Lymphocyte percentage and hemoglobin as a joint parameter for the prediction of severe and nonsevere COVID-19: a preliminary study
Introduction
Coronavirus disease 2019 (COVID-19) has spread rapidly around the world since December, 2019. As of August 7, 2020, 18,902,735 cases and 709,511 deaths had been confirmed globally, and a mortality rate of 3.75% had been reported (1-4). The clinical manifestations of COVID-19 are similar to those of severe acute respiratory syndrome (SARS) and Middle East respiratory syndrome (MERS), with symptoms including fever, dry cough, fatigue, and dyspnea. Severe cases might even progress to a severe life-threatening condition (5-8).
Recent studies on the clinical characteristics of COVID-19 patients have revealed that those with mild or common COVID-19 can experience quick recovery after appropriate clinical intervention. However, patients with severe COVID-19, especially elderly patients or those with underlying diseases, may deteriorate rapidly, leading to a higher risk of mortality (9-15). Dehkordi et al. reported that approximately 14% of COVID-19 patients could be classed as severely ill and 5% as critically ill, with the remaining 80% of COVID-19 patients experiencing a mild or common form of the disease (16). The treatment methods for severe and nonsevere COVID-19 patients differ. Patients with mild disease usually recover gradually after isolation and symptomatic treatment, while severe patients may need to be treated with antiviral drugs, hormones, antibiotics, or immunotherapy, and may even require comprehensive treatment and nursing care in an intensive care unit (ICU) (6-10). Therefore, differentially diagnosing severe from nonsevere COVID-19 is critical. A rapid and accurate assessment of the severity of the disease can guide clinical interventions in a timely manner and facilitate the rational allocation of medical resources, thus reducing the risk of mortality. However, laboratory tests, such as imaging examinations, nucleic acid detection, molecular sequencing, and microbial culture, which can accurately determine disease severity, treatment efficacy, and disease outcomes, are relatively complicated and time-consuming; consequently, disease progression cannot be detected in a timely manner. Therefore, rapid, convenient, and effective indicators that can differentiate between severe and nonsevere COVID-19 urgently need to be identified.
According to the Novel Coronavirus Pneumonia Diagnosis and Treatment Plan (Trial Version 6), issued by the National Health Commission of the People' s Republic of China, and the study conducted by Li et al., the progressive declination of peripheral blood lymphocytes in adults with COVID-19 is an early warning sign of progression from mild to severe disease (17,18). Furthermore, clinical studies conducted by Lippi and Zhang et al. showed that the levels of hemoglobin (HGB) declined in COVID-19 patients who progressed to severe disease (19,20). However, evidence to support the direct use of the percentage of lymphocytes (Lym%) and HGB for guiding the diagnosis and treatment of COVID-19 is insufficient (18,21). We hypothesized that a joint parameter obtained by integrating Lym% and HGB could be used to predict progression to severe COVID-19, with the potential to improve the efficiency of diagnosis and treatment in patients with severe disease. Lymphocyte count and peripheral blood HGB concentration can be obtained from routine blood tests. Complete blood count (CBC) is the most effective, economical, and commonly used test in clinical laboratories. The ability of the joint parameter to provide accurate information about disease progression and outcomes in COVID-19 patients would support its future use by clinicians in identifying patients with severe disease and would enable appropriate and timely measures to be taken.
In this study, two parameters, Lym% and HGB, as well as the joint parameter Lym% & HGB, in the peripheral blood of patients with severe and nonsevere COVID-19 were compared in order to assess the effectiveness of these parameters in differentiating between severe and nonsevere COVID-19 for guiding clinical treatment. We present the following article in accordance with the STARD reporting checklist (available at http://dx.doi.org/10.21037/atm-20-6001).
Methods
Patients
This retrospective analysis involved 159 COVID-19 patients who were admitted to The Third People’s Hospital of Shenzhen, China between January 23, 2020, and March 21, 2020. The patients were clinically diagnosed and classified according to the Novel Coronavirus Pneumonia Diagnosis and Treatment Plan (Trial Version 6), issued by the National Health Commission of the People’s Republic of China.
