Coronary artery disease: differential expression of ceRNAs and interaction analyses
Introduction
Coronary artery disease (CAD) is a complex phenotype that is driven by a range of genetic and environmental factors. However, current therapies for CAD focus solely on addressing the role of cholesterol and lifestyle. Despite advances in lipid-lowering therapies, clinical trials have shown that the recommended strategies for therapeutic intervention at present can leave patients with a substantial risk of cardiovascular disease (1). It is important to stratify the risk of subsequent coronary events in patients with CAD because this may be able to help guide the application of secondary preventive therapies. In a recent study, eight microRNAs (miRNAs) were identified and used to facilitate the diagnosis of the acute coronary syndrome (2). Another study showed that targeting Angptl3 messenger RNA (mRNA) was able to retard the progression of atherosclerosis and reduce the levels of atherogenic lipoproteins (3). The expressing 9p21.3-associated long non-coding RNA (lncRNA) ANRIL has also been shown to increase the risk of CAD phenotypes in non-risk vascular smooth muscle cells (4). However, the mechanisms underlying these RNA interactions have not yet been elucidated.
Previous research has also reported the existence of different types of RNA molecules that complete with other forms of RNA to bind to miRNA, thus reducing the inhibitory effect of miRNA targeting to mRNA (5). This group of competitive endogenous RNAs (ceRNAs) included various types of RNA transcripts, including lncRNAs, pseudogenes, and protein-encoded mRNAs; these forms of RNA competed for miRNA via mechanisms that are mediated by the miRNA response element (MRE) (6). More recently, researchers used bioinformatic techniques to predict regulatory networks involving ceRNAs. The effects of such ceRNAs on target genes, and the dependence of ceRNAs on miRNAs, can be verified by in vitro experiments involving proteins and RNAs, although functional verification can only be acquired by performing in vivo experiments in cells and animal models.
In the present study, we investigated the differential expression of mRNAs, lncRNAs and miRNAs between tissues from patients with CAD and healthy controls. We also investigated the interactions between these different forms of RNA and constructed a ceRNA regulatory network. Collectively, our findings will provide useful tools to investigate the molecular mechanisms underlying CAD. We present the following article in accordance with the MDAR reporting checklist (available at http://dx.doi.org/10.21037/atm-20-3487).
Methods
Data preprocessing
First, we used public databases to search for mRNA, lncRNA, and miRNA data relating to CAD, however, we were unable to find such data in public databases. Next, we focused on the relationship between lncRNA and mRNA. Finally, we selected a set of miRNA data that we could be used to accurately investigate the regulatory relationships between lncRNA, miRNA, and mRNA. The miRNA expression profile data that we used in this study (dataset serial number: GSE59421) were acquired from the NCBI (Gene Expression Omnibus, GEO, http://www.ncbi.nlm.nih.gov/geo/) Database (7); these data were normalized and then downloaded. A total of 96 blood samples (63 samples from healthy controls and 33 samples from patients with CAD) were acquired and detected using an Agilent 021827 Human miRNA MicroArray (V3) platform (miRBase release 12.0 miRNA ID version).
We also acquired mRNA and lncRNA data from the NCBI GEO database; dataset serial number GSE42148 were firstly normalized and then downloaded for analysis. A total of 24 blood samples (11 controls and 13 patients with CAD) were detected using an Agilent 028004 SurePrintG3 Human GE 8x60K Microarray platform (Feature Number version).
Because the CAD chip data were acquired from a public database, we ensured that we used the same inclusion and exclusion criteria for CAD and controls, as described in these data which were derived from dataset serial numbers GSE59421 and GSE42148 in the NCBI GEO database. The study was approved by the ethics board of Shanghai East hospital (No. 2020161), and granted a waiver of consent for the data analysis. The study was conducted in accordance with the Declaration of Helsinki (as revised in 2013).
Annotation of mRNAs and lncRNAs
The sequences that matched with probes used on the Agilent-028004 were acquired from the platform annotation file and human reference genome sequences (GRCh38) were downloaded from the GENCODE database (https://www.gencodegenes.org/releases/current.html) (8). Probe sequences were then aligned onto the reference genome using seqmap software (9). We retained the uniquely aligned (uniquely mapped) probes, and also referred their relative positions on the chromosome using positive and negative strand information. The gene that matched with each probe was then identified according to the human gene annotation file (Release 25) provided by GENCODE.
