Profiles of alternative splicing landscape in breast cancer and their clinical significance: an integrative analysis based on large-sequencing data
Introduction
Breast cancer (BRCA) is the most common malignancy and the predominant cause of cancer-associated death in women. BRCA is a heterogeneous tumor, with multiple molecular subtypes and distinct survival outcomes. Research illustrates that whilst the 5-year overall survival (OS) rate of BRCA is 91%, once distant metastasis occurs, the rate drops to 40.7% (1,2). At present, reducing the mortality of BRCA, especially advanced BRCA with distant metastasis, is an urgent challenge to be solved.
In the past few decades, scientists have made intensive efforts to determine the underlying molecular mechanisms of BRCA, identify its prognostic indicators and explore potential therapeutic strategies. This research has gradually revealed that the aberrant expression of key genes (3), mutations (4), copy number variations (5), and post-transcriptional modifications (6) (methylation, acetylation, etc.) participate in regulating the occurrence and progression of BRCA. Moreover, the molecular subtypes of BRCA have been constructed and therapeutic drugs developed (7). Although previous studies have greatly improved the survival time and quality of life in BRCA patients, there remains a need to decrypt this heterogeneous disease from a relatively new perspective.
As a post-transcriptional editing process, alternative splicing (AS) occurs in almost 95% of transcribed human genes. AS is a process that removes introns and concatenate specific exons which enrich the biodiversity of genes (8). Splicing variants generated from the same gene can produce distinct and even opposite effects. However, intensive evidence has confirmed that the abnormal expression of AS isoforms mediates oncogenesis and progression in various cancers and some experts have advanced the view that aberrant AS could be regarded as a novel hallmark of cancer (9). With the advancement of high-throughput sequencing, the correlation between specific AS events and the hallmarks of cancer including proliferation (10), anti-apoptosis (11), metastasis (12), angiogenesis (13), and metabolism (14) has been gradually identified. Moreover, research establishing AS-related prognostic factors has highlighted that aberrant AS not only contributes to recognize the pathogenesis of BRCA, but also facilitates the clinical diagnosis and development of therapeutic targets (15).
AS are finely regulated by a complex machine called a spliceosome, which is composed of five small nuclear RNA ribonucleoproteins (snRNPs) and more than 100 trans-acting factors including the well-known splicing factors (SFs): heterogeneous nuclear ribonucleoproteins (hnRNPs) and Ser/Arg-rich (SR) protein families. As trans-acting factors, SFs specifically recognize and combine with cis-regulatory sequences in pre-RNA to mediate splicing site selection and spliceosome assembly. Abnormal AS events are partially orchestrated by the aberrant regulation of SFs. In addition, it is well accepted that the methylation status of promoter and genetic copy number variants (CNV), influence expression levels of SFs (16,17). Consequently, it is meaningful to explore the potential correlation between SFs and the corresponding methylation/CNV status in BRCA.
The systematic profile of mRNA AS has been depicted in colorectal cancer (18), ovarian cancer (19), non-small cell lung cancer (20), et al. Several studies have focused on the prognostic value of AS in BRCA and triple-negative breast cancer (TNBC). However, the exploration of regulatory network, biological functions and clinical significance of AS events, is still lacking. In this study we profiled a relatively comprehensive analysis of AS events in BRCA from the TCGA dataset and identified BRCA-related differentially expressed AS (DEAS) events. Firstly, we analyzed the underlying biological function and potential regulatory mechanisms of DEAS events. Among them, we found 19 AS events that were differentially expressed in various cancers, suggesting that these AS events might have a broad role in regulating the initiation and development of cancer. Moreover, the combination analysis with clinical information shed light on the prognostic value of DEAS. Finally, we stratified BRCA patients into two groups based on DEAS-related AS events and explored the correlation between risk groups and clinicopathological features as well as cancer phenotypes. Overall, our analysis has created a relatively new perspective to understanding the cancer, biological, and clinical significance of AS events in BRCA.
We present the following article in accordance with the MDAR reporting checklist (available at http://dx.doi.org/10.21037/atm-20-7203).
Methods
Data acquisition and preparation process
The RNA-Seq data (Normal n=113; Tumor n=1,094) and clinical information (n=1,097) of BRCA patients were obtained from the TCGA dataset (https://tcga-data.nci.nih.gov/tcga/). Patients (n=152) who did not accord with any of the following standards were excluded: (I) definite histological diagnosis; (II) explicit T/N/stage/ER/PR/molecular subtype/OS/DFS information; (III) more than one month survival time after diagnosis. The cBioPortal for TCGA database (http://www.cbioportal.org/) was used to acquire the copy number variation (CNV) and methylation information (21). We referenced the data of DEAS events in colorectal cancer (CRC) or head and neck squamous cell carcinoma (HNSC) obtained from previous studies (22,23). Data of BRCA molecular subtypes identified by using PAM50, proliferation, and wound-healing value were referenced from Thorsson et al. (24).
The mRNA SpliceSeq data was downloaded for each BRCA patient by using the TCGA SpliceSeq tool (https://bioinformatics.mdanderson.org/TCGASpliceSeq/). The Percent-Spliced-In (PSI) index was utilized to quantify seven types of AS events. To obtain more credible AS events, a series of strict filters (percentage of Samples with PSI value ≥75, average of PSI value ≥0.05) were implemented resulting in the identification of 35,367 AS events from 10,274 genes. Interactive sets among seven types of AS were depicted by Upset plot generated by using UpSetR package (version 1.3.3) (25). The flowchart illustrates the design and implementation process of the study (Figure 1).