The clinical classifications of COVID-19 are as follows: (I) mild: mild clinical symptoms, with no manifestations of pneumonia observed by imaging. (II) Common: clinical symptoms, including fever and respiratory symptoms, and manifestations of pneumonia observed by imaging. (III) Severe: any of the following manifestations: (i) shortness of breath and a respiratory rate (RR) ≥30 times/minute; (ii) oxygen saturation at rest ≤93%; (iii) arterial partial oxygen pressure (PaO2)/oxygen absorption concentration (FiO2) ≤300 mmHg (1 mmHg =0.133 kPa); and (iv) lung imaging showing a >50% increase in lesions within 24–48 hours. (IV) Critically severe: any of the following conditions: (i) respiratory failure requiring mechanical ventilation; (ii) shock; or (iii) other organ failure, requiring ICU monitoring and treatment.
To simplify the statistical analysis and for intergroup comparison, the nonsevere patient group comprised 101 patients with mild or common COVID-19, and the severe patient group comprised 58 patients with severe or critically severe COVID-19.
All procedures performed in this study involving human participants were in accordance with the Declaration of Helsinki (as revised in 2013). This study was approved by the ethics committee of The Third People’s Hospital of Shenzhen. Reference Number is “2020-166”. Due to the study’s retrospective nature, the requirement to obtain signed informed consent from the patients was waived.
Data collection
The electronic medical records of COVID-19 patients were retrieved from the hospital information system (HIS). Data related to the patients’ epidemical characteristics, medical history, clinical manifestations, diagnosis and treatment, imaging findings, and laboratory test results were collected. Respiratory tract swabs, including samples from the upper respiratory tract, throat, and alveolar lavage fluid, were collected from patients during hospital admission and sent to Shenzhen Center for Disease Control and Prevention for laboratory testing. During hospitalization, the patients in our study underwent 1,503 CBC tests in total. The CBC data were divided into two groups according to the real-time disease condition and recovery status of the patients: the nonsevere sample group (662 tests) and the severe sample group (841 tests).
Statistical methods
Ages and number of days were expressed as medians and ranges. Categorical variables were expressed as absolute numbers and percentages. Chi-square and Fisher’s exact tests were performed for comparisons between two groups. The Kolmogorov-Smirnov test was used to test the normal distribution of continuous variables. Data with a normal distribution were expressed as the mean and standard deviation (SD) and comparisons between two groups were made using Student’s t-tests. Data with non-normal distribution were expressed as the median and interquartile range (IQR value) and comparisons between two groups were made using the Mann-Whitney U test. A two-sided P value <0.05 was considered to be statistically significant. The two parameters, Lym% and HGB, were integrated into a joint parameter, Lym% & HGB, for the differential diagnosis of nonsevere and severe COVID-19 through logistic regression. To evaluate the effectiveness of these parameters for estimating the severity of COVID-19, a receiver operating characteristic curve (ROC) was plotted and the area under the ROC (AUC) was calculated. The optimal cutoff value for each parameter was determined based on the Youden’s index and scenarios of clinical use, and then the sensitivity and specificity of Lym%, HGB, and Lym% & HGB were compared. Statistical analyses were performed with SPSS statistical software V21.0 (IBM, USA) was used for analysis, and GraphPad Prism V8.0 (GraphPad Software, USA) was used to plot graphs.
