Characteristics and clinical subtypes of cancer patients in the intensive care unit: a retrospective observational study for two large databases
Introduction
Cancer is a common comorbidity in patients admitted to the intensive care unit (ICU). Approximately one in seven ICU patients have cancer comorbidity (1,2). Previous studies have explored the characteristics and outcomes of critically ill cancer patients and reported highly varied mortality for this population (3-5). These mortality-related variations indicate the difficulty of prognostication in critically ill cancer patients (6). Some exceptional research has specified criteria to select these patients such as postoperative admission after elective surgery (4,7). These studies always had similar outcomes, whereas others had quite different outcomes. The disparity in the mortality rates of ICU patients with cancer may imply some clinical subtypes that are so different in clinical features and prognoses that they cannot be regarded as a whole population, both in clinical practice and research. Therefore, to identify these subtypes may help explain the abovementioned disparity in mortality rates and facilitate prognostication in this specific population.
Furthermore, previous studies only revealed varied mortality among different types of cancer at organ level (8,9). Cancers at different locations within the same organ have distinct prognoses, such as for hilar versus peripheral lung cancer (10). Therefore, more detailed evidence, particularly on mortality at International Classification of Diseases (ICD) level, is needed.
We conducted this study to explore the characteristics and outcomes, particularly the ICD-level mortality rate, of patients with cancer in the ICU. Moreover, we introduced an unsupervised machine learning algorithm to identify different patient subpopulations through the analysis of cancer patients from two public ICU databases derived from large medical centers. We also evaluated the calibration of SAPS II and APACHE IV in this population. We present the following article in accordance with the STROBE reporting checklist (available at http://dx.doi.org/10.21037/atm-20-4634).
Methods
Data source
We extracted data from two large public databases: the Medical Information Mart for Intensive Care (MIMIC-III) Database (https://mimic.physionet.org) and eICU Collaborative Research Database (https://eicu-crd.mit.edu) (11,12). The MIMIC database contains medical records of more than 50,000 distinct admissions in the critical care units of the Beth Israel Deaconess Medical Center that occurred between 2001 and 2012, whereas the eICU database collected medical records from over 200,000 admissions from multiple centers in 2014 and 2015.
Extraction criteria
The inclusion criteria were: (I) having diagnosis code of neoplasm (ICD-9 codes: 140–209) at any discharge diagnosis position; (II) the length of ICU stay was more than 4 hours; and (III) age between 16 and 89. We excluded ICU readmissions at the same hospital; in case of inter-ICU shift, only the first ICU stay was considered.
Data extraction and definition of variables
For both databases, the ICD-9 codes, along with their priority in each patient’s diagnoses (a sequence in the MIMIC database, but classified as “primary,” “main,” “other” in the eICU database), were extracted to identify specific cancer types.
In the MIMIC database, we extracted data on demographics (age, sex, type of admission, and type of care unit), clinical service provider (surgical or medical), main comorbidities, vital signs, laboratory parameters reflecting illness severity, and organ-supporting treatment (mechanical ventilation, vasopressor, and renal replacement treatment) for analysis.
The clinical service referred to the service that a patient was admitted under. The main comorbidities were identified as previously described (13), and classified as congestive heart failure (CHF), atrial fibrillation (AFIB), liver disease, chronic obstructive pulmonary disease (COPD), chronic renal disease (CRD), coronary artery disease (CAD), stroke according to ICD-9 codes. The three abovementioned organ-supporting treatments were defined as binomial variables: whether they were used during the first 24 hours. Severity scoring systems, including the Simplified Acute Physiology Score II (SAPS II), Sequential Organ Failure Assessment (SOFA), and the Elixhauser Comorbidity Score, were calculated based on the respective published papers (14-16), with the code posted in the Github repository of the MIMIC database (17).
For the eICU database, the SAPS II score was calculated similarly as for the MIMIC database. However, the APACHE IV system was integrated in itself. Therefore, we extracted the APACHE IV score, predictive hospital mortality based on APACHE IV, the calculated SAPS II scores, and actual hospital mortality from the eICU database.
Outcomes
The main outcome in the study was hospital mortality; length of ICU stay was demonstrated during the comparison of clinical subtypes.
