A novel workflow for cancer blood biomarker identification
Introduction
To achieve the best prognosis for cancer patients, one of the most important things is to ensure early detection of the tumor. For most malignancies, early detection of small localized malignant tissues can allow high curative rate by surgical resection or other treatments such as radiotherapy and chemotherapy (1-4). Thus, an array of cancer screening tests were developed for populations with different medical needs. For example, there were prostate specific antigen (PSA) testing in older men, electronic colonoscope examination for colorectal cancer and gastroscopy for gastric carcinoma (5-7). Among the currently available screening methods, LB has received a large amount of attention because of its broad application prospects (8-11). For example, LB has the potential to allow screening for a variety of tumor types in a large group of individuals at risk of developing cancer (12-15).
Over the past few years, LB has been developed as a new diagnostic and prognostic concept predicated on the analysis of circulating tumor cells (CTCs), circulating cell free tumor DNA (ctDNA) and other circulating tumor-derived factors, such as exosomes (16). By using LB, it is becoming easier and easier for detecting actionable mutations, prognosticating outcomes, monitoring treatment response and detecting early recurrence. Though this application is challenged by key technologies in the face of the very low concentrations of CTCs and ctDNA in blood samples (17). Thus, sufficient plasma volumes and highly sensitive methods are needed to interrogate minute amounts of analytes.
For early detection of cancers where the location of the primary tumor is unknown, identification of the origin tissue of tumor to determine the anatomical location of the primary tumor is of significance to guide medical intervention such as diagnostic imaging and tissue biopsy. In view of the fact that tumor cells rooting in particular sites exhibit specific gene expression patterns induced by the crosstalk with the microenvironment. And the cross-communication between tumor cells and blood composition plays a role in tumor progression (18-23). We can reasonably infer that the feature of different primary tumor types can be partly reflected by the characteristics of their corresponding blood samples. For example, it was reported that in the process of tumor-platelets education, tumor cells can directly promote the protein synthesis of platelet and stimulate some relevant signaling pathways (24). In turn, platelets can influence tumor cells through releasing RNA signaling complexes in the form of platelet-derived microparticles (25,26). Thus, the crosstalk between platelets and tumor cells plays an important role in tumor progression.
Despite such advances, few available blood biomarkers can be used in clinical practice until now. However, detecting several specific blood biomarkers can only cover a small range of tumor types while monitoring of CTCs and ctDNA for cancer patients was often done during post-surgical follow-up period (27). Moreover, blood biomarkers which were found based on data-driven methods may only be effective in few datasets. Whether the gene expression characteristic of blood samples can be used as the reliable predictive index for early tumorigenesis of specific tumor was now largely unknown. Here, we described a novel cancer blood biomarker identification workflow, blood biomarker inference (BB infer), for cancer screening and diagnosis. Moreover, we demonstrated the utility of this method in 13 datasets of blood samples from solid tumor patients, including 8 types of cancers. Different from traditional data-driven methods, our method still achieves good results without using these datasets for training.
Methods
Data collection
GTEx RNAseq data in transcripts per million reads (TPM) were downloaded from GTEx portal (https://www.gtexportal.org/). TCGA RNAseq gene expression data and relevant clinical information were obtained from the GDC data portal (https://portal.gdc.cancer.gov/) and the values were transformed to TPM. In addition, 13 blood or peripheral blood mononuclear cells (PBMC) gene expression profiling datasets of solid tumor patients (GSE11545, GSE12771, GSE16443, GSE20189, GSE31682, GSE34465, GSE37199, GSE37582, GSE43519, GSE45705, GSE49641, GSE74629, GSE96597) were available at GEO datasets (https://www.ncbi.nlm.nih.gov/gds/). Duplicate genes were dropped except their max values. The study was conducted in accordance with the Declaration of Helsinki (as revised in 2013).
Blood biomarker screening
Spearman’s correlation tests were performed between blood and the other tissues/organs using GTEx gene expression data. Survival hazard genes and differential expression genes of each cancer type in TCGA were selected by Cox regression model and Wilcoxon rank sum test, respectively. Only primary tumor tissues were used for Cox regression. And the differential expression genes were calculated between tumor and normal tissues. False discovery rate (FDR) were calculated by Benjamini and Hochberg method. P values of the two screening steps were combined by multiplication while both P values <0.05. The results of each step are provided in http://fp.amegroups.cn/cms/bd3c769c4cbb9f111d848bea5c90f6ad/ATM-20-2047-1.pdf.
