Enhanced expression of queuine tRNA-ribosyltransferase 1 (QTRT1) predicts poor prognosis in lung adenocarcinoma
Introduction
Lung cancer is the the leading cause of cancer death worldwide (1). Lung adenocarcinoma (LUAD) is more than any other subtypes of lung cancer (2), and patients with LUAD often die from relapse and metastasis (3-5). Bioinformatics analyses can help to discover novel biomarkers of LUAD, so as to help improve prognosis and treatment.
Queuine tRNA-ribosyltransferase 1 (QTRT1), also known as tRNA-guanine transglycosylase (TGT), is located on chromosome 19p13.2 (6). It contains 10 exons spanning approximately 12 kb. The enzyme queuine tRNA-ribosyltransferase (QTRT) is encoded by the gene QTRT1. Its molecular function is the exchange of a guanine (G) with queuine (Q) at position 34, resulting in hypermodified transfer RNAs (tRNAs) (7). QTRT1 is a key enzyme involved in the post-transcriptional modification of tRNAs. These tRNAs are central components of protein synthesis and the cell signaling network, and their modulation can specifically impact cancer progression (8). Specific tRNAs are related to human breast cancer, colon adenocarcinoma, and human lung cancer (9-12). At the protein level, western blotting results have shown that QTRT1 expression in the mitochondrion of human LUAD A549 cells was higher than that in normal human bronchial epithelial 16HBE cells. Meanwhile, immunohistochemistry (IHC) results have shown that positive expression of QTRT1 in LUAD was significantly higher compared to normal lung tissues (13). Previous studies have also shown that QTRT1 may be involved in the carcinogenesis of human colon adenocarcinoma through modulating tRNAs (11). However, the correlation between QTRT1 and LUAD remains largely unknown, and the specific mechanism of QTRT1 has not been reported.
In this study, we explored the relationship between QTRT1 expression, methylation, and clinical features (demographic factors, pathology stage, residual tumor, and survival outcome) in LUAD by using the gene expression data of 1,012 LUAD samples and 112 normal controls. For the first time, QTRT1 was found to be a risk factor for the onset and progression of LUAD. A significant increase in QTRT1 expression in LUAD patients was observed. Furthermore, a marked down-regulation in QTRT1 methylation in LUAD was also found. Higher expression of QTRT1 in LUAD was related to negative survival outcomes. Therefore, QTRT1 can be used as a novel predictor for survival in patients with LUAD. Furthermore, the biological functions of the identified target genes were interpreted. We present the following article in accordance with the REMARK reporting checklist (available at http://dx.doi.org/10.21037/atm-20-7424).
Methods
The study was conducted in accordance with the Declaration of Helsinki (as revised in 2013).
Data sources
Data was obtained from The Cancer Genome Atlas (TCGA) and Gene Expression Omnibus (GEO) sources, namely, microarrays, methylation, and RNA sequencing. A total of 502 LUAD patients with survival outcomes were selected from TCGA, however, 128 patients’ clinical information were not available. A total of 374 patients were further selected for analyzing the differences in clinical factors between QTRT1high and QTRT1low expression groups. The expression level, survival analysis, Gene Ontology (GO) term enrichment, Kyoto Encyclopedia of Genes and Genomes (KEGG) pathway analysis, module screening from the protein-protein interaction (PPI) network, and gene set enrichment analysis (GSEA) were also carried out in TCGA. All clinical information (including gender, age, pathological stage, as well as residual tumor) is available in TCGA Data Portal. The project ID is TCGA-LUAD.
Additionally, 510 LUAD samples and 112 control lung tissue samples from 4 GEO databases were further obtained to analyze expression, methylation, and survival status. GSE10072 (n=66) was used for expression analysis, and there were 2 methylation datasets [GSE49996 (n=88) and GSE63384 (n=70)]. In GSE63384, tumor samples and adjacent non-tumor tissues were selected from stage I LUAD. The fourth dataset [GSE72094 (n=398)] was used to assess the relationship between QTRT1 expression and prognosis.
Microarray analysis
Patients with QTRT1 expression values above the median for all LUAD cases were classified as high-QTRT1, whilst all other cases were considered to be low-QTRT1. P value <0.05 in unpaired t-test analysis and fold change (FC, log2) >0.5 or <−0.5 was utilized to determine the differential expression of genes (DEGs).
Functional analysis
GO term enrichment, KEGG pathway analysis, PPI network, and GSEA were constructed to reveal the hub genes of the potential target genes on STRING, a web portal for undermining the integrated function of multiple genes (14,15).
Statistical analysis
R software 3.5.0 was used for performing all statistical analyses. Kolmogorov-Smirnov test was done for evaluating each dataset for normality of distribution to decide whether a parametric analysis or a non-parametric rank-based analysis should be used. Wilcoxon rank-sum tests and Fisher’s exact were used to test hypotheses in continuous and categorical variables, respectively. The limma package was used for performing different gene expression analysis (16). The samples in TCGA were divided into 2 groups (high-QTRT1, n=251, low-QTRT1, n=251) based on the median expression value of QTRT1. GSE72094 was divided into 2 groups (high-QTRT1, n=199; low-QTRT1, n=199).