Statistical analysis
The morbidity and mortality of cancer patients in the ICU were calculated within ICD-9 level and visualized by a histogram. For each type of cancer, the diagnostic priority was demonstrated by a heatmap. As all patient diagnoses were sequenced in the MIMIC database, we normalized the diagnostic space into 10 segments (0%~, 10%~, ..) and assigned those diagnoses into segments by their priority—that is, the primary diagnosis was at the 0%-segment and the last diagnosis was at the 90%-segment. Diagnostic priority was normalized by the Eq. [1]:
[1]
Where pN refers to the normalized priority of the diagnosis, pmax refers to the total number of the patient’s diagnoses, and p refers to the priority of the diagnosis before they were normalized. For each type of cancer, the sum of diagnoses in each segment was calculated. When visualized on the heatmap, the sum of diagnoses of each segment was normalized by the maximal value of all segments, as shown in Eq. [2]:
[2]
Where SN refers to the normalized sum of diagnoses in each segment, S refers to the actual sum of diagnoses in each segment, and Smax refers to the sum of diagnoses in the segment with the largest number of diagnoses.
For the eICU database, we repeated the process above, but retained the three original diagnostic priorities (“primary,” “main,” and “other”) instead of ten segments.
We used an unsupervised machine-learning method, K-means clustering, to cluster the patient population into subtypes (18). Briefly, K-means clustering aims to partition n observations into k clusters according to the nearest Euclidean distance to the centroid of the belonging cluster (18). The centroid of each cluster, which is randomized at first, iteratively updates by calculating the average point of each cluster. Finally, observations with similar patterns are partitioned into the same cluster. Most of the abovementioned variables were used in the K-means model except for the severity-scoring systems and outcomes. Unlike variables calculated in the severity-scoring systems, we used the mean values of vital signs and laboratory parameters in the first 24 hours of ICU admission rather than the worst values. Missing values of variables fed into the K-means model were imputed with a logistic regression algorithm that predicted the missing value by other variables fed into the K-means model.
An “elbow plot” was drawn to help determine the best number of clusters. If failed, we would select it from different total numbers [2–4] of clusters (19). The results of the K-means clustering were visualized by a heatmap that demonstrated the relative values of all variables. Then, with an algorithm named principal component analysis (PCA) (20), all variables of each patient were compressed into two components (PCA1 and PCA2), whereby patients were visualized on a scatterplot with two dimensions that indicated the relationship between patients and clusters.
As the MIMIC database provided more detailed clinical data, we used the K-means method only for MIMIC database, and considered the eICU as the validation database. After clustering, variables were compared among different clusters, which implied different clinical subtypes. Variables that were closely related to the clusters were used as instrumentation variables to identify different clinical subtypes. We evaluated the interactions of clinical subtype, the severity scoring system, and outcomes by using the standard mortality ratio (SMR), calibration graph, and Hosmer-Lemeshow C statistic (21). The SMR was calculated by a ratio of the observed mortality divided by predicted mortality; 95% confidence intervals were calculated by Vandenbroucke method (22).
In this study, continuous and categorical data were summarized as mean (SD) and number (percentage) respectively. One-way Analysis of variance (ANOVA) and chi-square test were used for statistical inferences of continuous and categorical data. A P value less than 0.05 was considered indicative of statistically significant differences. Databases were created locally with Postgresql (version 10.0) and accessed by structured query language integrated into a Python 3.7 notebook, on which run all the data-analysis codes used in the study. The notebook was uploaded on the Github for maintaining reproducibility (https://github.com/gscfwid/Cancer_ICU).
Results
According to extractive criteria, we extracted data on 6,505 (13.8%) patients with cancer from 46,998 patients with non-specific diagnosis from the MIMIC database. Among these cancer patients, cancer-related diagnostic codes were counted 11,174 times. While in the eICU database, up to 7,351 (4.9%) patients with cancer were extracted from 148,532 patients with nonspecific diagnoses, and the cancer-related diagnosis was counted 7,893 times. The most common cancer types based on the ICD-9 system were metastatic cancer of bone/bone marrow in MIMIC and lung cancer in the eICU database. Moreover, the diagnosis with the highest mortality (for cancer type with more than 10 patients) was mycosis fungoides in the MIMIC database, and metastatic adenocarcinoma of unknown primary in the eICU database. The top 10 most common and most fatal cancer types in the ICD level were summarized in the Tables S1,S2.
