Identification and validation of key genes mediating intracranial aneurysm rupture by weighted correlation network analysis
Introduction
Intracranial aneurysm (IA) is the irreversible dilation of cerebral arteries, and the rupture of IA is the primary cause of subarachnoid hemorrhage (1,2). The prevalence of unruptured IA is about 3–5% in the general population, and about 20–30% of these patients have multiple aneurysms (3-5). The main risk factors for IA rupture include hypertension, smoking, aneurysm size, and location. Currently, endovascular coiling and surgical clipping are the two main preventative methods for IA rupture (6). Although widely used, the overall morbidity and mortality associated with these two invasive procedures are not negligible, and pharmacological therapies might provide an alternative for those who have high risks for invasive procedures (7). However, there are few pharmacological therapies available for the prevention of IA rupture (6,7). Thus, exploring the molecular mechanisms underlying the event of IA rupture and identifying the potential molecular targets for preventing IA rupture are vitally important.
Previous studies have suggested that inflammation and immune response, extracellular matrix degradation, hemodynamic stress, etc. participate in the pathogenesis and rupture of IA (8-12). Although many studies have performed detailed experiments to elucidate the molecular mechanisms behind IA rupture, few studies have used a weighted correlation network analysis (WGCNA) approach based on high-throughput data (such as mRNA microarray and RNA-seq) to identify potential targets that might have a critical biological function in the mechanism of IA rupture.
The WGCNA algorithm was created by Zhang & Horvath in 2005 and was initially used to create a gene co-expression network from mRNA microarray data (13). WGCNA generates a scale-free gene co-expression network based on Pearson’s correlation matrix of genes and detects gene modules from the network generated. These gene modules can be used for further analysis, such as correlating modules with clinical traits, functional enrichment, and hub gene identification (13). Furthermore, WGCNA can be used to construct weighted correlation networks based on proteome, metabolome, and even microbiome data (14,15). The ‘WGCNA’ R package was available on the R official website (https://cran.r-project.org/) (16).
Using WGCNA based on two GEO datasets, Zheng et al. identified FOS, CCL2, COL4A, and CXCL5 as crucial genes mediating the pathogenesis of IA, and Liao et al. have conducted a similar analysis using another GEO dataset (17,18). Alongside crucial genes, Bo et al. identified several crucial miRNAs as mediators of IA pathogenesis (19). Additionally, Landry et al. combined WGCNA and population-specific gene expression analysis (PSEA) to analyze the microenvironment associated with ruptured IA (20). Although WGCNA has partially elucidated the pathogenesis of IA, the crucial genes mediating the rupture of IA require exploration.
In our study, we focused on key genes underlying the molecular mechanism of IA rupture. We included 3 mRNA microarray datasets from the Gene Expression Omnibus (GEO) database (http://www.ncbi.nlm.nih.gov/geo/). Expression profiling data of unruptured IA and ruptured IA were used to construct the co-expression network. The gene modules were detected, and the genes in each module were subjected to functional enrichment analysis. Differentially expressed genes (DEGs) were mapped into the co-expression network and constructed into a DEG co-expression network. Key genes were identified based on this DEG co-expression network, and gene set enrichment analysis (GSEA) was conducted for them. Finally, the key genes were further validated by using another GEO dataset, and the potential diagnostic value of these key genes was determined. We present the following article in accordance with the MDAR reporting checklist (available at http://dx.doi.org/10.21037/atm-20-4083).
Methods
Medical ethics
The study was conducted in accordance with the Declaration of Helsinki (as revised in 2013). The raw datasets were available from the GEO database (http://www.ncbi.nlm.nih.gov/geo/; GSE13353, GSE15629, GSE54083 and GSE122897). In our study, neither human trials nor animal experiments were executed.
Data collection
The datasets GSE13353, GSE15629, and GSE54083 were selected from the GEO database. Series matrix files and data tables of these microarray platforms (GPL570, GPL6244, and GPL4133, respectively) were downloaded.
