3-point major cardiovascular event outcome for patients with T2D treated with dipeptidyl peptidase-4 inhibitor or glucagon-like peptide-1 receptor agonist in addition to metformin monotherapy
Introduction
Due to chronic hyperglycemia, persons with type 2 diabetes (T2D) are typically at an increased for many hyperglycemia-associated complications and outcomes, including retinopathy, nephropathy, and neuropathy. In addition, it has also been well-established that persons with T2D have a high likelihood of experiencing adverse macro-vascular events including stroke, myocardial infarction, coronary artery disease, and heart failure (1-3). Numerous studies of persons with T2D have demonstrated associations between glycemic control and incident cardiovascular and heart failure events (4-9). Healthcare professionals regularly work with adult patients who have T2D to achieve euglycemic targets as improved glycemic control has been shown to decrease long-term risk of hyperglycemia-related complications and cardiovascular events in persons with T2D. There have been many approvals of new anti-diabetes drugs in the last 10–15 years, and healthcare providers must consider multiple factors when selecting therapies for their patients. Metformin continues to be the most common first-line therapy of choice for patients newly-diagnosed with T2D (10,11). Metformin is a member of the biguanide drug class, and is a prized therapy option due to its clean safety profile, low cost, and glycemic efficacy in combination therapy regimens (12). While in recent years, the use of metformin as a first-line has continued to increase (13), unfortunately it alone is typically not sufficient therapeutically to achieve glycemic targets (11,14), so other anti-diabetes medications must be added to the therapeutic regimen as second and third-line choices.
More than a decade ago, several large epidemiological studies implicated certain anti-diabetes medications in adverse cardiovascular outcomes for adults with T2D (8,15-17), and as a response, in 2008, the FDA issued a guidance for all new T2D glycemic management drugs that outlined approval requirements specific for cardiovascular outcomes tested in clinical trials. The guidance stated that all new T2D drug programs should rule out unacceptable cardiovascular risk by demonstrating that an upper bound of the two-sided 95% confidence interval (CI) of the risk ratio for a composite endpoint of major adverse cardiac events (3P-MACE) consisting of at least CV death, nonfatal MI, and stroke, had to be <1.8 before preapproval would be considered. The guidance required that meaningful (specified) numbers of patients with comorbid conditions and diabetes-associated complications be included in these trials.
Since that time, nearly 20 large, randomized, controlled Cardiovascular risk Outcomes Trials (CVOTs) have been run (or are ongoing) to test new therapies for acceptable levels of cardiovascular risk, and the resultant trials have shown valuable results. The TECOS trial showed that patients randomized to DPP-4i improved glycemic control compared to those randomized to placebo treatment, without increasing adverse cardiovascular outcomes (18). The SUSTAIN-6 trial (19) and LEADER trials (20) showed that patients on metformin monotherapy receiving GLP-1RA therapies had a significantly lower rate of 3P-MACE outcomes than their placebo-treated counterparts. Despite historic studies, recent studies demonstrate that both classes of glucose-lowering agents (both DPP-4i and GLP-1RA) are beneficial in that they safely lower glucose without increasing risk of developing adverse cardiovascular events when compared to placebo. Three of the major trials, SAVOR-TIMI 53 (saxagliptin, n=16,492), EXAMINE (alogliptin, n=5,380), EMPA-REG OUTCOMES (empagliflozin, n=7,020) all used a 3P-MACE outcome to evaluate the cardiovascular risk of the (DPP-4i and GLP-1RA, respectively, compared to placebo treatments in their patients. Since the 3P-MACE outcome was commonly used as a primary endpoint in the majority of recent noninferiority studies (19-27), we attempted to duplicate inclusion/exclusion criteria from several recent major CVOT trials and compare DPP-4i to GLP-1RA as second-line therapies for the 3P-MACE outcome. These anti-diabetes agents are commonly added to metformin monotherapy and have shown additive glucose lowering benefits (28-33) in combination regimens (10,14). Both of these agents have been proven to be efficacious in different settings, and both have different contra-indication profiles. The choice of which therapy to use should be made using a combination of factors, including comorbidities and contra-indications, disease needs, patient preference, and financial feasibility. This study is novel in that it uses real-world data rather than studying data from a highly-controlled clinical trial setting. We present the following article in accordance with the STROBE reporting checklist (34) (available at: http://dx.doi.org/10.21037/atm-20-4063).