Pcombine = PSpearman × PCox/ranksum
Single sample gene set enrichment analysis
Single sample gene set enrichment analyses (28) (ssGSEA) were used to validate the potential blood biomarkers. After sorting the combined P values ascendingly, top 20/50 positive/negative blood biomarker gene sets for each cancer were selected and applied to ssGSEA. The gene sets were described in http://fp.amegroups.cn/cms/0f9c3342b04a4e5712de574363fcbe50/ATM-20-2047-2.pdf. The enrichment scores (ES) were processed by python (v3.6.8) package gseapy (v0.9.13).
Statistical analysis
Kaplan-Meier curves with log rank test were processed by R package survival (v 3.1-8) and survminer (v0.4.6). Significance of difference between two groups was calculated by two-side Wilcoxon rank sum test. Spearman’s correlation test, test Fisher’s exact test and log-linear model were used to evaluate the correlation between predictions and realistic outcomes. Receiver operating characteristic (ROC) curve and AUC were performed by R package pROC (29) (v1.15.3). Log-linear model were performed by SPSS 26. All other statistical analyses above were performed by R (v3.5.2). P values <0.05 were considered significant.
Results
Identification of potential positive and negative blood biomarkers
The Genotype-Tissue Expression (GTEx) project collected RNAseq data of ~17,000 non-disease samples, involving ~50 tissues, including whole blood, lung, breast, etc. Firstly, using data from GTEx, we analyzed the Spearman correlation between whole blood samples and the other tissues respectively for each gene and each tissue. As a result, the blood-non blood tissue correlated genes for each tissue were calculated. Secondly, survival hazard genes and differential expression genes of each cancer type in The Cancer Genome Atlas (TCGA) were selected by Cox regression model and Wilcoxon rank sum test, respectively (Figure 1, http://fp.amegroups.cn/cms/bd3c769c4cbb9f111d848bea5c90f6ad/ATM-20-2047-1.pdf). Only primary tumor tissues were used for Cox regression. And the differential expression genes were calculated between tumor and normal tissues. For example, we selected ovary cancer to test our workflow. We defined our inferred blood biomarkers as genes which show their significance in both correlation analysis and Cox regression analysis (FDR<0.2), including positive hazard genes and negative hazard genes (Figure 2A). For instance, Kruppel like factor 13 (KLF13) gene expression shows a significant correlation between ovary and blood. Meanwhile, Cox regression reveals that KLF13 is a significant survival risk factor in ovarian cancer. Combining these two results, KLF13 is screened out to be a potential up-regulated prognostic blood biomarker for ovarian cancer. In other words, it is reasonable to think that high expression of KLF13 in ovarian tumors means a reduced survival time, and that higher KLF13 expression may also be detected in the blood. Therefore, by measuring the expression of KLF13 in the blood, the prognosis of ovarian cancer may be evaluated.
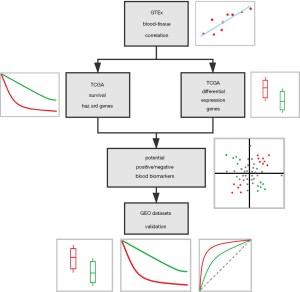
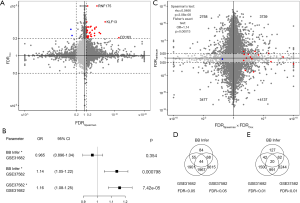
Next, to further explore the correlation between our inferred BB genes and results from real datasets, two ovarian cancer blood sample microarray datasets from the Gene Expression Omnibus (GEO) were collected (GSE31682, GSE37582). For GSE37582, the differences of blood gene expressions between ovarian cancer patients and healthy donors were calculated. Both Spearman’s correlation test and Fisher’s exact test show significant positive correlation between our BB Infer results and GSE37582 results (Figure 2C). While together with GSE31682, log-linear model again verified the correlation between our inference and the results of GSE37582 (Figure 2B, Table 1). However, the correlation between our inference and the results of GSE31682 was not significant. Furthermore, the Venn plot shows that, 167/251 of BB Infer genes were validated by at least one other dataset, which is more than the same rates of two datasets (2,066/3,967 and 2,079/5,694, respectively) (Figure 2D). This advantage was not lost when using a stricter threshold (FDR <0.01). That is, 124/251 to 1,053/2,553 and 1,073/4,317 (Figure 2E). These results demonstrated that our method is more robust than the differential expression genes method.