Data preprocessing and DEG screening
Microarray probes in the series matrix files were annotated with official gene symbols using the data table of corresponding platforms, and gene expression matrices were obtained. Then, these gene expression matrices were merged into one matrix, and the sva R package was used to normalize the expression data from 3 different datasets. The limma R package was used to conduct the DEG analysis based on the normalized gene expression matrix. The threshold for DEG analysis was set as |log2 (fold-change)| >0.5 and P<0.05.
Weighted co-expression network construction
The WGCNA R package was used to construct the co-expression networks of ruptured IA samples and unruptured IA samples. Genes with the top 25% variance were chosen, and 27 ruptured IA samples and 19 unruptured IA samples were involved in the two WGCNA analyses, respectively. The adjacency matrices which store the information of the whole co-expression network were created based on Pearson’s correlation matrices. Topological overlap measure (TOM) matrices were obtained from adjacency matrices to detect gene modules of the co-expression networks. Based on the TOM matrices, the average linkage hierarchical clustering method was used to construct clustering dendrograms with a minimum module size of 30. Finally, similar gene modules were merged, with a threshold of 0.25.
Functional annotation of gene modules
Genes in each module were subjected to gene ontology (GO) and Kyoto Encyclopedia of Genes Genomes (KEGG) pathway analysis to understand their biological function better. The Database for Annotation, Visualization, and Integrated Discovery (DAVID) v6.8 (http://david.abcc.ncifcrf.gov/) was used for analysis. The cut-off for functional annotation was set as count >2 and P<0.05. The co-expression patterns between ruptured IA samples and unruptured IA samples were compared based on the results of the functional annotations.
Hub gene identification and key gene mining
The intramodular connectivities of the genes were calculated using the WGCNA algorithm, and the genes with the highest intramodular connectivity were identified as hub genes for each module. Next, using Cytoscape v3.7.0 (https://cytoscape.org/), DEGs were mapped into the whole co-expression network of ruptured IA samples. The unruptured IA samples and DEG co-expression networks were obtained after removing isolated nodes and isolated node pairs. The Molecular Complex Detection (MCODE), a plugin in Cytoscape to detect densely connected regions in a given network, was used to generate the key gene cluster based on the DEG co-expression network of ruptured IA samples. Both the DEG co-expression network and hub gene cluster of ruptured IA samples were visualized.
GSEA and validation of key genes
The GSEA v4.0.3 software was used to conduct GSEA for each key gene. The c5.bp.v7.0.symbols.gmt downloaded from the GSEA official website (https://www.gsea-msigdb.org/gsea/downloads.jsp) was used as the reference gene sets. For each key gene, the IA samples were divided into two groups according to the median expression of the particular key gene to perform GSEA. Finally, another GEO dataset (GSE122897) containing RNA-seq data of ruptured IA samples and unruptured IA samples was chosen for validation of the key genes. Finally, key genes were used to conduct receiver operating characteristic (ROC) analysis to show the potential diagnostic value of these genes.
Statistical analysis
Data preprocessing, DEG screening and weighted co-expression network analysis were performed in R v3.6.2. Functional annotation analysis was performed by DAVID v6.8. Key genes were mined using MCODE in Cytoscape v3.7.0. GSEA was conducted by GSEA v4.0.3. The details of these bioinformatic analyses have been described in corresponding subsections. The potential diagnostic value of key genes was shown by ROC analysis using IBM SPSS 25.0. A P value <0.05 was considered statistically significant.
Results
Workflow
The workflow of our study is shown in Figure 1. After merging 3 GEO datasets and batch normalization (data preprocessing), we conducted DEG screening and WGCNA analysis. Gene modules were detected based on the co-expression networks. GO, and KEGG pathway analyses were conducted to compare the co-expression patterns between ruptured IA and unruptured IA. After that we created a DEG co-expression network by mapping the screened DEGs into the whole co-expression network of a ruptured IA co-expression network. MCODE was used to mine key genes based on the DEG co-expression network, and ROC analysis was used to determine the potential clinical significance of key genes. Finally, single-gene GSEA and validation of key genes were performed.