Mortality and diagnostic priority of different cancer types that had more than 10 victims are shown in Figure 1. The top part of Figure 1 depicts the mortality rates of all types of cancer. The top 5 cancer types with the highest mortality that had at least 50 victims were annotated. The middle of the figure lists manually classified cancer types according to the organ or system, and this manual classification is listed respectively in Tables S3,S4 in the MIMIC and eICU databases, respectively. The priorities for each diagnosis were illustrated in the bottom part of Figure 1. We analyzed the eICU database in a similar way (Figure S1). Generally, for both databases, ICU patients with metastatic cancer, hematologic malignancy had higher mortality rates than others; they were less likely to be the primary diagnosis, except in metastatic brain tumor. Moreover, in the MIMIC database, most non-metastatic solid cancers held the first position at diagnosis.
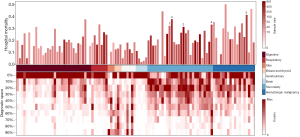
As the elbow plot hardly provided the best cluster number (Figure S2), we conducted a spectrum of K-means clustering with different cluster numbers [2–4] for the MIMIC database, as illustrated in Figure 2A and Figures S3,S4. The boundary of all 4 clusters were relatively clear (Figure 2B). Variables that were mostly associated with different clusters were admission type (elective or emergency) and clinical service provider (surgical or medical). We noticed that nearly all patients in the first cluster from the clustering of K=4 (Figure 2) were elective admissions (99.1%), whereas in the rest of the clusters, most were emergency admissions (93.7%). Most surgical patients were in the 1+2 clusters (92.0%) and most medical patients were in the 3+4 clusters (93.5%). Detailed disparities of the characteristics and outcomes from all four clusters are summarized in Table 1.
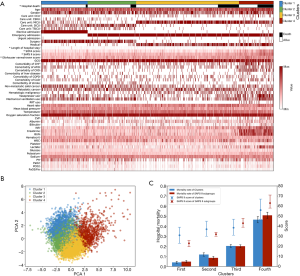
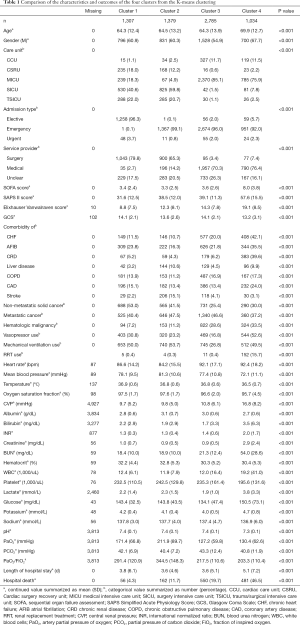
Full table
When comparing the clusters with different K numbers (Figure 2A, Figures S3 and S4), an evolutionary process could be observed. When K=2, it reflected the differences between clinical service providers (surgical or medical). When K=3, a new cluster was generated and featured worse cardiac and renal function. Finally, in the clustering of K=4, the cluster that featured with surgical service was divided into elective and emergency admissions.
Interestingly, although we did not use any outcome data or severity scoring data in the K-means model, the clusters were extremely associated with outcomes. The mortality rates of the four clusters were 4.0%, 11.8%, 20.4%, and 46.5%, which showed similar discrimination to the SAPS II system (4.8%, 8.5%, 20.1%, and 50.8%), as illustrated in Figure 2C. Here, we sorted all patients according to the SAPS II score in ascending order and divided them into four groups which had the same sizes with the K-means clusters. The mortality and SAPS II score were compared between these two systems. Except for the fourth cluster, we noticed similar SAPS II scores but distinct mortality among the clusters. Especially in the second and third clusters, the median and interquartile range of the SAPS II score was very close {37 [30–45] vs. 38.5 [31–47]}, but the mortality of the second cluster was nearly half that of the third cluster (11.8% vs. 20.3%; Figure 2C).
