Application of next generation sequencing-based rapid detection platform for microbiological diagnosis and drug resistance prediction in acute lower respiratory infection
Introduction
Acute lower respiratory infections (ALRIs) are common diseases worldwide, and have a high mortality rate, especially in children, where they account for approximately 30% of child deaths (1-3). Generally, ALRIs are caused by microbial organisms such as bacteria, viruses, mycoplasma, chlamydia, and legionella. At present, there are two major concerns with regards to ALRI detection and treatment. Firstly, the traditional sputum culture (SC) diagnosis has a low sensitivity in probing many microorganisms (4,5). Secondly, the qualified sputum comes from lower respiratory tract, as it passes through mouth, the microbes located in oral cavity may contaminate the sputum. Also, severe acute bacterial infections are an enormous and growing healthcare burden, and they typically lack timely diagnosis and evade adequate therapy in around 40% of clinical cases. Besides the common microorganisms, there are numerous pathogens that are difficult to detect, such as Mycoplasma pneumoniae, Chlamydia pneumoniae, Aspergillus niger, Cryptococcus neoformans, Pneumocystis carinii, Adenovirus, Human Bocavirus, Cytomegalovirus, and the Epstein-Barr (EB) virus. Many of these organisms are fastidious pathogens, but conventional culture methodologies (like SC) exhibit a low detection rate. Given the difficulties of pathogen identification in infectious diseases, there is a pressing need for alternative diagnostic methods that are rapid and easy with batch processing features to detect multiple microorganisms.
The problem of antibiotic abuse has become prominent, especially in China, and emerging pathogenic bacteria have developed resistance to single and even multiple antibiotics (6-9). Moreover, super bacteria, including methicillin-resistant Staphylococcus aureus (MRSA) (10,11), multidrug-resistant Streptococcus pneumoniae (MDRSP) (12), and multidrug resistance Acinetobacter baumannii (MRAB) (13) have recently emerged and rapidly developed a high prevalence. According to drug resistance monitoring data in China, up to 33% of isolated strains in 3-A grade hospitals are multi-drug resistant (14).
As has been widely reported, inappropriate antibacterial treatment can increase the mortality of respiratory infections (15,16). An early prediction of antibiotic resistance prior to treatment can largely improve the antibiotic treatment outcomes. To address the diagnosis and resistance prediction challenge in ALRI patients, we applied a platform that rapidly (within 16 hours) detects 36 microorganisms as well as 49 antibiotic resistance markers. Our findings exhibited significant advantages of the sequencing method compared with conventional SC methods. Next generation sequencing (NGS) could minimize the effect of contamination in sputum test. As the microbes colonized in oral cavity are mainly irresponsible to lower respiratory tract, they are not included in our pathogen-targeted panel, so the non-pathogen microbes would not disturb the result. In addition, doctors could judge which pathogens detected in our result could be mainly responsible for the symptom of the patient according to other clinical evidence.
We present the following article in accordance with the MDAR reporting checklist (available at http://dx.doi.org/10.21037/atm-20-7081).
Methods
Study population
This prospective study was approved by the Ethics Review Committee of the Second Hospital of Hebei Medical University (No. 2016150), and all the patients were given the informed consent before participated in. The procedures performed involving human were in accordance with the Declaration of Helsinki (as revised in 2013). Multicenter collection of clinical samples from patients with ALRIs was carried out from 2017 to 2018. The inclusion criteria were as follows: patients with obvious symptoms of lower respiratory tract infection, including: (I) pneumonia patients; any of following symptoms or signs: fever (>38 °C), suspension of breathing, shortness of breath, bradycardia, wheezing, coughing, dry snoring, and chest images showing new or progressive exudation, solid shadows, cavity or pleural effusion, etc.; (II) tracheitis or tracheobronchitis patients; two of the following symptoms or signs were observed: cough with increased sputum, dry voice, wheezing, respiratory distress, apnea, or bradycardia, but the patient showed no clinical symptoms or X-ray evidence of pneumonia; and (III) other infections of the lower respiratory tract based on lung radiographic examination, such as lung abscess or empyema.