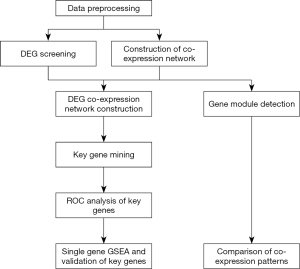
DEG screening
We screened 49 DEGs, of which 28 genes were up-regulated, and 21 genes were down-regulated in ruptured IA samples. DEGs with top-10 fold-change are shown in Table 1. The expression patterns of DEGs between ruptured IA and unruptured IA are detailed in Figure 2.
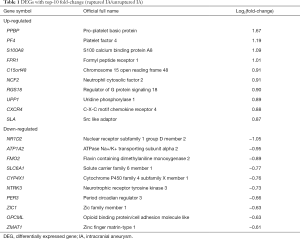
Full table
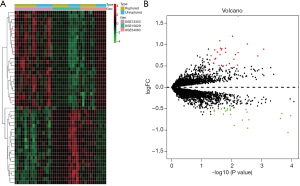
Weighted gene co-expression network construction
For the ruptured IA samples, 3 outliers (GSM337109, GSM337119, and GSM391293) were detected (Figure 3A), and for the unruptured IA samples, 1 outlier (GSM337112) was detected (Figure S1A). After removing the outliers, 24 ruptured IA samples and 18 unruptured IA samples were included in further analysis. The soft-threshold power β=16 and 18 were chosen for the ruptured IA samples and unruptured IA samples, respectively (Figure 3B,C,D,E & Figure S1B,C,D,E). Finally, we detected 8 gene modules for ruptured IA samples and 6 gene modules for unruptured IA samples (Figure 3F, Figure S1F).
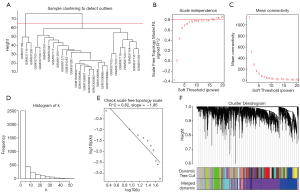
Co-expression pattern comparison
The results of GO-BP and KEGG analysis are shown in Table S1. The salmon module of ruptured IA samples was mainly associated with inflammation and immune response. The GO-BP terms and KEGG pathways with top-10 count numbers for ruptured IA samples are shown in Figure 4 and Table 2. However, these inflammation and immune-related pathways were scattered in different modules of unruptured IA samples.
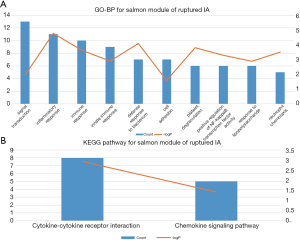
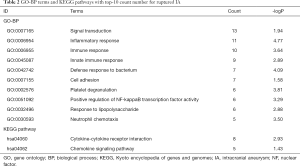
Full table
Hub gene and key gene mining
The WGCNA algorithm calculated the intramodular connectivity of genes in each module, and the gene with the highest intramodular connectivity was considered to be the hub gene of that module (Table S2). Thus, vanin 2 (VNN2), a pro-inflammatory gene, was deemed the hub gene for the salmon module of the ruptured IA group.
A DEG co-expression network with 35 nodes and 168 edges was generated by mapping DEGs into the whole co-expression network of DEGs (Figure 5A). MCODE obtained key genes based on this DEG co-expression network (Figure 5B & Table 3), of which 12 were up-regulated, and 2 were down-regulated. The gene with the highest degree in the key gene cluster was C-X-C motif chemokine receptor 4 (CXCR4).
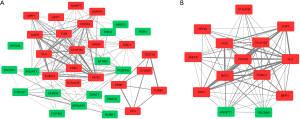
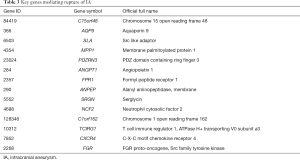
Full table
GSEA and validation of key genes
In the GSEA results for each key gene, we selected and visualized the significantly enriched gene sets with the highest normalized enrichment score (NES) value in each analysis (Figure 6). Most of the gene sets that we selected were associated with the inflammatory or immune responses, except for 3 gene sets, of which their related genes were MPP1, FPR1, and TCIRG1.
