The value of bi-exponential and non-gaussian distribution diffusion-weighted imaging in the differentiation of recurrent soft tissue neoplasms and post-surgical changes
Introduction
Recurrence of soft tissue neoplasms (STN) is commonly seen in daily practice (1), especially in sarcomas and some intermediate STN. About 1/3 sarcomas will recur (2). The average 5-year survival rate is 52–60% (1,3). The probability of resection declines dramatically along with the times of recurrences (2). There is only a 10% chance of resection for the third time recurrence. Therefore, it is pivotal to detect RSTN early and non-invasively.
The value of conventional MR in the assessment of STN was well-established (4-8). Conventional MR mainly focused on the T1 and T2 relaxation differences, and morphologic/anatomic appraisal. The diagnostic specificity was not high. A series of changes after surgery (scars, hematoma, inflammatory granuloma, and fibrosis), were also difficult to distinguish from recurrence (9). Lee et al. (10) found that conventional MR features based nomograms can predict the probability of malignancy. Inflammation or post-operative fibrosis may mimic a nodule or mass. Functional MR can serve as a useful complement for differentiation (11). Combined with conventional MR, and diffusion-weighted imaging (DWI) imaging with apparent diffusion coefficient (ADC) mapping, a specificity of 97% can be reached for benign and malignant differentiation.
Intravoxel incoherent motion (IVIM) was first reported by Le Bihan et al. (12), by employing a bi-exponential decay model to depict the water molecules diffusion phenomenon (13-18). The ADC value was affected by microcirculation (capillary network) at lower b-values (≤200 s/mm2). The pseudodiffusion coefficient (D*) value was named to assess the diffusion induced by microvascular perfusion, and it was thought much higher than the true diffusion coefficient (D) value (13). D* and perfusion fraction (f) values were correlated well with microvessel density of colorectal cancer animal models, and IVIM parameters were also correlated with cerebral blood volume in gliomas (13,19,20).
Diffusion kurtosis imaging (DKI) was derived from diffusion tensor imaging (DTI) (21-23). Water molecules’ diffusion didn’t always abide Gaussian distribution, for the impediment of cell membranes and compartments. Non-Gaussian diffusion was notable at high b value (13). DKI was employed to describe non-Gaussian distribution (24-27). Kurtosis, as a dimensionless metric, was employed to quantify the degree of non-Gaussian diffusion and characterize tumor’s heterogeneity.
We intended to explore the feasibility of IVIM and DKI in the differentiation of recurrent soft tissue neoplasms (RSTN) and post-surgery changes (PSC), and compare them with mono-exponential decay diffusion-weighted imaging (DWI). Mono- exponential, bi-exponential, and non-Gaussian distribution based predictive models for differentiation of RSTN and PSC were also built and compared. We present the following article in accordance with the STARD 2015 reporting checklist (available at http://dx.doi.org/10.21037/atm-20-2025).
Methods
Study population and clinical data
The study was conducted in accordance with the Declaration of Helsinki (as revised in 2013). The study was approved by the institutional ethics board of Fudan University Shanghai Cancer Center and informed consent of this prospective study was waived. Totally, the clinical, IVIM and DKI imaging of a cohort of 27 patients [15 RSTN (22 masses), and 12 PSC (12 lesions)] with 34 masses, from Nov 01 2017 to Sep 30 2018, were reviewed.
Inclusion criteria: (I) all patients with complete clinical history; (II) all the masses were histologically-proven by surgery or fine-needle aspiration biopsy. Sixteen cases were excluded for unproven by histology. Twenty-seven cases (34 masses) were histologically-proven and included. They were divided into RSTN and PSC groups, 15 cases (22 masses) were recurrences and 12 cases (12 lesions) were PSC.
Their clinical data were reviewed, including the age of onset, gender, locations (head and neck region, trunk, and extremity), and histology of primary tumors.