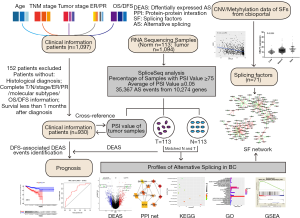
Identification and validation of DEAS events and functional analysis
The study was conducted in accordance with the Declaration of Helsinki (as revised in 2013) and was approved by the institutional review board of Zhongshan Hospital, Fudan University (approval number: B2020-108R). All written informed consent was obtained.
To recognize DEAS events in BRCA, the PSI value of AS events were compared between paired normal and tumor tissues (n=113) using the “limma” package. Benjamini & Hochberg (BH) correction was used to adjust P values. |log2FC| ≥0.5, (also equivalent to |logFC| ≥0.15) and adj.P<0.05 was considered as the criterion of DEAS events. Volcano plot and heat map were performed to visualize the profiling of DEAS events identified in BRCA. A Venn diagram was performed to demonstrate the intersection set of DEAS and differentially expressed genes (DEG) by using the “VennDiagram” package (26). Representative DEAS events between tumor and paired normal tissues were visualized by “ggplot2” package. 6 pairs of BRCA and normal tissues were obtained from patients underwent surgery at Zhongshan Hospital after accessing the written informed consent, then stored at 80 °C for RT-PCR assay. RNA extraction, reverse-transcription were performed. RT-PCR were performed by using the AceTaq Master Mix (P412-01) from Vazyme for amplification. Primers for RT-PCR were listed in Table S1. The products of RT-PCR were visualized and semi-quantified by nucleic acid electrophoresis and software Image J.
The parent genes of DEAS then underwent GO and KEGG pathways enrichment analyses by using the “clusterProfiler” package (version 3.4.4) (27). Genes were annotated from the aspects of molecular functions (MF), biological processes (BP), and cellular components (CC) of biology in the GO database. Gene set enrichment analysis (GSEA) was conducted to verify the enrichment results identified by GO or KEGG analysis. The correlation between DEAS and wound-healing values was explored by using spearman correlation analysis. In addition to this, the parent genes of the DEAS events were imported to the Retrieval of Interacting Genes/Proteins (STRING, version 11.0) to build a protein-protein interaction (PPI) network (combined score>0.7), which was then depicted by Cytoscape (version 3.4.0) (28,29). Cytohubba and MCODE in Cytoscope were used to identify hub genes. ClueGO and CluePedia in Cytoscape were used to visualize KEGG enrichment results of the proteins participating in PPI network.
Establishment of correlation network between SFs and DEAS events
The list of SFs was referenced from the SpliceAid-F database (30). The 71 experimentally validated SFs were composed of 13 SR proteins, 27 hnRNPs and other families including ELAV, KHDRBS, CELF, Nova, and Fox families. We then constructed the correlation network between the expression of SFs and the PSI value of DEAS events by conducting Spearman’s rank (non-normal distribution) and Pearson’s rank (normal distribution data) correlation analysis. BH correction was used to adjust p value (|R|>0.5, adj.P<0.05). The correlation plots were visualized by Cytoscape and the correlation analysis between CNV/methylation and mRNA expression of SFs was performed.
Construction of DEAS-related risk signature and survival analysis
Patients (n=930) with integrated clinical and AS data were included in further survival analysis. Univariate Cox regression was used to analyze the prognostic correlation between DEAS events and DFS. A penalized Cox regression model with LASSO penalty was then performed to construct a DEAS-related prognosis signature, and 10-time cross-validations were used to determine the optimal lambda (31). The risk score for each BRCA patient was calculated by using the PSI value of the prognostic AS events identified by the DEAS-related risk signature and its corresponding coefficient. Patients were stratified into low- and high-risk cohorts based on the risk score. A Kaplan-Meier analysis and log-rank test was then conducted to compare the DFS in different groups. The prognostic value of the DEAS-related risk signature was assessed by the ROC curves and area under curve (AUC) values (32).
Evaluation of the association between risk groups and clinical/molecular characteristics
The association between risk groups and clinicopathological features (age, histology, T/N/clinical stage, ER/PR status and molecular subtypes), survival status (DFS), and hallmarks of cancer, (proliferation, wound healing) were analyzed and visualized.
Statistical analysis
R software (version 3.5.1) was utilized to perform statistical analysis and P value <0.05 represented statistical significance. Student’s t-test was conducted for comparison of continuous variables and Pearson’s chi-square test was used to compare categorical variables. The correlation was evaluated by Spearman’s rank (non-normal distribution) and Pearson’s rank (continuous variables meeting normal distribution) correlation analysis.
Results
Profiles of AS events in BRCA TCGA cohort
The clinical information of 930 BRCA patients fitting the inclusion criteria were obtained and their baseline characteristics listed in Table S2. With a 33.1 months median follow-up time (range, 1–286.8 months), 96 (10.3%) of patients experienced recurrence and 119 (12.8%) patients died.
The corresponding RNA-Seq data were downloaded to construct the profile of AS events. Figure 2A depicts seven modes of AS: alternate acceptor site (AA), alternate donor site (AD), alternate promoter (AP), alternate terminator (AT), exon skip (ES), mutually exclusive exons (ME), and retained intron (RI). Considering a large component of AS events were discovered in only a few samples and some AS expression levels were very low (PSI value <0.05), we performed strict filters to ensure the credibility and accuracy of the analysis. After filtering, 35,367 AS events from 10274 genes were identified (https://cdn.amegroups.cn/static/public/10.21037atm-20-7203-1.xlsx). The details of the number of each type of AS and corresponding genes are illustrated in Figure 2B. The most common AS type was ES (42.2%), followed by AP (17.8%) and AT (17.0%). Upset plots showed that most genes contain more than one AS modes (Figure 2C).