The clinical data of each patient was recorded, and the blood and sputum samples were collected upon admission to hospital. The SCs were performed in the laboratory, which provided two outcomes: the detected pathogens and the resistance to different antibiotics (including Piperacillin, Ampicillin, Ampicillin/Sulbactam, Piperacillin/tazobactam, Cefoperazone/Sulbactam, Cefoperazone, Cefotaxime, Cefepime, Cefazolin, Cefuroxime axe, Ceffuxin sodium, Cefotitan, Ceftazidime, Ceftriaxone, Aztreonam, Meropenem, Imipenem, Biapenem, Amikacin, Gentamicin, Tobramycin, Ciprofloxacin, Levofloxacin, Nitrofurantoin, etc.). Additionally, the sputum sample was used to extract deoxyribonucleic acids (DNAs) followed by high-throughput sequencing.
Pathogen targets
All of the relevant pathogens explored in sequencing and SC are listed in Table 1. The results of the SC were used for comparison, and the suspected results based on sequencing data were used to analyze efficacy, including sensitivity and specificity.
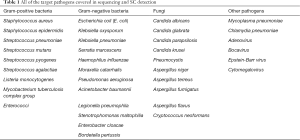
Full table
Sequencing for rapid detection of microorganisms and antibiotic resistance genes
The Curetis Unyvero™ System was used for rapid detection of microorganisms and antibiotic resistance genes. The sputum was liquefied by adding dissolving solutions if necessary. After the addition of 200 µL of a qualified sputum sample, the sample tube was loaded into the Lysator and treated according to a preselected protocol fully automatically. The Unyvero™ Sample Tube Cap seals the Unyvero™ Sample Tube, and contains Proteinase K, Lysozyme, Staphylococcus lysozyme, snail enzyme, and an internal control gene for the quality control of the entire workflow for each sample.
In combination with the lysis buffer and Proteinase K, liquefaction of each sample was performed within 30 min and all potentially infectious pathogens were inactivated. Following lysis, the Biotek extractor was applied to extract nucleic acids automatically. The nucleic acids were quantified by the Unyvero system and then amplified by polymerase chain reaction (PCR). The PCR assay consisted of multiplexed primer sets in combination with primers for an internal process control. The product was purified using the magnetic bead method to complete the establishment of targeted capture and sequencing libraries. The qualified DNA libraries were sequenced on the Illumina high-throughput sequencing platform. Sequencing data were automatically normalized based on the amplification calibration coefficient. A total of 10,000 sequencing reads were analyzed for each sample. Overall, if the pathogenic reads of a microorganism were below 100, it was regarded as negative (at the sequencing level), or otherwise reported as positive.
Statistical analysis
Data were analyzed using SPSS 22.0 software. For comparison of frequencies of the categorical data, Pearson chi-squared (χ2) test or Fisher’s exact test were used. Quantitative data were compared between the two groups using a t test (in normal distribution) or a non-parametric test (Mann-Whitney Test, not in normal distribution). The correlation between the reads of two pathogen markers was analyzed and expressed as the Spearman r values. A receiver operating characteristic (ROC) curve was drawn to acquire the optimal threshold in predicting the positive outcome of a specific pathogen. For all presented data, P<0.05 was considered statistically significant.
Results
Patient characteristics and clinical samples
As shown in Figure 1A, the SC revealed 147 patients with clear pathogens and 148 with no pathogens (from a total cohort of 295 subjects). An overwhelming majority of patients in the pathogen group were found with a single pathogen [105], 27 patients had two compound pathogens, and 15 patients had three Gram-negative bacteria. In the single pathogen group, Klebsiella pneumoniae exhibited the highest frequency (32 cases), followed by Pseudomonas aeruginosa (20 cases), Stenotrophomonas maltophilia (11 cases), and Candida albicans (nine cases). The most frequently observed outcomes are listed in Figure 1B.
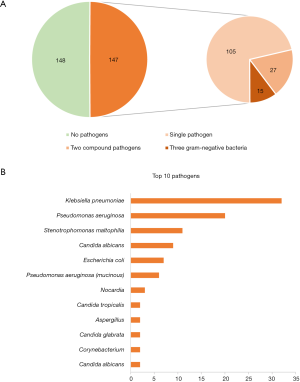
Performance of sequencing results focusing on SC outcomes
We also assessed the coverage of the sequencing results focusing on all SC positive samples. Eleven commonly observed pathogens (Acinetobacter baumannii, E. coli, Klebsiella pneumoniae, Staphylococcus aureus, Pseudomonas aeruginosa, Stenotrophomonas maltophilia, Haemophilus influenzae, Candida albicans, Candida glabrata, Serratia marcescens, and Aspergillus) were surveyed, and for all SC positive samples (137 cases) the overall coverage was 95.62% (131 cases) according to the sequencing results. The SC positive cases and sequencing findings, as well as the percent coverage (sequencing positive case number/SC case number) are presented in Figure 2A. For most SC positive pathogens, the sequencing result showed a 100% coverage, which suggests that this is a satisfactory detection method.