The scan parameters of conventional MR and DWI (mono-exponential, bi-exponential and non-gaussian distribution)
All the patients underwent MR scans with a 3T-superconducting system (Siemens MAGNETOM Skyra). Axial TSE T1WI and enhanced T1WI were performed with the following parameters: TR 230 ms, TE 2.5 ms, FA 60, matrix 240*350, number of excitation 2, slice thickness 5 mm, intersection gap 1 mm, FOV 350*350 mm (in-plane resolution 1.46*1 mm), and the scanning time was 1:40 (min: sec). Axial and coronal TSE or FS TSE were performed with the following parameters: TR 7,100 ms, TE 100–110 ms, ETL 6, matrix 240*350, number of excitation 2, slice thickness 5 mm, intersection gap 1 mm, FOV 350 mm*350 mm (in-plane resolution 1.46*1 mm), the scanning time was 1:35 (min: sec).
On the same geometry of FOV 350*350 mm, matrix 256*256 (in-plane resolution 1.37*1.37 mm), slice thickness 5 mm, intersection gap 1 mm, IVIM and DKI were performed pre-intravenous injection of Gd-DTPA (0.1 mmol/kg, bolus at a flow rate 2–3 mL/s) with the following parameters. The IVIM (SS-SE-EPI): TR 5,700 ms, TE 81 ms. Nine b values were exploited (0, 20, 40, 60, 100, 150, 200, 500 and 800 s/mm2), and the scanning time was 4:35 (min:sec). The DKI (SS-SE-EPI): TR 8,500 ms, TE 74 ms. Five b values were exploited (0, 100, 700, 1,400 and 2,100 s/mm2), and the scanning time was 4:30 (min: sec).
MR Body Diffusion Toolbox (www.siemens.com/syngo.via-frontier) was used for post-processing. Noise reduction and distortion correction of DWI was done. Mono-exponential and bi-exponential decay curves were generated respectively, by employing nine b values (0, 20, 40, 60, 100, 150, 200, 500 and 800 s/mm2). And mono-exponential and non-Gaussian distribution models were employed to generate decay curves, by employing five b values (0, 100, 700, 1,400 and 2,100 s/mm2).
The region of interest (ROI) was drawn manually on the greatest axial slice as larger as possible to cover the whole slice by a radiologist with 10 years experiences on MR imaging interpretation, and the conventional T1WI/T2WI/enhanced T1WI were referenced to avoid the infections of internal necrosis and partial volume effect. The quantitative values of IVIM (ADC_IVIM, D, D*, f), and DKI (ADC_DKI, MK, and MD) of the ROIs were generated and measured. The ADC_IVIM value was measured on ADC mapping that was generated by using the nine b values. And ADC_DKI value was measured on ADC mapping that was generated by using the five b values. These values were documented. The ROIs were drawn twice at a one-month interval. The average values of the two times were calculated and recorded.
Statistical analysis
SPSS 20.0 and MedCalc software were employed for data analysis. Kolmogorov-Smirnov test was used for the normality test. The intra-class correlation coefficient (ICC) was employed for repeatability of IVIM (ADC_IVIM, D, D*, f) and DKI (ADC_DKI, MK, and MD) values. Independent student’ t-tests or Mann-Whitney U tests were used to compare differences between recurrence and PSC (P<0.05). Receiver characteristic operator (ROC) curves were generated, and the cut-off values were determined by ROC analysis (MedCalc software). Logistic regression was employed to build predictive models. DeLong test was employed to compare different ROC curves, the level of α was set at 0.05 divided by the number of comparisons (P<0.05/3) (28).
Results
Clinical data
The gender ratio (female:male) was 16:11. The age ranged from 26 to 82 years old, and the median age was 58 years old. Eleven cases arose in extremities, 13 in the trunk (3 in retroperitoneum), and 3 in the head and neck region.