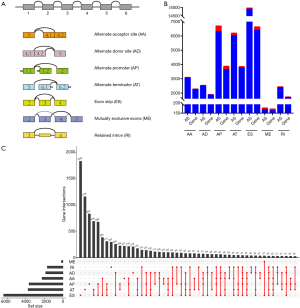
Identification and validation of DEAS events in BRCA patients
The PSI value of 113 tumor and paired normal tissues were compared to obtain the list of DEAS events in BRCA patients. Based on the threshold of |log2FC| >0.5 and adj.P value <0.05 (t-test and BH correction), a total of 973 DEAS events from 621 genes were screened (https://cdn.amegroups.cn/static/public/10.21037atm-20-7203-2.xlsx), including 382 APs, 311 ESs, 177 ATs, 43 RIs, 26 AAs, 25 ADs, and 9 MEs. Among them, DEAS events with |log2FC| >1.5 were represented by green dots in the volcano map, including 5 AS events upregulated in tumor (NTM-19519-AP, ISLR-31876-AP, KIF4A-89373-AT, LSP1-13865-AP and TNC-87357-ES, the number in the middle represented by the code of the AS events), and 4 AS events downregulated in tumor (FBLN2—63511-ES, NTM-19520-AP, KIF4A-898372-AT and ISLR-31677-AP) (Figure 3A). Then ES of exon 13 in SLK, exon 10 in STX2, exon 11 in CLSTN1 and exon 3 in NFYA were validated in 6 pairs of BRCA and normal tissues (Figure S1A). The PSI were presented under the gels. Then paired t-test results confirmed that the ES of SLK and CLSTN1 were significantly altered in paired samples. Besides, there were an alteration trend of PSI of STX2 exon 10 and NFYA exon 3 in BRCA and paired normal tissues (Figure S1B). These results verified the reliability of the analysis based on TCGA dataset. The tumor and tissue samples were clearly divided into discrete groups by using unsupervised hierarchical clustering methods according to DEAS events (Figure 3B). More intriguingly, by referring to previous studies, we found that 19 AS events were differentially expressed not only in BRCA, but also in HNSC and CRC. The AT of exon 2 in FAM72A, for example, was downregulated, while the AP of exon 2 in PODNL1 was upregulated (https://cdn.amegroups.cn/static/public/10.21037atm-20-7203-3.xlsx). These events revealed common AS events in different tumorigenesis processes.
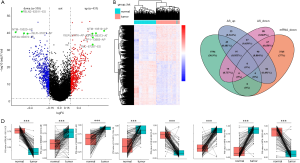
Considering AS events can directly affect gene expression levels through manners such as nonsense-mediated mRNA decay (NMD), we explored the intersection set of DEAS and DEG (Figure 3C). The details are summarized in https://cdn.amegroups.cn/static/public/10.21037atm-20-7203-3.xlsx. Among 428 AS events accompanied by changes in the RNA expression levels of the corresponding genes, 88.1% were accounted by three types of AS events, ES (157, 36.7%), AP (155, 36.2%), and AT (65, 15.2%). This indicated that AS events may be involved in regulating tumorigenesis and progression partly by changing gene expression. Representative DEAS events between tumor and paired normal tissues are illustrated in Figure 3D.
Functional enrichment analysis of DEAS
We next conducted functional enrichment analysis based on the corresponding parent genes of DEAS. GO enrichment analysis revealed that EDAS-related genes mainly enriched in metastasis-associated categories, such as regulation of cell adhesion (FDR =7.59E-05), cytoskeleton organization (FDR =0.034), extracellular matrix organization (FDR=0.038), and focal adhesion (FDR =5.58E-07) (Figure 4A, https://cdn.amegroups.cn/static/public/10.21037atm-20-7203-4.xlsx). Moreover, KEGG pathways related to cell movement had the trend of enrichment (Figure 4B, https://cdn.amegroups.cn/static/public/10.21037atm-20-7203-4.xlsx), such as ECM-receptor interaction (P=0.0019), regulation of actin cytoskeleton (P=0.0031), AMPK signaling pathway (P=0.0062), and adherens junction (P=0.0076). Partially consistent with the above findings, GSEA analysis showed that DEAS were enriched in cell adhesion (NES =1.8, FDR =0.017), natural killer cell mediated cytotoxicity (NES =1.58, FDR =0.083), pathways in cancer (NES =1.49, FDR =0.115), and PPAR signaling pathway (NES =1.68, FDR =0.055) (Figure 4C, https://cdn.amegroups.cn/static/public/10.21037atm-20-7203-4.xlsx). Collectively, this indicated that DEAS events were likely to participate in the remodeling of cancer cell movement and adhesion ability, and then affected cell invasion and migration. To confirm the inference, correlation analysis between PSI values of DEAS events and wound-healing values referenced from previous studies was conducted. Interestingly, some distinct AS events derived from a single gene were seen to exert opposite strong correlation with wound-healing values. The AT of exon 29 in KIF4A, for example, was negatively (R=0.79, P<2.2e-16), while the AT of exon 32 in KIF4A was positively (R=0.79, P<2.2e-16) associated with those values (Figure 4D).