Furthermore, we validated the abovementioned findings in the eICU database. Because the clinical subtypes of cancer patients in the ICU were actually based on the clinical service provider, we classified patients of the eICU database into surgical and non-surgical groups based on the ICU admission resource: patients from the operation room were identified as belonging to the surgical group; otherwise, they were assigned to the non-surgical group, corresponding to clusters 1+2 and 3+4 in the MIMIC database. The SMR, Hosmer–Lemeshow C statistic, and calibration graph were conducted on the basis of different scoring systems (Table 2 and Figure 3), and the corresponding number of patients in each risk decile were plotted in Figure S5. The calibration of SAPS II for both databases were poor (C=628.07, P<0.001 for the MIMIC database; C=827.64, P<0.001 for the eICU database; as shown in Table 2 and Figure 3A,3B). However, the calibration of APACHE IV in the eICU database was relatively better, despite a P value less than 0.05 (C=87.84, P<0.001; as shown in Table 2, Figure 3C,3D). Furthermore, the SMRs of the surgical groups (cluster 1+2 in the MIMIC database) were lower than those in the non-surgical groups (cluster 3+4 in the MIMIC database) for all comparisons (Table 2 and Figure 3).
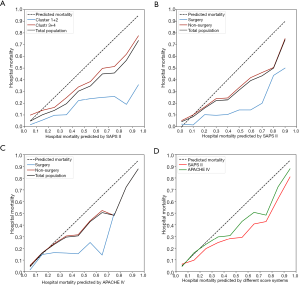
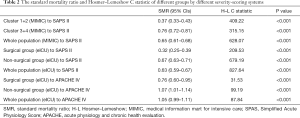
Full table
The number of patients in each risk decile of the APACHE IV were imbalanced: numbers in high-risk deciles were fewer (Figure S5). Therefore, we compared the actual and predicted mortality for lower risk subgroups (Figure 4). The population of each database was divided into segments according to different SPAS II or APACHE IV scores, and the actual mortality, with 95% CIs, of the surgical and non-surgical group in each segment were compared, along with the predicted mortality by scoring systems.
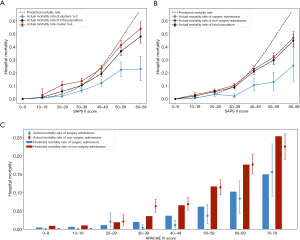
For both databases, the mortality of the surgical group (cluster 1+2 in MIMIC database) was significantly lower than that of the non-surgical group (cluster 3+4 in MIMIC database; Figure 4A,4B). However, as the mortality predicted by the APACHE IV was calculated by the APACHE score and admission information, including diagnoses and admission information, surgical and non-surgical groups had different predicted mortality (Figure 4C). As shown in Figure 4C, the actual mortality was close to the mortality predicted by APACHE IV in these lower risk segments (the mortality of those segments was less than 0.25).
Discussion
The present study showed that, according to the ICD-level mortality (Figure 1, Figure S1), secondary and hematologic malignancies have worse outcomes. Moreover, among the top 5 diagnoses that had the highest mortality rates in the two databases, three of the diagnoses were the same: (I) metastasis involving pleura; (II) metastasis involving the liver; and (III) acute myeloid leukemia. This is consistent with the mainstream opinion that metastatic cancer and hematologic malignancy are independent prognostic factors (23,24). In the SAPS II system, metastatic cancer was assigned an increasing score of 9 whereas hematologic malignancy was scored as 10 (14).
Generally, the primary diagnosis is always the main reason for ICU admission. As shown in Figure 1, most non-metastatic solid cancer held the first position of diagnosis. And in Figure 2, non-metastatic solid cancer more frequently occurs in cluster 1 and 2, which were featured as surgical patients. All the above imply that these patients were admitted to ICU for postoperative monitoring. On the contrary, metastatic cancer and hematologic malignancy are less likely to be the primary diagnosis, which implies that its complications, rather than the cancer itself, necessitated ICU admission for these patients.