There were 15 histologically proven RSTN with 22 masses. Three liposarcomas (2 dedifferentiated, 1 myxoid), 3 spindle cell sarcomas, 3 fibrosarcomas, 2 synovial sarcomas, 1 malignant peripheral never sheath tumor, 1 epithelioid sarcoma, 1 pleomorphic undifferentiated sarcoma, and 1 angiosarcoma were enrolled. Five of them were found with metastases, 4 in the lung (1 coexisted with liver metastasis), and 1 in the bone.
There were 12 histologically proven PSC. Their histology subtypes of primary tumors were synovial sarcoma (n=4), myxoid liposarcoma (n=2), fibrosarcoma (n=2), epithelioid sarcoma (n=1), pleomorphic undifferentiated sarcoma (n=1), alveolar soft part sarcoma (n=1) and undifferentiated sarcoma (n=1).
The comparisons of DWI and IVIM, DWI, and DKI parameters between recurrences and PSC
The repeatability of DWI/IVIM/DKI parameters was high. The ICCs were all ≥0.841.
Compared with the mono-exponential decay model, bi-exponential and non-Gaussian distribution decay models fit better with measured values (Figures 1,2).
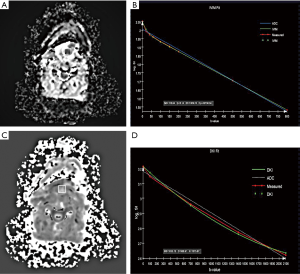
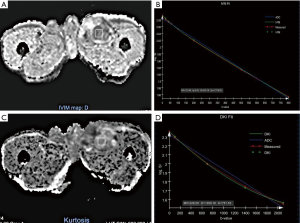
The ADC_IVIM, D, D*, and f values of recurrences overlapped those of PSC. The ADC_IVIM and D* values were higher than the D value. There were significant differences between recurrence and PSC in ADC_IVIM, D, and f values (P=1*10-4, 1.2*10-4, 0.036 respectively) (Table 1). The ADC_IVIM [(1,077.13±211.97) µm2/s], D [(1,020.6±20.63) µm2/s] and f [(97.99±7.996)‰] values of recurrence were lower than those of PSC [(1,933.6±323.21) µm2/s, (1,831.36±317.35) µm2/s and (143.87±38.35)‰]. However, there wasn’t significant difference between D* values of two groups (Table 1).
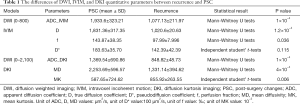
Full table
The AUC of ADC_IVIM and D values were 0.921 [95% confidence interval (CI): 0.772–0.986] and 0.925 (95% CI: 0.777–0.987) in differentiation and were higher than that of f value (0.714, 95% CI: 0.531–0.857, Delong test, P<0.0167) (Figure 3). Nevertheless, there was no significant difference in AUC between ADC and D values (Delong test, P=0.731) (Table 2).
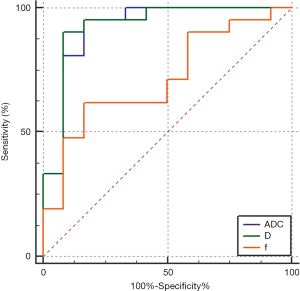

Full table
The cut-off values of ADC_IVIM, D, and f values in differentiation were ≤1,424.85 µm2/s, ≤1,274.25 µm2/s, <98.3‰ respectively (Table 2).
There were significant differences in ADC_DKI, MD, and MK values between these two groups. The ADC_DKI and MD values of recurrence [(848.82±48.73) µm2/s, (1,331.14±394.62) µm2/s] were lower than those of PSC [(1,369.54±690.86) µm2/s, P=1×10-4; (2,253.69±596.57) µm2/s, P=4×10-4], but the MK values of recurrence [(855.92±263.55)×10-3] was higher than that of PSC [(587.65±724.62)×10-3, P=0.006].
The cut-off values of ADC_DKI, MD, and MK were ≤1,040.95 µm2/s, ≤1,779 µm2/s, and >635×10-3 respectively (Table 3).

