A biomarker-based age, biomarkers, clinical history, sex (ABCS)-mortality risk score for patients with coronavirus disease 2019
Introduction
Coronavirus disease 2019 (COVID-19) caused by severe acute respiratory syndrome coronavirus 2 (SARS-CoV-2) is a newly recognized viral pneumonia that has been detected since December 2019 (1-4). Currently, COVID-19 is prevalent globally, and it is characterized as a pandemic by the World Health Organization (WHO). As of July 15, 2020, the total number of patients has risen sharply to 13,287,651 in 188 countries, areas or territories worldwide, with 577,954 (4.3%) deceased (5). There was a wide clinical spectrum of COVID-19, which ranged from mild to critically ill cases. Several studies have described the general epidemic characteristics, clinical manifestations, and clinical outcomes of patients with COVID-19 (4,6-13). Although severe and critical cases only accounted for about 19% of all patients (8), the mortality of critically ill cases was reported to be as high as 61.5% (6).
A surge of patients entering the hospital in a short period will result in the medical resources being overwhelmed, and it is therefore crucial to identify those potential patients with a high mortality risk, as such patients are most likely to benefit from immediate intensive care treatment. Previous studies have indicated that older age, comorbidities (e.g., cardiovascular disease, diabetes mellitus, or chronic respiratory disease), cardiac injury, elevated D-dimer and lactate dehydrogenase (LDH) concentrate, leukocytosis and lymphocytopenia were associated with adverse clinical outcomes of COVID-19 (2,6,10,13-18). However, the cumulative risk rendered by their combination on mortality has not been fully determined. In addition, the prognostic factors associated with COVID-19 are still uncertain. This study aimed to define the prognostic factors associated with mortality in hospitalized patients with COVID-19, and create a biomarker-based risk score for patients’ stratification and clinical decision-making. The clinical use was compared with the CURB-65 mortality risk score—a widely used severity score for community-acquired pneumonia (19). We present the following article in accordance with the TRIPOD reporting checklist (available at http://dx.doi.org/10.21037/atm-20-6205) (20).
Methods
Study design and participants
The derivation cohort was retrieved from Tongji Hospital of Huazhong University of Science and Technology (the largest teaching center in Hubei province), which is one of the designated hospitals for severely or critically ill COVID-19 cases. The study was conducted according to the principles of the Declaration of Helsinki (as revised in 2013). The study was approved by ethics board of Tongji Hospital and Hubei Xin Hua Hospital (NO.: TJ-IRB20200373) and informed consent was waived since the study was retrospectively designed and did not cause any harm to the patients. We retrospectively analyzed hospitalized adult patients (≥18 years old) from January 1, 2020, to April 10, 2020 (time of cut-off), who had been diagnosed with COVID-19 according to WHO interim guidance (21). A confirmed case of COVID-19 was defined as a positive result on reverse transcription-polymerase chain reaction (RT-PCR) of nasal and pharyngeal swab specimens. Only laboratory-confirmed patients were included in the final analysis.
An independent cohort of patients with the same clinical characteristics at Hubei Xinhua Hospital (another designated hospital for COVID-19) formed the external validation cohort, and it was also approved by the Ethics Commission of this institution.
Data collection
The patient list was filtered and derived from the His Medical System. We reviewed clinical electronic medical records, laboratory findings and radiological examinations for all laboratory confirmed COVID-19 cases. The admission data (demographic characteristics, major symptoms or signs) of these cases were documented. If the core records were missing or uncertain, requests for clarification were sent to involved health-care providers or their families. Age, sex, important chronic medical histories, key laboratory findings on admission, as well as living status up to the cut-off time were collected. Data were then entered into a computerized database for evaluation and cross-checking.
Statistical analysis
The endpoint for building this prediction model was death. Continuous variables were presented as median [interquartile range (IQR)], and were compared between survivors and non-survivors using Mann-Whitney U test or t-test as appropriate. Then, they were re-categorized into categorical variables using threshold values implicit in the existing severity assessment rules or clinical significance. Categorical variables were reported as whole numbers and proportions, and were compared by the χ2 test or Fisher exact test where appropriate.