We found that different clinical subtypes could be simply identified by the admission information. Hospital mortality varied among these subtypes, and this was consistent with the variation of prognoses reported by previous studies (6,25). The reported hospital mortality rates could be as low as 10%, often in postoperative monitoring cohorts (4,8), and as high as 50% or more, often in non-surgical diagnoses (3,9). This disparity cannot be explained only by illness severity but may indicate a different association of mortality other than that mentioned in the traditional severity scoring systems. One reason for this disparity may be that the acute postoperative stress status (tachycardia, intubation, and so on) causes a deterioration of the score evaluated in the first 24 hours in the ICU. However, more research is needed to validate this rationale.
The SAPS II scoring system didn’t perform well in calibration even though it considered the influence of admission type, partially due to its older age (introduced in 1993). The APACHE IV system takes the admission type and diagnosis into account when predicting mortality according to the APACHE score (26). The system performed well for cancer patients at low risk of mortality in the eICU database of the present study. However, as the number of ICU cancer patients at high risk of mortality in the present study (especially the surgical group) was too low to generate credible conclusions, more evidence is needed. Previous studies have found an apriority of APACHE IV compared to SAPS II in many ICU conditions (27,28), but the evidence in cancer patients was insufficient. The application of the APACHE IV system is limited by its complexity. However, as data science develops, the complexity of this system could be overcome by electronic recording techniques and massive computing power.
The present study supports the theory that cancer patients in the ICU need to be considered separately according to the admission type and clinical service provider. A similar opinion was proposed by Weissman and his colleagues based on their experience and intuition (29). They found significant differences between elective and emergency postoperative patients in the ICU, which corresponds to the first and second clusters in our study. However, our study used a method of machine learning to deal with the high-dimensional clinical data, based on the theory that data intraclusters were more consistent than interclusters. Furthermore, The K-mean algorithm tells us the distance among different clusters. When K=2 we have surgical and medical groups; until K=4 we get elective and emergent/urgent subgroups. It implies that the differences between surgical and medical groups are more obvious than those between elective and emergent/urgent subgroups. Separately analysing the surgical and medical patients (or elective and emergent/urgent surgical) with cancer in ICU can decrease heterogeneity. Some studies addressed this concern and specified patient-selection criteria (7,30,31), but others lacked this additional measure and only treated it as a variable for analysis (1,2,32). This is because there are some other concerns (e.g., sample size, study purpose) to take into account besides heterogeneity for observational research as well as systematic review or meta-analysis.
Puxty and colleagues conducted excellent work on a comprehensive meta-analysis for ICU patients with solid cancers (25). They found that cancer patients admitted as medical patients had increased risk (2- to 4-fold higher) of ICU mortality, and increased risk of in-hospital mortality (6- to 8-fold higher) compared with cancer patients who were surgical admissions; however, they only specified group stratification by different cancer sites. Furthermore, we noticed an outstanding variation among the enrolled studies for both hospital mortality (I2=98.8%, P<0.001) and ICU mortality (I2=99.1%, P<0.001). Therefore, the admission type should have been considered for stratified analysis in Puxty’s research, which may have also improved the heterogeneity of the enrolled studies.
Our study provided ICD-level data on the mortality rate of cancer patients in the ICU from multiple resources, and emphasized the importance of differentiating between admission types and clinical service providers. We discussed the calibration of different severity-scoring systems for cancer patients in the ICU across different clinical subgroups. However, this study has some limitations. First, selection bias might exist, especially in the eICU database, because there were fewer cancer patients in the ICU than reported on average by most studies (~15%). Second, unlike the eICU database, it was difficult to compare the two different severity-scoring systems in the MIMIC database, because the APACHE IV algorithm is only available online (33). Moreover, there were limits deriving from the retrospective nature of the analysis and relatively small sample size for machine learning. Therefore, prospective studies with larger populations are needed in the future.
Conclusions
Similar mortality rates for a spectrum of ICD-level cancer diagnoses were observed within two large ICU databases. Different clinical subtypes of ICU patients with cancer can be well identified by admission type and clinical service provider, and caution should be exercised when considering these patients as a whole population, both in clinical practice and research. The system of APACHE IV performs better than SAPS II for cancer patients at low risk of mortality in the ICU, whereas the same conclusion for those at high risk of mortality needs to be validated in further studies.
Data sharing and data accessibility
The datasets generated and/or analysed during the current study are available in the MIMIC-III v1.4 (https://mimic.physionet.org) and eICU (https://eicu-crd.mit.edu/).
