2014;42:D92-7. [Crossref] [PubMed]
- Paraskevopoulou MD, Vlachos IS, Karagkouni D, et al. DIANA-LncBase v2: indexing microRNA targets on non-coding transcripts. Nucleic Acids Res 2016;44:D231-8. [Crossref] [PubMed]
- Leinonen R, Sugawara H, Shumway M, et al. The sequence read archive. Nucleic Acids Res 2011;39:D19-21. [Crossref] [PubMed]
- Kanehisa M, Goto S, Hattori M, et al. From genomics to chemical genomics: new developments in KEGG. Nucleic Acids Res 2006;34:D354-7. [Crossref] [PubMed]
- Zhou Y, Zhou B, Pache L, et al. Metascape provides a biologist-oriented resource for the analysis of systems-level datasets. Nat Commun 2019;10:1523. [Crossref] [PubMed]
- Yan P, Su Z, Zhang Z, et al. LncRNA NEAT1 enhances the resistance of anaplastic thyroid carcinoma cells to cisplatin by sponging miR95p and regulating SPAG9 expression. Int J Oncol 2019;55:988-1002. [PubMed]
- Li J, Song J, Guo F. miR-186 reverses cisplatin resistance and inhibits the formation of the glioblastoma-initiating cell phenotype by degrading Yin Yang 1 in glioblastoma. Int J Mol Med 2019;43:517-24. [PubMed]
- Yan L, Liu G, Cao H, et al. Hsa_circ_0035483 sponges hsa-miR-335 to promote the gemcitabine-resistance of human renal cancer cells by autophagy regulation. Biochem Biophys Res Commun 2019;519:172-8. [Crossref] [PubMed]
- Li C, Zhao Z, Zhou Z, et al. Linc-ROR confers gemcitabine resistance to pancreatic cancer cells via inducing autophagy and modulating the miR-124/PTBP1/PKM2 axis. Cancer Chemother Pharmacol 2016;78:1199-207. [Crossref] [PubMed]
- Cun J, Yang Q. Bioinformatics-based interaction analysis of miR-92a-3p and key genes in tamoxifen-resistant breast cancer cells. Biomed Pharmacother 2018;107:117-28. [Crossref] [PubMed]
- Chen MW, Yang ST, Chien MH, et al. The STAT3-miRNA-92-Wnt Signaling Pathway Regulates Spheroid Formation and Malignant Progression in Ovarian Cancer. Cancer Res 2017;77:1955-67. [Crossref] [PubMed]
- Du J, He Y, Wu W, et al. Targeting EphA2 with miR-124 mediates Erlotinib resistance in K-RAS mutated pancreatic cancer. J Pharm Pharmacol 2019;71:196-205. [Crossref] [PubMed]
- Wang Z, Dai J, Yan J, et al. Targeting EZH2 as a novel therapeutic strategy for sorafenib-resistant thyroid carcinoma. J Cell Mol Med 2019;23:4770-8. [Crossref] [PubMed]
- Yan Y, Xu Z, Chen X, et al. Novel Function of lncRNA ADAMTS9-AS2 in Promoting Temozolomide Resistance in Glioblastoma via Upregulating the FUS/MDM2 Ubiquitination Axis. Front Cell Dev Biol 2019;7:217. [Crossref] [PubMed]
- Dai S, Yan Y, Xu Z, et al. SCD1 Confers Temozolomide Resistance to Human Glioma Cells via the Akt/GSK3beta/beta-Catenin Signaling Axis. Front Pharmacol 2018;8:960. [Crossref] [PubMed]