Clinical and biomarker variables associated with death were assessed a-priori based on scientific knowledge, clinical importance, and risk factors identified in previously published articles (6,12,15,16,22-25). The significance of each variable in the derivation cohort was evaluated by univariate Cox regression analysis. A correlation matrix was used to evaluate the variables for collinearity, and plausible interaction terms were tested, including interactions between aspartate aminotransferase (AST)-alanine transaminase (ALT), creatinine-blood urea nitrogen, neutrophil-white blood cell and lymphocytes-white blood cell. Variables were excluded if they had high collinearity with global scores. CURB-65 was used as a comparison to our nomogram, and it was not involved in the model construction. Then, backward stepwise selection based on the Akaike information criterion (AIC) was applied to identify independent variables for developing the multivariable Cox proportional hazards regression model. Hazard ratios (HRs) and their 95% confidence intervals (CIs) were calculated (26). Selected variables were used to construct the nomogram to predict the probability of 30-day mortality on admission. For allocating points in the nomogram, the regression coefficients of each variable were applied to define the linear predictor (27).
The discriminating ability of the nomogram was evaluated using the Harrell’s concordance index (C-index) in the derivation and validation cohort, which estimates the probability of concordance between predicted and observed event rate in rank order (28). By using a bootstrapped sample (n=1,000) of the derivation and validation group, we plotted calibration curves to evaluate the accuracy of prediction of the nomogram.
The nomogram predicted probability of 30-day mortality for each patient were calculated according to the multivariable Cox model. Then, we plotted Kaplan-Meier curves of the tertiles of predictions in the derivation and validation cohort, and three prognostic categories were created (low, intermediate and high mortality risk group) based on the tertiles. Differences between the three groups were compared by the log-rank test. Clinical usefulness and net benefit were assessed with decision curve analysis (29).
Statistical analyses were conducted with RStudio version 1.1.419 (RStudio Inc.) and SPSS 20.0 software (SPSS Inc., Chicago, IL, USA). A two-sided P value less than 0.05 was considered statistically significant.
Results
Demographic and clinical characteristics
The derivation cohort consisted of 1,717 adult patients who had been confirmed as COVID-19 between January 1, 2020, and April 10, 2020. The external validation cohort consisted of 188 patients that had been confirmed as COVID-19 between January 13, 2020, and March 25, 2020. The median follow-up was 45 days (IQR, 35–98 days) for the derivation cohort, and 39 days (IQR, 31–87 days) for the validation cohort.
In the derivation cohort, the median age was 64 years (IQR, 53–71 years) and 50.9% (874 of 1,717) were male. The Kaplan-Meier estimated survival was 85.2% (95% CI, 83.0–87.4%) at 30 days. The in-hospital mortality was 11.7% (201 of 1,717), 67.2% (135 of 201) of the deceased patients were male and 68.7% (138 of 201) were older than 65 years. Of the 201 total deaths, 198 (98.5%) happened within 30 days. The median duration from admission to death was 10 days (IQR, 4.5–15 days). Baseline characteristics of patients who remained alive and who had died up to the time of analysis are compared in Table 1. The baseline clinical characteristics were similar between the derivation and validation cohort for most comparisons.
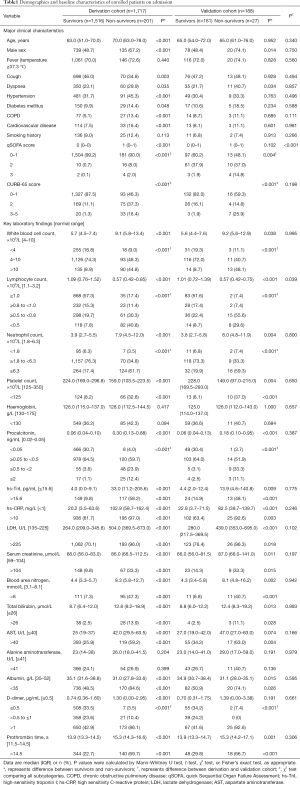
Full table
Predictors of mortality and model construction
The results of Cox regression analysis are presented in Table 2. Backward selection procedure based on the AIC identified the following ten variables that had the strongest association with death: age, sex, chronic obstructive pulmonary disease (COPD), AST, high-sensitivity C-reactive protein (hs-CRP), high-sensitive troponin I (hs-TnI), white blood cell count, lymphocyte count, D-dimer, and procalcitonin (all Wald tests =405.2, P<0.001). A nomogram for predicting 30-day mortality on admission was developed based on the 10 independent prognostic factors (Figure 1). The model was given the acronym age, biomarkers, COPD, sex (ABCS)-mortality score. The scoring system is shown in Table 3.