Results
Patient epidemiological and clinical characteristics
This study enrolled 159 COVID-19 patients, including 101 (63.5%) in the nonsevere patient group and 58 (36.5%) in the severe patient group. The enrolled patients had a median age of 53 (range, 37–63) years; the median age of the patients in the severe group was 63 (range, 54–66) years, which was significantly higher than that of the patients in the nonsevere group [47 (range, 34–58) years; P<0.01]. Eighty-two (51.6%) of the COVID-19 patients were male, and 77 (48.4%) were female. Of the patients, 129 patients (81.1%) had travelled to Wuhan within the previous 2 weeks, while 25 (15.7%) had a contact history with suspected COVID-19 patients and 36 (22.6%) had a contact history with confirmed COVID-19 patients. In terms of clinical symptoms, 115 patients (72.3%) had fever, 62 (39.0%) had cough, 12 (7.5%) had myalgia, and 12 (7.5%) had pharyngeal redness or pharyngalgia. The proportion of patients in the severe group with fever and dry cough was higher than that in the nonsevere group, and the differences in the incidences of fever and dry cough between the two groups were statistically significant (P<0.05). No significant differences existed between the two groups in relation to other symptoms (P>0.05). With regard to underlying diseases, 21 (13.2%) patients had hypertension, 7 (4.4%) had diabetes mellitus, and 6 (0.6%) had cardiovascular disease, and no significant differences existed between the groups (P>0.05) (Table 1).
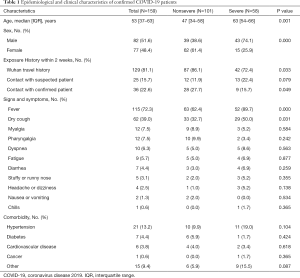
Full table
Establishment of the joint parameter Lym% & HGB by logistic regression
The joint parameter Lym% & HGB was formed by combining Lym% and HGB through binary logistic regression. With the 101 patients with nonsevere COVID-19 serving as the reference group and the 58 severe COVID-19 patients serving as the comparison group, binary logistic regression was performed to evaluate the impact of Lym% and HGB on the risk of developing severe COVID-19 (Table 2). Finally, the logistic model obtained by omnibus tests was found to be statistically significant (P<0.001), and its ability to correctly recognize the grouped data was 84.4% (1268/1503) when using a prediction probability of 0.5 as the cutoff point. The two independent variables in the model, Lym% and HGB, were statistically significant (P<0.001). For each unit Lym% decreased by, the risk of progression to severe disease increased by 17.3% (OR =0.827, 0.811–0.845), and for each unit HGB decreased by, the risk of progression increased by 4.9% (OR = 0.958, 0.951–0.965).

Full table
Differences in parameters between patients with severe and nonsevere COVID-19
Using 662 results from nonsevere patients and 841 results from severe patients, Mann-Whitney U tests were performed to compare the two-patient groups. As shown in Table 3, Lym% and HGB were significantly lower in the severe group than in the nonsevere group (P<0.001); however, Lym% & HGB in the severe group was significantly higher than that in the nonsevere group (P<0.001). The boxplots in Figure 1A,B,C show the differences in these parameters between the severe and nonsevere groups.

Full table
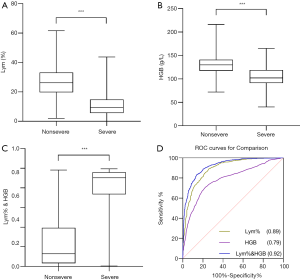
Subsequently, ROC analysis was performed, and the AUC was calculated to evaluate the effectiveness of these parameters for differentiating severe from nonsevere COVID-19 patients. The optimal cutoff value was determined based on the Youden’s index (sensitivity + specificity-1) and scenarios of clinical use, and then diagnostic sensitivity and specificity were calculated (Figure 1D). When Lym%, HGB, and Lym% & HGB were used to identify patients with severe COVID-19, the AUCs were 0.89, 0.79, and 0.92, respectively. When the cutoff values for Lym%, HGB, and Lym% & HGB were 18.8%, 116 g/L, and 0.481, respectively, the sensitivity rates were 85.6%, 71.1%, and 88.9%, and the specificity rates were 77.5%, 77.2% and 79.8%, respectively (Table 4). A joint parameter ≥0.481 (0.481 was the optimal cutoff point) indicated a high risk of progression to severe COVID-19. The AUC results suggested that the diagnostic effectiveness of the joint parameter Lym% & HGB was superior to those of the single parameters.

