Correlation between alterations of gut microbiota and miR-122-5p expression in patients with type 2 diabetes mellitus
Introduction
Type 2 diabetes mellitus (T2DM) is a major public health problem worldwide. At present, 366 million people are estimated to have T2DM globally; this figure is predicted to reach 422 million by 2030, and rise to 700 million by 2045 (1,2). Accounting for over 90% of cases, T2DM is the most common form of diabetes (3). T2DM is a metabolic disease characterized by insulin secretion defects and/or chronic hyperglycemia due to insulin resistance. T2DM affects all fields of physiological and biochemical activity, including genetics, metabolomics, and proteomics. Currently, blood glucose monitoring is the main diagnostic method for T2DM, and the pathogenic mechanism of T2DM occurrence has yet to be fully elucidated. There is no specific treatment that can improve islet function, which makes prevention and early diagnosis crucial. Consequently, the various complex interaction mechanisms in the host’s body at the initial stage of T2DM need to be illuminated, and new ideas and strategies for identifying key therapeutic targets in T2DM are urgently called for.
The gut microbiota is an extremely large microbial population in the host gastrointestinal tract (4), which can co-evolve with humans. It controls nutrient absorption and energy conversion, forming and regulating the intestinal mucosal barrier, and assisting the intestinal immune defense system in maintaining the body’s homeostasis, such as maturity after birth. The disruption of the symbiotic relationship between the gut microbiota and the host may lead to the occurrence of metabolic diseases such as T2DM. MicroRNAs (miRNAs) are a class of non-coding single-stranded molecules, the expression of which is tissue-specific and developmentally regulated. MiRNAs have been shown to be closely related to a broad range of physiological and pathological processes. The main function of miRNAs is to negatively regulate gene expression by cutting target gene transcripts or binding to the 3' untranslated region (3'-UTR) of target messenger RNAs (mRNAs) to inhibit mRNA translation at the post-transcriptional level (5). The regulatory effects of miRNAs on diabetes at the genetic level have been investigated in depth (6).
Accumulating evidence has illustrated that imbalance of the gut microbiota and changes in miRNA expression levels participate in the occurrence and progression of human diseases; both may act as triggers for various disorders and have been confirmed as promising biomarkers for T2DM. MiRNAs may mediate part of the crosstalk network between gut microbiota and the host (7,8). Gut microbiota can interact with miRNAs and play an intricate role in the regulation of related metabolic diseases (8). Therefore, through this interaction with miRNAs, the gut microbiota may affect far more genes than we expected. However, due to a lack of systematic clinical population studies, the role of this interaction in the pathophysiological mechanism of T2DM has not been well evaluated. In the present study, we conducted a case-control study, 16 srRNA third-generation full-length sequencing, microRNA high-throughput sequencing, bioinformatics analysis, and statistical methods were applied to detect variations in gut microbiota and the levels of circulating miRNAs in plasma between T2DM patients and healthy individuals. We aim to illuminate the crucial role of the interaction between gut microbiota and circulating miRNAs in T2DM, and to provide a theoretical basis for exploreing and developing more accurate and efficient methods for the early diagnosis and clinical interventions of patients with T2DM.
We present the following article in accordance with the STROBE reporting checklist (available at http://dx.doi.org/10.21037/atm-20-6717).
Methods
Clinical samples
According to the epidemiological characteristics, blood glucose indicators and consideration of 20% shedding rate, we confirmed the sample size of this case-control study. We recruited 60 volunteers and divided them in two groups. The T2DM group was composed of patients who are newly diagnosed with T2DM from the Endocrinology department, Hospital of Chengdu University of Traditional Chinese Medicine in Si Chuan province, China from March 2020 to September 2020. T2DM diagnosis based on World Health Organization criteria in 1999 (9). Simultaneously, healthy individuals were also enrolled from the medical examination center and public as a normal control group, this group was selected by stratified random sampling referring to the age and sex of type 2 diabetes group. The exclusion criteria were: a history of diabetes-related treatment and taking medication for serious diseases; patient had taken health care products, bulking agents, antidiarrheals, antispasmodics, probiotics, or antibiotics within the 3 months before the study or during the test period; and a history of smoking. Pregnant, breastfeeding or menstruating women were also excluded. All procedures performed in this study involving human participants were in accordance with the Declaration of Helsinki (as revised in 2013). This study was approved by the Ethics Committee of the Affiliated Hospital of Chengdu University of TCM (2020KL-031). Patients who met the inclusion criteria were informed of the details of the research and signed an informed consent form. We collected the volunteers’ venous blood samples and stool samples, and some basic biochemical indexes were detected immediately. Demographic characteristics, basic indicators for auxiliary diagnosis of T2DM including HAlbc, FBG, TG, TC, as well as insulin and so on, were recorded. And subsequently 16 srRNA third-generation full-length sequencing, microRNA high-throughput sequencing bioinformatics analysis, and statistical methods were applied to detect variations in gut microbiota and the levels of circulating miRNAs in plasma between T2DM patients and healthy individuals.