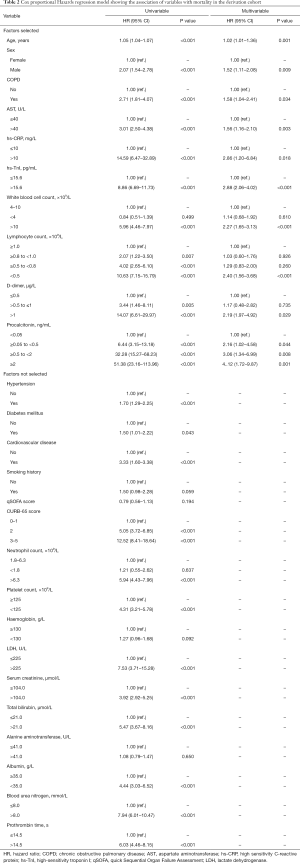
Full table
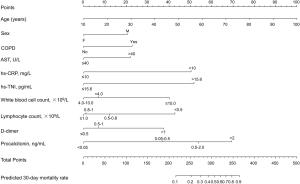
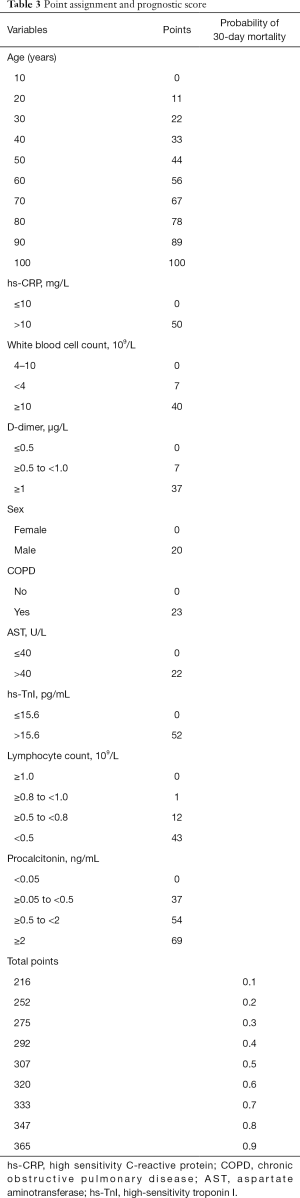
Full table
Model performance
The ABCS-mortality score showed good accuracy in estimating the risk of 30-day mortality in the derivation cohort, with a bootstrap-corrected C-index of 0.888 (95% CI, 0.869–0.907) (Table 4). In addition, calibration plots graphically demonstrated good agreement between the risk estimation by the prediction model and the Kaplan-Meier estimated 30-day mortality rate (Figure 2A). In the validation cohort, the model was also well calibrated (Figure 2B), achieving a C-index of 0.838 (95% CI, 0.777–0.899) for the prediction of 30-day mortality risk (Table 4). The CURB-65 score achieved a C-index of 0.696 (95% CI, 0.660–0.731) and 0.619 (95% CI, 0.519–0.720) in the derivation and validation cohort, respectively (Table 4).
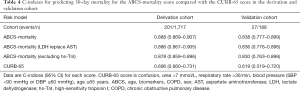
Full table
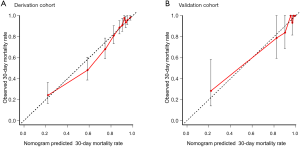
Clinical utility of the model
The cutoff value of the tertiles of the predictions was 2.0% and 9.0% based on the derivation cohort, and was applied to the validation cohort. Risk categories were created according to less than 2.0% (low mortality risk group), 2.0–9.0% (intermediate mortality risk group), and greater than 9.0% (high mortality risk group) risk for death within 30 days.