Full table
Figure 2 shows the two-dimensional scatter plot of Lym% and HGB for the two patient groups, clearly demonstrating the combination of Lym% and HGB to be highly effective. The data points for patients with severe COVID-19 are mainly distributed in the lower left area of the figure and can be clearly distinguished from the data points for patients with nonsevere COVID-19 in the upper right area. The dashed line represents the optimal cutoff value for Lym% & HGB obtained by ROC analysis. The data points for patients with severe and nonsevere COVID-19 are mostly distributed on opposite sides of the dashed line, indicating that Lym% & HGB, obtained by integrating the two individual parameters, can serve as an effective tool for differentiating severe from nonsevere COVID-19.
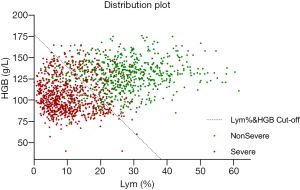
Dynamic profiles of the three parameters over time in COVID-19 patients
To explore the relationships between the three parameters (Lym%, HGB, and Lym% & HGB) and disease progression, dynamic change curves were created using the number of days after disease onset (i.e., the date when the patient first reported fever, dry cough, dyspnea, chest tightness, or other symptoms) as the horizontal axis and the median parameter value for each group as the vertical axis. The change patterns and trends for the three parameters in patients with severe and nonsevere COVID-19 were analyzed. As Figure 3A shows, Lym% was significantly lower in patients with severe COVID-19 than in patients with nonsevere COVID-19 throughout the disease course, and the median Lym% in patients with severe COVID-19 was lower than the cutoff level of 18.8% on day 4 after disease onset, indicating progression to severe disease. Additionally, HGB declined progressively from the end of the second week, decreasing by 24%, from 133 to 100 g/L, and in the third week, the median value was lower than the cutoff point of 116 g/L, which indicated disease progression, likely to the severe stage (Figure 3B). HGB was significantly lower in the severe group than in the nonsevere group throughout the disease course. The joint parameter showed an increasing trend, with disease progression in both groups, and it was significantly higher in the severe group than in the nonsevere group throughout the disease course (Figure 3C).
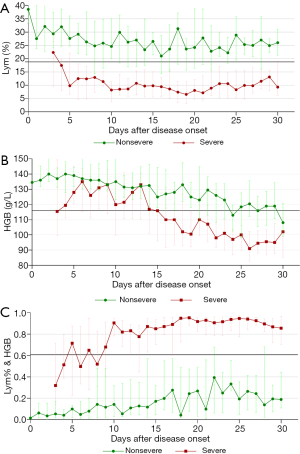
Discussion
Currently, the most difficult challenge in treating patients and saving lives amid the COVID-19 pandemic is the extreme shortage of medical resources, especially critical care resources. Therefore, differentiating between severe and nonsevere COVID-19 is crucial to providing the appropriate treatment for patients in different conditions (18,20,21). The rational allocation of medical resources is an important means of improving the efficiency of diagnosing and treating COVID-19 and reducing patient mortality. The use of routine blood testing, an economical and simple-to-operate tool with a short turnaround time, to determine disease severity can considerably accelerate the pace and reduce the cost of COVID-19 diagnosis and treatment. The proposed approach uses common laboratory parameters to assist clinicians with the preliminarily classification of COVID-19 patients and the proper allocation of medical resources, thus ensuring that patients with early-stage severe COVID-19 can be treated in a timely manner.
Consistent with previous reports, our investigation of the epidemiology and clinical symptoms of COVID-19 revealed that patients with severe disease were older than those with nonsevere disease. This finding may be attributable to the weakening of the body’s defense system, caused by the declination of immune function or the presence of underlying diseases, such as hypertension, chronic renal failure, and diabetes mellitus, in elderly patients (6,7,8,12). Therefore, to avoid missing the optimal treatment time, clinicians should closely monitor the disease progression of middle-aged and elderly patients. Furthermore, 72.3% of the 159 patients in our study developed clinical symptoms, including fever, with severe COVID-19 patients more likely to develop fever than those with nonsevere COVID-19.