For the estimation of the survival status, Kaplan-Meier method and Cox regression multivariate analyses were carried out. And the group comparisons were made by using the log-rank test. Cluster profiler package was used to identify GO enriched terms and KEGG pathways (17). P value <0.05 was considered significant.
Results
Expression and methylation of QTRT1 in LUAD
We employed 3 GEO datasets to analyze the expression and methylation level of QTRT1 in LUAD. In the GSE10072 dataset, a significant increase in QTRT1 expression in LUAD tissues (n=33) was found (P=0.0024) compared to normal control tissues (n=33; Figure 1A). An obvious downward trend in QTRT1 methylation in LUAD tissue was further validated in GSE49996, which included normal tissues (n=44) and LUAD samples (n=44; Figure 1B). The same decreasing trend was also found in the GSE63384 dataset in the LUAD group (n=35) compared to the control group (n=35; Figure 1C).
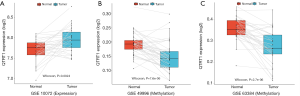
Differences in clinical factors in LUAD between the QTRT1high and QTRT1low groups
The baseline characteristics between the high and low QTRT1 expression groups were analyzed. Clinical factors included age, gender, stage, residual tumor (R0: without residual tumor, R1: microscopic residual, R2: macroscopic residual tumor, Rx: unknown), tumor size (T) (referring to the TNM stage), lymph node (N) (N0: negative for lymph node metastasis, N1/N2/N3: positive for lymph node metastasis, Nx: unknown lymph node metastasis), and distant metastasis (M) (M0: negative for distant metastasis, M1: positive for distant metastasis, Mx: unknown distant metastasis).
In the 502 LUAD cases selected from TCGA, clinical data from 128 patients were not available. Hence, clinical data from 374 patients were used for analyses. The samples were divided into two groups based on the median values of QTRT1 expression: QTRT1low (n=187) and QTRT1high (n=187). Consistent with tumor stage association, patients with higher QTRT1 expression had less residual tumors (R0 vs. R1/R2/Rx, P=0.029) and less distant metastasis (M0 vs. M1/Mx, P=0.013; Table 1). No significant correlation was observed for age (P=0.342), gender (P=0.756), stage (I/II/III/IV, P=0.509), T stage (P=0.071), and N stage (P=0.687; Table 1).
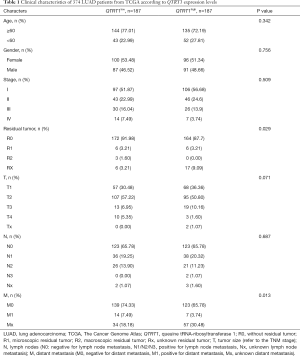
Full table
Association between survival and QTRT1 expression in meta-datasets
The GSE72094 dataset (n=398) was divided into 2 groups: high-QTRT1 (n=199) and low-QTRT1 (n=199). We observed that high expression of QTRT1 was associated with worse overall survival (OS; P=0.0033; Figure 2A). Survival analysis was also performed based on TCGA dataset (n=502). Differences were observed in the survival times for the low-QTRT1 group (n=251) and the high-QTRT1 group (n=251). For disease-free survival (DFS), higher QTRT1 expression had a worse outcome (P=0.0036; Figure 2B). Similarly, higher QTRT1 expression was associated with significantly shorter OS (P=0.0022; Figure 2C). These results suggest that QTRT1 might be an effective gene in the progression of LUAD.
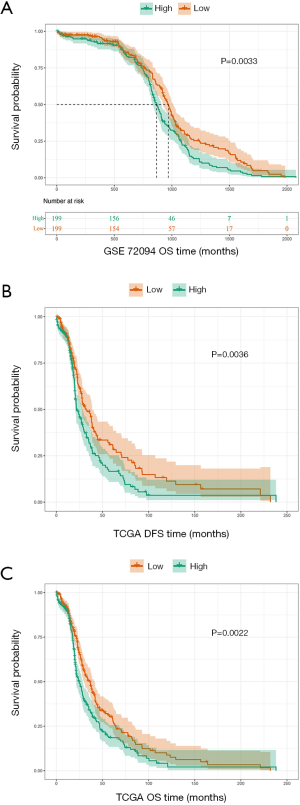
Prognostic value of QTRT1 expression in LUAD based on TCGA dataset
Hazard ratios (HRs) were computed for different variables based on TCGA dataset by using the Cox regression model. Univariate analysis results showed that QTRT1, EGFR, and KRAS were all significantly related to DFS and OS. The HR of the QTRT1high group was 1.32 (P=0.026) for DFS analysis. The HR of the QTRT1high group was 1.26 (P=0.031) for OS analysis. EGFR (HR =1.32, P=0.024) and KRAS mutations (HR =1.30, P=0.034) were both risk factors for DFS analysis. Similarly, for OS analysis, EGFR (HR =1.21, P=0.078) and KRAS mutations (HR =1.32, P=0.010) were also negative for survival (Table 2). Other clinical factors such as age, gender, and other genetic alterations (ALK, LHX5, NRAS, PIK3CA, TP53) were not significantly different.