Methods
The data used for this study were gathered from the enterprise-wide electronic health record system (EHR; EPIC My Practice) at Cleveland Clinic between January 2005 and September 2019. A modified Kho algorithm was used to confirm the correct identification of patients with T2D (35,36); and details for this algorithm and the International Classification of Diseases (ICD) codes that it uses are as follows: the eMERGE algorithm, modified to include ICD-10 codes in addition to ICD-9 codes as in the original algorithm, was used to calculate the earliest date when a patient record contained any of the following combinations: T2D code (ICD-9 codes 250.x0 or 250.x2; ICD-10 codes E11.xx) and T2D medication, T2D code and abnormal glucose, T2D code recorded twice and an outpatient insulin prescription, T2D medication and abnormal glucose, or insulin preceded by T2D medication. The patients with an outpatient insulin prescription were eventually excluded from analyses, as the insulin prescription would have preceded a metformin prescription if they had been included in the study cohort. If patients were correctly identified by the algorithm described above, they were then screened using the exclusion criteria described below. Patients with ICD-9 codes at any time specific for type 1 diabetes (250.x1, 250.x3) and/or ketoacidosis (250.10 and 250.12), or ICD-10 codes E08 (diabetes mellitus due to underlying conditions), E09 (drug or chemical induced diabetes mellitus), E10 (type 1 diabetes mellitus), or E13 (other specified diabetes mellitus), were excluded. Abnormal glucose was defined based on the ADA criteria [fasting blood glucose (BG) ≥126 mg/dL, A1C ≥48 mmol/mol (≥6.5%), or random BG ≥200 mg/dL]. The earliest date that any of the five conditions occurred was documented as the date on which the patient first met the criteria for T2D. Our cohort was restricted to patients who were identified by the modified Kho algorithm and who also were on Metformin as the initial active medication (the active medication was defined as a medication on the current medication list in the EHR for at least three months after being initiated). The cohort contained patients who entered and exited according to normal clinical courses, and there was no pre-specified follow-up period.
Baseline time was defined as the date of the first recorded prescription of a second hypoglycemic agent added to metformin in the Cleveland Clinic EHR. We restricted analysis to patients for whom the second-line anti-diabetes agent had been on the active medications list for >90 days to ensure that the medication was not discontinued in the early course of management or denied by insurance. Patients were also excluded from the analyses if they were on more than 2 oral diabetes medications at baseline. Consistent with the literature, 3P-MACE outcome was defined as the first occurrence of one of the following: death from a cardiovascular cause, nonfatal stroke, or nonfatal myocardial infarction (nonfatal MI). Nonfatal stroke and nonfatal MI were stroke and MI that did not lead to death within 30 days after occurrence. Hypertension drugs included were the following: calcium channel blockers, methyldopa, alpha blockers, beta blockers, diuretics, clonidine, angiotensin-converting enzyme inhibitors, mineralocorticoid receptor agonists, adenosine A2A receptor antagonists, and angiotensin receptor blockers.
Patients were further excluded from the dataset if they had any of the following conditions present at baseline (diagnoses identified by ICD 9/10 codes in Cleveland Clinic EHR unless otherwise indicated): diabetic nephropathy, glomerulonephritis, hypertensive renal disease, polycystic kidney disease, chronic kidney disease (defined as a minimum of two documented events of estimated glomerular filtration (eGFR) rate of less than or equal to 30 mL/min/1.73 m2, at least 90 days apart), patients with albuminuria (defined as two records of either urine albumin to creatinine ration of >30 mg/g or urine protein creatinine ratio >150 mg/g separated by 90 days), and patients with history of renal failure (defined as documented eGFR <30). Patients were excluded from analysis if they had a recorded eGFR of <30 or if they had a history of prior myocardial infarction prior to start of cohort data collection Persons with history of heart disease or established cardiovascular disease (CVD) were identified by ICD codes. A flow diagram depicting the algorithm for cohort selection can be reviewed in Figure S1.
Statistical analysis
SAS was used to extract the data and build the dataset. R Software, version 3.6.1 (37), was used for programming and statistical analysis. Missing values were imputed using the Multivariate Imputation by Chained Equations (mice) package, version 3.6.0. Imputation was performed to maximize the available information and to reduce potential bias that could be incurred by deleting incomplete records (38). The imputation was performed using regression techniques that include using all baseline patients and all baseline variables as predictors.
Cohort characteristics were reported using medians with interquartile ranges (IQR for 25th and 75th percentile) for continuous variables, and categorical variables were reported using counts with percentages. Differences in patient characteristics between the two drug classes, DPP-4i and GLP-1RA, were assessed using chi-square tests for categorical variables and the Wilcoxon rank sum test for continuous variables.