In this study, two parameters, Lym% and HGB, were integrated to form a joint parameter, Lym% & HGB, through binary logistic regression. Both Lym% and HGB were statistically significant when used as independent variables in the model (P<0.001, Table 2). This finding suggests that both parameters have a significant impact on the risk of developing severe COVID-19. Meanwhile, Lym% & HGB had an accuracy of 84.4% in identifying the data of different groups, suggesting the great potential of this parameter in the differential diagnosis of severe and nonsevere COVID-19.
The grouping analysis of the results from 1503 routine blood tests found that Lym% and HGB continued to decline and Lym% & HGB continued to rise along with disease progression in COVID-19 patients. The data from the blood tests were divided into the severe sample group and the nonsevere sample group and were subjected to Mann–Whitney U nonparametric tests. Lym% and HGB were significantly lower, while Lym% & HGB was significantly higher, in the severe sample group compared to the nonsevere sample group (P<0.001). This indicated that the number of lymphocytes and the concentration of HGB gradually decreased with disease progression. Therefore, Lym%, HGB, and Lym% & HGB are all potential tools for distinguishing severe from nonsevere COVID-19.
Subsequently, ROC analysis was conducted to assess the diagnostic performance of the three parameters in identifying patients with severe or nonsevere COVID-19. The results showed both Lym% and HGB to be good predictors, as evidenced by AUCs of 0.89 and 0.79, respectively. When 18.8% and 116 g/L were used as the cutoff points for Lym% and HGB, respectively, the sensitivity rates were 85.6% and 71.1%, and the specificity rates were 77.5% and 77.2%, respectively. Moreover, the AUC for Lym% & HGB was 0.92. When 0.481 was used as the cutoff point for Lym% & HGB, the sensitivity and specificity were 88.9% and 79.8%, respectively, suggesting that Lym% & HGB has advantages in distinguishing patients with severe COVID-19 from those with nonsevere COVID-19.
To more clearly present the effectiveness of Lym% & HGB in distinguishing patients with severe COVID-19 from those with nonsevere COVID-19, a two-dimensional scatter plot of the results from the 1503 blood tests was generated, with Lym% as the horizontal axis and HGB as the vertical axis. As shown in Figure 2, the data points for patients with severe disease are scattered mostly below the cutoff for Lym% & HGB, while those for patients with nonsevere disease are mostly above the line, indicating that the joint parameter is superior to the single parameters of Lym% and HBG in distinguishing patients with severe disease from those with nonsevere disease.
The dynamic profile demonstrated that Lym% was significantly lower in the severe patient group than that in the nonsevere patient group, as well as that the median Lym% in the severe patient group began to drop below the cutoff point of 18.8% on day 4 after disease onset, suggesting a high possibility of progression to severe disease. Similarly, HGB declined progressively from the end of the second week after disease onset, and the median fell below the cutoff point of 116 g/L in the third week, showing a decrease of 24%, from 133 to 100 g/L. This indicated that the disease was likely to progress to a severe stage. However, Lym% & HGB showed an opposite change trend to those of Lym% and HGB. Compared with patients with nonsevere COVID-19, whose Lym% & HGB slightly fluctuated and increased, patients with severe COVID-19 had a higher Lym% & HGB level, above the cutoff point of 0.481, throughout the course of their disease. This observation could help clinicians to identify patients with severe disease more easily.