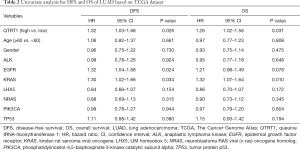
Full table
Furthermore, in the multivariate analysis for DFS, the HR of EFGR was 1.32 (P=0.034), the HR of KRAS was 1.37 (P=0.016), while the HR of QTRT1 expression was 1.37 (P=0.020). These three factors were significantly related to DFS in LUAD patients. The HR of KRAS was 1.38 (P=0.006) and the HR of QTRT1 was 1.27 (P=0.044) for OS, indicating that these 2 mutation genes were closely associated with OS. QTRT1 expression was a stable risk factor affecting the survival time of LUAD (Table 3). Therefore, these results suggest that QTRT1, EGFR, and KRAS are involved in the occurrence and development of LUAD, and QTRT1 expression can be used as a novel biomarker for the prognosis of LUAD.
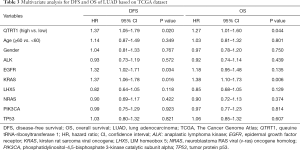
Full table
Differential expression genes between the QTRT1high and QTRT1low groups
The different expression profiles of QTRT1high versus QTRT1low were analyzed to find the genes associated with QTRT1. As many as 101 genes were up-regulated and 157 genes were down-regulated [P<0.05, (FC, log2) >0.5, or <−0.5, Figure 3A]., The enriched GO terms were analyzed by using DEGs. Among the biological process terms of GO, most of the DEGs were enriched in ‘axoneme assembly’, ‘microtubule bundle formation’, ‘axonemal dynein complex assembly’, and ‘inner dynein arm assembly’ (Figure 3B). KEGG analysis showed that ‘olfactory transduction’ and ‘inoleic acid metabolism’ were the most enriched pathways (Figure 3C). Top 13 DEGs were used to screen the PPI network in the STRING database. Two down-regulated genes (DEFB128 and OR10G9) and eleven of the up-regulated genes and were interactional in the PPI network (Figure 3D).
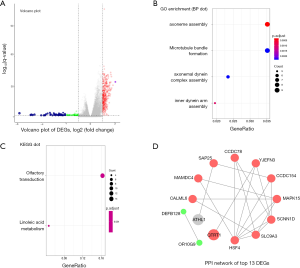
Hallmark analyses of differential expression genes between the QTRT1high and QTRT1low groups
While the DEGs can give important clues about the mechanistic aspects of cellular regulation, many genes are regulated in concert. In order to categorize such modules of cellular regulation, bioinformatics approaches for GSEA have been developed (18). We performed GSEA on transcriptomics data downloaded from TCGA. These genes were involved in the ‘androgen response’, ‘epithelial mesenchymal transition (EMT)’, ‘protein secretion’, and ‘UV-response-DN’ (Figure 4). Significantly enriched gene sets (P<0.05) are shown in Figure 5A,B,C,D.
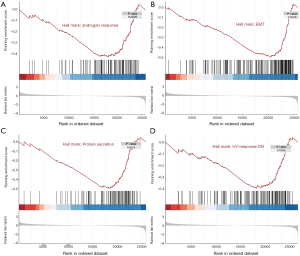
Discussion
At present, the specific molecular mechanism of QTRT1 in LUAD remains unknown. A highlight of this study was the use of computational bioinformatics methods to explore the latent mechanism of QTRT1 in LUAD at the gene level. At the protein level, western blotting results have shown that QTRT1 expression in the mitochondrion of human LUAD A549 cells was higher than that in the normal human bronchial epithelial 16HBE cells. Meanwhile, IHC results showed that positive QTRT1 expression in LUAD was significantly higher than that in normal lung tissues (13). However, there are currently no reports on the correlation between QTRT1 expression and the clinical features of LUAD at the gene level.
For the first time, QTRT1 was found to be a risk factor for the onset and progression of LUAD. A significant increase in QTRT1 expression in LUAD patients was observed compared with normal tissues. An obvious downregulation in QTRT1 methylation in LUAD was also found. Furthermore, higher expression of QTRT1 in LUAD was associated with worse prognosis. Therefore, QTRT1 can be considered a tumor promoter gene in LUAD, and can be used as a novel predictor of survival in LUAD patients. However, the negative prognostic value of increased expression of QTRT1 of other pathological types of lung cancer have not been found.
QTRT1 is a protein coding gene located on chromosome 19p13.2, and contains 10 exons spanning approximately 12 kb. The enzyme QTRT, also known as TGT, is encoded by the gene QTRT1. The active eukaryotic TGT is a heterodimer in solution, comprised of a catalytic QTRT1 subunit and a noncatalytic QTRT2 subunit (6). Previous studies have shown that the molecular function of QTRT is the exchange of a G with Q at position 34, resulting in hypermodified tRNAs. QTRT is a key enzyme involved in the post-transcriptional modification of tRNAs. It has been reported that QTRT1 may be involved in the carcinogenesis of human colon adenocarcinoma through modulating tRNAs (11).
These tRNAs have been primarily viewed as static contributors to gene expression. However, they can specifically impact cancer progression, being involved in enhanced invasiveness (8-10,19-21). Goodarzi et al. found that specific tRNAs were upregulated in human breast cancer metastasis (22). Q negative tRNA is the original transcript, which contains G at the wobble’s position (the 34th nucleotide from the 5' end). When this G is replaced by Q in a direct base for base exchange, Q negative tRNA is modified to Q positive tRNA (23-25). This modification of tRNAs is one manifestation of the process of glycosylation. Glycosylation is a form of co-translational and post-translational modification (26). It plays an important role in protein folding and stability. Abnormal glycosylation is associated with cell-to-cell adhesion and malignant transformation (27,28). As one of the metabolites, glycosylation is currently considered as a target for cancer treatments (29).