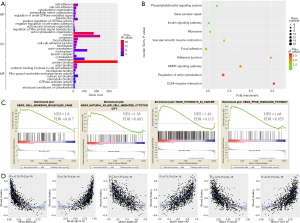
AS could directly affect protein function by changing the length of the transcript, regulating the level of protein expression, and changing the inclusion status of functional domains. Hence, it is meaningful to explore the AS events in BRCA from the perspective of the corresponding PPI network of DEAS events. The PPI network of DEAS profiled interactions in normal condition and disclosed the potential impact of DEAS at the protein level (Figure 5A). We further analyzed the ten hub genes (Figure 5B) and enrichment pathways (Figure 5C) based on the PPI network. Interrelated proteins were significantly enriched in adherens junction, regulation of actin cytoskeleton, ECM-receptor interaction, salmonella infection, and AMPK signaling pathway.
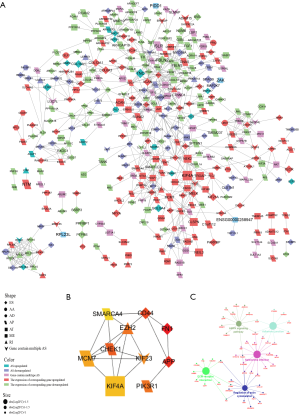
Collectively, the results indicated that AS events dysregulation played an important role in BRCA and were closely related with the regulation of the motility, invasion, and migration of cancer cells.
Construction of correlation network between DEAS events and SFs
As trans-acting factors, SFs are the key components of the spliceosome, participating in AS through binding to pre-RNA to regulate splicing site selection. This means DEASs may be driven by several key SFs. On this basis, we built a significant correlation network between the expression of 71 SFs identified by referring to previous studies and the PSI values of DEASs (Figure 6, https://cdn.amegroups.cn/static/public/10.21037atm-20-7203-5-1.xlsx). The representative plots between SFs and AS events are illustrated in Figure 6B. Significant correlation was found between 108 AS events and 18 SFs. Most SFs were associated with several AS events and a specific AS event could be significantly correlated with six SFs. This may partially reveal the reason why a few SFs widely regulated the AS of many pre-RNAs.
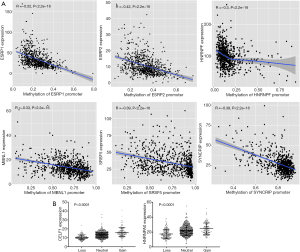
Following this, we further explored the possible reasons changes occurred in the expression levels of SFs. Correlation analysis indicated that among the 51 SFs, the expression levels of seven SFs were significantly negatively correlated with the methylation levels of their promoter regions (https://cdn.amegroups.cn/static/public/10.21037atm-20-7203-5-2.xlsx) (|R|>0.3, adj.P value <0.05). The representative correlation relationship of ESRP1, ESRP2, hnRNP F, MBNL1, SRSF5, and SYNCRIP, is seen in Figure 7. The expression levels of SFs significantly correlated with CNV status counted for 92.4% (Figure 7B, https://cdn.amegroups.cn/static/public/10.21037atm-20-7203-5-3.xlsx). This indicated that genetic and epigenetic dysregulation is involved in the modulation of AS by changing the expression levels of SFs.
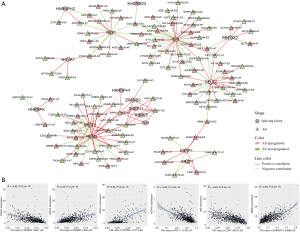
Identification of DFS related DEAS events and generation of the DEAS-related risk signature
Based on the previous enrichment analysis results that DEAS events would be closely related to biological functions such as cell movement and migration, we investigated the relationship between DEAS events and DFS in a BRCA TCGA cohort. The median PSI value of each DEAS were utilized to divide patients into two groups, then univariate Cox regression analyses for DFS were performed. Consequently, 103 AS with a prognostic value of DFS were identified (https://cdn.amegroups.cn/static/public/10.21037atm-20-7203-6.xlsx), among which APs accounted for 43.7%.
To establish a practical and precise DEAS-related risk signature, a LASSO Cox model determined by the optimal lambda was constructed (Figure 8A). Consequently, eight AS events were involved in the prognostic signature (Figure 8B), among which, UGT1A1-58054-AT, EPB41L1-59273-ES, CYP46A1-29229-AT, USHBP1-48246-AA, and DST-76557-AT related to poor DFS, whereas UBE2E1-63715-AP, TOM1L1-42558-ES, and CHTOP-91133-ES related to improved DFS. The risk score of each patient was identified based on the coefficients of these AS events in the LASSO Cox model and the PSI values. Patients were then separated into high- and low-risk groups according to the median of the risk score (Figure 8C). Patients with an increasing risk score tended to possess shorter DFS time (Figure 8D). The heat map depicts the PSI values of these AS events in high- and low-risk groups (Figure 8E).
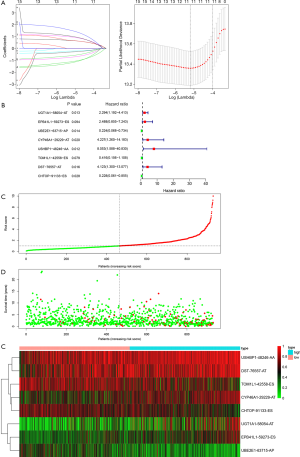
Prognostic value of DEAS-related risk signature in BRCA
Kaplan-Meier and log-rank tests revealed that patients in high-risk groups were correlated with a better prognosis in DFS (P=6.956e-10, Figure 9A). The AUC of the DEAS-related risk signature was 0.754 (Figure 9B). These results indicated that the DEAS-related prognostic signature would be a reliable predictor for DFS of BRCA patients.