Differences of blood sample between solid tumor patients and healthy donors using BB Infer genes
Next, we investigated the clinical relevance of these BB Infer genes in an array of malignancies including lung cancer (including bronchial carcinoma), ovarian cancer, colorectal cancer, pancreatic ductal adenocarcinoma, prostate cancer, esophagus cancer, and renal cell carcinoma. We defined combined P values, by multiplying the P values of two steps, to assess the potential of a gene as a blood biomarker. Take the inference process of lung cancer prognostic blood biomarkers as an example, we firstly calculated the expression correlation between blood and lung for each gene using GTEx RNAseq data. For the second step, we collected the survival hazard genes of Lung squamous cell carcinoma (LUSC) by Cox regression using TCGA RNAseq data. The genes with P values ≥0.05 in any steps were excluded and the combined P values were calculated.
Besides the P values, the directions were also being considered. The genes with rho >0 in Spearman analyses and hazard ratio (HR) >1 in Cox regression, or rho <0 in Spearman analyses and HR <1 in Cox regression were considered as positive (or called: hazard, risk, up-regulated) blood biomarkers. Oppositely, the genes with rho>0 in Spearman analyses and HR <1 in Cox regression, or rho <0 in Spearman analyses and HR >1 in Cox regression were considered as negative (or called: risk-free, down-regulated) blood biomarkers.
Then we defined several gene sets. For example, the ‘Lung LUSC Cox Positive 20’ gene set means: The Spearman tests were used between blood and lung data of GTEx, and the Cox hazard genes were calculated using LUSC data of TCGA, and the positive blood biomarker genes with top 20 smallest combined P values were selected. For another example, the ‘Breast BRCA Diff. Negative 50’ gene set means: The Spearman tests were used between blood and breast data of GTEx, and the differential expression genes were calculated using breast invasive carcinoma (BRCA) data of TCGA, and the negative blood biomarker genes with top 50 smallest combined P values were selected. The gene sets used in this paper can be found in http://fp.amegroups.cn/cms/0f9c3342b04a4e5712de574363fcbe50/ATM-20-2047-2.pdf.
Next, single sample gene set enrichment analyses were performed using these gene sets, and the ES were calculated for each sample. As we can see, in all the blood samples that were analyzed, the ES of positive BB Infer genes in cancer patients are higher than healthy people. Conversely, the ES of negative BB Infer genes in cancer patients are lower than healthy people (Figure 3A,B,C,D,E,F,G,H, Figure 4A,B,C,D). These results indicated that the identified BB Infer genes have excellent clinical relevance in a variety of tumors, suggesting the extensive feasibility of our workflow for cancer blood biomarker identification.
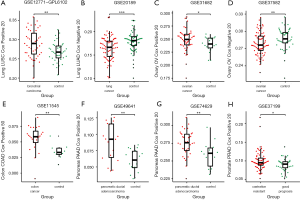
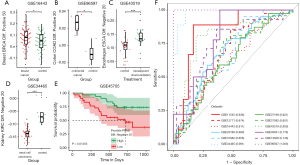
In addition, using the available prostate cancer dataset GSE37199, we performed Kaplan-Meier curve analysis (Figure 4E). Of note, lower ES of negative BB infer genes signify the dismal outcome of patients while higher ES of negative BB Infer genes signify relatively favorable prognosis, which suggested our BB Infer genes has both diagnostic value and potential prognostic value for solid tumor patients. Next, we determined the sensitivity and specificity of these BB infer genes sets in 12 different datasets which represent 8 tumor types through ROC curve. Combined with the area under of the curve (AUC), the expression level of BB Infer genes showed reliable predictive value in various malignancies (Figure 4F). In summary, these results suggest some potential usages of our BB Infer workflow and its identified genes in solid tumor screening, diagnosis and prognosis.