Predictor variables for each of the models were chosen based on extensive literature review and clinical judgment of subject matter experts and were adjusted for by the model. A Cox proportional hazards regression (39) was used to explore associations of baseline characteristics and the risk of 3P-MACE, and 5% was used to declare statistical significance.
The study was conducted in accordance with the Declaration of Helsinki (as revised in 2013). The data were supplied relating to ethical approvals by the Institutional Review Board of Cleveland Clinic #19-066. A waiver of informed consent was granted as patient data were de-identified.
Results
The baseline characteristics, stratified by second-line oral medication, are shown in Table 1. These are comparable to characteristics seen in other Type 2 populations (36). In the cohort of 5,518 patients, we observed 534 3P-MACE composite events, comprised of 283 strokes, 157 MI, and 94 CV-deaths.
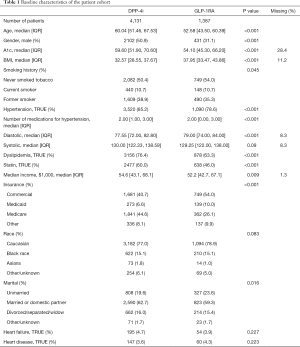
Full table
Study cohort
The overall population was predominantly Caucasian with a gender distribution showing a higher percentage of male users of DPP-4i (50.9%) than GLP-1RA (31.1%). Persons receiving GLP-1RA therapy were on average younger than those receiving DPP-4i (mean ages of approximately 53 vs. 60, respectively), had a higher body mass index than those receiving DPP-4i (approximately 38 vs. 32.5), and had a lower A1C (54.1 and 59.6 mmol/L, respectively). Median follow-up time for the overall cohort is 3.6 years. Loss to follow up was managed by time-to-event methodology.
Model covariates
The results of the Cox regression are shown in Table 2. The following covariates were the most influential prognostic factors associated with 3P-MACE: age (hazard ratio 1.057, P<0.001), male gender (hazard ratio 1.362, P=0.001), hypertension (hazard ratio 1.593, P=0.021), prior heart failure (hazard ratio 1.515, P=0.008), and insurance type (medicaid in particular, hazard ratio 1.952).
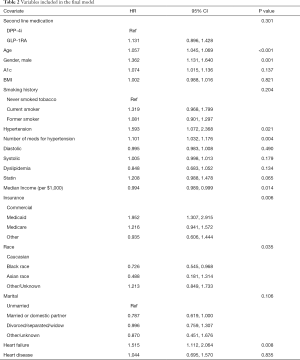
Full table
Although we saw some evidence that likelihood of developing a 3P-MACE outcome was higher for patients who were treated with GLP-1RA compared to patients who were treated with DPP-4i (hazard ratio 1.131, 95% confidence interval 0.896 to 1.428), the difference between groups did not reach a conventional level of statistical significance. To probe this finding further, we considered the possibility that the effect of the drug on 3P-MACE might depend upon whether the patient had established cardiovascular disease (CVD, identified by ICD codes in the EHR). To test that hypothesis, we evaluated an interaction term between drug and CVD but found no significant effect (hazard ratio 1.072, P=0.836), suggesting that the effect of the drug on 3P-MACE did not depend upon a history of CVD. We created a nomogram as a graphical depiction of the model, and it can be used by healthcare providers as a prognostic tool for choosing between DPP-4i and GLP-1RA as a second-line therapy for their patients (Figure 1).
Conclusions
While there has been some discussion surrounding use of composite outcomes, and whether to use a 3P or a 4P-MACE (40), we believe that it makes sense to group outcomes with common pathophysiological mechanisms. Some limitations to our study must be noted. First, we are not able to factor duration of disease into our review, as it is possible that patients may have pre-diabetes symptoms or early-stage disease prior to their entry into our healthcare system (41,42). However, we are able to adjust for many proxies of disease duration (i.e., other variables that reflect disease duration such as A1C level and comorbidities) such that it is of unclear value what additional information would be gained from having the actual disease duration value. Our study is also limited somewhat by the prescription data in the electronic health record in that we cannot gauge compliance, and we cannot provide data per manufacturer of each drug. We also do not have complete dosing data to make conclusions based on medication usage or compliance. We are not able to make conclusions based on different DPP-4i or GLP-1RA subcategories such as dosage level subgroups due to sample size limitations; however, this may be possible in the future as more patients use GLP-1RA and DPP-4i as a part of glycemic control combination therapies. Our model could also be limited by residual confounding. Given that patients were not randomized to either DPP-4i or GLP-1RA as they would have been in a clinical trial, and given that the two therapies were compared directly against each other, there could be potential differences in biases that are not adjusted for in our analysis. Nonetheless, our model included factors that are generally considered by healthcare providers when making the decision as to which drug to choose, and also included the factors that are generally considered to be predictive of 3P-MACE outcomes. Our database was sufficient for our study in that we were able to include all factors noted in the literature and suggested by our experts.