srRNA third-generation full-length sequencing of gut microbiota
Fecal specimens were collected from the subjects in normal control group (n=30) and T2DM group (n=30). First, total DNA was extracted from the samples using the PowerSoil® DNA Isolation kit according to the manufacturer’s instructions. After extraction, the 16S full-length primer was designed according to the conservative region 27F-1492R, for polymerase chain reaction (PCR) amplification of the target area (10 µL system, Solexa PCR). The synthesized specific primers (27F-1492R) were designed as follows:
- Forward primer 27F: AGRGTTTGATYNTGGCTCAG
- Reverse primer 1492R: TASGGHTACCTTGTTASGACTT
Subsequently, quality inspection was performed on the formed sequencing library, and processing, including barcode recognition, was performed on the high-quality circular consensus sequencing (CCS) sequence obtained. The generated optimization CCS was clustered at the level of 97% similarity (USEARCH, version 10.0), and its species classification was obtained based on the sequence composition of the operational taxonomic unit (OTU). The platforms 16S: Silva database and RDP Classifier were used to analyze species annotation and taxonomy as well as the diversity of gut microbiota. Alpha diversity analysis was performed to examine the species richness and diversity within a single sample, and the ACE, Chao, Shannon, and Simpson indices of each sample were calculated. The differences in the community composition and structure of different samples were compared through Beta diversity analysis. Finally, Metastats analysis was carried out to compare the significant differences between the groups at the genus level, and linear discriminant analysis effect size (LEfSe) analysis was used to screen biomarkers that were statistically different between the groups (biomarker screening criteria: LDA score >4).
Extraction of circulating microRNAs from plasma and microRNA high-throughput sequencing
Total RNA was extracted from the circulating plasma of 5 T2DM patients and 5 normal controls, drawn by random number table method, using the miRNeasy Serum/Plasma Kit (Qiagen, Hilden, Germany). Agilent 2100 Eukaryote RNA Pico (Agilent, Santa Clara, CA, USA) was then used to test the concentration and purity of 1.5 µL samples of the extracted total RNA. The remaining total RNA was stored at −80 °C. Quality inspection standards: total RNA >2.5 ng, RNA concentration >500 pg/µL, 28S/18S ≥1.5; no increase in baseline; 5S peak was normal. After qualification, the NEBnext multiplex small RNA (sRNA) library prep kit was used to build the library. Low-quality sequences were removed, as were reads with more than 10% unrecognizable bases. The RNA 3'end and 5'end adapters were connected, and reverse transcription was carried out to obtain cDNA. Then, PCR amplification of the target region with primers on the adapter was performed. After amplification, 140–160 bp fragments of DNA were recovered with polyacrylamide gel electrophoresis (PAGE). Agilent 2100 was used for detection, and after qualification, these fragments were sequenced using the Illumina NOVA platform. Then, Bowtie software was employed to classify and annotate sRNA, and unannotated reads were obtained by comparing clean reads with the database. Homo sapiens. GRCh38_release95 was used as the reference genome for sequence alignment, and the position information of the reference genome was obtained for the subsequent cluster analysis.
Bioinformatics prediction
The PICRUSt algorithm, based on the species composition information obtained from 16S sequencing data, was applied to infer the functional gene composition of the target bacteria, to obtain the Kyoto Encyclopedia of Genes and Genomes (KEGG) information corresponding to the OTU, and to analyze the differences in function. Additionally, according to the results of microRNA high-throughput sequencing analysis, the screened-out statistically significant miRNAs were predicted using TargetScan, and then Metascape was used to perform pathway enrichment analysis of target genes using the Gene Ontology biological process (GO BP), KEGG Pathway, and Canonical Pathway databases. P<0.01 was statistically significant.