Full table
The AUC of ADC_DKI, MD and MK in differentiation were 0.877 (95% CI: 0.716–0.965), 0.913 (95% CI: 0.761–0.982) and 0.853 (95% CI: 0.687–0.952) respectively, without significant differences (Figure 4).
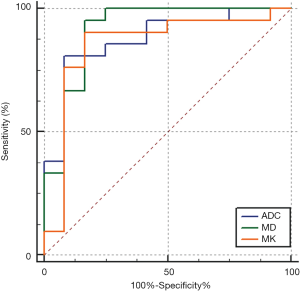
The comparisons of AUC between mono- and bi-exponential, and mono-exponential and non-Gaussian distribution based predictive models
Logistic regression was employed to build predictive models, using the values with significant statistical differences generated by IVIM (D and f values) and mono-exponential (ADC_IVIM) respectively. The AUC of bi-exponential based predictive model (0.933, 95% CI: 0.788–0.990) for RSTN and PSC differentiation was slightly higher than that of mono-exponential based predictive model (0.921, 95% CI: 0.772–0.986), but without significant difference (Figure 5A). The sensitivity and specificity were 95.45% and 83.33% respectively for mono-exponential decay, and 100% and 83.33% respectively for bi-exponential decay based predictive model.
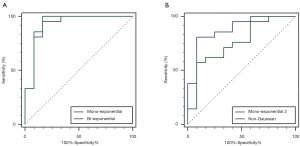
Logistic regression was employed to build predictive models, using the values with significant statistical differences generated by non-Gaussian distribution (MD and MK values) and mon-exponential (ADC_DKI) respectively. The AUC of mono-exponential decay based predictive model (0.877, 95% CI: 0.716–0.965) was slightly higher than that of the non-Gaussian distribution based predictive model (0.766, 95% CI: 0.586–0.895), but without significant difference (Figure 5B). The sensitivity and specificity were 80.95% and 91.67% respectively for mono-exponential decay, 57.14%, and 91.67% for bi-exponential decay based predictive model.
Discussion
The quantitative parameters of recurrence and PSC demonstrated great repeatability (29). The ADC, D, f, MD, and MK values of recurrence were lower than those of PSC. The ADC_IVIM and D values performed better than f value in differentiation. No differences were found among AUCs of ADC_DKI, MD, and MK values. The AUCs didn’t show significant differences between bi- and mono-exponential decay, and between mono-exponential decay and non-Gaussian distribution based predictive models. IVIM and DKI were useful in the differentiation of RSTN and PSC.
In this group, most lesions occurred in the trunk (48%) and extremities (41%). Synovial sarcoma (22%) and liposarcoma (19%) were the common types of our group.
Diffusion-weighted imaging depicted the movement of water molecules at the microscopic level, and the interpretation of the DWI evolved from the mono-exponential decay to the bi-exponential decay and non-Gaussian distribution model (5,9,12,23-25). Mono-exponential decay DWI has been used for the characterization of recurrence and PSC by other researchers (9,11,30). Similar to their findings, there were significant differences in ADC values between recurrence and PSC, although the ADC value of recurrence and PSC can be overlapped. Qi et al. found that the ADC, D, and f values of benign lymph node were higher than those of malignant ones, and the specificity and accuracy were improved remarkably when combining the D and f values (15). The ADC value was affected remarkably by microvascular perfusion at lower b value (≤200 s/mm2). To explore the microvessel perfusion of RSTN and PSC, 7 of 9 b values we exploited were lower than 200 s/mm2. We found that the bi-exponential decay model fitted better with measured values than the mono-exponential model, additionally, it can provide more quantitative values for differentiation. D and f values can be used in differentiation. The D value of recurrence was lower that of PSC, we speculated it may due to their higher cellular density. Although the AUC of D value was slightly higher than that of ADC value, without significant difference. We also found that the f value of recurrence was smaller than that of PSC (non-tumor tissue). Although its diagnostic performance was not as high as that of D value, f value can serve as another valuable quantitative feature for differentiation.