Modification of tRNA was found to be highly correlated with the histopathological grading of human malignant tumors (30,31). The tRNAs of tumors, especially those with poor prognosis and malignant tumors, are often undermodified (32). Chen et al. demonstrated that the key role of tRNA modification was to support specific translation during breast cancer progression (7). Shindo-Okada et al. found that Q negative tRNA levels in murine erythroleukemic cells decreased markedly when the cells became more differentiated (33). Huang et al. reported that Q negative tRNA was found to be highly correlated with the histopathological classification and prognosis of human lung cancer (12). There was also a strong relationship between the malignancy grading of leukemias and lymphomas, and Q positive tRNA (34,35).
Bioinformatics analyses were performed to fully understand the specific molecular mechanism of QTRT1 in LUAD. According to the GO term enrichment, QTRT1 might have an important effect on the progression of LUAD by modulating several cellular processes, such as ‘axoneme assembly’, ‘microtubule bundle formation’, ‘axonemal dynein complex assembly’ and ‘inner dynein arm assembly’. Optimal cellular function requires the precise regulation of axoneme assembly and membrane biogenesis. Axoneme, microtubules, and dynein are important for maintaining tissue architecture and homeostasis. Disruption of primary cilia structure and function is increasingly recognized in cancers (36). Recent studies have suggested roles for these organelles in oncogenesis and tumor suppression. Zhu et al. reported that ‘cilium axoneme assembly’ was one of key pathways in nasopharyngeal carcinoma (37), and O’Toole et al. demonstrated that ciliary disruption contributes to tumorigenesis in endocrine-related cancers (38).
The results of the KEGG analysis also demonstrated the role of QTRT1 in LUAD. The top 2 enriched pathways were ‘olfactory transduction’ and ‘linoleic acid metabolism’. However, few studies have been conducted on these pathways in LUAD. Mukhopadhyay et al. found that sensory signaling was required to maintain the architecture of the specialized olfactory neuron cilia in C. elegans, and decreased sensory signaling resulted in alterations in axoneme length and expansion of the membranous structure (39). A study by Bai et al. showed that these pathways were connected mainly to differentially expressed genes, such as low Raps homolog gene family member A (RhoA), and RhoA affects the development of hepatocellular carcinoma (HCC) (40). After integrating the GO and KEGG analyses results above, QTRT1 might therefore relate to other associated genes (such as EGFR and KRAS) and play a key role in the occurrence and development of LUAD.
The PPI network results indicated that QTRT1 was a hub gene in the network. QTRT1 interacted with many other genes (YJEFN3, SAP25, OR10G9, HSF4, CALML6, MAMDC4, SLC9A3, SCNN1D, MAPK15, and CCDC154). Human YJEFN domain containing protein family was found to be related to cholesterol processing and steroid hormone metabolism in spermiogenesis and oogenesis (41). IHC of human testes and ovaries showed expression of hYJEFN3 only in Leydig cells and theca cells, respectively, indicating a role in steroid hormone metabolism (42). Sin3A-associated protein 25 (SAP25) was related to ovarian cancer chemoresistance (43). The developing human ovaries express the distinct gene OR10G9 (olfactory receptor, family 10, subfamily G, member 9) which is related to adrenal and reproductive disorders (44). HSF4 (heat shock factor 4) is a member of the HSF family, and its expression correlates with its role in cell growth and differentiation (45). High HSF4 expression was an independent prognostic factor of poor OS for colorectal cancer (46). Decreased levels of calmodulin-like 6 (CALML6) has been linked to poorer colon cancer patient survival (47), and MAM domain containing 4 (MAMDC4) was associated with the prognosis of esophageal adenocarcinoma (48). Cancer cells maintain low intracellular pH. SLC9A3 is involved in pH regulation to eliminate acids generated by active metabolism or to counter adverse environmental conditions. Dorfman et al. reported that the SLC9A3 gene encoded an Na+/H+ exchanger (49). Kang et al. reported that SLC9A3 was one of the most frequent genetic events in the early stages of non-small cell lung cancer (50), and Bai et al. showed that the expression level of SLC9A3-AS1 in lung cancer was significantly different from healthy controls (51). Meanwhile, SCNNl genes encode epithelial sodium channels (ENaCs). ENaCs play a crucial role in maintaining sodium and water balance in the pulmonary system. Mutations in SCNN1 genes have been shown to affect the structure and function of ENaCs, leading to a series of lung diseases (52). Mitogen-activated protein kinase 15 (MAPK15) is an atypical member of the mitogen-activated protein (MAP) kinase family that has recently emerged as a key modulator of cell transformation. Knockdown of MAPK15 in gastric cancer cells significantly suppressed cell proliferation (53). For lung cancer, Wu et al. reported that As2O3 can selectively kill lung cancer cells with high MAPK15 expression by activating NF-κB (54). Overexpression of coiled-coil domain-containing 154 (CCDC154) inhibited tumor cell growth by inducing G2/M arrest (55). We therefore hypothesized that QTRT1 might exert its oncogenic effects in LUAD via some of these genes. As demonstrated in this study, up-regulation of QTRT1 expression was closely related to the progression of LUAD.