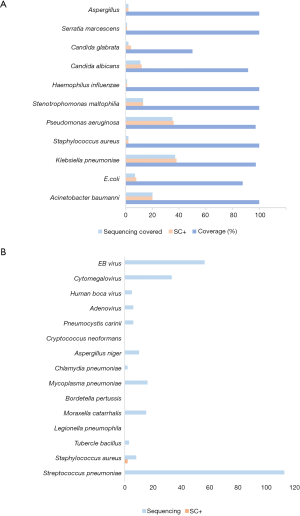
Subsequently, we observed some special pathogens that are difficult to detect using the normal SC method but have a high clinical severity. The sequencing method exhibited notable superiority, especially for Streptococcus pneumoniae, EB virus, and Cytomegalovirus (Figure 2B). This result strongly suggests that sequencing diagnosis is efficacious for pathogen recognition, especially for those difficult to observe using the traditional SC method.
To explore the accuracy threshold of commonly-observed pathogens, we applied ROC curves and calculated the cutoff reads for diagnosis of the most frequently detected pathogens (Figure 3). Here, SC outcomes were regarded as the actual results, and sequencing results (reads) were used as diagnostic references. For Klebsiella pneumoniae, the sequencing data provided an area under the curve (AUC) of 0.637 and an optimal cutoff value of 57 reads (Figure 3A). The sequencing method showed an AUC of 0.622, with an optimal cutoff value of 168 reads for the detection of Pseudomonas aeruginosa (Figure 3B). For the mucinous type of Pseudomonas aeruginosa, sequencing exhibited an extremely high AUC (0.986), with an optimal cutoff value of 9,677 (sensitivity =1, specificity =0.976), which suggests that at high reads, sequencing results are very useful to distinguish the mucinous Pseudomonas aeruginosa (Figure 3D). Moreover, the performance of sequencing in E. coli detection showed a high sensitivity and specificity, with an AUC of 0.96 and a recommended cutoff value of 17 reads (Figure 3C). However, the efficacy of the sequencing method was relatively low for the diagnosis of Candida albicans and Stenotrophomonas maltophilia (AUC =0.41 and 0.516, respectively). Overall, sequencing had much higher sensitivity in the detection of pathogens compared with the traditional SC method, with a generally satisfactory specificity.
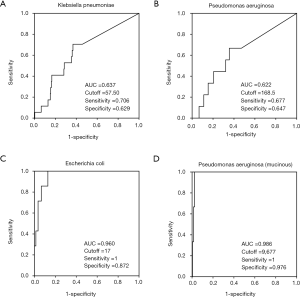
Correlation between different pathogen markers
For the first time, we noticed that there were clear associations between different pathogen markers. Several common pathogens were analyzed using the Spearman method, and some significant relationships of their sequencing reads were observed, including Acinetobacter baumannii and Candida glabrata (Figure 4A), Acinetobacter baumannii and Klebsiella pneumoniae (Figure 4B), Mycoplasma pneumoniae and Candida albicans (Figure 4C), E. coli and Candida albicans (Figure 4D), Pseudomonas aeruginosa and Klebsiella pneumoniae (Figure 4E), Streptococcus pneumoniae and Klebsiella pneumoniae (Figure 4F), Klebsiella pneumoniae and Candida glabrata (Figure 4G), Klebsiella pneumoniae and Enterobacter cloacae (Figure 4H), Klebsiella pneumoniae and E. coli (Figure 4I), Klebsiella pneumoniae and Streptococcus agalactiae (Figure 4J), E. coli and Streptococcus agalactiae (Figure 4K), E. coli and Streptococcus mutans (Figure 4L), EB virus and Enterococcus (Figure 4M), EB virus and E. coli (Figure 4N), Adenovirus and Haemophilus influenzae (Figure 4O), and EB virus and pneumocystis (Figure 4P), among others. There were also positive correlations between fungi results (for the limited positive number of fungi, such as Aspergillus or Aspergillus niger, data not shown), as well as associations between fungi and other pathogens. These correlations were seldom noticed in the traditional SC method.