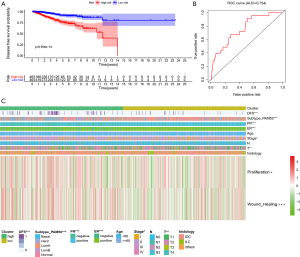
Risk groups associated with prognosis, clinicopathological features and hallmarks of cancer
In addition to exploring AS events at the molecular level, we also wished to determine whether risk groups were associated with the prognosis, clinicopathological features and hallmarks of cancer (proliferation and wound-healing value) (Figure 9C). This revealed the distribution of different molecular subtypes, T stage, clinical stage, ER/PR status, survival status (DFS), and cancer features (proliferation and wound healing value) in BRCA samples between risk groups, were not random. Patients in the high-risk group, for example, were seen to have enriched basel-like molecular subtype, negative ER/PR status, advanced clinical stages, and proliferation and metastasis potency. These results suggested that grouping based on DEAS-related risk signature can not only be used as a prognostic indicator of DFS but can also guide the estimation of tumor malignancy and molecular targeted strategies.
Discussion
BRCA is a heterogeneous disease with distinct biological features. Extensive progress in the individualized treatment and survival outcomes of BRCA patients has been made as a result of research at the molecular level, such as that proposing molecular subtypes. However, its high incidence and diverse therapeutic responses indicate that research is urgently required to decipher the complex biological process of BRCA from a new perspective. Recently, AS data from the TCGA database has been used to interpret the complex biological functions of cancer, and predictive signatures based on AS events have been constructed in various cancers (18-20,33-36). There is increasing evidence to show that individual AS events promote the progression of BRCA. For instance, Mcl-1, participating in the control of intrinsic cell death pathway, possesses two splice isoforms, Mcl-1L (anti-apoptosis) and Mcl-1s (pro-apoptosis). The splice switching of Mcl-1 is regulated by hnRNP F, H1 and K in BRCA cells, which mediates the tumorigenesis of BRCA (37). Besides, SF ESRP1 regulates the CD44 splice switching from CD44 variants splice isoforms (CD44v) to CD44 standard splice isoform (CD44s), which enhances BRCA stem cell properties (38). Furthermore, SF PCBP1 binds to the exonic splicing suppressor (ESS) region in the exon 3 of STAT3, then facilitating the switch from STAT3α (pro-tumor) to STAT3β (anti-tumor) isoforms (39). However, there are only a few studies focusing on the systematical AS regulation network in a large-scale BRCA cohort. In this study, we analyzed and constructed the landscape of AS in BRCA based on the TCGA database.
Abnormal AS events regulate many aspects of cancer, including proliferation (40), apoptosis (11), migration (12), angiogenesis (13), and metabolism (14). In view of its vital role in cancer, some experts regard AS as one of the hallmarks of cancer (9). Although abnormal AS have been widely explored in the past few decades, due to the limitations of database platforms, sequencing technologies, and analytical methods, research on AS events is relatively rare. In recent years, studies have increasingly shown that SpliceSeq based on RNA-Seq is a reliable and robust technology for analyzing large-scale sequencing data of AS. In addition, QRCR verification for the DEAS results obtained from TCGA database analyses are generally consistent (41). We used TCGA SpliceSeq to obtain the PSI value of BRCA AS events. Taking into account the difference in the basic expression level of the PSI value in distinct patients, 113 pairs of tumors and matched normal tissues were analyzed, with 973 DEAS events from the 621 genes obtained. Notably, there were 19 DEAS co-existing in BC, HNSC and CRC, suggesting that these AS events have the potential to drive distinct cancers. Through screening the 19 DEAS-related studies, we found that tenascin-C (TNC), an extracellular matrix glycoprotein, had different predominant isoforms in tumor and normal breast tissues. The completely truncated TNC isoform mainly existed in normal and benign tissues, whereas the higher molecular weight isoforms were mainly found in cancer. In addition, exon 14 and 16 were proved to be tumor-related, having previously been shown to affect the invasion and growth of breast cell lines to a greater extent than the full-length TNC isoform in vitro (42). Studies have also reported that TNC and the polypeptide TNIIIA2, which is derived from the AS domain A2 of TNC, can promote colon cancer invasion through the MMP pathway in vivo and in vitro (43). Moreover, TNC-L and its cell surface receptor ANXA2 are associated with a poor prognosis in patients with pancreatic cancer (44). The above research indicates that the abnormal AS of TNC has universal significance in regulating the occurrence and progression of different tumors. In addition, gene exon array research has found that the cancer-specific exon 6 of COL6A3, a component of the microfiber network, is expressed in colon, bladder, and prostate cancer (45,46). Moreover, the high expression of COL6A3 E5-E6 junction is associated with a better prognosis in CRC (47). The above reports confirm the reliability of our results and reveal the common function of shared AS events in oncogenesis.