Statistical analysis
Data were analyzed with SPSS version 23.0 (Statistical Package for the Social Science, Chicago, IL, USA). Continuous data were expressed as
Results
Baseline characteristics
There was no statistical difference in demographic characteristics of sex distribution and age between the two groups (P >0.05), while the body mass index (BMI) was calculated from the following formula: weight (kg)/height (m2) is significantly different (P<0.001) (Table 1). The basic blood biochemical indicators of the T2DM patients and normal controls are shown in Figure 1A. Among them, the HAlbc, FBG, TG, LDL, and insulin of T2DM patients were significantly increased compared to those of the normal controls, while the HDL was decreasing, with statistical significance (P<0.001, P<0.001, P=0.0125, P<0.001, P=0.022, and P=0.0013, respectively) (Figure 1A).
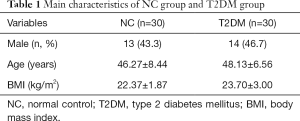
Full table
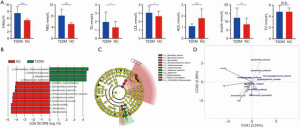
Third-generation full-length sequencing analysis of 16srRNA of gut microbiota
From the 60 samples sequenced, 514,080 CCS sequences were obtained after barcode identification. Each sample produced at least 428 CCS sequences, with an average of 8,568 CCS sequences. The number of OTUs, and the chao and ACE indexes of the two groups were statistically different (P=0.01, 0.007, and 0.037, respectively), which suggested that T2DM may cause changes in intestinal microbial diversity. Shannon and Simpson index analyses showed that the depth of sequencing was sufficient to capture all bacterial species and that the samples were representative. The composition of the differences between the two groups was preliminarily interpreted by analyzing α diversity and β diversity. At the phylum level, Firmicutes, Bacteroides, Proteobacteria, and Actinomycetes were the dominant species of gut microbiota in the two groups, and all were found in relatively high proportions. Firmicutes, Bacteroides, and Proteobacteria accounted for 40.5%, 45.1%, and 18.7%, respectively, of gut microbiota in the normal control group, compared with 47.4%, 25.8%, and 21.4%, respectively, in the T2DM group. The difference in Bacteroides as the dominant flora was statistically significant (P=0.0049), and the ratio of Bacteroides to Firmicutes was reduced in the T2DM group. LEfSe analysis identified 53 significantly different species between the two groups (biomarker screening criteria: LDA score >4) (10) (P<0.05) (Figure 1B,C). The results of CCA canonical correlation analysis of the correlation between the levels of different gut microbiota and basic indicators are shown in Figure 1D.
miRNAs high-throughput sequencing analysis
Through miRNA high-throughput sequencing analysis, 4,542 miRNAs were obtained from all samples, including 1,913 known miRNAs and 2,629 newly predicted miRNAs. The number of newly predicted miRNAs in the normal control group was significantly higher than that in the T2DM group. With FDR<0.05 and fold change (FC ≥2) set as the screening criteria, 405 circulating miRNAs differentially expressed between the groups were initially screened using a volcano map, and the undetectable miRNAs and non-human miRNAs were eliminated. Compared with the control group, the expression of 88 miRNAs was significantly down-regulated and the expression of 22 miRNAs was significantly up-regulated in the newly diagnosed T2DM group (Figure 2A). Nine candidate miRNAs with significant differential expression in T2DM group were selected; these included miR-122-5p, miR-193a, miR-3151, miR-582-3p, and miR-184, which were significantly down-regulated, and miR-200a-3p, miR-487a, miR-34c-3p, and miR-449a, which were up-regulated. Subsequently, correlation and association analyses of the gut microbiota were performed.