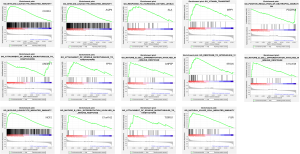
These key genes were validated using GSE122891, and the expression level of all key genes showed significant differences between the ruptured IA samples and unruptured IA samples (Figure 7 & Table S3).
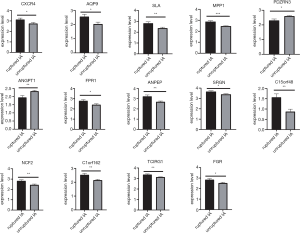
Finally, the potential clinical values of key genes were shown by ROC analysis. All the genes showed potential diagnostic values (P<0.05) (Table S4). ROC curves of key genes with the top-6 degree were visualized (Figure 8).
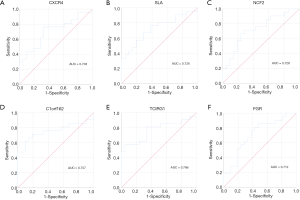
Discussion
In our research, 49 DEGs were screened, among these, 28 genes were up-regulated, and 21 genes were down-regulated in the ruptured IA samples (Figure 2 & Table 1). The weighted gene co-expression networks for both ruptured IA and unruptured IA were constructed. Based on the co-expression networks, 8 and 6 gene modules were detected for ruptured IA samples and unruptured IA samples, respectively (Figure 3, Figure S1). Genes in each module were subjected to GO and KEGG pathway enrichment analysis and co-expression patterns between ruptured IA samples. Pathways related to inflammation and immune response were clustered in the salmon module of ruptured IA samples, yet scattered in different modules of unruptured IA samples (Figure 4 & Table 2), and the hub gene of the salmon module of ruptured IA samples was VNN2, which is a pro-inflammatory gene (21). Then, a DEG co-expression network with 35 nodes and 168 edges was generated by mapping DEGs into the whole co-expression network of ruptured IA samples (Figure 5A). MCODE obtained key genes based on the DEG co-expression network (Figure 5B & Table 3). Single gene GSEA showed that the gene sets most enriched in groups divided by the expression level of key genes were associated with inflammation and immune response (Figure 6). Finally, all these key genes were validated by another GEO dataset (Figure 7 & Table S3), and they also showed potential diagnostic value (Figure 8 & Table S4).
Kleinloog summarized the DEGs that were screened by their research and other previous studies. Almost all of the key genes in our study (except PDZRN3 and ANGPT1) were following the DEGs reported by the previous studies (22-26). However, some DEGs were not overlapping with DEGs in previous studies. The difference might be the result of the datasets selected, different thresholds, and different algorithms for the DEG screening.
Previous and recent studies have indicated that inflammatory and immune response played a critical role in the rupture of IA. Using high throughput data (mRNA microarray and RNA-seq), Kleinloog, Pera, and Nakaoka et al. discovered that inflammation and immune response participated in the rupture of IA (22,24,26). Inflammatory and immune cells such as macrophages, T, and B lymphocytes were also found to be potential mediators of IA rupture. Kataoka et al. observed significantly increased macrophage infiltration in ruptured human IA (27). In lymphocyte deficient and wild-type mice models, Sawyer et al. discovered that both the formation of IA and rupture of IA were significantly fewer in lymphocyte deficient mice compared with wild-type mice (28). Also, pro-inflammatory factors have critical roles in the progression of IA rupture. A well-known pro-inflammatory cytokine, TNF-α, can cause the weakening of arterial walls through downstream pathways associated with endothelial dysfunction, vascular smooth muscle phenotypic modulation, activating pro-inflammatory cells, and other mechanisms (29,30). Another inflammatory mediator, NF-κB, participates in both the formation and rupture of IA (31,32). Our finding that the pathways associated with inflammation and immune response were clustered in the salmon module of ruptured IA samples, and our GSEA results that inflammation and immune-related gene sets were up-regulated, were consistent with these previous and recent studies.