These limitations aside, our findings were provocative. First, we saw no major difference between drug classes with respect to risk of 3P-MACE. While one might predict a higher risk of 3P-MACE for someone with a history of heart disease, the increase is minor after adjusting for other baseline characteristics. Second, the effect of baseline heart disease upon risk of 3P-MACE was not meaningfully different between the two drugs (see Figure 2). Taken together, these findings would suggest that, for the purposes of medical decision making in individual patients, weighing the risk of 3P-MACE should not be much of a consideration when deciding between these two drugs, regardless of whether the patient has a history of heart disease. Moreover, history of heart disease should not be a contraindication for either of these drugs.
In future studies, it would be useful to add other anti-diabetes drugs and combination therapies into this analysis, such as 3P-MACE outcome for metformin plus SGLT-2 inhibitors, metformin plus insulins, and metformin plus thiazolidinediones, which are other commonly-used combination therapies. Given the increased cardiovascular burden for persons with T2D, and possibility for multiple cardiovascular interventions over a lifetime, it could be useful to modify the model to include patients with a history of prior cardiovascular procedure (common procedures such as arteriograft, balloon pump, stent, arthrectomy, etc.) to see whether comparable risk of 3P-MACE would be present in these patients if treated with DPP-4i or GLP-1RA as a second-line drug. It would also be useful to look further into external factors contributing to increased risk for some of the groups in our cohort, for example social determinants of health, but these factors are complex and not readily captured in electronic health record data or quantifiable by standard methods.
It will be essential to continue to closely monitor and evaluate adverse cardiovascular signals observed in T2D drug development programs as more pharmacological interventions continue to be developed and approved for use.
Acknowledgments
The authors wish to thank Jian Jin, who extracted the EHR data used in this study.
Funding: None.
Footnote
Reporting Checklist: The authors have completed the STROBE reporting checklist, available at: http://dx.doi.org/10.21037/atm-20-4063
Data Sharing Statement: Available at http://dx.doi.org/10.21037/atm-20-4063
Conflicts of Interest: All authors have completed the ICMJE uniform disclosure form (available at: http://dx.doi.org/10.21037/atm-20-4063). The authors have no conflicts of interest to declare.
Ethical Statement: The authors are accountable for all aspects of the work in ensuring that questions related to the accuracy or integrity of any part of the work are appropriately investigated and resolved. The study was conducted in accordance with the Declaration of Helsinki (as revised in 2013). The data were supplied relating to ethical approvals by the Institutional Review Board of Cleveland Clinic #19-066. A waiver of informed consent was granted as patient data were de-identified.
Open Access Statement: This is an Open Access article distributed in accordance with the Creative Commons Attribution-NonCommercial-NoDerivs 4.0 International License (CC BY-NC-ND 4.0), which permits the non-commercial replication and distribution of the article with the strict proviso that no changes or edits are made and the original work is properly cited (including links to both the formal publication through the relevant DOI and the license). See: https://creativecommons.org/licenses/by-nc-nd/4.0/.