Lymphocytes play a decisive role in maintaining systemic immune balance and in regulating the body’s inflammatory response. Currently, there are four possible explanations for the decrease in the number of lymphocytes caused by novel coronavirus infection. Firstly, the virus directly attacks and kills lymphocytes. In the early stage of infection, B lymphocytes produce antibodies that directly bind to and kill the virus, and T lymphocytes engulf the virus-infected cells, thereby clearing the virus. Therefore, the reduction in lymphocytes in COVID-19 patients may be attributable to the massive consumption of lymphocytes (22-26). Secondly, the virus may directly destroy lymphatic organs. The attack on lymphatic organs, including the thymus and spleen, by the novel coronavirus affects lymphocyte production, resulting in a drastic decline in the number of lymphocytes. An autopsy report published by Hanley supports this view (27). In previous reports, SARS and MERS patients showed similar changes, with their lymphatic organs attacked or even destroyed as their disease progressed (6,28-30). Thirdly, inflammatory factors induce lymphocyte apoptosis. Basic research has confirmed that tumor necrosis factor (TNF)-α, interleukin 6 (IL-6), and other pro-inflammatory cytokines can induce lymphocyte apoptosis, leading to an acute decrease in the number of lymphocytes (31). Fourthly, lymphocytes are inhibited by the metabolic molecules produced in metabolic diseases, such as hyperlactic acidemia. In severe COVID-19 patients, a continuous increase in the levels of blood lactic acid may inhibit the proliferation of lymphocytes (32). The abovementioned mechanisms may jointly cause lymphopenia; however, this needs to be verified by further research. The significant change in HGB may be explained by the fact that the virus adheres to the surface of hematopoietic cells through the angiotensin-converting enzyme (ACE) 2 receptor (26) and enters the hematopoietic system. The substances released by the virus, viremia, and endotoxins jointly influence the release of immune factors and immune regulatory function, affect hematopoietic stem/progenitor cells, and lead to an abnormal hematopoietic microenvironment; thereby, the hematopoietic function of bone marrow is inhibited. This ultimately affects the compensatory production of HGB, causing a continuous decrease in HGB and even hematopoietic failure or aplastic anemia (33). Among the 99 COVID-19 patients admitted to Wuhan Jinyintan Hospital in China, 51% experienced a decrease in HGB (6), which is consistent with the finding in the present study. In this study, two parameters, Lym% and HGB, were linearly integrated into a joint parameter, Lym% & HGB, using binary logistic regression.
Conclusions
The new parameter, Lym% & HGB, which is associated with the production of peripheral blood leucocytes and erythrocytes, can reflect both immune function and the overall nutritional status of the body. Therefore, it may serve as a superior indicator of disease severity in COVID-19 patients. The findings of this single-center retrospective study, which was based on the routine blood test data of 159 COVID-19 patients in our hospital, need to be verified by further investigations at other centers and with larger sample sizes.
Acknowledgments
We would like to thank all of the patients who participated in this study, as well as the healthcare workers at our hospital for their efforts in caring for these patients. Thank you to all of the people are working hard in the fight against COVID-19.
Funding: This work was supported by Shenzhen Key Laboratory of Biochip (ZDSYS201504301534057) and Special Support Fund of Shenzhen for Introduced High-Level Medical Team, Guangdong Basic and Applied Basic Research Foundation (2020A1515010586), Science and Technology program of Shenzhen (JCYJ20190809144005609), and a grant from the Bill & Melinda Gates Foundation. The funders provided financial support for the labor, communication, and reagent purchasing fees arising from the research.
Footnote
Reporting Checklist: The authors have completed the STARD reporting checklist. Available at http://dx.doi.org/10.21037/atm-20-6001
Data Sharing Statement: Available at http://dx.doi.org/10.21037/atm-20-6001
Conflicts of Interest: All authors have completed the ICMJE uniform disclosure form (available at http://dx.doi.org/10.21037/atm-20-6001). The authors have no conflicts of interest to declare.
Ethical Statement: The authors are accountable for all aspects of the work in ensuring that questions related to the accuracy or integrity of any part of the work are appropriately investigated and resolved. All procedures performed in this study involving human participants were in accordance with the Declaration of Helsinki (as revised in 2013). This study was approved by the Ethics Committee of The Third People’s Hospital of Shenzhen China. Reference Number is “2020-166”. The requirement to obtain signed informed consent from the patients was waived due to the study’s retrospective nature.