VNN2, also named GPI-80, was the hub gene in the salmon module of ruptured IA samples. It was first discovered on human neutrophils, and it regulates neutrophil adhesion and transendothelial migration (33,34). VNN2 was also found on a subpopulation of monocytes with a superior ability of reactive oxygen species production and phagocytosis (35). Being that neutrophils and monocytes are involved in inflammation and participate in the formation and rupture of IA (12,36,37), VNN2 might have potential roles in mediating IA rupture.
One of the widely studied chemokine receptors, CXCR4, was the gene with the highest degree in the key gene cluster. CXCR4 was firstly described as a G protein coupled-receptor that mediates the fusion of HIV-1 with host cells, and CXCL12 and SDF-1 were the specific ligands for CXCR4 (38-40). The signaling pathway of CXCR4 plays various roles in essential biological processes such as vascular development, cardiogenesis, hematopoiesis, and inflammation, among others (41).
Previous studies have described the association between CXCR4 and pathophysiology of the aneurysm. In mice models with carotid or IA, Hoh et al. found that expression of CXCR4 is increased in circulating progenitor cells, which may later differentiate into inflammatory cells (42). Besides, in the abdominal aortic aneurysm (AAA), research has shown that CXCR4 mediated aneurysmal progression, possibly through the inflammatory response. Michineau et al. found that SDF-1α and CXCR4 were up-regulated in CaCl2 induced AAA mice and that the block of CXCR4 by AMD3100 inhibited AAA formation and progression. They also observed decreased infiltration of macrophages along with decreased levels of pro-inflammatory factors such as MCP-1, IL-1β, and TNF-α (43). Another group discovered that CXCR4 was highly expressed in T/B lymphocytes and macrophages in AAA, also suggesting that CXCR4 promotes AAA formation and progression through the inflammatory response (44). Kodali et al. also found that metalloproteinase-2, implicated in aneurysm formation and progression, could be induced by SDF-1 via binding to CXCR4 (45). Our results that CXCR4 was the up-regulated key gene with the highest degree and inflammatory and immune response-related gene sets were up-regulated in the CXCR4 high group were consistent with these previous studies. Furthermore, CXCR4, as well as other key genes, also showed potential diagnostic values and might serve as biomarkers to predict IA rupture.
In our study, we constructed co-expression networks for ruptured IA and unruptured IA samples using 3 GEO datasets by WGCNA, detected gene modules, and identified key genes mediating the rupture of IA for the first time. Also, we conducted a GSEA analysis for the key genes and validated the key genes we identified using another GEO dataset. However, our study had some limitations. Due to the lack of detailed information about GEO datasets, it is difficult for us to associate the gene modules and key genes with clinical traits.
Our study revealed that the co-expression pattern between ruptured IA and unruptured IA was different. These findings implicated that the inflammatory and immune response might play important roles in IA rupture. The key genes that we identified in this study might have crucial biological functions in mediating rupture of IA.
Conclusions
Using a weighted gene co-expression network approach, we identified 8 and 6 modules for ruptured IA and unruptured IA, respectively. Next, we identified the hub genes for each module and the key genes based on the DEG co-expression network. These key genes were validated by another GEO dataset (GSE122897) and might serve as potential targets for pharmacological therapies and diagnostic markers for predicting IA rupture. Further studies are needed to elucidate the detailed molecular mechanisms and biological functions of these key genes that underlie the rupture of IA.
Acknowledgments
This study used data or information from the GEO database (GSE13353, GSE15629, and GSE54083, GSE122897).
Funding: This work was supported by the Natural Science Foundation of China (81770481 and 51890894). The funders had no role in the study design, data collection, and analysis, decision to publish, or preparation of the manuscript.
Footnote
Reporting Checklist: The authors have completed the MDAR reporting checklist. Available at http://dx.doi.org/10.21037/atm-20-4083
Data Sharing Statement: Available at http://dx.doi.org/10.21037/atm-20-4083
Conflicts of Interest: All authors have completed the ICMJE uniform disclosure form (Available at http://dx.doi.org/10.21037/atm-20-4083). The authors have no conflicts of interest to declare.