References
- McMurray JJ, Gerstein HC, Holman RR, et al. Heart failure: a cardiovascular outcome in diabetes that can no longer be ignored. Lancet Diabetes Endocrinol 2014;2:843-51. [Crossref] [PubMed]
- Kannel WB, Hjortland M, Castelli WP. Role of diabetes in congestive heart failure: the Framingham study. Am J Cardiol 1974;34:29-34. [Crossref] [PubMed]
- Rawshani A, Rawshani A, Gudbjornsdottir S. Mortality and Cardiovascular Disease in Type 1 and Type 2 Diabetes. N Engl J Med 2017;377:300-1. [Crossref] [PubMed]
- Iribarren C, Karter AJ, Go AS, et al. Glycemic control and heart failure among adult patients with diabetes. Circulation 2001;103:2668-73. [Crossref] [PubMed]
- van Melle JP, Bot M, de Jonge P, et al. Diabetes, glycemic control, and new-onset heart failure in patients with stable coronary artery disease: data from the heart and soul study. Diabetes Care 2010;33:2084-9. [Crossref] [PubMed]
- Held C, Gerstein HC, Yusuf S, et al. Glucose levels predict hospitalization for congestive heart failure in patients at high cardiovascular risk. Circulation 2007;115:1371-5. [Crossref] [PubMed]
- Nielson C, Lange T. Blood glucose and heart failure in nondiabetic patients. Diabetes Care 2005;28:607-11. [Crossref] [PubMed]
- Action to Control Cardiovascular Risk in Diabetes Study G, Gerstein HC, Miller ME, et al. Effects of intensive glucose lowering in type 2 diabetes. N Engl J Med 2008;358:2545-59. [Crossref] [PubMed]
- Stratton IM, Adler AI, Neil HA, et al. Association of glycaemia with macrovascular and microvascular complications of type 2 diabetes (UKPDS 35): prospective observational study. BMJ 2000;321:405-12. [Crossref] [PubMed]
- ADA. Standards of Medical Care in Diabetes: 20202020 January 2020.
- Garber AJ, Abrahamson MJ, Barzilay JI, et al. Consensus Statement by the American Association of Clinical Endocrinologists and American College of Endocrinology on the Comprehensive Type 2 Diabetes Management Algorithm - 2019 Executive Summary. Endocr Pract 2019;25:69-100. [Crossref] [PubMed]
- Sanchez-Rangel E, Inzucchi SE. Metformin: clinical use in type 2 diabetes. Diabetologia 2017;60:1586-93. [Crossref] [PubMed]
- Montvida O, Shaw J, Atherton JJ, et al. Long-term Trends in Antidiabetes Drug Usage in the U.S.: Real-world Evidence in Patients Newly Diagnosed With Type 2 Diabetes. Diabetes Care 2018;41:69-78. [Crossref] [PubMed]
- Singh AK. Deciding oral drugs after metformin in type 2 diabetes: An evidence-based approach. Indian J Endocrinol Metab 2014;18:617-23. [PubMed]
- Meinert CL, Knatterud GL, Prout TE, et al. A study of the effects of hypoglycemic agents on vascular complications in patients with adult-onset diabetes. II. Mortality results. Diabetes 1970;19 Suppl:789-830. [PubMed]
- Nissen SE, Wolski K, Topol EJ. Effect of muraglitazar on death and major adverse cardiovascular events in patients with type 2 diabetes mellitus. JAMA 2005;294:2581-6. [Crossref] [PubMed]
- Nissen SE, Wolski K. Effect of rosiglitazone on the risk of myocardial infarction and death from cardiovascular causes. N Engl J Med 2007;356:2457-71. [Crossref] [PubMed]
- Green JB, Bethel MA, Armstrong PW, et al. Effect of Sitagliptin on Cardiovascular Outcomes in Type 2 Diabetes. N Engl J Med 2015;373:232-42. [Crossref] [PubMed]
- Marso SP, Bain SC, Consoli A, et al. Semaglutide and Cardiovascular Outcomes in Patients with Type 2 Diabetes. N Engl J Med 2016;375:1834-44. [Crossref] [PubMed]
- Marso SP, Daniels GH, Brown-Frandsen K, et al. Liraglutide and Cardiovascular Outcomes in Type 2 Diabetes. N Engl J Med 2016;375:311-22. [Crossref] [PubMed]
- Scirica BM, Bhatt DL, Braunwald E, et al. Saxagliptin and cardiovascular outcomes in patients with type 2 diabetes mellitus. N Engl J Med 2013;369:1317-26. [Crossref] [PubMed]
- White WB, Cannon CP, Heller SR, et al. Alogliptin after acute coronary syndrome in patients with type 2 diabetes. N Engl J Med 2013;369:1327-35. [Crossref] [PubMed]
- Zannad F, Cannon CP, Cushman WC, et al. Heart failure and mortality outcomes in patients with type 2 diabetes taking alogliptin versus placebo in EXAMINE: a multicentre, randomised, double-blind trial. Lancet 2015;385:2067-76. [Crossref] [PubMed]