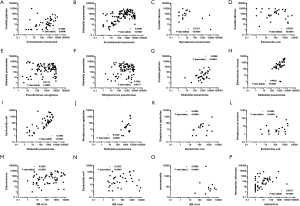
Performance of sequencing in antimicrobial-resistance prediction
Next, the sensitivity to different types of antibiotics in the SC were recorded. The current SC method is generally regarded as a benchmark for the diagnosis of drug-resistant infections. However, studies that have surveyed the resistance-associated mutations in sputum samples are limited.
We also investigated the correlation between antibiotic resistance gene phenotypes and the actual outcomes of the drug sensitivity test. Detected samples were treated with multiple antibiotics, and were later divided into three groups: susceptible (S), intermediate (I), and resistant (R). The observed significant correlations between antimicrobial-resistance and drug-resistance mutations were as follows: OXA-58 family mutations and Piperacillin-Tazobactam (Figure 5A), CcrA and Cefepime (Figure 5B), VIM and Cefepime (Figure 5C), CcrA and Ceftazidime (Figure 5D), blaOXA7 and Amikacin (Figure 5E), OXA50 family and Ciprofloxacin (Figure 5F), OXA51 family and Levofloxacin (Figure 5G), and NMD mutation was associated with Nitrofurantoin resistance (Figure 5H). This trend was most typical in the resistance to Amikacin in the presence of blaOXA7, which has not yet been reported. In particular, a sample (sample ID s8-35) was found to be Methicillin-resistant Staphylococcus aureus (MRSA), and showed a broad-spectrum drug resistance (including towards Ciprofloxacin, Levofloxacin, Oxacillin, Erythromycin, Benzylpenicillin, Tetracycline, Moxifloxacin, Clindamycin, etc.). As expected, mecA mutant was detected in this case. However, for most of the positive results, the sample sizes of the intermediate groups were small, and more evidence is urgently required to validate and confirm the current conclusion. Collectively, sequencing results can predict antibiotic resistance in advance, especially for the use of Amikacin.

Discussion
In the present study, we compared the detection results of the sequencing and SC techniques, and observed significant advantages of the sequencing method. First, sequencing has an overall satisfactory accuracy but can yield a markedly higher sensitivity for pathogen identification, especially in detecting Streptococcus pneumoniae and viruses. Also, for the first time, we identified some clear associations between different pathogen markers, which were seldom noticed in the traditional SC method. Finally, the sequencing approach exhibited a good performance in antibiotic resistance prediction.
PCR combined with sequencing had been used more than a decade ago for pathogen detection and drug resistance, particularly when targeting Mycobacterium (towards rifampin resistance) (17-20). In recent years, sequencing methods have been applied to detect viruses from sputum samples that might be associated with ALRI onset, without virus culture (21). More recently, there have been limited similar studies that used sequencing to identify pathogens. In 2018, scholars sequenced M. tuberculosis from 43 sputum samples by targeted DNA enrichment, and reported that direct sputum sequencing has the potential to provide more rapid comprehensive resistance detection than SC in clinical practice (22). In 2019, British researchers found that DNA directly sequenced from sputum samples had more within-sample diversity than that from culture, and resistance-associated variants could be probed in a timely manner. More importantly, genome sequencing may allow for the detection of pathogens that are not actively replicating (23). To date, sequencing-based platforms have been regarded as promising and user-friendly for pathogen detection and drug-resistant diagnosis (24), and have been applied in the detection and resistance assessment of tuberculosis, influenza A virus, and cystic fibrosis-related pathogens, among others (25-27).
Consistent with previous research, our results indicated that sequencing of sputum samples offers advantages in pathogen discrimination and also provides an early warning of antimicrobial resistance. Some novel links between gene polymorphism and antibiotic resistance were observed in this study. Taking the most typical association between Amikacin and blaOXA7 as an example, we found, for the first time, that this phenotype may confer an Amikacin resistance, which may help to develop a more appropriate treatment strategy.