The results of GSEA indicated that QTRT1 might affect LUAD through ‘androgen response’, ‘epithelial mesenchymal transition (EMT)’, ‘protein secretion’ and ‘UV-response-DN’. The reasons for gender-associated differences in lung cancer mortality are unclear. Although the incidence of lung cancer in men has declined, it had sharply risen in women since 2004 (56,57). Estrogen is a known risk factor for the development of LUAD (58-61). Estrogen can directly stimulate transcription in the nucleus of pulmonary cells and activate growth factor signaling pathways (62). On the other hand, androgen depletion might significantly improve the short-term survival of men with lung cancer (63). Liu et al. reported that positive expression of androgen receptors might play a role in the progression and lymph node metastasis of NSCLC, and predict poor prognosis (64). Maßberg et al. found that the olfactory receptor OR51E1 [prostate-specific G-protein coupled receptor 2 (PSGR2)] had an impact on androgen receptor-mediated signaling in the growth processes of prostate cancer cells (65). EMT has an important function in the rebuilding of the fibrillar connective tissue and LUAD (66,67). During EMT, epithelial proteins disappear and mesenchymal proteins are expressed. This transition can enhance lung cancer cell invasiveness (68,69). As for ‘protein secretion’, many proteins act as chemokines (a family of small cytokines), and CX3CL1 serves as a prognostic marker for LUAD (70). ‘UV-response-DN’ refers to genes that are down-regulated by ultraviolet (UV) radiation. UV radiation is an omnipresent exposure with confirmed detrimental effects on cancer risk (71). It has been shown to be involved in regulating lung cancer cell apoptosis through the p38 MAPK signaling pathway (72-74).
The current study has limitations. The association between QTRT1 expression and LUAD should be validated in large prospective trials before it can be translated into clinical practice. The hypothesis that the mechanism involved in the negative regulation of QTRT1 expression is the methylation of QTRT1 should be validated. Furthermore, functional studies are required to fully understand the molecular mechanism of QTRT1 in LUAD progression (cell migration, cell cycle, and animal model experiments), as well as to elucidate its role in pathophysiology. There are no medicine targeting QTRT1 for the treatment of lung adenocarcinoma right now. QTRT1 would be a potential target gene therapy in the future medical research. The clinical transformation of the results is a biomarker for early screening of lung adenocarcinoma.
In conclusion, this is the first study to find that QTRT1 is highly expressed in LUAD. A marked down-regulation in QTRT1 methylation in LUAD was found, and more importantly, LUAD patients with low QTRT1 expression consistently had longer survival times. Therefore, our results indicate that high QTRT1 expression is a biomarker for poor prognosis in LUAD patients, and QTRT1 may function as a latent tumor biomarker in the prognostic prediction for LUAD. The results of bioinformatics analyses provided new insights into the pathological processes associated with QTRT1 expression in LUAD. Further studies, including deeper genomic research, are therefore warranted.
Acknowledgments
We thank the TCGA and GEO database and all the providers of the datasets used in this report. We also thank Chaozeng Si and Yang Chen for their help with data analyses.
Funding: The 63rd National Science Foundation for Postdocs (179300).
Footnote
Reporting Checklist: The authors have completed the REMARK reporting checklist. Available at http://dx.doi.org/10.21037/atm-20-7424
Conflicts of Interest: Both authors have completed the ICMJE uniform disclosure form (available at http://dx.doi.org/10.21037/atm-20-7424). The authors have no conflicts of interest to declare.
Ethical Statement: The authors are accountable for all aspects of the work in ensuring that questions related to the accuracy or integrity of any part of the work are appropriately investigated and resolved. The study was conducted in accordance with the Declaration of Helsinki (as revised in 2013).
Open Access Statement: This is an Open Access article distributed in accordance with the Creative Commons Attribution-NonCommercial-NoDerivs 4.0 International License (CC BY-NC-ND 4.0), which permits the non-commercial replication and distribution of the article with the strict proviso that no changes or edits are made and the original work is properly cited (including links to both the formal publication through the relevant DOI and the license). See: https://creativecommons.org/licenses/by-nc-nd/4.0/.