To further evaluate the discriminative ability of the model, the risk stratified Kaplan-Meier curves based on the risk categories were plotted for the derivation (Figure 3A) and validation cohort (Figure 3B). As shown, patients with the highest predicted 30-day mortality rate (>9%) had a substantially worse outcome compared with patients in the other two groups. Comparison of mortality frequencies within different risk categories for both the derivation (Figure 3C) and the validation cohort (Figure 3D) shows that the ABCS-mortality score had good discriminative ability in different subgroups of patients with COVID-19. The ABCS-mortality score displayed improved risk stratification within the range of the CURB-65 score risk classes (Figure 4), including patients with a low CURB-65 score of 0–1 point. By contrast, the CURB-65 score did not improve the risk stratification within the different ABCS-mortality risk groups (Figure S1).
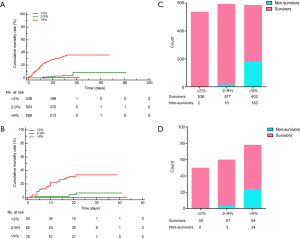
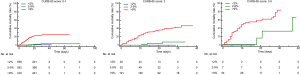
Finally, decision curve analysis was conducted to compare different prediction models. As seen in Figure 5, the decision curve analysis reveals the clinical usefulness of each model based on a continuum of potential thresholds for mortality risk (x axis) and the net benefit of applying the model to stratify patients (y axis). In this analysis, the ABCS-mortality risk score provided a larger net benefit across the range of mortality risk compared with the CURB-65 score.
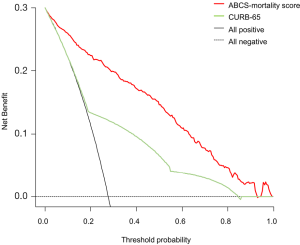
Considering differences between healthcare systems, additional models were created in the same manner using alternative biomarkers, i.e., replacing AST with LDH, or excluding the hs-TnI (for it might not be routinely tested in other hospitals). The ABCS-mortality score including LDH (instead of AST) provided similar results with C-index of 0.886 (95% CI, 0.867–0.905) in the derivation and 0.836 (95% CI, 0.776–0.896) in the external validation cohort (Table 4). The nomogram and calibration curves for this alternative ABCS-mortality model are shown in the Figure S2 and Figure S3, and the scoring system is shown in Table S1.
When excluded hs-TnI, while the other variables remained in the model (Figure S4), the ABCS-mortality score was slightly poorer than the model with hs-TnI, but still consistently outperformed the CURB-65 score (Table 4). The calibration curves for this alternative model is shown in Figure S5, and the scoring system is shown in Table S2.
Discussion
COVID-19 is spreading rapidly worldwide, and about 81% cases were classified as mild (non-pneumonia and mild pneumonia). However, it can also be deadly, for 14% cases were severe and presented as dyspnea, lung infiltrates and hypoxemia, and 5% progressed rapidly with acute respiratory distress syndrome, septic shock, multiorgan failure, and died finally (2,8). Therefore, early identification and a proper therapeutic strategy for cases with high mortality risk is of crucial importance to save lives.
CURB-65 is a widely used tool for severity assessment of community acquired pneumonia (19), but has not been developed in the setting of acute viral infection. A previously reported tool, the MuLBSTA scoring system, has been developed to predict mortality risk among patients with viral pneumonia, and it provided accurate estimates of patient prognosis (22). However, as a new coronavirus pneumonia, the intrinsic pathogenesis of SARS-CoV-2 caused pneumonia may differ significantly from other viral pneumonias, and a severity assessment model focusing on this specific subgroup of patients is urgently needed. Recently, a prediction model for assessing progression risk in patients with COVID-19 was proposed (18). However, we don’t know whether the identified risk factors were also associated with short-term mortality risk of COVID-19. What’s more, the sample size was relatively small and a validation dataset was absent. In this context, we developed and validated an ABCS-mortality score for predicting 30-day mortality risk in adult patients with COVID-19 on admission, and we hope that this model could be helpful for stratifying patients into different prognostic groups, and facilitate clinical decision-making.