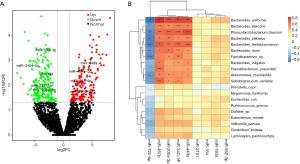
Correlation and association analysis
Spearman’s correlation analysis was performed. Figure 2B shows a heat map depicting five miRNAs that have statistically significant positive and negative correlations with certain microflora (P<0.05). Among these five miRNAs, miR-122-5p is highly conserved in the evolution of mammals and is related to eight types of intestinal bacteria, including two types of intestinal bacteria [Bacteroides.uniformis (B.uniformis) and Phascolarctobacterium.faecium (P.faecium)] that are significantly different (P=0.023 and 0.031, respectively) before and after T2DM disease onset (r=−0.68 and −0.60; P=0.01 and 0.01, respectively). After the selection of intestinal bacteria species with differences between groups, bioinformatics methods were used to further predict and analyze the potential pathogenic mechanism of the interaction between miR-122-5p and related intestinal bacteria.
Bioinformatics prediction results
To confirm the findings of the analysis of the interaction between certain gut microbiota and miRNAs, bioinformatics prediction and correlation analyses were conducted. The KEGG information corresponding to the OTU was obtained using PICRUSt software (Figure 3A). Some differences in the metabolic pathways of functional genes of intestinal bacterias such as B.uniformis, B.plebeius, B.taiotaomicron, P.faecium, and Faecalibacterium.sp were observed. These intestinal bacterias were found to play an important regulatory role in the metabolism of amino acids, carbohydrates, polysaccharides, vitamins, cofactors, membrane transport and energy conversion. Due to the significant differences in B.uniformis and P.faecium between the normal control and T2DM groups, CCA analysis of gut microbiota and blood indicators was carried out and showed that B.uniformis and P.faecium were negatively correlated with insulin, FBG, LDL, and TG. B. uniformis has been confirmed to be related to autoimmunity, and in animal models, has been found to have considerable efficacy in treating metabolic disorders and immune function disorders. Oral administration of this strain can reduce serum cholesterol, triglycerides, glucose, and insulin in high-fat diet (HFD)-fed mice, as well as leptin levels, and can improve tolerance to oral glucose. An increase in the abundance of B.uniformis has been shown to down-regulate inflammatory cytokines and play a key role in improving low-grade inflammation and insulin sensitivity (11,12).
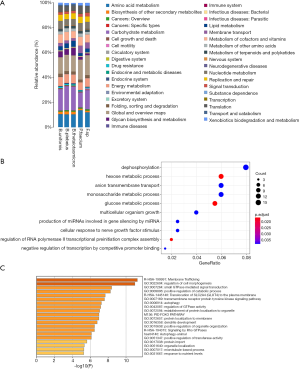
Next, TargetScan Human 2.1 was used to predict miR-122-5p target genes. A total of 226 target genes were predicted. The clusterprofiler package of R Studio was used to perform GO enrichment analysis of all predicted target genes, and the results are visualized in Figure 3B. The Metascpe platform was also used to perform for enrichment analysis using the GO BP, KEGG Pathway, Reactome Pathway, Canonical Pathway databases. Forty-eight biologically corresponding metabolic pathways with statistical significance (P<0.01) were obtained for the target gene set relative to the background. Figure 3C shows the top 20 enriched pathways with functional redundancy removed (13). miR-122-5p was found to be significantly enriched in glucose metabolism-related signaling pathways (P<0.01), including the gluconeogenesis and glycolysis pathways. The target genes of the regulated signal pathways included glucose 6-phosphatase (G6PC), G6PC3, PDK, KAT2A, and many other genes; these genes have been proved to have a direct regulatory effect on sugar metabolism and energy metabolism (14-16). Further, the significantly enriched HIF signaling pathway also involves several miR-122-5p target genes. HIF signaling pathway has been demonstrated to be related to the expression of glucose-producing enzyme genes, such as Pepck, G6PC, and glucose transporter type 2 (GLUT2), which can promote cells metabolism by increasing the expression of GLUT1 uptake and up-regulate the expression of PDK and other enzymes to increase glycolysis (17-19).