Discussion
Cancer is the second leading cause of death globally, and it is responsible for an estimated 9.6 million deaths in 2018 (30). Globally, about 1 in 6 deaths is due to cancer (31). To optimize cancer treatment in clinical practice, it is essential to integrate tumor molecular profiling for early detection. Currently, diagnostic strategies basically make use of tissue samples to estimate the burden of cancers (32-34). However, this method has its inherent disadvantages. For example, invasive procedures are inevitable to genotype tumor tissues while about 25% of biopsies of lung cancer can’t obtain enough tissue for further assessment (35). As the progression of cancer is a dynamic progress, the genomic profiles of cancer can also change in the course of cancer treatment. Moreover, owing to the influence of different tumor microenvironment and intrinsic molecular heterogeneity, the genomic landscape of primary tumors and metastases are not always concordant (36,37). Thus, tissue biopsies are not enough to profile the real-time evolving feature of patients with solid tumors. Under this tricky circumstance, a procedure known as LB has been introduced as a new diagnostic concept predicated on the analysis of blood biomarkers such as CTCs and ctDNA or exosomes (38-40). As the development of this technology in the past 10 years, various body fluids were involved in this strategy (primarily blood but also cerebrospinal fluid, urine and saliva) (41). Compared with tissue biopsy, LB allow for repeat sampling for their minimally-invasive characteristic. Besides, without sampling bias, LB allow longitudinal monitoring of cancers during the process of treatment (42). Even though, LB can fail to cancer detection in some instances which can be otherwise recognized in tissue biopsies partly for the reason that there are very low concentrations of CTCs and ctDNA in body liquid samples (38). In face of these limitations, more reliable and advanced methods are need to be empoldered urgently. Based on the fact that there is close communication between tumor and blood composition, we hypothesized that the sequencing results of peripheral blood has also the potential to predict cancer. So, we designed a novel workflow, BB infer, to infer potential blood biomarkers of solid tumors.
However, cancer is essentially a genetic disease while different cancer types have different genetic landscapes, so it is necessary that each cancer type has its own molecular markers in the context of personalized, precision medicine (43). With the progress of high throughput and precise analytical methods, efforts have already been made to characterize the whole genomes of individual samples including blood samples, cancerous tissue samples and normal tissue samples for getting the optimum clinical guidance (44). For example, for accurately depicting brain tumors, cerebrospinal fluid has been exploited as a source of ctDNA (45). Previous studies had shown that the somatic alterations of brain tumors including gene mutations and copy number alterations can be comprehensively characterized in the DNA present in the cerebrospinal fluid of brain tumor patients (46). While for bladder cancer, with the use of liquid biopsy, ultra-deep sequencing of blood-derived samples provide the high degree of similarity of tissue specimen in mutational landscapes (47). Furthermore, this method can even overcome the limitation of mutational burden and tumor heterogeneity due to the detection of driver genetic mutations (48). For the tremendous potential of LB, it is critical to set up a standard system to ensure high reproducibility of the results. Among this process, standardization of laboratory procedures is key but difficult (49). Blood biomarkers which were found in one study may be not effective in other datasets. However, through clinical relevance analysis, we validated the genes identified by our workflow in a variety of tumors. The method generally performs well in the diagnosis or screening of cancers.
LB, as we know, was first putted forward as a diagnostic concept in oncology introduced approximately 10 years ago (50). Nowadays, it has various clinical applications, including early detection of cancer, risk stratification of cancer patients, early detection of cancer relapse, identification of the tissue of origin of CTCs and ctDNA and even identification of therapeutic targets and resistance mechanisms of cancer (51). Under risk stratification, the prognostic significance speculated from LB at the time of initial diagnosis was well verified in breast carcinoma and various other malignancies have exhibited similar results including lung, bladder, testicular, colorectal, and head and neck cancer (16,52-54). Here, based on the feasibility of LB and sequencing results of blood sample, we validated the prognostic value of our method in prostate cancer.
In summary, unlike the previous data-driven methods, our study developed a novel workflow for solid tumor blood biomarker inference without using blood samples’ sequencing data. After applying the genes identified by our workflow in 13 datasets of blood samples from 8 types of solid tumor patients, our method generally performs well. Revealing some potential usages of our method in blood biopsy-based solid tumor screening, diagnosis and prognosis. The feasibility of this workflow also provides a new point of view to search blood markers for other diseases.