References
- Siegel R, Naishadham D, Jemal A. Cancer statistics, 2012. CA Cancer J Clin 2012;62:10-29. [Crossref] [PubMed]
- Travis WD. Pathology of Lung cancer. Clin Chest Med 2011;32:669-92. [Crossref] [PubMed]
- Ahn S, Jeong JY, Kim HW, et al. Robotic lobectomy for lung cancer: initial experience of a single institution in Korea. Ann Cardiothorac Surg 2019;8:226-32. [Crossref] [PubMed]
- Greenhalgh J, Dwan K, Boland A, et al. First-line treatment of advanced epidermal growth factor receptor (EGFR) mutation positive non-squamous non-small cell lung cancer. Cochrane Database Syst Rev 2016.CD010383. [Crossref] [PubMed]
- Buss EJ, Wang TJC. Treatment of lung adenocarcinoma brain metastases: what is the role of radiotherapy in the age of precision medicine? Transl Lung Cancer Res. 2018;7:S318-20. [Crossref] [PubMed]
- Johannsson S, Neumann P, Ficner R. Crystal Structure of the Human tRNA Guanine Transglycosylase Catalytic Subunit QTRT1. Biomolecules 2018;8:1-16. [Crossref] [PubMed]
- Chen YC, Kelly VP, Stachura SV, et al. Characterization of the human tRNA-guanine transglycosylase: confirmation of the heterodimeric subunit structure. RNA 2010;16:958-68. [Crossref] [PubMed]
- Liu F, Clark W, Luo G, et al. ALKBH1-Mediated tRNA Demethylation Regulates Translation. Cell 2016;167:1897. [Crossref] [PubMed]
- Goodarzi H, Liu X, Nguyen HC, et al. Endogenous tRNA-derived fragments suppress breast cancer progression via YBX1 displacement. Cell 2015;161:790-802. [Crossref] [PubMed]
- Delaunay S, Rapino F, Tharun L, et al. Elp3 links tRNA modification to IRES-dependent translation of LEF1 to sustain metastasis in breast cancer. J Exp Med 2016;213:2503-23. [Crossref] [PubMed]
- Deshpande KL, Katze JR. Characterization of cDNA encoding the human tRNA-guanine transglycosylase (TGT) catalytic subunit. Gene 2001;265:205-12. [Crossref] [PubMed]
- Huang BS, Wu RT, Chien KY. Relationship of the queuine content of transfer ribonucleic acids to histopathological grading and survival in human lung cancer. Cancer Res 1992;52:4696-700. [PubMed]
- Liu Y, Mu J, Wang S, et al. The expression and clinical signifcance of tRNA-guanine transglycosylase in lung adenocarcinoma. Chinese Journal of the Frontiers of Medical Science 2017;9:55-8.
- Szklarczyk D, Franceschini A, Wyder S, et al. STRING v10: protein–protein interaction networks, integrated over the tree of life. Nucleic Acids Res 2015;43:D447-52. [Crossref] [PubMed]
- Chandrashekar DS, Bashel B, Sah B, et al. UALCAN: A Portal for Facilitating Tumor Subgroup Gene Expression and Survival Analyses. Neoplasia 2017;19:649-58. [Crossref] [PubMed]
- Ritchie ME, Phipson B, Wu D, et al. limma powers differential expression analyses for RNA-sequencing and microarray studies. Nucleic Acids Res 2015;43:e47. [Crossref] [PubMed]
- Yu G, Wang LG, Han Y, et al. cluster Profiler: an R package for comparing biological themes among gene clusters. OMICS 2012;16:284-7. [Crossref] [PubMed]
- Subramanian A, Tamayo P, Mootha VK, et al. Gene set enrichment analysis: a knowledge-based approach for interpreting genome-wide expression profiles. Proc Natl Acad Sci U S A 2005;102:15545-50. [Crossref] [PubMed]
- Ghildiyal M, Zamore PD. Small silencing RNAs: an expanding universe. Nat Rev Genet 2009;10:94-108. [Crossref] [PubMed]
- Rapino F, Delaunay S, Zhou Z, et al. tRNA Modification: Is Cancer Having a Wobble? Trends Cancer 2017;3:249-52. [Crossref] [PubMed]
- Balatti V, Nigita G, Veneziano D, et al. tsRNA signatures in cancer. Proc Natl Acad Sci U S A 2017;114:8071-6. [Crossref] [PubMed]
- Goodarzi H, Nguyen HCB, Zhang S, et al. Modulated Expression of Specific tRNAs Drives Gene Expression and Cancer Progression. Cell 2016;165:1416-27. [Crossref] [PubMed]
- Okada N, Noguchi S, Kasai H, et al. Novel mechanism of post-transcriptional modification of tRNA. Insertion of bases of Q precursors into tRNA by a specific tRNA transglycosylase reaction. J Biol Chem 1979;254:3067-73. [PubMed]
- Farkas WR. Queuine, The Q-containing tRNAs and the Enzymes Responsible for Their Formation. Nucleosides and Nucleotides 1983;2:1-20. [Crossref]
- Reuter K, Slany R, Ullrich F, et al. Structure and organization of Escherichia coli genes involved in biosynthesis of the deazaguanine derivative queuine, a nutrient factor for eukaryotes. J Bacteriol 1991;173:2256-64. [Crossref] [PubMed]
- Dalziel M, Crispin M, Scanlan CN, et al. Emerging principles for the therapeutic exploitation of glycosylation. Science 2014;343:1235681. [Crossref] [PubMed]
- Geng F, Shi B, Yuan Y, et al. The expression of core fucosylated E-cadherin in cancer cells and lung cancer patients: prognostic implications. Cell Res 2004;14:423-33. [Crossref] [PubMed]
- Kannagi R, Izawa M, Koike T, et al. Carbohydrate-mediated cell adhesion in cancer metastasis and angiogenesis. Cancer Sci 2004;95:377-84. [Crossref] [PubMed]