Ethical Statement: The authors are accountable for all aspects of the work in ensuring that questions related to the accuracy or integrity of any part of the work are appropriately investigated and resolved. The study was conducted in accordance with the Declaration of Helsinki (as revised in 2013). This study was not involved in the experiments of humans or animals. The raw datasets were available from the GEO database (http://www.ncbi.nlm.nih.gov/geo/; GSE13353, GSE15629, GSE54083 and GSE122897).
Open Access Statement: This is an Open Access article distributed in accordance with the Creative Commons Attribution-NonCommercial-NoDerivs 4.0 International License (CC BY-NC-ND 4.0), which permits the non-commercial replication and distribution of the article with the strict proviso that no changes or edits are made and the original work is properly cited (including links to both the formal publication through the relevant DOI and the license). See: https://creativecommons.org/licenses/by-nc-nd/4.0/.
References
- Broderick JP, Brott TG, Duldner JE, et al. Initial and recurrent bleeding are the major causes of death following subarachnoid hemorrhage. Stroke 1994;25:1342-7. [Crossref] [PubMed]
- Bederson JB, Connolly ES Jr, Batjer HH, et al. Guidelines for the management of aneurysmal subarachnoid hemorrhage: a statement for healthcare professionals from a special writing group of the Stroke Council, American Heart Association. Stroke 2009;40:994-1025. [Crossref] [PubMed]
- Vernooij MW, Ikram MA, Tanghe HL, et al. Incidental findings on brain MRI in the general population. N Engl J Med 2007;357:1821-8. [Crossref] [PubMed]
- Vlak MH, Algra A, Brandenburg R, et al. Prevalence of unruptured intracranial aneurysms, with emphasis on sex, age, comorbidity, country, and time period: a systematic review and meta-analysis. Lancet Neurol 2011;10:626-36. [Crossref] [PubMed]
- Weir B. Unruptured intracranial aneurysms: a review. J Neurosurg 2002;96:3-42. [Crossref] [PubMed]
- Etminan N, Rinkel GJ. Unruptured intracranial aneurysms: development, rupture and preventive management. Nat Rev Neurol 2016;12:699-713. [Crossref] [PubMed]
- Thompson BG, Brown RD Jr, Amin-Hanjani S, et al. Guidelines for the Management of Patients With Unruptured Intracranial Aneurysms: A Guideline for Healthcare Professionals From the American Heart Association/American Stroke Association. Stroke 2015;46:2368-400. [Crossref] [PubMed]
- Pawlowska E, Szczepanska J, Wisniewski K, et al. NF-kappaB-Mediated Inflammation in the Pathogenesis of Intracranial Aneurysm and Subarachnoid Hemorrhage. Does Autophagy Play a Role? Int J Mol Sci 2018;19:1245. [Crossref] [PubMed]
- Korostynski M, Morga R, Piechota M, et al. Inflammatory Responses Induced by the Rupture of Intracranial Aneurysms Are Modulated by miRNAs. Mol Neurobiol 2020;57:988-96. [Crossref] [PubMed]
- Zhang X, Ares WJ, Taussky P, et al. Role of matrix metalloproteinases in the pathogenesis of intracranial aneurysms. Neurosurg Focus 2019;47:E4. [Crossref] [PubMed]
- Lv N, Karmonik C, Chen S, et al. Wall Enhancement, Hemodynamics, and Morphology in Unruptured Intracranial Aneurysms with High Rupture Risk. Transl Stroke Res 2020;11:882-9. [Crossref] [PubMed]
- Mitsui K, Ikedo T, Kamio Y, et al. TLR4 (Toll-Like Receptor 4) Mediates the Development of Intracranial Aneurysm Rupture. Hypertension 2020;75:468-76. [Crossref] [PubMed]
- Zhang B, Horvath S. A general framework for weighted gene co-expression network analysis. Stat Appl Genet Mol Biol 2005;4:Article17.