An interesting finding of our study is that there were significant and novel associations between different pathogens based on the sequencing results, yet these were not noticed in the traditional SC method. However, some findings are theoretically reasonable and consistent with known reports. For example, the markers of Enterobacter cloacae and Klebsiella pneumoniae were highly positively correlated (r=0.4652, P<0.0001), and it has been reported that they share some common mechanisms in spreading drug resistance, such as producing NDM (28-30), OXA48 (28) and VIM1 (31-33). Further studies can be conducted to explore the deeper mechanisms of the comorbidity, and our observations provide a new perspective in warning of specific pathogenic infection when another pathogen is diagnosed.
Though sequencing of sputum samples has such advantages, there are some possible reasons that could cause the deficiencies of identification. First, we used just one pair of primers to detect each pathogen. It might be not enough to cover all the clinical strains of the pathogen. A larger panel which contains three primer pairs for each pathogen we targeted is being developed. It might work better in further studies.
As to the problem of high cost, the multiplex-primers-panel-driven targeted pathogen screening could be the answer. It would use only several thousands of sequencing reads to finish the job while mNGS needs millions. The great reduction of cost could make the approach much more acceptable. The multiplex-primers-panel approach is much more sensitive on genotyping comparing with mNGS, such as drug resistance detection. Also the multiplex-primers-panel has such advantages, it still needs to be performed in well-managed PCR laboratories due to the susceptibility of contamination. Except for a few limited drug resistance genes, the biggest problem in molecular drug resistance gene diagnosis is the lack of sufficient clinical research data between many drug resistance genes and bacterial resistance phenotypes, thus making it difficult for these drug resistance genes to serve as resistance markers in clinical application. For resistance genes in the form of plasmid, molecular biological detection method cannot determine if the resistance genes came from disease-causing pathogens or nonpathogenic bacteria.
On all accounts, NGS exceeds traditional bacterial culture in many aspects. Though there still are much work to be done in the future, it’s already proven to be a promising detection method to guide the treatment of acute lower respiratory infection.
Conclusions
In conclusion, sequencing-based sputum metagenomics can reveal a profile of the lung pathogen microbiome. The sequencing method is offers both sufficient accuracy and significantly higher sensitivity in pathogen detection, and can be at least a complementary approach to traditional SC reporting. The sequencing technique also revealed some novel potential correlations between the presence of different pathogens, as well as new antimicrobial-resistant genes.
Acknowledgments
Funding: This work was supported by Science and Technology Planning Project of Hebei Province of China (No. 17277790D).
Footnote
Reporting Checklist: The authors have completed the MDAR checklist. Available at http://dx.doi.org/10.21037/atm-20-7081
Data Sharing Statement: Available at http://dx.doi.org/10.21037/atm-20-7081
Conflicts of Interest: All authors have completed the ICMJE uniform disclosure form (available at http://dx.doi.org/10.21037/atm-20-7081). The authors have no conflicts of interest to declare.
Ethical Statement: The authors are accountable for all aspects of the work in ensuring that questions related to the accuracy or integrity of any part of the work are appropriately investigated and resolved. This prospective study was approved by the Ethics Review Committee of the Second Hospital of Hebei Medical University (No. 2016150), and all the patients were given the informed consent before participated in. The procedures performed involving human were in accordance with the Declaration of Helsinki (as revised in 2013).
Open Access Statement: This is an Open Access article distributed in accordance with the Creative Commons Attribution-NonCommercial-NoDerivs 4.0 International License (CC BY-NC-ND 4.0), which permits the non-commercial replication and distribution of the article with the strict proviso that no changes or edits are made and the original work is properly cited (including links to both the formal publication through the relevant DOI and the license). See: https://creativecommons.org/licenses/by-nc-nd/4.0/.