The probe with “protein_coding” annotation was used as the matching probe for mRNA, while probes with “antisense”, “sense_intronic”, “lincRNA”, “sense_overlapping” or “processed_transcript” annotation were used as matching probes for lncRNA.
Finally, the probe numbers and mRNAs/lncRNAs (by gene symbol) were matched one-by-one to remove probes that did not match the gene symbol. When different probes mapped to the same gene, we used an average of the data derived from different probes as the final expression values for individual mRNAs and lncRNAs.
Screening for differentially expressed mRNAs, lncRNAs and miRNAs
We used the limma package in R software with classical Bayesian methodology (version 3.10.3, http://www.bioconductor.org/packages/2.9/bioc/html/limma.html) (10). We then performed differential analysis between CAD and control samples. When analyzing miRNAs, mRNAs, and lncRNAs, we considered both the fold difference of logFC values and the statistical significance (P values). With regards to P values, several papers have reported different thresholds for differential analyses. We compared a variety of thresholds to screen differentially expressed genes; we found that results were optimized when we selected a significance level of P<0.05, as described previously (11). In our analyses, the threshold for differential expression was to a miRNA P value <0.05 and a |logFC| >0.263 (>1.2-fold).
Functional enrichment and pathway analysis of differentially expressed mRNAs
Enrichment analysis was performed using DAVID, a powerful tool for the analysis of enrichment (version 6.8, https://david-d.ncifcrf.gov/); this allowed us to analyze up- and down-regulated genes (12), and map their association with several aspects of Gene Ontology (GO): biological process (BP), cellular component (CC), and molecular function (MF) (13). We also performed Kyoto Encyclopedia of Genes and Genomes (KEGG) analyses (14). Significant enrichment was considered when P<0.05 and at least 2 of enrichment number (count).
Construction of a protein- protein interaction (PPI) network and node connectivity analysis
Next, we used the STRING database (version: 10.0, http://www.string-db.org/) (15) to predict whether there were any specific interactions/relationships between the proteins encoded by the differentially expressed genes. The differentially expressed mRNAs were analyzed as gene sets with the species set to ‘homo’. The PPI score was set to 0.9 (highest confidence) and we looked for protein nodes (interactions) for genes that were up- or down-regulated. Once we identified relationships in the PPI network, we used Cytoscape software (version 3.4.0, http://chianti.ucsd.edu/cytoscape-3.4.0/) (16) to create a network map. Next, node connectivity analysis was performed using non-weighted parameters and the CytoNCA plugin (Version 2.1.6, http://apps.cytoscape.org/apps/cytonca) (17). Data highlighted the important nodes within the PPI network that were involved in key protein interactions; in other words, this allowed us to identify the connectivity of hub proteins by ranking the degree centrality (DC) of individual nodes.
lncRNA and mRNA co-expression analysis
Next, we performed correlation analyses and calculated Pearson’s correlation coefficients for the differentially expressed mRNAs and lncRNAs; we did this by considering matching samples of mRNA and lncRNA. Pair with an r>0.7 (coordinate expression) and a P value <0.05 were subsequently used to screen the ceRNA network. In addition, these mRNAs were considered to be significantly correlated with lncRNAs; mRNAs were considered as potential target genes of the lncRNAs.
Identification of the target genes for miRNA and predicting the lncRNAs upstream
Next, the differentially expressed miRNAs were analyzed by the miRWalk2.0 database (http://zmf.umm.uni-heidelberg.de/apps/zmf/mirwalk2/) database (18), which featured four major components: miRWalk, miRanda, RNA22, and TargetScan. If the predicted target genes were present in all four of these key databases, then we considered the matching mRNA to be regulated by the miRNA. Following the prediction of miRNA-mRNA relationship pairs, the mRNAs were further intersected with the differential mRNAs in order to produce differentially expressed miRNA-mRNA relationship pairs.
Next, we used miRanda software (v3.3a) (19) to predict differentially expressed miRNA-lncRNA relationship pairs using a screen score (sc) ≥140 and an energy (en) ≤−20.