Conclusions
Collectively, we developed a novel solid tumor BB Infer workflow for cancer screening and diagnosis. Moreover, we demonstrated the utility of this inference method in a series of blood sample datasets of solid tumor patients. These results suggested the potential value of this method in the screening, diagnosis and prognosis of cancers.
Acknowledgments
Funding: This study was supported by funds from the National Key R&D Program of China (2019YFC1316104).
Footnote
Peer Review File: Available at http://dx.doi.org/10.21037/atm-20-2047
Conflicts of Interest: All authors have completed the ICMJE uniform disclosure form (available at http://dx.doi.org/10.21037/atm-20-2047). The authors have no conflicts of interest to declare.
Ethical Statement: The authors are accountable for all aspects of the work in ensuring that questions related to the accuracy or integrity of any part of the work are appropriately investigated and resolved. The study was conducted in accordance with the Declaration of Helsinki (as revised in 2013).
Open Access Statement: This is an Open Access article distributed in accordance with the Creative Commons Attribution-NonCommercial-NoDerivs 4.0 International License (CC BY-NC-ND 4.0), which permits the non-commercial replication and distribution of the article with the strict proviso that no changes or edits are made and the original work is properly cited (including links to both the formal publication through the relevant DOI and the license). See: https://creativecommons.org/licenses/by-nc-nd/4.0/.
References
- Pisano ED. AI shows promise for breast cancer screening. Nature 2020;577:35-6. [Crossref] [PubMed]
- Omuro A, Beal K, McNeill K, et al. Multicenter Phase IB Trial of Carboxyamidotriazole Orotate and Temozolomide for Recurrent and Newly Diagnosed Glioblastoma and Other Anaplastic Gliomas. J Clin Oncol 2018;36:1702-9. [Crossref] [PubMed]
- Chow PKH, Gandhi M, Tan SB, et al. SIRveNIB: Selective Internal Radiation Therapy Versus Sorafenib in Asia-Pacific Patients With Hepatocellular Carcinoma. J Clin Oncol 2018;36:1913-21. [Crossref] [PubMed]
- Siegel RL, Miller KD, Jemal A. Cancer statistics, 2020. CA Cancer J Clin 2020;70:7-30. [Crossref] [PubMed]
- Fenton JJ, Weyrich MS, Durbin S, et al. Prostate-Specific Antigen-Based Screening for Prostate Cancer: Evidence Report and Systematic Review for the US Preventive Services Task Force. JAMA 2018;319:1914-31. [Crossref] [PubMed]
- Rutter MD, Beintaris I, Valori R, et al. World Endoscopy Organization Consensus Statements on Post-Colonoscopy and Post-Imaging Colorectal Cancer. Gastroenterology 2018;155:909-25.e3. [Crossref] [PubMed]
- Kai K, Satake M, Tokunaga O. Gastric adenocarcinoma of fundic gland type with signet-ring cell carcinoma component: A case report and review of the literature. World J Gastroenterol 2018;24:2915-20. [Crossref] [PubMed]
- van der Pol Y, Mouliere F. Toward the Early Detection of Cancer by Decoding the Epigenetic and Environmental Fingerprints of Cell-Free DNA. Cancer Cell 2019;36:350-68. [Crossref] [PubMed]
- Dong J, Chen JF, Smalley M, et al. Nanostructured Substrates for Detection and Characterization of Circulating Rare Cells: From Materials Research to Clinical Applications. Adv Mater 2020;32:e1903663. [Crossref] [PubMed]
- Comparison of cfDNA and Tissue Biopsy Shows Heterogeneity in Resistance. Cancer Discov 2019;9:1479.
- Romero D. Liquid outperforms tissue. Nat Rev Clin Oncol 2019;16:660. [PubMed]
- Parikh AR, Leshchiner I, Elagina L, et al. Liquid versus tissue biopsy for detecting acquired resistance and tumor heterogeneity in gastrointestinal cancers. Nat Med 2019;25:1415-21. [Crossref] [PubMed]
- Liquid Biopsy Holds Its Own in NSCLC. Cancer Discov 2019;9:570-1.