- Tennant DA, Durán RV, Gottlieb E. Targeting metabolic transformation for cancer therapy. Nat Rev Cancer 2010;10:267-77. [Crossref] [PubMed]
- Emmerich B, Zubrod E, Weber H, et al. Relationship of queuinelacking transfer RNAs to the grade of malignancy in human leukemias and lymphomas. Cancer Res 1985;45:4308-14. [PubMed]
- Baranowski W, Dirheimer G, Jakowicki JA. Deficiency of queuine, a highly modified purine base, in transfer RNAs from primary and metastatic ovarian malignant tumors in women. Cancer Res 1994;54:4468-71. [PubMed]
- Kasai H, Oashi Z, Harada F, et al. Structure of the modified nucleoside Q isolated from Esherichia coli transfer ribonucleic acid. 7-(4, 5-cis-dihydroxy-1-cyclopenten-3-ylaminomethyl)-7-deazaguanosine. Biochemistry 1975;14:4198-208. [Crossref] [PubMed]
- Shindo-Okada N, Terada M, Nishimura S. Changes in amount of hypomodified tRNA having guanine in place of queuine during erythroid differentiation of murine erythroleukemia cells. Eur J Biochem 1981;115:423-8. [Crossref] [PubMed]
- Ishiwata S, Katayama J, Shindo H. Increased expression of queuosine synthesizing enzyme, tRNA-guanine transglycosylase, and queuosine levels in tRNA of leukemic cells. J Biochem 2001;129:13-7. [Crossref] [PubMed]
- Emmerich B, Zubrod E, Weber H, et al. Relationship of queuine-lacking transfer RNAs to the grade of malignancy in human leukemias and lymphomas. Cancer Res 1985;45:4308-14. [PubMed]
- Seeley ES, Nachury MV. Constructing and deconstructing roles for the primary cilium in tissue architecture and cancer. Methods Cell Biol 2009;94:299-313. [Crossref] [PubMed]
- Zhu HM, Fei Q, Qian LX, et al. Identification of key pathways and genes in nasopharyngeal carcinoma using bioinformatics analysis. Oncol Lett 2019;17:4683-94. [Crossref] [PubMed]
- O'Toole SM, Chapple JP. Primary cilia: a link between hormone signalling and endocrine-related cancers? Biochem Soc Trans 2016;44:1227-34. [Crossref] [PubMed]
- Mukhopadhyay S, Lu Y, Shaham S, et al. Sensory signaling-dependent remodeling of olfactory cilia architecture in C. elegans. Dev Cell 2008;14:762-74. [Crossref] [PubMed]
- Bai Y, Xie F, Miao F, et al. The diagnostic and prognostic role of RhoA in hepatocellular carcinoma. Aging (Albany NY) 2019;11:5158-72. [Crossref] [PubMed]
- Rudolph C, Sigruener A, Hartmann A, et al. ApoA-I-binding protein (AI-BP) and its homologues hYjeF_N2 and hYjeF_N3 comprise the YjeF_N domain protein family in humans with a role in spermiogenesis and oogenesis. Horm Metab Res 2007;39:322-35. [Crossref] [PubMed]
- Fang L, Choi SH, Baek JS, et al. Control of angiogenesis by AIBP-mediated cholesterol efflux. Nature 2013;498:118-22. [Crossref] [PubMed]
- Li LY, Kim HJ, Park SA, et al. Genetic Profiles Associated with Chemoresistance in Patient-Derived Xenograft Models of Ovarian Cancer. Cancer Res Treat 2019;51:1117-27. [Crossref] [PubMed]
- Del Valle I, Buonocore F, Duncan AJ, et al. A genomic atlas of human adrenal and gonad development. Wellcome Open Res 2017;2:25. [Crossref] [PubMed]
- Jin X, Eroglu B, Cho W, et al. Inactivation of heat shock factor Hsf4 induces cellular senescence and suppresses tumorigenesis in vivo. Mol Cancer Res 2012;10:523-34. [Crossref] [PubMed]
- Yang Y, Jin L, Zhang J, et al. High HSF4 expression is an independent indicator of poor overall survival and recurrence free survival in patients with primary colorectal cancer. IUBMB Life 2017;69:956-61. [Crossref] [PubMed]
- Penrose HM, Heller S, Cable C, et al. In colonic ρ0 (rho0) cells reduced mitochondrial function mediates transcriptomic alterations associated with cancer. Oncoscience 2017;4:189-98. [Crossref] [PubMed]
- Chen C, Tong G, Zhang J, et al. Construction of prognostic hub gene network of esophageal adenocarcinoma by weighted gene co-expression network analysis. Zhejiang Medical Journal 2019;41:2181-4.
- Dorfman R, Taylor C, Lin F, et al. Modulatory effect of the SLC9A3 gene on susceptibility to infections and pulmonary function in children with cystic fibrosis. Pediatr Pulmonol 2011;46:385-92. [Crossref] [PubMed]
- Kang JU, Koo SH, Kwon KC, et al. Gain at chromosomal region 5p15.33, containing TERT, is the most frequent genetic event in early stages of non-small cell lung cancer. Cancer Genet Cytogenet 2008;182:1-11. [Crossref] [PubMed]
- Bai Y, Qu Y, Wu Z, et al. Absolute quantification and analysis of extracellular vesicle lncRNAs from the peripheral blood of patients with lung cancer based on multi-colour fluorescence chip-based digital PCR. Biosens Bioelectron 2019;142:111523. [Crossref] [PubMed]
- Su Z, Ji H. Relationship between mutations of SCNNl genes and respiratory diseases. International Journal of Respiration 2015;35:1105-8.