Pathway enrichment analysis for lncRNAs and miRNAs
Based on the identified lncRNA-mRNA co-expression relationship pairs, and the miRNA-mRNA relationship pairs, we then used mRNAs as potential target genes for matching lncRNAs and miRNAs, respectively. KEGG pathway enrichment analysis was then performed using the R package clusterProfiler (version: 3.8.1, http://bioconductor.org/packages/release/bioc/html/clusterProfiler.html) (20); the results of this analysis indirectly predicted the functional roles of the lncRNAs and miRNAs. The threshold for significance was set at P<0.05.
Construction of a ceRNA network
Using the identified mRNA-miRNA and lncRNA-miRNA relationship pairs, we then screened the miRNA-lncRNA-mRNA relationship pairs that were regulated by the same miRNA and then combined the positive co-expression relationship between mRNA and lncRNA (where the correlation coefficient >0.7). Next, we carried out further screening using the miRNA-lncRNA-mRNA relationship pairs in order to construct a ceRNA network. Consequently, the lncRNAs and mRNAs that were regulated by the same miRNAs and exhibited a positive co-expression relationship in the ceRNA network, these lncRNAs and mRNAs were all considered to be ceRNAs.
Finally, we carried out node connectivity (degree) analysis using the Cytoscape plugin CytoNCA using non-weighted parameters. The higher the connectivity, the higher the importance of a specific node within the network.
Results
Basic data relating to differential expression analyses
We identified a total of 456 miRNA expression profiles (Dataset 1), 16,325 mRNA expression profiles (Dataset 2), and 2,869 lncRNA expression profiles (see Dataset 3).
Eighteen differentially expressed miRNAs were identified according to our strict thresholds, including 16 that were down-regulated and 2 that were up-regulated (Dataset 4). A total of 92 differentially expressed lncRNAs were identified, including 46 that were down-regulated and 46 that were up-regulated (Dataset 5). A total of 610 differentially expressed mRNAs were identified, including 244 that were down-regulated and 366 that were up-regulated (Dataset 6).
Based on the identification of these differentially expressed miRNAs, lncRNAs, and mRNAs, we next created a heat map (Figure S1) and a volcano map (Figure S2).
Functional and pathway enrichment analysis for up- and down-regulated mRNAs
Next, we used the differentially expressed mRNAs in GO enrichment analyses with regards to BP, MF, and CC. This was then followed by KEGG pathway enrichment analyses. GO analyses identified enrichment in 36 BPs, 3 CCs, 15 MFs, and 2 KEGG pathways (Dataset 7). Figure S3 shows the top 10 enrichments, along with the corresponding P values. Key results arising from the GO and KEGG pathway enrichment analyses are shown in Table S1.
PPI network construction and module analysis
We identified a total of 388 protein interaction pairs; network construction was performed using Cytoscape software, as shown in Figure 1. The resultant network included a total of 171 nodes.
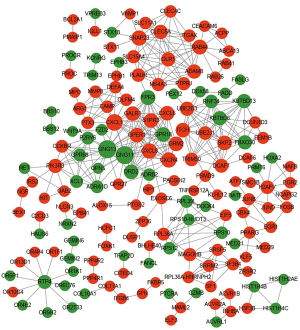
The network was analyzed for node connectivity according to specific parameters; the top 15 nodes, as ranked by DC, are shown in Table 1. CXCL8, FPR2, IL6, and PPBP, were all ranked in the top 15 and may represent the hub proteins in our network (Dataset 8). The top 15 nodes arising from connectivity ranking within the PPI network are shown in Table 1.
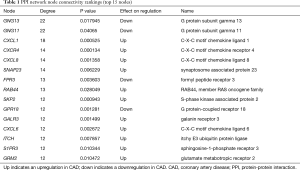
Full table
Co-expression analysis of mRNAs and lncRNAs
We performed co-expression analysis of differentially expressed mRNAs and lncRNAs. According to specific thresholds, we screened a total of 1,487 significant relationship pairs that showed the coordinated expression, including 381 mRNAs and 74 lncRNAs (Dataset 9).
miRNA target genes and upstream lncRNA prediction analysis
Based on the differentially expressed miRNAs and lncRNAs, we were able to predict a total of 452 lncRNA-miRNA relationship pairs (Dataset 10), including 18 miRNAs and 72 lncRNAs.