The strength of this study lies in the focusing of statistical analyses of association on a-priori chosen predictors based on the current clinical evidence (2,6,8,13,15,19,22), which could avoid spurious associations. Although ten risk factors were eventually included in the model, it is an easy-to-use visual graphic tool for clinical application. Apart from demographic characteristics like age, sex and clinical history of COPD, the other biomarkers are routinely tested in many hospitals and are easily obtained. As shown in Figure 1, the model has incorporated important biomarkers that have been confirmed to provide incremental information about the risk of mortality in patients with COVID-19: AST, a marker of liver function; hs-TnI, indicating cardiac injury; white blood cell count and lymphocyte count, reflecting the status of immune system; D-dimer, a marker of coagulation disorders (4,6,7,15,24). Additionally, biomarkers reflecting inflammatory activity and bacterial infection such as hs-CRP and procalcitonin were also reliable indicators of adverse outcomes for COVID-19 (13). This information can be applied to inform the prognosis of the patients, and to make individualized decisions regarding the treatment and follow-up.
Accurate risk stratification is important because the prognosis of the COVID-19 is heterogeneous. Since no specific treatment (such as targeted antiviral drugs) is available for COVID-19 currently, the mainstay of treatment has been supportive care. For non-severe patients, isolation and close follow-up might be sufficient to manage this disease (1,2). However, for potential critical patients, aggressive therapeutic strategies or intensive care are needed. Thus, stratifying patients into different prognostic groups on admission is crucial for rational medical resources allocation and management selection.
For clinical use of the ABCS-mortality score, patients were stratified into different mortality risk groups based on the tertiles of predicted 30-day mortality. This model showed good discriminative ability in distinct groups of patients who were at different risk of mortality. It also consistently predicted 30-day mortality with a higher accuracy than the CURB-65 risk score and provided better clinical usefulness throughout the range of mortality risk as assessed by the decision curve analysis. The ABCS-mortality score could enable patients to be categorized into suitable groups for three distinct management options: patients at low risk of mortality (<2.0%) might be suitable for isolation at temporary health clinics (such as Fang Cang Shelter in China) and close medical surveillance; patients at intermediate risk of mortality (2.0–9.0%) should be considered for supervised treatment at designated hospital; for patients at high risk of mortality on admission (>9.0% or even higher), initial care in an intensive care unit may be appropriate.
Limitations of this study should be acknowledged. First, the external validation was only performed in a small Chinese dataset, validation in larger, international datasets is needed to further improve the generalizability and robustness of this model. Second, owing to differences between healthcare systems in managing patients with COVID-19, this model will need to be adjusted to the local setting, even though two alternative models have been proposed in the current study. Third, the patients who were asymptomatic or had mild symptoms that were managed at home or isolation sites were not included, so our study cohort may represent a more severe subgroup of COVID-19 cases. Furthermore, during the onset of the pandemic, several drugs (hydroxychloroquine, azithromycin, lopinavir/ritonavir, dexamethasone, remdesivir etc.) had been proposed that might be useful to improve the prognosis of COVID-19. The use of any of these drugs might change the predictions of the proposed model and lessen its applicability. However, since none treatment standards (dosage and timing of administration) were available about these drugs, it was hard to evaluate the effect they had on mortality. More high-level evidence based on randomized controlled trials are needed to prove their effectiveness. Finally, objective parameters on lung function at disease presentation (such as oxygen saturation percentage, PaO2/FiO2, SaO2/FiO2, oxygen requirements) were not included in the model due to missing data in the His Medical System. In addition, some potential mortality predictors identified in previous literatures (such as hypertension, diabetes, and chronic cardiovascular diseases) were linked to mortality by univariate Cox regression analysis in the current study (P<0.05 for all), but were excluded during the backward stepwise selection procedure due to relatively low prediction power. Other variables like obesity and chronic kidney diseases were also not involved in the analysis due to incomplete information. Therefore, the choice of potential prognostic factors could not be entirely informed by a systematic review of previous literatures.