Discussion
In normal circumstances, the processes of insulin synthesis and secretion are both regulated by the level of glucose in pancreatic cells. However, under pathological conditions, continuous gluconeogenesis causes the glucose levels in the body to increase. The body responds by oversecreting insulin in an attempt to maintain stable blood glucose metabolism. Free fatty acids produced by the increase in fat mobilization enter and accumulate in the liver, simultaneously inhibiting insulin signal transduction and weakening tissue sensitivity to insulin. This eventually leads to the development of insulin resistance (20). When T2DM occurs, the imbalance between the abnormal glycoside production pathway and the body’s insulin action in turn induces chronic hyperglycemia in the body. The involvement of gut microbiota and miRNAs in the regulation of glucose and lipid metabolism has been supported by many studies. For instance, Clark and Mach found that the metabolites of gut microbiota can indirectly regulate key transcriptional co-activators, transcription factors, and enzymes related to mitochondrial biogenesis through regulating the endocrine and immune systems, and participate in the metabolism of non-bound lipids and glucose, as well as energy conversion function adjustment (21). MiRNAs can directly or indirectly affect almost all biological processes of the body. They participate in the uptake and consumption of glucose by affecting the expression levels of cellular glucose transporters and the efficiency of intracellular glucose metabolism, and then indirectly regulating insulin signal transduction (22). Furthermore, the gut microbiota in the current study is found to interact with miRNAs to play an authoritative role in the occurrence and progression of multiple diseases. Therefore, to investigate insulin intolerance at the initial stage of T2DM, our study drew on the combination of the recent research hotspots of gut microbiota and miRNA in an effort to further clarify their role in the molecular mechanism of the pathogenesis of T2DM. We hope that our findings will lead to a breakthrough in the efforts to discover a key molecular target for the treatment of T2DM.
Our research data show that newly diagnosed patients with T2DM have specific gut microbiota distribution and miRNA expression characteristics, with some significantly different miRNAs showing a statistically significant positive or negative correlation with certain microflora (P<0.05). It can be inferred that these miRNAs may facilitate host control of the gut microbiota by functioning as selective regulators of bacteria gene transcripts and growth (23). Among them, miR-122-5p is highly conserved in the evolution of mammals and is significantly related to a variety of intestinal bacteria, including two intestinal bacteria that are significantly different before and after T2DM (r=−0.68 and −0.60; P=0.01 and 0.01, respectively). Bioinformatics and statistical methods were used to analyze the potential pathogenic mechanisms of B.uniformis, P.faecium, and miR-122-5p, and their possible mechanisms in maintaining body homeostasis and shaping the host’s regulation of T2DM were explored. Through KEGG metabolic pathway analysis, some differences in the metabolic pathways of the functional genes of B.uniformis and P.faecium were observed. CCA analysis showed that both bacteria were negatively related to insulin, fasting glucose, LDL, and TG. Previous studies have proved that B.uniformis has a key regulatory role in liver metabolism (24). It is negatively related to liver G6PC, which reduces the ectopic lipid deposition and endoplasmic reticulum stress in the liver and muscle tissues, this regulatory mechanism can improve the liver glucose metabolism phenotype of mice. B.uniformis has strong glycolytic ability and can utilize diet and endogenous polysaccharides to provide bacteria and hosts with more beneficial end products, such as butyrate. It also participates in the metabolism of polyphenols in the form of O-glycosylation and C-terminal fission, and regulates plasma insulin and glucagon-like peptides by indirectly regulating key gluconeogenesis genes, such as peroxisome proliferator activated receptor γ (PPARγ), to improve glucose homeostasis and increase insulin sensitivity (11,25,26). Combined with the results of this test and previous studies, B.uniformis has been suggested to play an important role in glucose metabolism and plasma insulin synthesis and secretion.