Furthermore, based on our differentially expressed miRNAs, we were able to perform target gene prediction using mirWalk. After considering the intersection formed by differentially expressed mRNAs, we were able to identify 276 miRNA-mRNA relationship pairs, including 17 miRNAs and 170 mRNAs (Dataset 11).
Pathway enrichment analysis for lncRNAs and miRNAs
KEGG pathway analysis identified a total of 27 lncRNAs and 12 miRNAs that showed enrichment (Datasets 12 and 13, respectively). These data are presented in Figure 2.
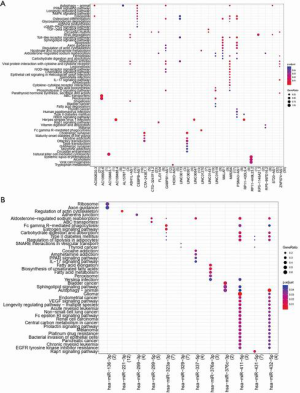
ceRNA network analysis
Next, we screened the identified miRNA-lncRNA-mRNA relationship pairs that were regulated by the same miRNAs, along with the positive co-expression relationships between mRNAs and lncRNAs (with a correlation coefficient >0.7). We also screened the lncRNA-miRNA-mRNA relationship pairs by constructing a ceRNA network (Figure 3). The network featured a total of 87 lncRNA-miRNA relationship pairs, 88 miRNA-mRNA relationship pairs, and 137 lncRNA-mRNA co-expression relationships (Dataset 14); in total, there were 36 lncRNAs, 64 mRNAs, and 15 miRNAs. Connectivity analysis was performed on each node of the ceRNA network to identify connectivity between mRNAs, miRNAs, and lncRNAs (Table 2).
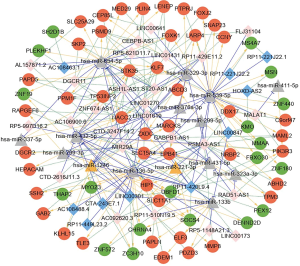
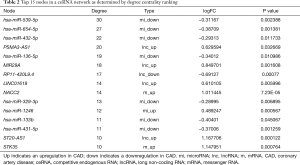
Full table
Discussion
The study aimed to identify statistically significant and differentially expressed genes between patients with CAD and healthy controls. With regards to connectivity, GO and KEGG analyses (count ≥9) identified the top 11 PPI network nodes in rank order. We also identified the top 15 significant nodes for ceRNAs that were identified according to degree centrality (P<0.05). Collectively, our analyses confirmed that the differential expression of certain ceRNAs, and their respective signaling pathways, were all associated with CAD.
GO and KEGG pathway analyses identified enrichment in 36 BPs, 3 CCs, 15 MFs, and 2 KEGG pathways (Table S1). These enrichments were all statistically significant and were probably associated with the development and progression of CAD. These enrichments should be investigated further in future research as they could form targets for diagnostic or therapeutic intervention.
By ranking the nodes in our PPI network by connectivity, we were able to identify the top 15 nodes and identified statistically significant protein interactions in CAD (Table 1) that were also associated with specific signaling pathways (Table 3). Some of these nodes and pathways were identified to play a known role in cardiovascular diseases. For example, GNG11 is a member of the gamma subunit family of heteromeric G-proteins. The over-expression of GNG11 has been shown to activate ERK1/2 in the MAP kinase family, but not Ras (21). These previous findings provide clinically relevant biological insight into the heritable variation of vagal heart rhythm regulation and demonstrate that genetic variants (GNG11, RGS6) play a key role by influencing the action of G-protein heterotrimers during GIRK channel- induced pacemaker membrane hyperpolarization (see Table 3) (22).
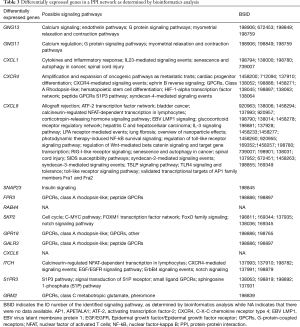
Full table
CXCL1 is known to be produced mainly by TNF-stimulated endothelial cells (ECs) and pericytes; these support luminal and sub-EC neutrophil crawling. CXCL1 and CXCL2 act in a sequential manner to guide neutrophils through venular walls as governed by their distinct cellular sources (23). The angiotensin II-induced infiltration of monocytes in the heart is largely mediated by CXCL1-CXCR2 signaling which initiates and aggravates cardiac remodeling. Consequently, the inhibition of CXCL1 and/or CXCR2 may represent new therapeutic targets for treating hypertensive heart diseases (see Table 3) (24).