References
- Kini S, Kalal BS, Chandy S, et al. Prevalence of respiratory syncytial virus infection among children hospitalized with acute lower respiratory tract infections in Southern India. World J Clin Pediatr 2019;8:33-42. [Crossref] [PubMed]
- Smith-Vaughan HC, Binks MJ, Beissbarth J, et al. Bacteria and viruses in the nasopharynx immediately prior to onset of acute lower respiratory infections in Indigenous Australian children. Eur J Clin Microbiol Infect Dis 2018;37:1785-94. [Crossref] [PubMed]
- Nhung NTT, Schindler C, Dien TM, et al. Acute effects of ambient air pollution on lower respiratory infections in Hanoi children: An eight-year time series study. Environ Int 2018;110:139-48. [Crossref] [PubMed]
- Deschaght P, De Baere T, Van Simaey L, et al. Comparison of the sensitivity of culture, PCR and quantitative real-time PCR for the detection of Pseudomonas aeruginosa in sputum of cystic fibrosis patients. BMC Microbiol 2009;9:244. [Crossref] [PubMed]
- Schmalz M, Joysula M, Staddon JH, et al. Caspofungin resistant disseminated candidiasis in a 7-year-old girl with T cell lymphoma: a case report. Transl Pediatr 2018;7:63-6. [Crossref] [PubMed]
- Rao C, Hu Z, Chen J, et al. Molecular epidemiology and antibiotic resistance of Burkholderia pseudomallei isolates from Hainan, China: A STROBE compliant observational study. Medicine (Baltimore) 2019;98:e14461. [Crossref] [PubMed]
- Li S, Zhang R, Hu J, et al. Occurrence and removal of antibiotics and antibiotic resistance genes in natural and constructed riverine wetlands in Beijing, China. Sci Total Environ 2019;664:546-53. [Crossref] [PubMed]
- Wang L, Su H, Hu X, et al. Abundance and removal of antibiotic resistance genes (ARGs) in the rearing environments of intensive shrimp aquaculture in South China. J Environ Sci Health B 2019;54:211-8. [Crossref] [PubMed]
- Guan Y, Jia J, Wu L, et al. Analysis of Bacterial Community Characteristics, Abundance of Antibiotics and Antibiotic Resistance Genes Along a Pollution Gradient of Ba River in Xi'an, China. Front Microbiol 2018;9:3191. [Crossref] [PubMed]
- Setchanova L, Alexandrova A, Pencheva D, et al. Rise of multidrug-resistant Streptococcus pneumoniae clones expressing non-vaccine serotypes among children following introduction of the 10-valent pneumococcal conjugate vaccine in Bulgaria. J Glob Antimicrob Resist 2018;15:6-11. [Crossref] [PubMed]
- Raddaoui A, Tanfous FB, Chebbi Y, et al. High prevalence of multidrug-resistant international clones among macrolide-resistant Streptococcus pneumoniae isolates in immunocompromised patients in Tunisia. Int J Antimicrob Agents 2018;52:893-7. [Crossref] [PubMed]
- Nakano S, Fujisawa T, Ito Y, et al. Whole-Genome Sequencing Analysis of Multidrug-Resistant Serotype 15A Streptococcus pneumoniae in Japan and the Emergence of a Highly Resistant Serotype 15A-ST9084 Clone. Antimicrob Agents Chemother 2019;63:e02579-18. [Crossref] [PubMed]
- Shirmohammadlou N, Zeighami H, Haghi F, et al. Resistance pattern and distribution of carbapenemase and antiseptic resistance genes among multidrug-resistant Acinetobacter baumannii isolated from intensive care unit patients. J Med Microbiol 2018;67:1467-73. [Crossref] [PubMed]
- Jia XQ, Pang F, Chen JZ, et al. Prevalence and clinical distribution of multidrug-resistant bacteria (3537 isolates) in a tertiary Chinese hospital (January 2012-December 2013). Pathol Biol (Paris) 2015;63:21-3. [Crossref] [PubMed]
- Iregui M, Ward S, Sherman G, et al. Clinical importance of delays in the initiation of appropriate antibiotic treatment for ventilator-associated pneumonia. Chest 2002;122:262-8. [Crossref] [PubMed]
- Ibrahim EH, Sherman G, Ward S, et al. The influence of inadequate antimicrobial treatment of bloodstream infections on patient outcomes in the ICU setting. Chest 2000;118:146-55. [Crossref] [PubMed]
- Patnaik M, Liegmann K, Peter JB. Rapid detection of smear-negative Mycobacterium tuberculosis by PCR and sequencing for rifampin resistance with DNA extracted directly from slides. J Clin Microbiol 2001;39:51-2. [Crossref] [PubMed]