- Keller L, Pantel K. Unravelling tumour heterogeneity by single-cell profiling of circulating tumour cells. Nat Rev Cancer 2019;19:553-67. [Crossref] [PubMed]
- Page K, Shaw JA, Guttery DS. The liquid biopsy: towards standardisation in preparation for prime time. Lancet Oncol 2019;20:758-60. [Crossref] [PubMed]
- Pantel K, Alix-Panabieres C. Liquid biopsy and minimal residual disease - latest advances and implications for cure. Nat Rev Clin Oncol 2019;16:409-24. [Crossref] [PubMed]
- Li W, Wang H, Zhao Z, et al. Emerging Nanotechnologies for Liquid Biopsy: The Detection of Circulating Tumor Cells and Extracellular Vesicles. Adv Mater 2019;31:e1805344. [Crossref] [PubMed]
- Sharonov GV, Serebrovskaya EO, Yuzhakova DV, et al. B cells, plasma cells and antibody repertoires in the tumour microenvironment. Nat Rev Immunol 2020;20:294-307. [Crossref] [PubMed]
- Sahai E, Astsaturov I, Cukierman E, et al. A framework for advancing our understanding of cancer-associated fibroblasts. Nat Rev Cancer 2020;20:174-86. [Crossref] [PubMed]
- Zahalka AH, Frenette PS. Nerves in cancer. Nat Rev Cancer 2020;20:143-57. [Crossref] [PubMed]
- Daassi D, Mahoney KM, Freeman GJ. The importance of exosomal PDL1 in tumour immune evasion. Nat Rev Immunol 2020;20:209-15. [Crossref] [PubMed]
- Fane M, Weeraratna AT. How the ageing microenvironment influences tumour progression. Nat Rev Cancer 2020;20:89-106. [Crossref] [PubMed]
- Plantureux L, Mege D, Crescence L, et al. Impacts of Cancer on Platelet Production, Activation and Education and Mechanisms of Cancer-Associated Thrombosis. Cancers (Basel) 2018;10:441. [Crossref] [PubMed]
- In ‘t Veld S, Wurdinger T. Tumor-educated platelets. Blood 2019;133:2359-64. [Crossref] [PubMed]
- Best MG, Sol N, In ‘t Veld S, et al. Swarm Intelligence-Enhanced Detection of Non-Small-Cell Lung Cancer Using Tumor-Educated Platelets. Cancer Cell 2017;32:238-52.e9.
- Best MG, Sol N, Kooi I, et al. RNA-Seq of Tumor-Educated Platelets Enables Blood-Based Pan-Cancer, Multiclass, and Molecular Pathway Cancer Diagnostics. Cancer Cell 2015;28:666-76. [Crossref] [PubMed]
- Kouba E, Lopez-Beltran A, Montironi R, et al. Liquid biopsy in the clinical management of bladder cancer: current status and future developments. Expert Rev Mol Diagn 2020;20:255-64. [Crossref] [PubMed]
- Barbie DA, Tamayo P, Boehm JS, et al. Systematic RNA interference reveals that oncogenic KRAS-driven cancers require TBK1. Nature 2009;462:108-12. [Crossref] [PubMed]
- Robin X, Turck N, Hainard A, et al. pROC: an open-source package for R and S+ to analyze and compare ROC curves. BMC Bioinformatics 2011;12:77. [Crossref] [PubMed]
- Siegel RL, Miller KD, Jemal A. Cancer statistics, 2019. CA Cancer J Clin 2019;69:7-34. [Crossref] [PubMed]
- Think globally about cancer. Nat Med 2019;25:351. [Crossref] [PubMed]
- Gerlinger M, Rowan AJ, Horswell S, et al. Intratumor heterogeneity and branched evolution revealed by multiregion sequencing. N Engl J Med 2012;366:883-92. [Crossref] [PubMed]
- Longo DL. Tumor heterogeneity and personalized medicine. N Engl J Med 2012;366:956-7. [Crossref] [PubMed]
- Kirk R. Genetics: Personalized medicine and tumour heterogeneity. Nat Rev Clin Oncol 2012;9:250. [Crossref] [PubMed]
- Pisapia P, Malapelle U, Troncone G. Liquid Biopsy and Lung Cancer. Acta Cytol 2019;63:489-96. [Crossref] [PubMed]
- Tasdogan A, Faubert B, Ramesh V, et al. Metabolic heterogeneity confers differences in melanoma metastatic potential. Nature 2020;577:115-20. [Crossref] [PubMed]