Wnt-Cxcr4 (C-X-C motif chemokine receptor 4) signaling is known to regulate the interactions between oligodendrocyte precursor cells (OPCs) and endothelial cells and coordinates OPC migration and differentiation (25). It has been shown that a number of neutrophils re-enter the vasculature and follow a pre-programmed journey that entails transportation to the lungs to up-regulate CXCR4 before entering the bone marrow, where they subsequently undergo apoptosis (26). Vascular CXCR4 limits atherosclerosis by maintaining arterial integrity, and by preserving endothelial barrier function and a normal contractile smooth muscle cell phenotype. Enhancing these beneficial functions of arterial CXCR4 by selective modulators might provide new therapeutic options for atherosclerosis (Table 3) (27).
Oleic acid treatment has been shown to reduce the insulin sensitivity of heart muscle cells and that such sensitivity can be completely restored by transfection with SNAP23. Thus, SNAP23 might represent a link between insulin sensitivity and the flow of fatty acids into a cell (Table 3) (28).
Co-activator-associated arginine methyltransferase 1 (CARM1) is a crucial component of autophagy in mammals. CARM1-dependent histone arginine methylation is a crucial nuclear event in autophagy and takes part in the AMPK-SKP2-CARM1 signaling axis in the regulation of autophagy induction following nutrient starvation (Table 3) (29).
GPR18 is a cannabinoid-activated orphan G protein-coupled receptor (GPCR) that is selectively expressed on immune cells (30). GPR18 is known to play a role in the cardiovascular system, at least in part, by mediating the elevation of adiponectin levels (Table 3) (31).
By ranking the top 15 nodes in our ceRNA network by degree centrality, we identified a range of significant ceRNA interactions in CAD (see Table 2); these findings concurred with previous literature (see Table 4) (32-47). It was evident from our results that several ceRNAs were related to cardiovascular diseases. For example, by regulating CDKN2A and inhibiting G1- to S-phase transition, STK35L1 may act as a central kinase that links the cell cycle and migration of endothelial cells. Specifically, the interaction between STK35L1 and nuclear actin may be critical in the regulation of these fundamental endothelial functions (45). Serine/threonine kinase 35 (STK35) is a recently identified human kinase that plays a key role in autophosphorylation, which is functionally linked with actin stress fibers, cell cycle progression, and survival (see Table 4) (46). Microarray analysis previously showed that nuclear-retained importin α2 binds with DNase I-sensitive nuclear component(s) and controls the selective upregulation of mRNA encoding STK35. Chromatin immunoprecipitation and promoter analysis further demonstrated that importin α2 can access the promoter region of STK35 to accelerate transcription in response to hydrogen peroxide exposure. Furthermore, the constitutive overexpression of STK35 proteins enhances caspase-independent cell death under oxidative stress conditions (see Table 4) (47).
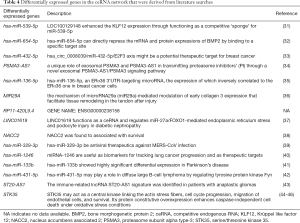
Full table
Our hypothesis was that the analysis of ceRNAs, which compete with miRNA for the MRE, might help us to distinguish between patients with CAD and controls. Our findings identified a significant number of differentially expressed genes and identified enrichment of these genes in CAD. We also identified key GO terms, pathways, and several key interactions by degree centrality ranking.
There are some limitations associated with our study that should be taken into consideration. For example, our bioinformatics analysis was based on GEO and could have been affected by variability between different subjects. Furthermore, the lncRNA molecules identified from specific tissues would acquire the reliable conclusion, thus the magnitude of connectivity and the matching lncRNA-miRNA-mRNA relationships should be combined in future experimental validations.