- Holman RR, Bethel MA, Mentz RJ, et al. Effects of Once-Weekly Exenatide on Cardiovascular Outcomes in Type 2 Diabetes. N Engl J Med 2017;377:1228-39. [Crossref] [PubMed]
- Zinman B, Wanner C, Lachin JM, et al. Empagliflozin, Cardiovascular Outcomes, and Mortality in Type 2 Diabetes. N Engl J Med 2015;373:2117-28. [Crossref] [PubMed]
- Wanner C, Inzucchi SE, Zinman B. Empagliflozin and Progression of Kidney Disease in Type 2 Diabetes. N Engl J Med 2016;375:1801-2. [Crossref] [PubMed]
- Neal B, Perkovic V, Matthews DR. Canagliflozin and Cardiovascular and Renal Events in Type 2 Diabetes. N Engl J Med 2017;377:2099. [Crossref] [PubMed]
- Rosenstock J, Chuck L, Gonzalez-Ortiz M, et al. Initial Combination Therapy With Canagliflozin Plus Metformin Versus Each Component as Monotherapy for Drug-Naive Type 2 Diabetes. Diabetes Care 2016;39:353-62. [Crossref] [PubMed]
- Deacon CF, Mannucci E, Ahren B. Glycaemic efficacy of glucagon-like peptide-1 receptor agonists and dipeptidyl peptidase-4 inhibitors as add-on therapy to metformin in subjects with type 2 diabetes-a review and meta analysis. Diabetes Obes Metab 2012;14:762-7. [Crossref] [PubMed]
- Arechavaleta R, Seck T, Chen Y, et al. Efficacy and safety of treatment with sitagliptin or glimepiride in patients with type 2 diabetes inadequately controlled on metformin monotherapy: a randomized, double-blind, non-inferiority trial. Diabetes Obes Metab 2011;13:160-8. [Crossref] [PubMed]
- Scott R, Loeys T, Davies MJ, et al. Efficacy and safety of sitagliptin when added to ongoing metformin therapy in patients with type 2 diabetes. Diabetes Obes Metab 2008;10:959-69. [Crossref] [PubMed]
- Bailey CJ, Gross JL, Pieters A, et al. Effect of dapagliflozin in patients with type 2 diabetes who have inadequate glycaemic control with metformin: a randomised, double-blind, placebo-controlled trial. Lancet 2010;375:2223-33. [Crossref] [PubMed]
- Softeland E, Meier JJ, Vangen B, et al. Empagliflozin as Add-on Therapy in Patients With Type 2 Diabetes Inadequately Controlled With Linagliptin and Metformin: A 24-Week Randomized, Double-Blind, Parallel-Group Trial. Diabetes Care 2017;40:201-9. [Crossref] [PubMed]
- von Elm E, Altman DG, Egger M, et al. The Strengthening the Reporting of Observational Studies in Epidemiology (STROBE) statement: guidelines for reporting observational studies. J Clin Epidemiol 2008;61:344-9. [Crossref] [PubMed]
- Kho AN, Hayes MG, Rasmussen-Torvik L, et al. Use of diverse electronic medical record systems to identify genetic risk for type 2 diabetes within a genome-wide association study. J Am Med Inform Assoc 2012;19:212-8. [Crossref] [PubMed]
- Pantalone KM, Hobbs TM, Wells BJ, et al. Changes in Characteristics and Treatment Patterns of Patients with Newly Diagnosed Type 2 Diabetes in a Large United States Integrated Health System between 2008 and 2013. Clin Med Insights Endocrinol Diabetes 2016;9:23-30. [Crossref] [PubMed]
- R Development Core Team. R: A language and environment for statistical computing. R Foundation for Statistical Computing 3.6.1 ed. Vienna, Austria 2019.
- van der Heijden GJ, Donders AR, Stijnen T, et al. Imputation of missing values is superior to complete case analysis and the missing-indicator method in multivariable diagnostic research: a clinical example. J Clin Epidemiol 2006;59:1102-9. [Crossref] [PubMed]
- Cox DL. Regression Models and Life-Tables. Journal of the Royal Statistical Society 1972;34:187-202.
- Marx N, McGuire DK, Perkovic V, et al. Composite Primary End Points in Cardiovascular Outcomes Trials Involving Type 2 Diabetes Patients: Should Unstable Angina Be Included in the Primary End Point? Diabetes Care 2017;40:1144-51. [Crossref] [PubMed]
- Harris MI, Klein R, Welborn TA, et al. Onset of NIDDM occurs at least 4-7 yr before clinical diagnosis. Diabetes Care 1992;15:815-9. [Crossref] [PubMed]
- Porta M, Curletto G, Cipullo D, et al. Estimating the delay between onset and diagnosis of type 2 diabetes from the time course of retinopathy prevalence. Diabetes Care 2014;37:1668-74. [Crossref] [PubMed]