In conclusion, this study offers a simple prediction model based on the clinical features of age, sex, clinical history of COPD, as well as seven biomarkers as a practical tool of stratifying COVID-19 patients into distinct prognostic groups. The ABCS-mortality score performed well on the derivation and independent external validation cohort. This tool can be offered to clinicians to improve their ability to predict patients’ prognosis on admission, and strengthen the prognosis-based clinical decision making, which might be helpful for rational allocation of scarce medical resources and reducing mortality of COVID-19 patients.
Acknowledgments
Funding: This study is supported by the youth medical talent support program of Hubei Province.
Footnote
Reporting Checklist: The authors have completed the TRIPOD reporting checklist. Available at http://dx.doi.org/10.21037/atm-20-6205
Data Sharing Statement: Available at http://dx.doi.org/10.21037/atm-20-6205
Conflicts of Interest: All authors have completed the ICMJE uniform disclosure form (available at http://dx.doi.org/10.21037/atm-20-6205). The authors have no conflicts of interest to declare.
Ethical Statement: The authors are accountable for all aspects of the work in ensuring that questions related to the accuracy or integrity of any part of the work are appropriately investigated and resolved. The study was conducted according to the principles of the Declaration of Helsinki (as revised in 2013). The study was approved by Ethics Board of Tongji Hospital and Hubei Xin Hua Hospital (No.: TJ-IRB20200373) and informed consent was waived since the study was retrospectively designed and did not cause any harm to the patients.
Open Access Statement: This is an Open Access article distributed in accordance with the Creative Commons Attribution-NonCommercial-NoDerivs 4.0 International License (CC BY-NC-ND 4.0), which permits the non-commercial replication and distribution of the article with the strict proviso that no changes or edits are made and the original work is properly cited (including links to both the formal publication through the relevant DOI and the license). See: https://creativecommons.org/licenses/by-nc-nd/4.0/.
References
- Huang C, Wang Y, Li X, et al. Clinical features of patients infected with 2019 novel coronavirus in Wuhan, China. Lancet 2020;395:497-506. [Crossref] [PubMed]
- Chen N, Zhou M, Dong X, et al. Epidemiological and clinical characteristics of 99 cases of 2019 novel coronavirus pneumonia in Wuhan, China: a descriptive study. Lancet 2020;395:507-13. [Crossref] [PubMed]
- Zhu N, Zhang D, Wang W, et al. A Novel Coronavirus from Patients with Pneumonia in China, 2019. New Engl J Med 2020;382:727-33. [Crossref] [PubMed]
- Guan WJ, Ni ZY, Hu Y, et al. Clinical Characteristics of Coronavirus Disease 2019 in China. New Engl J Med 2020;382:1708-20. [Crossref] [PubMed]
- Available online: https://gisanddata.maps.arcgis.com/apps/opsdashboard/index.html#/bda7594740fd40299423467b48e9ecf6
- Yang X, Yu Y, Xu J, et al. Clinical course and outcomes of critically ill patients with SARS-CoV-2 pneumonia in Wuhan, China: a single-centered, retrospective, observational study. Lancet Respir Med 2020;8:475-81. [Crossref] [PubMed]
- Wang D, Hu B, Hu C, et al. Clinical Characteristics of 138 Hospitalized Patients With 2019 Novel Coronavirus–Infected Pneumonia in Wuhan, China. JAMA 2020;323:1061-9. [Crossref] [PubMed]
- Wu Z, McGoogan JM. Characteristics of and Important Lessons From the Coronavirus Disease 2019 (COVID-19) Outbreak in China: Summary of a Report of 72 314 Cases From the Chinese Center for Disease Control and Prevention. JAMA 2020;323:1239-42. [Crossref] [PubMed]
- Chen H, Guo J, Wang C, et al. Clinical characteristics and intrauterine vertical transmission potential of COVID-19 infection in nine pregnant women: a retrospective review of medical records. Lancet 2020;395:809-15. [Crossref] [PubMed]