Acknowledgments
We appreciate the researchers at the MIT Laboratory for Computational Physiology for publicly sharing of the clinical databases including Medical Information Mart for Intensive Care-III and eICU database.
Funding: The study was funded by the National Natural Science Foundation of China (No. 81770619).
Footnote
Reporting Checklist: The authors have completed the STROBE reporting checklist. Available at http://dx.doi.org/10.21037/atm-20-4634
Peer Review File: Available at http://dx.doi.org/10.21037/atm-20-4634
Conflicts of Interest: All authors have completed the ICMJE uniform disclosure form (available at http://dx.doi.org/10.21037/atm-20-4634). All the authors report grants from National Natural Science Foundation of China, during the conduct of the study. The authors have no other conflicts of interest to declare.
Ethical Statement: The authors are accountable for all aspects of the work in ensuring that questions related to the accuracy or integrity of any part of the work are appropriately investigated and resolved.
Open Access Statement: This is an Open Access article distributed in accordance with the Creative Commons Attribution-NonCommercial-NoDerivs 4.0 International License (CC BY-NC-ND 4.0), which permits the non-commercial replication and distribution of the article with the strict proviso that no changes or edits are made and the original work is properly cited (including links to both the formal publication through the relevant DOI and the license). See: https://creativecommons.org/licenses/by-nc-nd/4.0/.
References
- Soares M, Caruso P, Silva E, et al. Characteristics and outcomes of patients with cancer requiring admission to intensive care units: a prospective multicenter study. Crit Care Med 2010;38:9-15. [Crossref] [PubMed]
- Taccone FS, Artigas AA, Sprung CL, et al. Characteristics and outcomes of cancer patients in European ICUs. Crit Care 2009;13:R15. [Crossref] [PubMed]
- Caruso P, Ferreira AC, Laurienzo CE, et al. Short- and long-term survival of patients with metastatic solid cancer admitted to the intensive care unit: prognostic factors. Eur J Cancer Care (Engl) 2010;19:260-6. [Crossref] [PubMed]
- Leath CA 3rd, Kendrick JE 4th, Numnum TM, et al. Outcomes of gynecologic oncology patients admitted to the intensive care unit following surgery: A university teaching hospital experience. Int J Gynecol Cancer 2006;16:1766-9. [Crossref] [PubMed]
- Darmon M, Bourmaud A, Georges Q, et al. Changes in critically ill cancer patients' short-term outcome over the last decades: results of systematic review with meta-analysis on individual data. Intensive Care Med 2019;45:977-87. [Crossref] [PubMed]
- Shimabukuro-Vornhagen A, Böll B, Kochanek M, et al. Critical care of patients with cancer. CA Cancer J Clin 2016;66:496-517. [Crossref] [PubMed]
- Puxty K, McLoone P, Quasim T, et al. Characteristics and Outcomes of Surgical Patients With Solid Cancers Admitted to the Intensive Care Unit. JAMA Surg 2018;153:834-40. [Crossref] [PubMed]
- Park DP, Welch CA, Harrison DA, et al. Outcomes following oesophagectomy in patients with oesophageal cancer: a secondary analysis of the ICNARC Case Mix Programme Database. Crit Care 2009;13 Suppl 2:S1. [Crossref] [PubMed]
- Reichner CA, Thompson JA, O'Brien S, et al. Outcome and code status of lung cancer patients admitted to the medical ICU. Chest 2006;130:719-23. [Crossref] [PubMed]
- Ishida T, Inoue T, Sugio K, et al. Early squamous lung cancer and longer survival rates. Respiration 1993;60:359-65. [Crossref] [PubMed]
- Johnson AEW, Pollard TJ, Shen L, et al. MIMIC-III, a freely accessible critical care database. Sci Data 2016;3:160035. [Crossref] [PubMed]
- Pollard TJ, Johnson AEW, Raffa JD, et al. The eICU Collaborative Research Database, a freely available multi-center database for critical care research. Sci Data 2018;5:180178. [Crossref] [PubMed]
- Feng M, McSparron JI, Kien DT, et al. Transthoracic echocardiography and mortality in sepsis: analysis of the MIMIC-III database. Intensive Care Med 2018;44:884-92. [Crossref] [PubMed]
- Le Gall JR, Lemeshow S, Saulnier F. A new Simplified Acute Physiology Score (SAPS II) based on a European/North American multicenter study. JAMA 1993;270:2957-63. [Crossref] [PubMed]
- van Walraven C, Austin PC, Jennings A, et al. A modification of the Elixhauser comorbidity measures into a point system for hospital death using administrative data. Med Care 2009;47:626-33. [Crossref] [PubMed]
- Vincent JL, Moreno R, Takala J, et al. The SOFA (Sepsis-related Organ Failure Assessment) score to describe organ dysfunction/failure. Intensive Care Med 1996;22:707-10. [Crossref] [PubMed]
- mimic-code. Accessed Feb 4th 2020. Available online: https://github.com/MIT-LCP/mimic-code/tree/master/concepts/severityscore
- Yadav J, Sharma M. A Review of K-mean Algorithm. Int J Latest Trends Eng Technol 2013;4:2972-6.