Further functional enrichment analysis also found that the corresponding genes of DEAS were enriched in cell adhesion, cytoskeletal organization, ECM-receptor interaction, and AMPK signaling pathways. Cell adhesion to the extracellular matrix is the key to maintaining tissue integrity (48) and plays a vital role in controlling the spread and migration of tumor cells. Genes in the ECM-receptor interaction pathway have been reported to be widely dysregulated in BRCA and are related to patient prognosis (49). The AMPK pathway is a key pathway that regulates energy balance and participates in regulating the metabolic reprogramming of BRCA (50). Our enrichment analysis suggested that DEAS might play an important function in the occurrence and progression of BRCA. On this basis we extracted the quantified wound-healing value of TCGA BRCA patients from the previous study and analyzed its correlation with the PSI value of DEAS events. Unexpectedly, many DEAS events showed a strong correlation with the wound-healing value indicating metastatic ability. Interestingly, different AS isoforms of the same gene were shown to exhibit diametrically opposite metastatic capabilities. For example, AT from exon 29 in KIF4A was downregulated in tumor compared to normal tissue, which was negatively correlated with migration, while AT from exon 32 in KIF4A was upregulated and positively associated with migration. The function of these isoforms in BRCA needs further exploration both in vivo and in vitro. As trans-acting factors, SFs can specifically bind to the cis-acting element on pre-RNA and participate in the selection of splicing sites and the assembly of the spliceosome. Therefore, we analyzed and constructed a possible splicing regulatory network based on the correlation between the RNA expression level of SFs and the PSI value of DEAS events. Our results suggested that SFs were closely related with DEAS events. The analysis results indicated that the SFs ESRP1 and SRSF1, which had been reported to participate in the regulation of abnormal AS events in the development of BRCA (51,52), were cooperatively involved in the regulation of multiple DEAS events. While there are rare reports of abnormal AS events being cooperatively regulated by SFs ESRP1 and SRSF1 in BRCA, this requires further investigation. We also explored the possible regulatory mechanisms of SFs and found that the expression of certain SFs was affected by methylation of the promoter region or CNV. In other words, the results suggested that epigenetic modification mediated DEAS events in BRCA by regulating the expression of SFs.
The analysis results showed that DEAS events had potential significance in tumor biology, especially in the aspects of invasion and metastasis, and its clinical significance in BRCA was worthy of attention. Based on this, we analyzed the DFS-related DEAS events in BRCA and used the LASSO Cox model to construct the DEAS-related risk signature. We identified eight AS events and their corresponding genes which had been reported to effect tumor biological functions. For instance, TOM1L is a pivotal component of the ERBB2-driven proteolytic invasive programme. In ERBB2-positive BRCA, TOM1L1 upregulation potentially improves metastasis (53), whereas UBE2E1 is a predictive marker in acute myeloid leukemia (54). In addition, the DFS of the low-risk group determined by the DEAS-related signature was significantly longer than the high-risk group. Moreover, patients in the high-risk group were associated with clinical features that indicated a poorer prognosis, such as basal-like molecular subtype, higher grade/ T/clinical stage, and more invasive tumor behavior.
Conclusions
Previous analyses based on BRCA TCGA AS data mainly focused on constructing prognostic prediction models for OS (33,35,55). In comparison, our analysis used PAM_50-based molecular subtype, included BRCA patients with relatively complete clinical information, adopted more stringent AS events screening criteria, and identified 973 DEAS events. Functional enrichment analysis revealed the potential tumor biological mechanism of DEAS events. Interestingly, we found 19 AS events that were differentially expressed in three different cancers, suggesting these events might have general effects on oncogenesis. Construction of the correlation network between DEAS events and SFs further revealed the potential regulatory mechanism of AS events. Analysis of the methylation of promoter and the CNV status of SFs partially deciphered the drivers of abnormal SFs. The results of survival and clinical correlation analysis of DEAS events suggested that DEAS events not only have important value in revealing oncology mechanisms but might also be used as a prognostic predictor and potential therapeutic target.
Acknowledgments
Funding: This work was supported by grants from the Excellent Backbone Plan of Zhongshan Hospital in 2019 (2019ZSGG08), National Natural Science Fund of China (Grant numbers: No. 81871916; No.81672330; No. 81472218) and Talent Development Program of Zhongshan Hospital (2015ZSYXGG).
Footnote
Reporting Checklist: The authors have completed the MDA reporting checklist. Available at http://dx.doi.org/10.21037/atm-20-7203
Conflicts of Interest: All authors have completed the ICMJE uniform disclosure form (available at http://dx.doi.org/10.21037/atm-20-7203). The authors have no conflicts of interest to declare.
Ethical Statement: The authors are accountable for all aspects of the work in ensuring that questions related to the accuracy or integrity of any part of the work are appropriately investigated and resolved. The study was conducted in accordance with the Declaration of Helsinki (as revised in 2013) and was approved by the institutional review board of Zhongshan Hospital, Fudan University (approval number: B2020-108R). All written informed consent was obtained.
Open Access Statement: This is an Open Access article distributed in accordance with the Creative Commons Attribution-NonCommercial-NoDerivs 4.0 International License (CC BY-NC-ND 4.0), which permits the non-commercial replication and distribution of the article with the strict proviso that no changes or edits are made and the original work is properly cited (including links to both the formal publication through the relevant DOI and the license). See: https://creativecommons.org/licenses/by-nc-nd/4.0/.