- Pei G, Chen L, Zhang W. WGCNA Application to Proteomic and Metabolomic Data Analysis. Methods Enzymol 2017;585:135-58. [Crossref] [PubMed]
- Maffei VJ, Kim S. Biological Aging and the Human Gut Microbiota. J Gerontol A Biol Sci Med Sci 2017;72:1474-82. [Crossref] [PubMed]
- Langfelder P, Horvath S. WGCNA: an R package for weighted correlation network analysis. BMC Bioinformatics 2008;9:559. [Crossref] [PubMed]
- Zheng X, Xue C, Luo G, et al. Identification of crucial genes in intracranial aneurysm based on weighted gene coexpression network analysis. Cancer Gene Ther 2015;22:238-45. [Crossref] [PubMed]
- Liao B, Zhou FK, Zhong SX, et al. Construction and analysis of gene co-expression networks in intracranial aneurysm. Zhonghua Yi Xue Za Zhi 2019;99:525-31. [PubMed]
- Bo L, Wei B, Wang Z, et al. Screening of Critical Genes and MicroRNAs in Blood Samples of Patients with Ruptured Intracranial Aneurysms by Bioinformatic Analysis of Gene Expression Data. Med Sci Monit 2017;23:4518-25. [Crossref] [PubMed]
- Landry AP, Balas M, Spears J, et al. Microenvironment of ruptured cerebral aneurysms discovered using data driven analysis of gene expression. PLoS One 2019;14:e0220121. [Crossref] [PubMed]
- Martinez L, Tabbara M, Duque JC, et al. Transcriptomics of Human Arteriovenous Fistula Failure: Genes Associated With Nonmaturation. Am J Kidney Dis 2019;74:73-81. [Crossref] [PubMed]
- Kleinloog R, Verweij BH, van der Vlies P, et al. RNA Sequencing Analysis of Intracranial Aneurysm Walls Reveals Involvement of Lysosomes and Immunoglobulins in Rupture. Stroke 2016;47:1286-93. [Crossref] [PubMed]
- Marchese E, Vignati A, Albanese A, et al. Comparative evaluation of genome-wide gene expression profiles in ruptured and unruptured human intracranial aneurysms. J Biol Regul Homeost Agents 2010;24:185-95. [PubMed]
- Pera J, Korostynski M, Krzyszkowski T, et al. Gene expression profiles in human ruptured and unruptured intracranial aneurysms: what is the role of inflammation? Stroke 2010;41:224-31. [Crossref] [PubMed]
- Kurki MI, Hakkinen SK, Frosen J, et al. Upregulated signaling pathways in ruptured human saccular intracranial aneurysm wall: an emerging regulative role of Toll-like receptor signaling and nuclear factor-kappaB, hypoxia-inducible factor-1A, and ETS transcription factors. Neurosurgery 2011;68:1667-75; discussion 75-6. [Crossref] [PubMed]
- Nakaoka H, Tajima A, Yoneyama T, et al. Gene expression profiling reveals distinct molecular signatures associated with the rupture of intracranial aneurysm. Stroke 2014;45:2239-45. [Crossref] [PubMed]
- Kataoka K, Taneda M, Asai T, et al. Structural fragility and inflammatory response of ruptured cerebral aneurysms. A comparative study between ruptured and unruptured cerebral aneurysms. Stroke 1999;30:1396-401. [Crossref] [PubMed]
- Sawyer DM, Pace LA, Pascale CL, et al. Lymphocytes influence intracranial aneurysm formation and rupture: role of extracellular matrix remodeling and phenotypic modulation of vascular smooth muscle cells. J Neuroinflammation 2016;13:185. [Crossref] [PubMed]
- Starke RM, Raper DM, Ding D, et al. Tumor necrosis factor-alpha modulates cerebral aneurysm formation and rupture. Transl Stroke Res 2014;5:269-77. [Crossref] [PubMed]