Conclusions
Our research identified 11 GO terms and KEGG pathways; based on these, we identified the top 15 nodes in a PPI network by connectivity ranking. We also identified the top 15 nodes in a ceRNA network by degree centrality ranking in a population of CAD subjects. Our findings are likely to facilitate the further investigation of molecular mechanisms underlying CAD.
Acknowledgments
We would like to thank Wei Song and Hong-Chun Fan for their technical support during this study.
Funding: This work was supported in part by a Project of National Natural Science Foundation of China (81870247 to SK), a Project of National Natural Science Foundation of China (81800224 to YY), a Project of National Natural Science Foundation of China (81770350 to HBL), a Key Disciplines Group Construction Project of Pudong Health Bureau of Shanghai (Grant number: PWZxq 2017-05), and a Top-level Clinical Discipline Project of Shanghai Pudong District (Grant number: PWYgf 2018-02).
Footnote
Reporting Checklist: The authors have completed the MDAR reporting checklist. Available at http://dx.doi.org/10.21037/atm-20-3487
Conflicts of Interest: All authors have completed the ICMJE uniform disclosure form (available at http://dx.doi.org/10.21037/atm-20-3487). The authors have no conflicts of interest to declare.
Ethical Statement: The authors are accountable for all aspects of the work in ensuring that questions related to the accuracy or integrity of any part of the work are appropriately investigated and resolved. This study involved bioinformatics analysis using data that were acquired from the databases regulated by the NCBI. As such, the study was approved by the ethics board of Shanghai East hospital (No. 2020161), and granted a waiver of consent for the data analysis. The study was conducted in accordance with the Declaration of Helsinki (as revised in 2013).
Open Access Statement: This is an Open Access article distributed in accordance with the Creative Commons Attribution-NonCommercial-NoDerivs 4.0 International License (CC BY-NC-ND 4.0), which permits the non-commercial replication and distribution of the article with the strict proviso that no changes or edits are made and the original work is properly cited (including links to both the formal publication through the relevant DOI and the license). See: https://creativecommons.org/licenses/by-nc-nd/4.0/.
References
- Cannon CP, Blazing MA, Giugliano RP, et al. Ezetimibe Added to Statin Therapy after Acute Coronary Syndromes. N Engl J Med 2015;372:2387-97. [Crossref] [PubMed]
- Karakas M, Schulte C, Appelbaum S, et al. Circulating microRNAs strongly predict cardiovascular death in patients with coronary artery disease-results from the large AtheroGene study. Eur Heart J 2017;38:516-23. [PubMed]
- Graham MJ, Lee RG, Brandt TA, et al. Cardiovascular and Metabolic Effects of ANGPTL3 Antisense Oligonucleotides. N Engl J Med 2017;377:222-32. [Crossref] [PubMed]
- Lo Sardo V, Chubukov P, Ferguson W, et al. Unveiling the Role of the Most Impactful Cardiovascular Risk Locus through Haplotype Editing. Cell 2018;175:1796-1810.e20. [Crossref] [PubMed]
- Salmena L, Poliseno L, Tay Y, et al. A ceRNA hypothesis: the Rosetta Stone of a hidden RNA language? Cell 2011;146:353-8. [Crossref] [PubMed]
- de Giorgio A, Krell J, Harding V, et al. Emerging roles of competing endogenous RNAs in cancer: insights from the regulation of PTEN. Mol Cell Biol 2013;33:3976-82. [Crossref] [PubMed]
- Barrett T, Suzek TO, Troup DB, et al. NCBI GEO: mining millions of expression profiles--database and tools. Nucleic Acids Res 2005;33:D562-6. [Crossref] [PubMed]
- Harrow J, Frankish A, Gonzalez JM, et al. GENCODE: the reference human genome annotation for The ENCODE Project. Genome Res 2012;22:1760-74. [Crossref] [PubMed]
- Jiang H, Wong WH. SeqMap: mapping massive amount of oligonucleotides to the genome. Bioinformatics 2008;24:2395-6. [Crossref] [PubMed]
- Smyth GK. limma: Linear Models for Microarray Data, in Bioinformatics and Computational Biology Solutions Using R and Bioconductor, R. Gentleman, et al., Editors. Springer New York: New York, NY. 2005:397-420.