References
- Siegel RL, Miller KD. Cancer Statistics, 2019. CA Cancer J Clin 2019;69:7-34. [Crossref] [PubMed]
- Wang Y, Yang Y, Chen Z, et al. Development and validation of a novel nomogram for predicting distant metastasis-free survival among breast cancer patients. Ann Transl Med 2019;7:537. [Crossref] [PubMed]
- Qian JY, Gao J, Sun X, et al. KIAA1429 acts as an oncogenic factor in breast cancer by regulating CDK1 in an N6-methyladenosine-independent manner. Oncogene 2019;38:6123-41. [Crossref] [PubMed]
- Dustin D, Gu G, Fuqua SAW. ESR1 mutations in breast cancer. Cancer 2019;125:3714-28. [Crossref] [PubMed]
- Kumaran M, Cass CE, Graham K, et al. Germline copy number variations are associated with breast cancer risk and prognosis. Sci Rep 2017;7:14621. [Crossref] [PubMed]
- Chen X, Zhang J, Dai X. DNA methylation profiles capturing breast cancer heterogeneity. BMC Genomics 2019;20:823. [Crossref] [PubMed]
- Zhao S, Zuo WJ, Shao ZM, et al. Molecular subtypes and precision treatment of triple-negative breast cancer. Ann Transl Med 2020;8:499. [Crossref] [PubMed]
- Matera AG, Wang Z. A day in the life of the spliceosome. Nat Rev Mol Cell Biol 2014;15:108-21. [Crossref] [PubMed]
- Oltean S, Bates DO. Hallmarks of alternative splicing in cancer. Oncogene 2014;33:5311-8. [Crossref] [PubMed]
- Muñoz Ú, Puche JE, Hannivoort R, et al. Hepatocyte growth factor enhances alternative splicing of the Kruppel-like factor 6 (KLF6) tumor suppressor to promote growth through SRSF1. Mol Cancer Res 2012;10:1216-27. [Crossref] [PubMed]
- Stevens M, Oltean S. Modulation of the Apoptosis Gene Bcl-x Function Through Alternative Splicing. Front Genet 2019;10:804. [Crossref] [PubMed]
- Pradella D, Naro C, Sette C, et al. EMT and stemness: flexible processes tuned by alternative splicing in development and cancer progression. Mol Cancer 2017;16:8. [Crossref] [PubMed]
- Bowler E, Oltean S. Alternative Splicing in Angiogenesis. Int J Mol Sci 2019;20:2067. [Crossref] [PubMed]
- Kozlovski I, Siegfried Z, Amar-Schwartz A, et al. The role of RNA alternative splicing in regulating cancer metabolism. Hum Genet 2017;136:1113-27. [Crossref] [PubMed]
- Yang Q, Zhao J, Zhang W, et al. Aberrant alternative splicing in breast cancer. J Mol Cell Biol 2019;11:920-9. [Crossref] [PubMed]
- Piqué L, Martinez de Paz A, Piñeyro D, et al. Epigenetic inactivation of the splicing RNA-binding protein CELF2 in human breast cancer. Oncogene 2019;38:7106-12. [Crossref] [PubMed]
- Yan Y, Yang X, Liu Y, et al. Copy number variation of functional RBMY1 is associated with sperm motility: An azoospermia factor-linked candidate for asthenozoospermia. Hum Reprod 2017;32:1521-31. [Crossref] [PubMed]
- Zhao J, Li J, Hassan W, et al. Sam68 promotes aerobic glycolysis in colorectal cancer by regulating PKM2 alternative splicing. Ann Transl Med 2020;8:459. [Crossref] [PubMed]
- Zhu J, Chen Z, Yong L. Systematic profiling of alternative splicing signature reveals prognostic predictor for ovarian cancer. Gynecol Oncol 2018;148:368-74. [Crossref] [PubMed]
- Li Y, Sun N, Lu Z, et al. Prognostic alternative mRNA splicing signature in non-small cell lung cancer. Cancer Lett 2017;393:40-51. [Crossref] [PubMed]
- Cerami E, Gao J, Dogrusoz U, et al. The cBio Cancer Genomics Portal: An open platform for exploring multidimensional cancer genomics data. Cancer Discov 2012;2:401-4. [Crossref] [PubMed]
- Xiong Y, Deng Y, Wang K, et al. Profiles of alternative splicing in colorectal cancer and their clinical significance: A study based on large-scale sequencing data. EBioMedicine 2018;36:183-95. [Crossref] [PubMed]
- Li ZX, Zheng ZQ, Wei ZH, et al. Comprehensive characterization of the alternative splicing landscape in head and neck squamous cell carcinoma reveals novel events associated with tumorigenesis and the immune microenvironment. Theranostics 2019;9:7648-65. [Crossref] [PubMed]
- Thorsson V, Gibbs DL, Brown SD, et al. The Immune Landscape of Cancer. Immunity 2018;48:812-30.e14. [Crossref] [PubMed]
- Conway JR, Lex A, Gehlenborg N. UpSetR: An R package for the visualization of intersecting sets and their properties. Bioinformatics 2017;33:2938-40. [Crossref] [PubMed]
- Chen H, Boutros PC. VennDiagram: a package for the generation of highly-customizable Venn and Euler diagrams in R. BMC Bioinformatics 2011;12:35. [Crossref] [PubMed]
- Yu G, Wang LG, Han Y, et al. clusterProfiler: an R package for comparing biological themes among gene clusters. OMICS 2012;16:284-7. [Crossref] [PubMed]
- Szklarczyk D, Franceschini A, Kuhn M, et al. The STRING database in 2011: functional interaction networks of proteins, globally integrated and scored. Nucleic Acids Res 2011;39:D561-8. [Crossref] [PubMed]
- Su G, Morris JH, Demchak B, et al. Biological network exploration with Cytoscape 3. Curr Protoc Bioinformatics 2014;47:8.13.1-8.13.24.