The initial transcription of miR-122 is derived from the shearing of the her gene transcript and is located on human chromosome 18, 18q21.31 (27). miR-122-5p is continuously expressed and is highly conserved during the development of the liver in vertebrates, and plays a vital role in the homeostasis of liver glucose and lipid metabolism (28). In this study’s bioinformatics analysis of the miR-122-5p target gene set, many signal transduction pathways were found to be closely related to glucose metabolism pathways, including the gluconeogenesis and glycolysis pathways. These pathways are related to G6PC, PEPCK, PDK, KAT2A, and GLUT2, which are key genes involved in sugar metabolism. Previous studies have also revealed that the miR-122 family can maintain the body’s energy requirements by participating in pyruvate metabolism, glycolysis/gluconeogenesis, and the citric acid cycle (TCA cycle), and can indirectly regulate insulin resistance, miR-122 plays an important role in reversing insulin resistance (29,30). The miR-122-5p target gene human headbox transcription protein O (FoxO) is also closely related to the sugar metabolism pathway. FoxO, as a signal of liver insulin conduction which involved in conducting insulin signaling and an important regulator of gluconeogenesis-related genes, can combine with the promoter region of genes encoding key gluconeogenesis enzymes to control insulin-mediated glucose uptake (31). Interestingly, the expression of FoxO can be induced to regulate glucose metabolism and mitochondrial biogenesis by PPARγ (32), which is mentioned above can be regulated by B.uniformis when it play a regulative role of plasma insulin and glucagon-like peptides (25,26). Silencing of miR-122 has been shown to inhibit gluconeogenesis in the livers of miR-122 knockout (KO) mice, which manifested as a decrease in the levels of phosphoenolpyruvate and glucose-6-phosphate (G6P), leading to glucose intolerance and insulin resistance (31,33,34). As a new circulatory factor involved in insulin resistance, miR-122-5p was found to be able to mediate changes in the expression levels of key genes involved in insulin signaling, including GLUT4 and IRS1 (35), and to target the insulin-like growth factor receptor (IGF-1R) to regulate insulin resistance in liver cells (36). Therefore, from these perspectives, the molecular mechanism involved in the abnormal expression of miR-122-5p can be considered to be a new therapeutic target for T2DM.
In conclusion, our research has illuminated the different expression characteristics of the host gut microbiota and miRNAs before and after the illness of T2DM onset patients. Under the precondition that there is an interconnected cycle between miRNAs and intestinal microbes, we have reason to believe that miR-122-5p and B.uniformis are likely to be regulated by each other in the process of regulating gluconeogenesis genes and insulin signal transmission, and play an authoritative role in controlling the homeostasis of glucose metabolism and insulin secretion. The crosstalk network of circulating miRNAs and gut microbiota is a way to maintain the stability and state of the host’s body, and shape another mechanism for the regulation of T2DM. However, further study is needed to determine the genes involved in sugar metabolism that are jointly controlled by the two, as well as the specific signaling pathways affecting the function of pancreatic islet cells and the synthesis and secretion of insulin. At the same time, this experiment has certain limitations, such as the small number of specimens and the inability to strictly control the recent lifestyles of volunteers, which may have impacted on the results. Therefore, considering the persistently increasing incidence of T2DM and the lack of specific treatment, the value of gut microbiota and miRNA as potential therapeutic targets needs to be further investigated. More rigorous and in-depth in vivo and in vitro experiments will be conducted to verify the results of this study in order to obtain stronger evidence at the level of molecular action and to bring new opportunities for the early diagnosis and precise treatment of T2DM.
Acknowledgments
Funding: Key Project of Sichuan Provincial Department of Science and Technology (2020YFS0375).
Footnote
Reporting Checklist: The authors have completed the STROBE reporting checklist. Available at http://dx.doi.org/10.21037/atm-20-6717
Data Sharing Statement: Available at http://dx.doi.org/10.21037/atm-20-6717
Conflicts of Interest: All authors have completed the ICMJE uniform disclosure form (available at http://dx.doi.org/10.21037/atm-20-6717). The authors have no conflicts of interest to declare.
Ethical Statement: The authors are accountable for all aspects of the work in ensuring that questions related to the accuracy or integrity of any part of the work are appropriately investigated and resolved. All procedures performed in this study involving human participants were in accordance with the Declaration of Helsinki (as revised in 2013). This study was approved by the Ethics Committee of the Affiliated Hospital of Chengdu University of TCM (Approval number: 2020KL-031) and informed consent was taken from all the patients.
Open Access Statement: This is an Open Access article distributed in accordance with the Creative Commons Attribution-NonCommercial-NoDerivs 4.0 International License (CC BY-NC-ND 4.0), which permits the non-commercial replication and distribution of the article with the strict proviso that no changes or edits are made and the original work is properly cited (including links to both the formal publication through the relevant DOI and the license). See: https://creativecommons.org/licenses/by-nc-nd/4.0/.