- Wang X, Li G, Luo Q, et al. Identification of crucial genes associated with esophageal squamous cell carcinoma by gene expression profile analysis. Oncol Lett 2018;15:8983-90. [Crossref] [PubMed]
- Huang W, Sherman BT, Lempicki RA. Systematic and integrative analysis of large gene lists using DAVID bioinformatics resources. Nat Protoc 2009;4:44-57. [Crossref] [PubMed]
- Ashburner M, Ball CA, Blake JA, et al. Gene ontology: tool for the unification of biology. The Gene Ontology Consortium. Nat Genet 2000;25:25-9. [Crossref] [PubMed]
- Kanehisa M, Goto S. KEGG: kyoto encyclopedia of genes and genomes. Nucleic Acids Res 2000;28:27-30. [Crossref] [PubMed]
- Szklarczyk D, Franceschini A, Wyder S, et al. STRING v10: protein-protein interaction networks, integrated over the tree of life. Nucleic Acids Res 2015;43:D447-52. [Crossref] [PubMed]
- Shannon P, Markiel A, Ozier O, et al. Cytoscape: a software environment for integrated models of biomolecular interaction networks. Genome Res 2003;13:2498-504. [Crossref] [PubMed]
- Tang Y, Li M, Wang J, et al. CytoNCA: a cytoscape plugin for centrality analysis and evaluation of protein interactionnetworks. Biosystems 2015;127:67-72. [Crossref] [PubMed]
- Yu G, Wang LG, Han Y, et al. clusterProfiler: an R package for comparing biological themes among gene clusters. OMICS 2012;16:284-7. [Crossref] [PubMed]
- Dweep H, Gretz N. miRWalk2.0: a comprehensive atlas of microRNA-target interactions. Nat Methods 2015;12:697. [Crossref] [PubMed]
- Enright AJ, John B, Gaul U, et al. MicroRNA targets in Drosophila. Genome Biol 2003;5:R1. [Crossref] [PubMed]
- Hossain MN, Sakemura R, Fujii M, et al. G-protein gamma subunit GNG11 strongly regulates cellular senescence. Biochem Biophys Res Commun 2006;351:645-50. [Crossref] [PubMed]
- Nolte IM, Munoz ML, Tragante V, et al. Genetic loci associated with heart rate variability and their effects on cardiac disease risk. Nat Commun 2017;8:15805. [Crossref] [PubMed]
- Girbl T, Lenn T, Perez L, et al. Distinct Compartmentalization of the Chemokines CXCL1 and CXCL2 and the Atypical Receptor ACKR1 Determine Discrete Stages of Neutrophil Diapedesis. Immunity 2018;49:1062-1076.e6. [Crossref] [PubMed]
- Wang L, Zhang YL, Lin QY, et al. CXCL1-CXCR2 axis mediates angiotensin II-induced cardiac hypertrophy and remodelling through regulation of monocyte infiltration. Eur Heart J 2018;39:1818-31. [Crossref] [PubMed]
- Tsai HH, Niu J, Munji R, et al. Oligodendrocyte precursors migrate along vasculature in the developing nervous system. Science 2016;351:379-84. [Crossref] [PubMed]
- Wang J, Hossain M, Thanabalasuriar A, et al. Visualizing the function and fate of neutrophils in sterile injury and repair. Science 2017;358:111-6. [Crossref] [PubMed]
- Döring Y, Noels H, van der Vorst EPC, et al. Vascular CXCR4 Limits Atherosclerosis by Maintaining Arterial Integrity: Evidence From Mouse and Human Studies. Circulation 2017;136:388-403. [Crossref] [PubMed]
- Boström P, Andersson L, Rutberg M, et al. SNARE proteins mediate fusion between cytosolic lipid droplets and are implicated in insulin sensitivity. Nat Cell Biol 2007;9:1286-93. [Crossref] [PubMed]
- Shin HJ, Kim H, Oh S, et al. AMPK-SKP2-CARM1 signalling cascade in transcriptional regulation of autophagy. Nature 2016;534:553-7. [Crossref] [PubMed]
- Reyes-Resina I, Navarro G, Aguinaga D, et al. Molecular and functional interaction between GPR18 and cannabinoid CB2 G-protein-coupled receptors. Relevance in neurodegenerative diseases. Biochem Pharmacol 2018;157:169-79. [Crossref] [PubMed]