- Kodinariya TM, Makwana PR. Review on determining number of Cluster in K-Means Clustering. Int J Adv Res Comp Sci Manag Stud 2013;1:90-5.
- Ivosev G, Burton L, Bonner R. Dimensionality reduction and visualization in principal component analysis. Anal Chem 2008;80:4933-44. [Crossref] [PubMed]
- Hosmer DW, Hosmer T, Le Cessie S, et al. A comparison of goodness-of-fit tests for the logistic regression model. Stat Med 1997;16:965-80. [Crossref] [PubMed]
- Vandenbroucke JP. A shortcut method for calculating the 95 per cent confidence interval of the standardized mortality ratio. Am J Epidemiol 1982;115:303-4. [Crossref]
- Mendoza V, Lee A, Marik PE. The hospital-survival and prognostic factors of patients with solid tumors admitted to an ICU. Am J Hosp Palliat Care 2008;25:240-3. [Crossref] [PubMed]
- Oeyen SG, Benoit DD, Annemans L, et al. Long-term outcomes and quality of life in critically ill patients with hematological or solid malignancies: a single center study. Intensive Care Med 2013;39:889-98. [Crossref] [PubMed]
- Puxty K, McLoone P, Quasim T, et al. Survival in solid cancer patients following intensive care unit admission. Intensive Care Med 2014;40:1409-28. [Crossref] [PubMed]
- Zimmerman JE, Kramer AA, McNair DS, et al. Acute Physiology and Chronic Health Evaluation (APACHE) IV: hospital mortality assessment for today's critically ill patients. Crit Care Med 2006;34:1297-310. [Crossref] [PubMed]
- Lee H, Yoon S, Oh SY, et al. Comparison of APACHE IV with APACHE II, SAPS 3, MELD, MELD-Na, and CTP scores in predicting mortality after liver transplantation. Sci Rep 2017;7:10884. [Crossref] [PubMed]
- Norouzi K, Mashmool Z, Dalvandi A, et al. Comparison of two tools APACHE IV and SAPS II in predicting mortality rate in patients hospitalized in intensive care unit. Koomesh 2015;16:347-55.
- Weissman C, Klein N. The importance of differentiating between elective and emergency postoperative critical care patients. J Crit Care 2008;23:308-16. [Crossref] [PubMed]
- Aygencel G, Turkoglu M, Turkoz Sucak G, et al. Prognostic factors in critically ill cancer patients admitted to the intensive care unit. J Crit Care 2014;29:618-26. [Crossref] [PubMed]
- Ostermann M, Ferrando-Vivas P, Gore C, et al. Characteristics and Outcome of Cancer Patients Admitted to the ICU in England, Wales, and Northern Ireland and National Trends Between 1997 and 2013. Crit Care Med 2017;45:1668-76. [Crossref] [PubMed]
- Bos MMEM, Bakhshi-Raiez F, Dekker JWT, et al. Outcomes of intensive care unit admissions after elective cancer surgery. Eur J Surg Oncol 2013;39:584-92. [Crossref] [PubMed]
- Apache IV Score. Accessed Feb 16th 2020. Available online: https://intensivecarenetwork.com/Calculators/Files/Apache4.html