DKI was employed to depict the non-Gaussian distribution of water molecules (21,22). The reported b value that was employed sometimes exceeds 3,000 s/mm2 and can be as high as 20,000 s/mm2. K value, as a dimensionless value, can quantitatively describe the degree of non-Gaussian distribution. DKI could provide more subtle information than DTI (21,22), which was used in brain tumors, Parkinson’s disease, and breast tumors (22,23,31,32). We used five b values to depict the curve of non-Gaussian distribution. Three of them were higher than 1,000 s/mm2. We found that ADC, MD, and MK values were helpful in the differentiation of RSTN and PSC. The ADC and MD values of recurrence were lower than those of PSC, but it had higher MK values. We speculated two main reasons for these results. One reason was the heterogeneity of tumor pathology types included, and the other was that recurrences of STN had more complicated microstructure, and showed notable heterogeneity. The AUC of ADC, MD, and MK values in differentiation were 0.877, 0.913, and 0.853 respectively, and there were no significant differences among them.
Del et al. (11) found that sensitivity of 100% and specificity of 97% can be obtained, combining the conventional and dynamic contrast-enhanced (DCE) MR imaging. The differential performance also can be improved when combined with the conventional and DWI with the ADC map. We constructed and compared logistic regression models based on mono-exponential and bi-exponential decay, and mono-exponential decay and non-Gaussian distribution. There were no statistically significant differences in AUCs between mono-exponential (0.933, 95% CI: 0.788–0.990) and bi-exponential decay (0.921, 95% CI: 0.772–0.986), and mono-exponential decay (0.877, 95% CI: 0.716–0.965) and non-Gaussian distribution (0.766, 95% CI: 0.586–0.895). Unlike in literature (11), the sensitivity of and specificity of these models were all high, although this was on basis of a relatively small sample size. The final diagnosis of RSTN still relied on surgical or fine-needle biopsy pathology.
IVIM and DKI worked in different b-values, and they reflected different tissue properties (12,13,15,22,23). The D* and f values of IVIM reflected the status of microvascular perfusion and diffusion. Whereas the k value was an indicator of evaluation of diffusion with non-Gaussian distribution and indirectly reflected the heterogeneity. In this research, we did not compare the differences in the values of these two methods.
Several limitations should be mentioned. Foremost, the sample size was small. Secondly, we drew ROIs on the greatest axial slice (with most tumor pixels). Although we measured twice at a one-month interval and documented the mean value, the selection bias cannot be avoided. Lastly, the PSC was complex, including hematoma, inflammatory granuloma, scar tissue, and fibrosis and so on, the differences between these changes and recurrence should be explored further.
Conclusions
IVIM and DKI both can serve useful techniques in differentiating recurrences from PSC. ADC, D, f, MD, and MK values can be used in differentiation.
Acknowledgments
Funding: This research was supported in part by grants from the Science and Technology Council of Shanghai (grant No. 15ZR1408000, grant No. 18. No. 12140901302, and grant No. 18140901200).
Footnote
Reporting Checklist: The authors have completed the STARD 2015 reporting checklist. Available at http://dx.doi.org/10.21037/atm-20-2025
Data Sharing Statement: Available at http://dx.doi.org/10.21037/atm-20-2025
Peer Review File: Available at http://dx.doi.org/10.21037/atm-20-2025
Conflicts of Interest: All authors have completed the ICMJE uniform disclosure form (available at http://dx.doi.org/10.21037/atm-20-2025). The authors have no conflicts of interest to declare.
Ethical Statement: The authors are accountable for all aspects of the work in ensuring that questions related to the accuracy or integrity of any part of the work are appropriately investigated and resolved. The study was conducted in accordance with the Declaration of Helsinki (as revised in 2013). The study was approved by the institutional ethics board of Fudan University Shanghai Cancer Center (No.: 1310128-1) and informed consent of this prospective study was waived.