- Ali MS, Starke RM, Jabbour PM, et al. TNF-alpha induces phenotypic modulation in cerebral vascular smooth muscle cells: implications for cerebral aneurysm pathology. J Cereb Blood Flow Metab 2013;33:1564-73. [Crossref] [PubMed]
- Aoki T, Kataoka H, Shimamura M, et al. NF-kappaB is a key mediator of cerebral aneurysm formation. Circulation 2007;116:2830-40. [Crossref] [PubMed]
- Aoki T, Frosen J, Fukuda M, et al. Prostaglandin E2-EP2-NF-kappaB signaling in macrophages as a potential therapeutic target for intracranial aneurysms. Sci Signal 2017;10:eaah6037. [Crossref] [PubMed]
- Suzuki K, Watanabe T, Sakurai S, et al. A novel glycosylphosphatidyl inositol-anchored protein on human leukocytes: a possible role for regulation of neutrophil adherence and migration. J Immunol 1999;162:4277-84. [PubMed]
- Galland F, Malergue F, Bazin H, et al. Two human genes related to murine vanin-1 are located on the long arm of human chromosome 6. Genomics 1998;53:203-13. [Crossref] [PubMed]
- Sendo D, Takeda Y, Ishikawa H, et al. Localization of GPI-80, a beta2-integrin-associated glycosylphosphatidyl-inositol anchored protein, on strongly CD14-positive human monocytes. Immunobiology 2003;207:217-21. [Crossref] [PubMed]
- Tutino VM, Poppenberg KE, Li L, et al. Biomarkers from circulating neutrophil transcriptomes have potential to detect unruptured intracranial aneurysms. J Transl Med 2018;16:373. [Crossref] [PubMed]
- Tutino VM, Poppenberg KE, Jiang K, et al. Circulating neutrophil transcriptome may reveal intracranial aneurysm signature. PLoS One 2018;13:e0191407. [Crossref] [PubMed]
- Feng Y, Broder CC, Kennedy PE, et al. HIV-1 entry cofactor: functional cDNA cloning of a seven-transmembrane, G protein-coupled receptor. Science 1996;272:872-7. [Crossref] [PubMed]
- Oberlin E, Amara A, Bachelerie F, et al. The CXC chemokine SDF-1 is the ligand for LESTR/fusin and prevents infection by T-cell-line-adapted HIV-1. Nature 1996;382:833-5. [Crossref] [PubMed]
- Rempel SA, Dudas S, Ge S, et al. Identification and localization of the cytokine SDF1 and its receptor, CXC chemokine receptor 4, to regions of necrosis and angiogenesis in human glioblastoma. Clin Cancer Res 2000;6:102-11. [PubMed]
- Garcia-Cuesta EM, Santiago CA, Vallejo-Diaz J, et al. The Role of the CXCL12/CXCR4/ACKR3 Axis in Autoimmune Diseases. Front Endocrinol (Lausanne) 2019;10:585. [Crossref] [PubMed]
- Hoh BL, Hosaka K, Downes DP, et al. Stromal cell-derived factor-1 promoted angiogenesis and inflammatory cell infiltration in aneurysm walls. J Neurosurg 2014;120:73-86. [Crossref] [PubMed]
- Michineau S, Franck G, Wagner-Ballon O, et al. Chemokine (C-X-C motif) receptor 4 blockade by AMD3100 inhibits experimental abdominal aortic aneurysm expansion through anti-inflammatory effects. Arterioscler Thromb Vasc Biol 2014;34:1747-55. [Crossref] [PubMed]
- Tanios F, Pelisek J, Lutz B, et al. CXCR4: A Potential Marker for Inflammatory Activity in Abdominal Aortic Aneurysm Wall. Eur J Vasc Endovasc Surg 2015;50:745-53. [Crossref] [PubMed]
- Kodali R, Hajjou M, Berman AB, et al. Chemokines induce matrix metalloproteinase-2 through activation of epidermal growth factor receptor in arterial smooth muscle cells. Cardiovasc Res 2006;69:706-15. [Crossref] [PubMed]
(English Language Editors: J. Jones and J. Chapnick)