References
- Cho NH, Shaw JE, Karuranga S, et al. IDF Diabetes Atlas: Global estimates of diabetes prevalence for 2017 and projections for 2045. Diabetes Res Clin Pract 2018;138:271-81. [Crossref] [PubMed]
- Rathmann W, Giani G. Global prevalence of diabetes: estimates for the year 2000 and projections for 2030. Diabetes care 2004;27:2568-9; author reply 2569. [Crossref] [PubMed]
- Hall V, Thomsen R, Henriksen O, et al. Diabetes in Sub Saharan Africa 1999-2011: epidemiology and public health implications. A systematic review. BMC public health 2011;11:564. [Crossref] [PubMed]
- Sender R, Fuchs S, Milo R. Revised Estimates for the Number of Human and Bacteria Cells in the Body. PLoS Biol 2016;14:e1002533. [Crossref] [PubMed]
- Ferruelo A, Peñuelas Ó, Lorente JA. MicroRNAs as biomarkers of acute lung injury. Ann Transl Med 2018;6:34. [Crossref] [PubMed]
- Al-Kafaji G, Al-Mahroos G, Alsayed NA, et al. Peripheral blood microRNA-15a is a potential biomarker for type 2 diabetes mellitus and pre-diabetes. Mol Med Rep 2015;12:7485-90. [Crossref] [PubMed]
- Teng Y, Ren Y, Sayed M, et al. Plant-Derived Exosomal MicroRNAs Shape the Gut Microbiota. Cell Host Microbe 2018;24:637-52.e8. [Crossref] [PubMed]
- Williams MR, Stedtfeld RD, Tiedje JM, et al. MicroRNAs-Based Inter-Domain Communication between the Host and Members of the Gut Microbiome. Front Microbiol 2017;8:1896. [Crossref] [PubMed]
- Alberti KG, Zimmet PZ. Definition, diagnosis and classification of diabetes mellitus and its complications. Part 1: diagnosis and classification of diabetes mellitus provisional report of a WHO consultation. Diabet Med 1998;15:539-53. [Crossref] [PubMed]
- Segata N, Izard J, Waldron L, et al. Metagenomic biomarker discovery and explanation. Genome Biol 2011;12:R60. [Crossref] [PubMed]
- Corrêa TA, Rogero MM, Hassimotto NMA, et al. The Two-Way Polyphenols-Microbiota Interactions and Their Effects on Obesity and Related Metabolic Diseases. Front Nutr 2019;6:188. [Crossref] [PubMed]
- Gauffin Cano P, Santacruz A, Moya A, et al. Bacteroides uniformis CECT 7771 ameliorates metabolic and immunological dysfunction in mice with high-fat-diet induced obesity. PLoS One 2012;7:e41079. [Crossref] [PubMed]
- Zhou Y, Zhou B, Pache L, et al. Metascape provides a biologist-oriented resource for the analysis of systems-level datasets. Nat Commun 2019;10:1523. [Crossref] [PubMed]
- Agudelo LZ, Ferreira DMS, Dadvar S, et al. Skeletal muscle PGC-1alpha1 reroutes kynurenine metabolism to increase energy efficiency and fatigue-resistance. Nat Commun 2019;10:2767. [Crossref] [PubMed]
- Kalemba KM, Wang Y, Xu H, et al. Glycerol induces G6pc in primary mouse hepatocytes and is the preferred substrate for gluconeogenesis both in vitro and in vivo. J Biol Chem 2019;294:18017-28. [Crossref] [PubMed]
- Park BY, Jeon JH, Go Y, et al. PDK4 Deficiency Suppresses Hepatic Glucagon Signaling by Decreasing cAMP Levels. Diabetes 2018;67:2054-68. [Crossref] [PubMed]
- Hu CJ, Wang LY, Chodosh LA, et al. Differential roles of hypoxia-inducible factor 1alpha (HIF-1alpha) and HIF-2alpha in hypoxic gene regulation. Mol Cell Biol 2003;23:9361-74. [Crossref] [PubMed]
- Pescador N, Villar D, Cifuentes D, et al. Hypoxia promotes glycogen accumulation through hypoxia inducible factor (HIF)-mediated induction of glycogen synthase 1. PLoS One 2010;5:e9644. [Crossref] [PubMed]