Open Access Statement: This is an Open Access article distributed in accordance with the Creative Commons Attribution-NonCommercial-NoDerivs 4.0 International License (CC BY-NC-ND 4.0), which permits the non-commercial replication and distribution of the article with the strict proviso that no changes or edits are made and the original work is properly cited (including links to both the formal publication through the relevant DOI and the license). See: https://creativecommons.org/licenses/by-nc-nd/4.0/.
References
- Zou Y, Wang QD, Zong M, et al. Apparent diffusion coefficient measurements with diffusion-weighted imaging for differential diagnosis of soft-tissue tumor. J Cancer Res Ther 2016;12:864-70. [Crossref] [PubMed]
- Kane JM 3rd. Surveillance strategies for patients following surgical resection of soft tissue sarcomas. Curr Opin Oncol 2004;16:328-32. [Crossref] [PubMed]
- Brewer P, Riddell Z, Grimer RJ, et al. Perioperative mortality following above-knee amputations indicated for bone and soft tissue tumours. Eur J Surg Oncol 2012;38:706-10. (EJSO). [Crossref] [PubMed]
- May DA, Good RB, Smith DK, et al. MR imaging of musculoskeletal tumors and tumor mimickers with intravenous gadolinium: experience with 242 patients. Skeletal Radiol 1997;26:2-15. [Crossref] [PubMed]
- Subhawong TK, Jacobs MA, Fayad LM. Diffusion-weighted MR imaging for characterizing musculoskeletal lesions. Radiographics 2014;34:1163-77. [Crossref] [PubMed]
- van Rijswijk CS, Geirnaerdt MJ, Hogendoorn PC, et al. Soft-tissue tumors: value of static and dynamic gadopentetate dimeglumine-enhanced MR imaging in prediction of malignancy. Radiology 2004;233:493-502. [Crossref] [PubMed]
- Patni RS, Boruah DK, Sanyal S, et al. Characterisation of musculoskeletal tumours by multivoxel proton MR spectroscopy. Skeletal Radiol 2017;46:483-95. [Crossref] [PubMed]
- Kransdorf MJ, Murphey MD. Radiologic evaluation of soft-tissue masses: a current perspective. AJR Am J Roentgenol 2000;175:575-87. [Crossref] [PubMed]
- Khoo MM, Tyler PA, Saifuddin A, et al. Diffusion-weighted imaging (DWI) in musculoskeletal MRI: a critical review. Skeletal Radiol 2011;40:665-81. [Crossref] [PubMed]
- Lee JH, Yoon YC, Jin W, et al. Development and Validation of Nomograms for Malignancy Prediction in Soft Tissue Tumors Using Magnetic Resonance Imaging Measurements. Sci Rep 2019;9:4897. [Crossref] [PubMed]
- Del Grande F, Subhawong T, Weber K, et al. Detection of soft-tissue sarcoma recurrence: added value of functional MR imaging techniques at 3.0 T. Radiology 2014;271:499-511. [Crossref] [PubMed]
- Le Bihan D, Breton E, Lallemand D, et al. Separation of diffusion and perfusion in intravoxel incoherent motion MR imaging. Radiology 1988;168:497-505. [Crossref] [PubMed]
- Iima M, Le Bihan D. Clinical Intravoxel Incoherent Motion and Diffusion MR Imaging: Past, Present, and Future. Radiology 2016;278:13-32. [Crossref] [PubMed]
- van Rijswijk CS, Kunz P, Hogendoorn PC, et al. Diffusion-weighted MRI in the characterization of soft-tissue tumors. J Magn Reson Imaging 2002;15:302-7. [Crossref] [PubMed]
- Qi LP, Yan WP, Chen KN, et al. Discrimination of Malignant versus Benign Mediastinal Lymph Nodes Using Diffusion MRI with an IVIM Model. Eur Radiol 2018;28:1301-9. [Crossref] [PubMed]