- Jin DH, Lee J, Kim KM, et al. Overexpression of MAPK15 in gastric cancer is associated with copy number gain and contributes to the stability of c-Jun. Oncotarget 2015;6:20190-203. [Crossref] [PubMed]
- Wu DD, Lau ATY, Yu FY, et al. Extracellular signal-regulated kinase 8-mediated NF-κB activation increases sensitivity of human lung cancer cells to arsenic trioxide. Oncotarget 2017;8:49144-55. [Crossref] [PubMed]
- Liao W, Zhao R, Lu L, et al. Overexpression of a novel osteopetrosis-related gene CCDC154 suppresses cell proliferation by inducing G2/M arrest. Cell Cycle 2012;11:3270-9. [Crossref] [PubMed]
- Visbal AL, Williams BA, Nichols FC, et al. Gender differences in non-small cell lung cancer survival: an analysis of 4,618 patients diagnosed between 1997 and 2002. Ann Thorac Surg 2004;78:209-15. [Crossref] [PubMed]
- Cerfolio RJ, Bryant AS, Scott E, et al. Women with pathologic stage I, II, and III non-small cell lung cancer have better survival than men. Chest 2006;130:1796-802. [Crossref] [PubMed]
- Ganti AK, Sahmoun AE, Panwalkar AW, et al. Hormone replacement therapy is associated with decreased survival in women with lung cancer. J Clin Oncol 2006;24:59-63. [Crossref] [PubMed]
- Stabile LP, Davis AL, Gubish CT, et al. Human non-small cell lung tumors and cell derived from normal lung express both estrogen receptor and show biological responses to estrogen. Cancer Res 2002;62:2141-50. [PubMed]
- Canver CC, Memoli VA, Vanderveer PL, et al. Sex hormonereceptors in non-small cell lung cancer in human beings. J Thorac Cardiovasc Surg 1994;108:153-57. [Crossref] [PubMed]
- Olak J, Colson Y. Gender differences in lung cancer: have we really come a long way, baby? J Thorac Cardiovasc Surg 2004;128:346-51. [Crossref] [PubMed]
- Stabile LP, Siegfried JM. Estrogen receptor pathways in lung cancer. Curr Oncol Rep 2004;6:259-67. [Crossref] [PubMed]
- Johnston EM, McIntyre LM, Hoff JA, et al. The effect of orchiectomy on lung cancer survival. Anticancer Res 1999;19:5567-70. [PubMed]
- Liu X, Wang S, Yan M, et al. Expressions of androgen and estrogen receptors in lung adenocarcinoma and squamous cell carcinoma. Jiangsu Med J 2010;36:1000-2.
- Maßberg D, Jovancevic N, Offermann A, et al. The activation of OR51E1 causes growth suppression of human prostate cancer cells. Oncotarget 2016;7:48231-49. [Crossref] [PubMed]
- Xiao D, He J. Epithelial mesenchymal transition and lung cancer. J Thorac Dis 2010;2:154-9. [PubMed]
- Kalluri R, Weinberg RA. The basics of epithelial-mesenchymal transition. J Clin Invest 2009;119:1420-8. [Crossref] [PubMed]
- Shi Y, Wu H, Zhang M, Ding L, Meng F, Fan X. Expression of the epithelial-mesenchymal transition-related proteins and their clinical significance in lung adenocarcinoma. Diagn Pathol 2013;8:89. [Crossref] [PubMed]
- Klymkowsky MW, Savagner P. Epithelial-mesenchymal transition: a cancer researcher's conceptual friend and foe. Am J Pathol 2009;174:1588-93. [Crossref] [PubMed]
- Liu J, Li Y, Zhu X, et al. Increased CX3CL1 mRNA expression level is a positive prognostic factor in patients with lung adenocarcinoma. Oncol Lett 2019;17:4877-90. [Crossref] [PubMed]
- Hiller TWR, O'Sullivan DE, Brenner DR, et al. Solar Ultraviolet Radiation and Breast Cancer Risk: A Systematic Review and Meta-Analysis. Environ Health Perspect 2020;128:16002. [Crossref] [PubMed]
- Bulavin DV, Saito S, Hollander MC, et al. Phosphorylation of human p53 by p38 kinase coordinates N-terminal phosphorylation and apoptosis in response to UV radiation. EMBO J 1999;18:6845-54. [Crossref] [PubMed]
- Kang KA, Piao MJ, Madduma Hewage SR, et al. Fisetin induces apoptosis and endoplasmic reticulum stress in human non-small cell lung cancer throuugh inhlbition of the MAPK signallng pathway. Tumour Biol 2016;37:9615-24. [Crossref] [PubMed]
- Bistrović A, Grbčić P, Harej A, et al. Small molecule purine and pseudopurine derivatives: synthesis, cytostatic evaluations and investigation of growth inhibitory effect in non-small cell lung cancer A549. J Enzyme Inhib Med Chem 2018;33:271-85. [Crossref] [PubMed]
(English Language Editor: C. Betlazar-Maseh)