- Boire A, Brastianos PK, Garzia L, et al. Brain metastasis. Nat Rev Cancer 2020;20:4-11. [Crossref] [PubMed]
- Wan JCM, Massie C, Garcia-Corbacho J, et al. Liquid biopsies come of age: towards implementation of circulating tumour DNA. Nat Rev Cancer 2017;17:223-38. [Crossref] [PubMed]
- Anfossi S, Babayan A, Pantel K, et al. Clinical utility of circulating non-coding RNAs - an update. Nat Rev Clin Oncol 2018;15:541-63. [Crossref] [PubMed]
- Xu R, Rai A, Chen M, et al. Extracellular vesicles in cancer - implications for future improvements in cancer care. Nat Rev Clin Oncol 2018;15:617-38. [Crossref] [PubMed]
- Murillo OD, Thistlethwaite W, Rozowsky J, et al. exRNA Atlas Analysis Reveals Distinct Extracellular RNA Cargo Types and Their Carriers Present across Human Biofluids. Cell 2019;177:463-77.e15. [Crossref] [PubMed]
- Miller AM, Shah RH, Pentsova EI, et al. Tracking tumour evolution in glioma through liquid biopsies of cerebrospinal fluid. Nature 2019;565:654-8. [Crossref] [PubMed]
- Longo DL. Personalized Medicine for Primary Treatment of Serous Ovarian Cancer. N Engl J Med 2019;381:2471-4. [Crossref] [PubMed]
- Dash S, Kinney NA, Varghese RT, et al. Differentiating between cancer and normal tissue samples using multi-hit combinations of genetic mutations. Sci Rep 2019;9:1005. [Crossref] [PubMed]
- Bounajem MT, Karsy M, Jensen RL. Liquid biopsies for the diagnosis and surveillance of primary pediatric central nervous system tumors: a review for practicing neurosurgeons. Neurosurg Focus 2020;48:E8. [Crossref] [PubMed]
- Azad TD, Jin MC, Bernhardt LJ, et al. Liquid biopsy for pediatric diffuse midline glioma: a review of circulating tumor DNA and cerebrospinal fluid tumor DNA. Neurosurg Focus 2020;48:E9. [Crossref] [PubMed]
- Batista R, Vinagre N, Meireles S, et al. Biomarkers for Bladder Cancer Diagnosis and Surveillance: A Comprehensive Review. Diagnostics (Basel) 2020;10:39. [Crossref] [PubMed]
- Christensen E, Birkenkamp-Demtroder K, Sethi H, et al. Early Detection of Metastatic Relapse and Monitoring of Therapeutic Efficacy by Ultra-Deep Sequencing of Plasma Cell-Free DNA in Patients With Urothelial Bladder Carcinoma. J Clin Oncol 2019;37:1547-57. [Crossref] [PubMed]
- Siravegna G, Mussolin B, Venesio T, et al. How liquid biopsies can change clinical practice in oncology. Ann Oncol 2019;30:1580-90. [Crossref] [PubMed]
- Pantel K, Alix-Panabieres C. Circulating tumour cells in cancer patients: challenges and perspectives. Trends Mol Med 2010;16:398-406. [Crossref] [PubMed]
- Heitzer E, Haque IS, Roberts CES, et al. Current and future perspectives of liquid biopsies in genomics-driven oncology. Nat Rev Genet 2019;20:71-88. [Crossref] [PubMed]
- Riethdorf S, Muller V, Loibl S, et al. Prognostic Impact of Circulating Tumor Cells for Breast Cancer Patients Treated in the Neoadjuvant "Geparquattro" Trial. Clin Cancer Res 2017;23:5384-93. [Crossref] [PubMed]
- Yokobori T, Iinuma H, Shimamura T, et al. Plastin3 is a novel marker for circulating tumor cells undergoing the epithelial-mesenchymal transition and is associated with colorectal cancer prognosis. Cancer Res 2013;73:2059-69. [Crossref] [PubMed]
- Effenberger KE, Schroeder C, Hanssen A, et al. Improved Risk Stratification by Circulating Tumor Cell Counts in Pancreatic Cancer. Clin Cancer Res 2018;24:2844-50. [Crossref] [PubMed]