- Xu Y, Xu Q, Sun H, et al. Could IVIM and ADC help in predicting the KRAS status in patients with rectal cancer? Eur Radiol 2018;28:3059-65. [Crossref] [PubMed]
- Li H, Zhang J, Zheng Z, et al. Preoperative histogram analysis of intravoxel incoherent motion (IVIM) for predicting microvascular invasion in patients with single hepatocellular carcinoma. Eur J Radiol 2018;105:65-71. [Crossref] [PubMed]
- Sauer M, Klene C, Kaul M, et al. Preoperative evaluation of pelvine lymph node metastasis in high risk prostate cancer with intravoxel incoherent motion (IVIM) MRI. Eur J Radiol 2018;107:1-6. [Crossref] [PubMed]
- Lee HJ, Rha SY, Chung YE, et al. Tumor perfusion-related parameter of diffusion-weighted magnetic resonance imaging: correlation with histological microvessel density. Magn Reson Med 2014;71:1554-8. [Crossref] [PubMed]
- Federau C, Meuli R, O’Brien K, et al. Perfusion measurement in brain gliomas with intravoxel incoherent motion MRI. AJNR Am J Neuroradiol 2014;35:256-62. [Crossref] [PubMed]
- Jensen JH, Helpern JA. MRI quantification of non-Gaussian water diffusion by kurtosis analysis. NMR Biomed 2010;23:698-710. [Crossref] [PubMed]
- Wang JJ, Lin WY, Lu CS, et al. Parkinson disease: diagnostic utility of diffusion kurtosis imaging. Radiology 2011;261:210-7. [Crossref] [PubMed]
- Raab P, Hattingen E, Franz K, et al. Cerebral gliomas: diffusional kurtosis imaging analysis of microstructural differences. Radiology 2010;254:876-81. [Crossref] [PubMed]
- Yamada I, Yoshino N, Hikishima K, et al. Oral carcinoma: Clinical evaluation using diffusion kurtosis imaging and its correlation with histopathologic findings. Magn Reson Imaging 2018;51:69-78. [Crossref] [PubMed]
- Yin J, Sun H, Wang Z, et al. Diffusion Kurtosis Imaging of Acute Infarction: Comparison with Routine Diffusion and Follow-up MR Imaging. Radiology 2018;287:651-7. [Crossref] [PubMed]
- Goryawala MZ, Heros DO, Komotar RJ, et al. Value of diffusion kurtosis imaging in assessing low-grade gliomas. J Magn Reson Imaging 2018;48:1551-8. [Crossref] [PubMed]
- Yamada I, Sakamoto J, Kobayashi D, et al. Diffusion kurtosis imaging of endometrial carcinoma: Correlation with histopathological findings. Magn Reson Imaging 2019;57:337-46. [Crossref] [PubMed]
- DeLong ER, DeLong DM, Clarke-Pearson DL. Comparing the areas under two or more correlated receiver operating characteristic curves: a nonparametric approach. Biometrics 1988;44:837-45. [Crossref] [PubMed]
- Zidan M, Thomas RL, Slovis TL. What you need to know about statistics, part II: reliability of diagnostic and screening tests. Pediatr Radiol 2015;45:317-28. [Crossref] [PubMed]
- Baur A, Huber A, Arbogast S, et al. Diffusion-weighted imaging of tumor recurrencies and posttherapeutical soft-tissue changes in humans. Eur Radiol 2001;11:828-33. [Crossref] [PubMed]
- Van Cauter S, Veraart J, Sijbers J, et al. Gliomas: diffusion kurtosis MR imaging in grading. Radiology 2012;263:492-501. [Crossref] [PubMed]
- Sun K, Chen X, Chai W, et al. Breast Cancer: Diffusion Kurtosis MR Imaging-Diagnostic Accuracy and Correlation with Clinical-Pathologic Factors. Radiology 2015;277:46-55. [Crossref] [PubMed]