Open Access Statement: This is an Open Access article distributed in accordance with the Creative Commons Attribution-NonCommercial-NoDerivs 4.0 International License (CC BY-NC-ND 4.0), which permits the non-commercial replication and distribution of the article with the strict proviso that no changes or edits are made and the original work is properly cited (including links to both the formal publication through the relevant DOI and the license). See: https://creativecommons.org/licenses/by-nc-nd/4.0/.
References
- Zhu N, Zhang D, Wang W, et al. A novel coronavirus from patients with Pneumonia in China, 2019. A Novel Coronavirus from Patients with Pneumonia in China, 2019. N Engl J Med 2020;382:727-33. [Crossref] [PubMed]
- Lu H, Stratton CW, Tang YW. Outbreak of pneumonia of unknown etiology in Wuhan, China: The mystery and the miracle. J Med Virol 2020;92:401-2. [Crossref] [PubMed]
- Bogoch II, Watts A, Thomas-Bachli A, et al. Potential for global spread of a novel coronavirus from China. J Travel Med 2020;27:taaa011.
- WHO. Coronavirus disease (COVID-2019) situation reports. August 7, 2020. Available online: https://www.who.int/emergencies/diseases/novel-coronavirus-2019/situation-reports
- WHO. Novel coronavirus - China. Jan 12, 2020. Available online: (accessed Feb 19, 2020).http://www.who.int/csr/don/12-january-2020-novel-coronavirus-china/en/
- Chen N, Zhou M, Dong X, et al. Epidemiological and clinical characteristics of 99 cases of 2019 novel coronavirus pneumonia in Wuhan, China: a descriptive study. Lancet 2020;395:507-13. [Crossref] [PubMed]
- Huang C, Wang Y, Li X, et al. Clinical features of patients with 2019 novel coronavirus in Wuhan, China. Lancet 2020;395:497-506. [Crossref] [PubMed]
- Lu R, Zhao X, Li J, et al. Genomic characterization and epidemiology of 2019 novel coronavirus: implications of virus origins and receptor binding. Lancet 2020;395:565-74. [Crossref] [PubMed]
- Wang D, Hu B, Hu C, et al. Clinical Characteristics of 138 Hospitalized Patients With 2019 Novel Coronavirus-Infected Pneumonia in Wuhan, China. JAMA 2020;323:1061-9. [Crossref] [PubMed]
- Chen J, Qi TK, Liu L, et al. Clinical progression of patients with COVID-19 in Shanghai, China. J Infect 2020;80:e1-e6. [Crossref] [PubMed]
- Fu L, Fei J, Xiang HX, et al. Influence factors of death risk among COVID-19 patients in Wuhan, China: a hospital-based case-cohort study. medRxiv 2020. doi: 10.1101/2020.03.13.20035329. [Crossref]
- Gao QJ, Hu YF, Dai ZG, et al. The epidemiological characteristics of 2019 novel coronavirus diseases (COVID-19) in Jingmen, Hubei, China. medRxiv 2020. doi: 10.1101/2020.03.07.20031393. [Crossref]
- Wu YJ, Guo W, Liu H, et al. Clinical outcomes of 402 patients with COVID-2019 from a single center in Wuhan, China. medRxiv 2020. doi: 10.1101/2020.03.07.20032672. [Crossref]
- Yang Y, Peng F, Wang R, et al. The deadly coronaviruses: The 2003 SARS pandemic and the 2020 novel coronavirus epidemic in China. J Autoimmun 2020;109:102434. [Crossref] [PubMed]
- Gralinski LE, Menachery VD. Return of the Coronavirus: 2019-nCoV. Viruses. 2020;12:135. [Crossref] [PubMed]
- Dehkordi AH, Alizadeh M, Derakhshan M, et al. Understanding Epidemic Data and Statistics: A case study of COVID-19. medRxiv 2020. doi: 10.1101/2020.03.15.20036418. [Crossref]