- Chan JF, Yuan S, Kok K, et al. A familial cluster of pneumonia associated with the 2019 novel coronavirus indicating person-to-person transmission: a study of a family cluster. Lancet 2020;395:514-23. [Crossref] [PubMed]
- Wu JT, Leung K, Leung GM. Nowcasting and forecasting the potential domestic and international spread of the 2019-nCoV outbreak originating in Wuhan, China: a modelling study. Lancet 2020;395:689-97. [Crossref] [PubMed]
- Xu XW, Wu XX, Jiang XG, et al. Clinical findings in a group of patients infected with the 2019 novel coronavirus (SARS-Cov-2) outside of Wuhan, China: retrospective case series. BMJ 2020;368:m606. [Crossref] [PubMed]
- Chen T, Wu D, Chen H, et al. Clinical characteristics of 113 deceased patients with coronavirus disease 2019: retrospective study. BMJ 2020;368:m1091. [Crossref] [PubMed]
- Zhang J, Zhou L, Yang Y, et al. Therapeutic and triage strategies for 2019 novel coronavirus disease in fever clinics. Lancet Respir Med 2020;8:e11-e12. [Crossref] [PubMed]
- Zhou F, Yu T, Du R, et al. Clinical course and risk factors for mortality of adult inpatients with COVID-19 in Wuhan, China: a retrospective cohort study. Lancet 2020;395:1054-62. [Crossref] [PubMed]
- Shi S, Qin M, Shen B, et al. Association of Cardiac Injury With Mortality in Hospitalized Patients With COVID-19 in Wuhan, China. JAMA Cardiol 2020;5:802-10. [Crossref] [PubMed]
- Inciardi RM, Lupi L, Zaccone G, et al. Cardiac Involvement in a Patient With Coronavirus Disease 2019 (COVID-19). JAMA Cardiol 2020;5:819-24. [Crossref] [PubMed]
- Ji D, Zhang D, Xu J, et al. Prediction for Progression Risk in Patients with COVID-19 Pneumonia: the CALL Score. Clin Infect Dis 2020;71:1393-9. [Crossref] [PubMed]
- Lim WS, van der Eerden MM, Laing R, et al. Defining community acquired pneumonia severity on presentation to hospital: an international derivation and validation study. Thorax 2003;58:377-82. [Crossref] [PubMed]
- Moons KGM, Altman DG, Reitsma JB, et al. Transparent Reporting of a multivariable prediction model for Individual Prognosis or Diagnosis (TRIPOD): explanation and elaboration. Ann Intern Med 2015;162:W1-73. [Crossref] [PubMed]
- World Health Organization. Clinical management of severe acute respiratory infection when novel coronavirus (2019-nCoV) infection is suspected: interim guidance. January 28, 2020 Available online: https://www.who.int/docs/default-source/coronaviruse/clinical-management-of-novel-cov.pdf
- Guo L, Wei D, Zhang X, et al. Clinical Features Predicting Mortality Risk in Patients With Viral Pneumonia: The MuLBSTA Score. Front Microbiol 2019;10:2752. [Crossref] [PubMed]
- Uranga A, Quintana JM, Aguirre U, et al. Predicting 1-year mortality after hospitalization for community-acquired pneumonia. PLoS One 2018;13:e0192750. [Crossref] [PubMed]
- Ruan Q, Yang K, Wang W, et al. Clinical predictors of mortality due to COVID-19 based on an analysis of data of 150 patients from Wuhan, China. Intensive Care Med 2020;46:1294-7. [Crossref] [PubMed]
- Wynants L, Van Calster B, Bonten MMJ, et al. Prediction models for diagnosis and prognosis of covid-19 infection: systematic review and critical appraisal. BMJ 2020;369:m1328. [Crossref] [PubMed]
- Simon R. Confidence intervals for reporting results of clinical trials. Ann Intern Med 1986;105:429-35. [Crossref] [PubMed]
- Shariat SF, Karakiewicz PI, Suardi N, et al. Comparison of Nomograms With Other Methods for Predicting Outcomes in Prostate Cancer: A Critical Analysis of the Literature. Clin Cancer Res 2008;14:4400-7. [Crossref] [PubMed]
- Harrell FE Jr, Califf RM, Pryor DB, et al. Evaluating the yield of medical tests. JAMA 1982;247:2543-6. [Crossref] [PubMed]
- Vickers AJ, Cronin AM, Elkin EB, et al. Extensions to decision curve analysis, a novel method for evaluating diagnostic tests, prediction models and molecular markers. BMC Med Inform Decis Mak 2008;8:53. [Crossref] [PubMed]