- Kim BJ, Lee KH, Park BN, et al. Detection of rifampin-resistant Mycobacterium tuberculosis in sputa by nested PCR-linked single-strand conformation polymorphism and DNA sequencing. J Clin Microbiol 2001;39:2610-7. [Crossref] [PubMed]
- Suresh N, Singh UB, Gupta C, et al. Rapid detection of rifampin-resistant Mycobacterium tuberculosis directly from stained sputum smears using single-tube nested polymerase chain reaction deoxyribonucleic acid sequencing. Diagn Microbiol Infect Dis 2007;58:217-22. [Crossref] [PubMed]
- Yam WC, Tam CM, Leung CC, et al. Direct detection of rifampin-resistant mycobacterium tuberculosis in respiratory specimens by PCR-DNA sequencing. J Clin Microbiol 2004;42:4438-43. [Crossref] [PubMed]
- Ren X, Yang F, Hu Y, et al. Full genome of influenza A (H7N9) virus derived by direct sequencing without culture. Emerg Infect Dis 2013;19:1881-4. [Crossref] [PubMed]
- Doyle RM, Burgess C, Williams R, et al. Direct Whole-Genome Sequencing of Sputum Accurately Identifies Drug-Resistant Mycobacterium tuberculosis Faster than MGIT Culture Sequencing. J Clin Microbiol 2018;56:e00666-18. [Crossref] [PubMed]
- Nimmo C, Shaw LP, Doyle R, et al. Whole genome sequencing Mycobacterium tuberculosis directly from sputum identifies more genetic diversity than sequencing from culture. BMC Genomics 2019;20:389. [Crossref] [PubMed]
- Dolinger DL, Colman RE, Engelthaler DM, et al. Next-generation sequencing-based user-friendly platforms for drug-resistant tuberculosis diagnosis: A promise for the near future. Int J Mycobacteriol 2016;5 Suppl 1:S27-8. [Crossref] [PubMed]
- Feigelman R, Kahlert CR, Baty F, et al. Sputum DNA sequencing in cystic fibrosis: non-invasive access to the lung microbiome and to pathogen details. Microbiome 2017;5:20. [Crossref] [PubMed]
- Hu Y, Zhang Y, Ren X, et al. A case report demonstrating the utility of next generation sequencing in analyzing serial samples from the lung following an infection with influenza A (H7N9) virus. J Clin Virol 2016;76:45-50. [Crossref] [PubMed]
- Nimmo C, Doyle R, Burgess C, et al. Rapid identification of a Mycobacterium tuberculosis full genetic drug resistance profile through whole genome sequencing directly from sputum. Int J Infect Dis 2017;62:44-6. [Crossref] [PubMed]
- Haciseyitoglu D, Dokutan A, Abulaila A, et al. The First Enterobacter cloacae Co-Producing NDM and OXA-48 Carbapenemases and Interhospital Spread of OXA-48 and NDM-Producing Klebsiella pneumoniae in Turkey. Clin Lab 2017;63:1213-22. [Crossref] [PubMed]
- Khan AU, Nordmann P. NDM-1-producing Enterobacter cloacae and Klebsiella pneumoniae from diabetic foot ulcers in India. J Med Microbiol 2012;61:454-6. [Crossref] [PubMed]
- Alcántar-Curiel MD, Fernandez-Vazquez JL, Toledano-Tableros JE, et al. Emergence of IncFIA Plasmid-Carrying blaNDM-1 Among Klebsiella pneumoniae and Enterobacter cloacae Isolates in a Tertiary Referral Hospital in Mexico. Microb Drug Resist 2019;25:830-8. [Crossref] [PubMed]
- Sianou E, Kristo I, Petridis M, et al. A cautionary case of microbial solidarity: concurrent isolation of VIM-1-producing Klebsiella pneumoniae, Escherichia coli and Enterobacter cloacae from an infected wound. J Antimicrob Chemother 2012;67:244-6. [Crossref] [PubMed]
- Coelho A, Piedra-Carrasco N, Bartolome R, et al. Role of IncHI2 plasmids harbouring blaVIM-1, blaCTX-M-9, aac(6')-Ib and qnrA genes in the spread of multiresistant Enterobacter cloacae and Klebsiella pneumoniae strains in different units at Hospital Vall d'Hebron, Barcelona, Spain. Int J Antimicrob Agents 2012;39:514-7. [Crossref] [PubMed]
- Miró E, Segura C, Navarro F, et al. Spread of plasmids containing the bla(VIM-1) and bla(CTX-M) genes and the qnr determinant in Enterobacter cloacae, Klebsiella pneumoniae and Klebsiella oxytoca isolates. J Antimicrob Chemother 2010;65:661-5. [Crossref] [PubMed]
(English Language Editor: A. Kassem)