- General Office of National Health Commission, Office of the State Administration of Traditional Chinese Medicine. New Coronavirus Pneumonia Diagnosis and Treatment Plan (Trial Version 6). Feb 18, 2020. National Health Office Medical Letter [2020] 145. Available online: http://www.nhc.gov.cn/yzygj/s7653p/202002/8334a8326dd94d329df351d7da8aefc2/files /b218cfeb1bc54639af227f922bf6b817.pdf
- Li T, Qi W, Zhang D, et al. Lymphopenia predicts disease severity of COVID-19: a descriptive and predictive study. medRxiv 2020. doi: 10.1101/2020.03.01.20029074. [Crossref]
- Lippi G, Mattiuzzi C. Hemoglobin value may be decreased in patients with severe coronavirus disease 2019. Hematol Transfus Cell Ther 2020;42:116-7. [Crossref] [PubMed]
- Zhang HZ, Wang XY, Fu ZQ. Potential Factors for Prediction of Disease Severity of COVID-19 Patients. medRxic 2020. doi . [Crossref]
- Nie S, Zhao XQ, Zhao K, et al. Metabolic disturbances and inflammatory dysfunction predict severity of coronavirus disease 2019 (COVID-19): a retrospective study. medRxic 2020. doi: 10.1101/2020.03.24.20042283. [Crossref]
- Yang X, Yu Y, Xu JQ, et al. Clinical course and outcomes of critically ill patients with SARS-CoV-2 pneumonia in Wuhan, China: a single-centered, retrospective, observational study. Lancet Respir Med 2020;8:475-81. [Crossref] [PubMed]
- Ruan Q, Yang K, Wang W, et al. Clinical predictors of mortality due to COVID-19 based on an analysis of data of 150 patients from Wuhan. Intensive Care Med 2020;46:846-8. [Crossref] [PubMed]
- Bizzarro MJ, Conrad SA, Kaufman DA, et al. Infections acquired during extracorporeal membrane oxygenation in neonates, children, and adults. Pediatr Crit Care Med 2011;12:277-81. [Crossref] [PubMed]
- Henry BM. COVID-19, ECMO, and lymphopenia: a word of caution. Lancet Respir Med 2020;8:e24. [Crossref] [PubMed]
- Xu H, Zhong L, Deng J, et al. High expression of ACE2 receptor of 2019-nCoV on the epithelial cells of oral mucosa. Int J Oral Sci 2020;12:8. [Crossref] [PubMed]
- Hanley B, Lucas SB, Youd E, et al. Autopsy in suspected COVID-19 cases. J Clin Pathol 2020;73:239-42. [Crossref] [PubMed]
- Yang M, Li CK, Li K, et al. Hematological findings in SARS patients and possible mechanisms Int J Mol Med 2004;14:311-5. (review). [Crossref] [PubMed]
- Alraddadi B, Bawareth N, Omar H, et al. 766Clinical Features and Outcome of Patients with Middle East Respiratory Syndrome-Coronavirus (MERS-CoV) Infection. Open Forum Infect Dis 2014;1:S216. [Crossref]
- Xu P, Sun GD, Li ZZ. Clinical Characteristics of Two Human to Human Transmitted Coronaviruses: Corona Virus Disease 2019 versus Middle East Respiratory Syndrome Coronavirus. medRxiv 2020. doi: 10.1101/2020.03.08.20032821. [Crossref]
- Liao YC, Liang WG, Chen FW, et al. IL-19 induces production of IL-6 and TNF-alpha and results in cell apoptosis through TNF-alpha. J Immunol 2002;169:4288-97. [Crossref] [PubMed]
- Fischer K, Hoffmann P, Voelkl S, et al. Inhibitory effect of tumor cell-derived lactic acid on human T cells. Blood 2007;109:3812-9. [Crossref] [PubMed]
- Wu T, Kang S, Feng W, et al. A case study of COVID-19 infected patient combined with aplastic anemia. Chinese Journal of Hematology 2020;41:253-2727.
(English Language Editor: J. Reynolds)