- Matouk AI, Taye A, El-Moselhy MA, et al. The Effect of Chronic Activation of the Novel Endocannabinoid Receptor GPR18 on Myocardial Function and Blood Pressure in Conscious Rats. J Cardiovasc Pharmacol 2017;69:23-33. [Crossref] [PubMed]
- Sun KY, Peng T, Chen Z, et al. Long non-coding RNA LOC100129148 functions as an oncogene in human nasopharyngeal carcinoma by targeting miR-539-5p. Aging (Albany NY) 2017;9:999-1011. [Crossref] [PubMed]
- Wei JQ, Chen H, Zheng XF, et al. Hsa-miR-654-5p regulates osteogenic differentiation of human bone marrow mesenchymal stem cells by repressing bone morphogenetic protein 2. Nan Fang Yi Ke Da Xue Xue Bao 2012;32:291-5. [PubMed]
- Liu Y, Lu C, Zhou Y, et al. Circular RNA hsa_circ_0008039 promotes breast cancer cell proliferation and migration by regulating miR-432-5p/E2F3 axis. Biochem Biophys Res Commun 2018;502:358-63. [Crossref] [PubMed]
- Xu H, Han H, Song S, et al. Exosome-Transmitted PSMA3 and PSMA3-AS1 Promote Proteasome Inhibitor Resistance in Multiple Myeloma. Clin Cancer Res 2019;25:1923-35. [Crossref] [PubMed]
- Thiebaut C, Chesnel A, Merlin JL, et al. Dual Epigenetic Regulation of ERα36 Expression in Breast Cancer Cells. Int J Mol Sci 2019;20:2637. [Crossref] [PubMed]
- Watts AE, Millar NL, Platt J, et al. MicroRNA29a Treatment Improves Early Tendon Injury. Mol Ther 2017;25:2415-26. [Crossref] [PubMed]
- Bai X, Geng J, Li X, et al. Long Noncoding RNA LINC01619 Regulates MicroRNA-27a/Forkhead Box Protein O1 and Endoplasmic Reticulum Stress-Mediated Podocyte Injury in Diabetic Nephropathy. Antioxid Redox Signal 2018;29:355-76. [Crossref] [PubMed]
- Shivakumar M, Lee Y, Bang L, et al. Identification of epigenetic interactions between miRNA and DNA methylation associated with gene expression as potential prognostic markers in bladder cancer. BMC Med Genomics 2017;10:30. [Crossref] [PubMed]
- Hasan MM, Akter R, Ullah MS, et al. A Computational Approach for Predicting Role of Human MicroRNAs in MERS-CoV Genome. Adv Bioinformatics 2014;2014:967946. [Crossref] [PubMed]
- Zhang WC, Chin TM, Yang H, et al. Tumour-initiating cell-specific miR-1246 and miR-1290 expression converge to promote non-small cell lung cancer progression. Nat Commun 2016;7:11702. [Crossref] [PubMed]
- Schulz J, Takousis P, Wohlers I, et al. Meta-analyses identify differentially expressed micrornas in Parkinson's disease. Ann Neurol 2019;85:835-51. [Crossref] [PubMed]
- Meng Y, Quan L, Liu A. Identification of key microRNAs associated with diffuse large B-cell lymphoma by analyzing serum microRNA expressions. Gene 2018;642:205-11. [Crossref] [PubMed]
- Wang W, Zhao Z, Yang F, et al. An immune-related lncRNA signature for patients with anaplastic gliomas. J Neurooncol 2018;136:263-71. [Crossref] [PubMed]
- Goyal P, Behring A, Kumar A, et al. STK35L1 associates with nuclear actin and regulates cell cycle and migration of endothelial cells. PLoS One 2011;6:e16249. [Crossref] [PubMed]
- Miyamoto Y, Whiley PAF, Goh HY, et al. The STK35 locus contributes to normal gametogenesis and encodes a lncRNA responsive to oxidative stress. Biol Open 2018;7:bio032631. [Crossref] [PubMed]
- Yasuda Y, Miyamoto Y, Yamashiro T, et al. Nuclear retention of import in α coordinates cell fate through changes in gene expression. EMBO J 2012;31:83-94. [Crossref] [PubMed]