- Rankin EB, Rha J, Selak MA, et al. Hypoxia-inducible factor 2 regulates hepatic lipid metabolism. Mol Cell Biol 2009;29:4527-38. [Crossref] [PubMed]
- Wang Y, Xue J, Li Y, et al. Telmisartan protects against high glucose/high lipid-induced apoptosis and insulin secretion by reducing the oxidative and ER stress. Cell Biochem Funct 2019;37:161-8. [Crossref] [PubMed]
- Clark A, Mach N. The Crosstalk between the Gut Microbiota and Mitochondria during Exercise. Front Physiol 2017;8:319. [Crossref] [PubMed]
- Handschin C, Spiegelman BM. Peroxisome proliferator-activated receptor gamma coactivator 1 coactivators, energy homeostasis, and metabolism. Endocr Rev 2006;27:728-35. [Crossref] [PubMed]
- Liu S, da Cunha AP, Rezende RM, et al. The Host Shapes the Gut Microbiota via Fecal MicroRNA. Cell Host Microbe 2016;19:32-43. [Crossref] [PubMed]
- Xie G, Wang X, Liu P, et al. Distinctly altered gut microbiota in the progression of liver disease. Oncotarget 2016;7:19355-66. [Crossref] [PubMed]
- Benítez-Páez A, Gomez Del Pulgar EM, Sanz Y. The Glycolytic Versatility of Bacteroides uniformis CECT 7771 and Its Genome Response to Oligo and Polysaccharides. Front Cell Infect Microbiol 2017;7:383. [Crossref] [PubMed]
- Rodrigues RR, Greer RL, Dong X, et al. Antibiotic-Induced Alterations in Gut Microbiota Are Associated with Changes in Glucose Metabolism in Healthy Mice. Front Microbiol 2017;8:2306. [Crossref] [PubMed]
- Wu Q, Liu HO, Liu YD, et al. Decreased expression of hepatocyte nuclear factor 4alpha (Hnf4alpha)/microRNA-122 (miR-122) axis in hepatitis B virus-associated hepatocellular carcinoma enhances potential oncogenic GALNT10 protein activity. J Biol Chem 2015;290:1170-85. [Crossref] [PubMed]
- Thakral S, Ghoshal K. miR-122 is a unique molecule with great potential in diagnosis, prognosis of liver disease, and therapy both as miRNA mimic and antimir. Curr Gene Ther 2015;15:142-50. [Crossref] [PubMed]
- Castaño C, Kalko S, Novials A, et al. Obesity-associated exosomal miRNAs modulate glucose and lipid metabolism in mice. Proc Natl Acad Sci U S A 2018;115:12158-63. [Crossref] [PubMed]
- Sun J, Liu Q, Zhao L, et al. Potential regulation by miRNAs on glucose metabolism in liver of common carp (Cyprinus carpio) at different temperatures. Comp Biochem Physiol Part D Genomics Proteomics 2019;32:100628. [Crossref] [PubMed]
- Penniman CM, Suarez Beltran PA, Bhardwaj G, et al. Loss of FoxOs in muscle reveals sex-based differences in insulin sensitivity but mitigates diet-induced obesity. Mol Metab 2019;30:203-20. [Crossref] [PubMed]
- Barthel A, Schmoll D, Unterman TG. FoxO proteins in insulin action and metabolism. Trends Endocrinol Metab 2005;16:183-9. [Crossref] [PubMed]
- Barajas JM, Reyes R, Guerrero MJ, et al. The role of miR-122 in the dysregulation of glucose-6-phosphate dehydrogenase (G6PD) expression in hepatocellular cancer. Sci Rep 2018;8:9105. [Crossref] [PubMed]
- Sengupta D, Cassel T, Teng KY, et al. Regulation of hepatic glutamine metabolism by miR-122. Mol Metab 2020;34:174-86. [Crossref] [PubMed]
- Sendi H, Mead I, Wan M, et al. miR-122 inhibition in a human liver organoid model leads to liver inflammation, necrosis, steatofibrosis and dysregulated insulin signaling. PLoS One 2018;13:e0200847. [Crossref] [PubMed]
- Dong L, Hou X, Liu F, et al. Regulation of insulin resistance by targeting the insulin-like growth factor 1 receptor with microRNA-122-5p in hepatic cells. Cell Biol Int 2019;43:553-64. [Crossref] [PubMed]