- Giulietti M, Piva F, D'Antonio M, et al. SpliceAid-F: a database of human splicing factors and their RNA-binding sites. Nucleic Acids Res 2013;41:D125-31. [Crossref] [PubMed]
- Goeman JJ. L1 penalized estimation in the Cox proportional hazards model. Biom J 2010;52:70-84. [PubMed]
- Heagerty PJ. Time-Dependent ROC Curves for Censored Survival Data and a Diagnostic Marker. Biometrics 2000;56:337-44. [Crossref] [PubMed]
- Gong S, Song Z, Spezia-Lindner D, et al. Novel Insights Into Triple-Negative Breast Cancer Prognosis by Comprehensive Characterization of Aberrant Alternative Splicing. Front Genet 2020;11:534. [Crossref] [PubMed]
- Qiu Y, Lyu J, Dunlap M, et al. A combinatorially regulated RNA splicing signature predicts breast cancer EMT states and patient survival. RNA 2020;26:1257-67. [Crossref] [PubMed]
- Liu Q, Wang X, Kong X, et al. Prognostic Alternative mRNA Splicing Signature and a Novel Biomarker in Triple-Negative Breast Cancer. DNA Cell Biol. 2020;39:1051-63. [Crossref] [PubMed]
- Guiqi Z, Yujie Z, Lixin Q, et al. Prognostic alternative mRNA splicing signature in hepatocellular carcinoma: A study based on large-scale sequencing data. Carcinogenesis 2019. Epub ahead of print. [Crossref] [PubMed]
- Tyson-Capper A, Gautrey H. Regulation of Mcl-1 alternative splicing by hnRNP F, H1 and K in breast cancer cells. RNA Biol 2018;15:1448-57. [Crossref] [PubMed]
- Zhang H, Brown RL, Wei Y, et al. CD44 splice isoform switching determines breast cancer stem cell state. Genes Dev 2019;33:166-79. [Crossref] [PubMed]
- Wang X, Guo J, Che X, et al. PCBP1 inhibits the expression of oncogenic STAT3 isoform by targeting alternative splicing of STAT3 exon 23. Int J Biol Sci 2019;15:1177-86. [Crossref] [PubMed]
- Aigner A, Juhl H, Malerczyk C, et al. Expression of a truncated 100 kDa HER2 splice variant acts as an endogenous inhibitor of tumour cell proliferation. Oncogene 2001;20:2101-11. [Crossref] [PubMed]
- Feng H, Qin Z, Zhang X. Opportunities and methods for studying alternative splicing in cancer with RNA-Seq. Cancer Lett 2013;340:179-91. [Crossref] [PubMed]
- Guttery DS, Shaw JA, Lloyd K, et al. Expression of tenascin-C and its isoforms in the breast. Cancer Metastasis Rev 2010;29:595-606. [Crossref] [PubMed]
- Suzuki H, Sasada M, Kamiya S, et al. The Promoting Effect of the Extracellular Matrix Peptide TNIIIA2 Derived from Tenascin-C in Colon Cancer Cell Infiltration. Int J Mol Sci 2017;18:181. [Crossref] [PubMed]
- Hagiwara K, Harimoto N, Yokobori T, et al. High Co-expression of Large Tenascin C Splice Variants in Stromal Tissue and Annexin A2 in Cancer Cell Membranes is Associated with Poor Prognosis in Pancreatic Cancer. Ann Surg Oncol 2020;27:924-30. [Crossref] [PubMed]
- Thorsen K, Sørensen KD, Brems-Eskildsen A, et al. Alternative splicing in colon, bladder, and prostate cancer identified by exon array analysis. Mol Cell Proteomics. 2008;7:1214-24. [Crossref] [PubMed]
- Arafat H, Lazar M, Salem K, et al. Tumor-specific expression and alternative splicing of the COL6A3 gene in pancreatic cancer. Surgery 2011;150:306-15. [Crossref] [PubMed]
- Lian H, Wang A, Shen Y, et al. Identification of novel alternative splicing isoform biomarkers and their association with overall survival in colorectal cancer. BMC Gastroenterol 2020;20:171. [Crossref] [PubMed]
- Hamidi H, Ivaska J. Every step of the way: integrins in cancer progression and metastasis. Nat Rev Cancer 2018;18:533-48. [Crossref] [PubMed]
- Bao Y, Wang L, Shi L, et al. Transcriptome profiling revealed multiple genes and ECM-receptor interaction pathways that may be associated with breast cancer. Cell Mol Biol Lett 2019;24:38. [PubMed]
- Zhao H, Orhan YC, Zha X, et al. AMP-activated protein kinase and energy balance in breast cancer. Am J Transl Res 2017;9:197-213. [PubMed]
- Gökmen‐Polar Y, Neelamraju Y, Goswami CP, et al. Splicing factor ESRP 1 controls ER ‐positive breast cancer by altering metabolic pathways. EMBO Rep 2019;20:1-19. [Crossref] [PubMed]
- Anczuków O, Akerman M, Cléry A, et al. SRSF1-Regulated Alternative Splicing in Breast Cancer. Mol Cell 2015;60:105-17. [Crossref] [PubMed]
- Chevalier C, Collin G, Descamps S, et al. TOM1L1 drives membrane delivery of MT1-MMP to promote ERBB2-induced breast cancer cell invasion. Nat Commun 2016;7:10765. [Crossref] [PubMed]
- Luo H, Qin Y, Reu F, et al. Microarray-based analysis and clinical validation identify ubiquitin-conjugating enzyme E2E1 (UBE2E1) as a prognostic factor in acute myeloid leukemia. J Hematol Oncol 2016;9:125. [Crossref] [PubMed]
- Zhang D, Duan Y, Cun J, et al. Identification of Prognostic Alternative Splicing Signature in Breast Carcinoma. Front Genet 2019;10:278. [Crossref] [PubMed]
(English Language Editor: B. Draper)