Alteration of spatial patterns at the network-level in facial synkinesis: an independent component and connectome analysis
Introduction
Post-facial palsy synkinesis (PFPS) is a sequela of facial nerve injury. It manifests as involuntary abnormal facial movements accompanied by volitional movement in another area of the face, such as eye closure, triggered by the volitional movement of the mouth (1,2). However, the common therapeutic modalities for synkinesis, including facial neuromuscular retraining with physical therapy, targeted chemodenervation, and surgical procedures, are not sufficiently efficacious. Therefore, synkinesis remains a significant clinical challenge owing to its unclear causative mechanisms (3-5). In particular, it is unclear how abnormal information exchanges occur within the architecture of the sub-networks. This hinders our understanding of the full spectrum of pathophysiologic interactions in this debilitating movement disorder.
Previous neuroimaging studies on movement disorders have demonstrated brain regions responsible for sensorimotor control, aberrant excitation, inhibition of the basal ganglia circuitry, and abnormal integration of the basal ganglia, thalamus, insula, and cerebellum, with the primary sensorimotor engaged in disorder-specific alterations (6-9). Also, many studies of task-dependent on facial synkinesis lead us to conclude that there is one particular module that focuses on the projection area in the brain map, which specifically corresponds to ocular-oral or oral-ocular synkinesis (10). This isolated activation has been well studied. However, the brain works as an integrity of several regions but not several isolated modules (7,11). The brain network recruits neurons to complete various functional activities, such as sensorimotor, speech, and cognition, in an integrated circuit (12). Instead of exploring large-scale and distributed properties for information processing, research on facial synkinesis research has started to focus on regional alterations patterns or deficits (13).
To examine the brain plasticity changes associated with facial paralysis, we used an independent component analysis (ICA) to compare patients with PFPS and healthy controls (HCs) in resting-state functional magnetic resonance imaging (fMRI). We built a graph theory analysis based on the results of the ICA that identified the topological changes in patients with PFPS compared to HCs on the whole-brain network scale and functional sub-network range, to expand our understanding of global and focal alterations. PFPS is a sequela of facial palsy, which generally does not cause a strong reaction in the whole brain. However, most researchers still prefer to target an area (14). We hypothesize that functional sub-networks, rather than the whole-brain network, can better explain the abnormal movement, collectively inducing neural plasticity of pathologic involuntary activity. This study used a high-accuracy network analysis that was able to detect mild changes to a limited extent from the perspective of the connectome; this is useful for increasing our understanding of PFPS.
We present the following article in accordance with the MDAR reporting checklist (available at http://dx.doi.org/10.21037/atm-20-4865).
Methods
Participants
Thirty-one patients with PFPS (20 females; age range: 12–49 years; mean age: 33.61 years) and 19 HCs (17 females; age range: 23–42 years; mean age: 33.21 years) participated in this cross-sectional study (Table 1). Patients with Bell’s palsy were included, who were suffered from unilateral facial synkinesis, as well as participants that were recruited via two experienced plastic surgeons from the Department of Plastic Surgery. Inclusion criteria were medical history of 9 months or over and signed informed consent for the study. Participants with one or more of the following characteristics were excluded: Hunt syndrome or other causes (e.g., trauma, iatrogenic, or parotid tumors); central nervous system pathology; recurrent BP or concurrent peripheral neuropathy; a history of nerve transposition, and contraindications to an investigation by MRI. All fMRI measurements and clinical assessments were performed on the same day. The whole procedure lasts about 75 minutes. Besides, a neurological examination (normal vision and the capability to follow instructions) and an interview (the Sunny-Brook facial grading system for patients) were conducted. The HCs had to be free of facial palsy or other neurological impairments. All participants signed informed consent forms before proceeding to the trial according to the Helsinki declaration (as revised in 2013). The study was approved by the local ethics committee (approval number 2017-365-T267), and the study protocol was registered in the Chinese Clinical Trial Registry (ChiCTR1800014630).
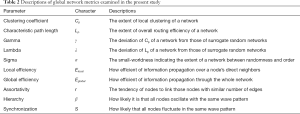
Full table
Data acquisition and preprocessing
All subjects went through a whole-brain scan of resting-state fMRI (rs-fMRI). fMRI data were taken on a 3 T MRI scanner (GE Signa VH/I) using a 32-channel phased-array head coil. The rs-fMRI in whole-brain used a gradient echo-planar imaging sequence (interleaved scanning order, slice number =43, TR =2,000 ms, matrix size =64×64, FOV =220×220 mm, voxel size 3.4×3.4×3.2 mm3, number of acquisitions =240). The preprocessing and analysis of fMRI data was performed using SPM12 (http://www.fil.ion.ucl.ac.uk/spm) and the graph-theoretical network analysis was developed on the GRaph thEoreTical Network Analysis (GRETNA) (http://www.nitrc.org/projects/gretna/) toolbox (15). Briefly, after discarding the first 10 volumes of each fMRI run, slice timing was performed to correct the inconsistency of temporal collection. Then the data were motion-corrected, normalized to stereotactic Montreal Neurological Institute (MNI) space via a standard EPI template, and spatially smoothed (6×6×6 mm3 Gaussian kernel). Point-to-point head motion and mean head motion was estimated for subjects to control the motion-induced artifacts. Therefore, one patient with excessive head motion (cumulative translation or rotation >3 mm or 3°) was excluded (16).
Node and edge definition
Topological analyses in whole-brain network and functional sub-network were conducted in this study. We used an AAL90 structure-labeled atlas for parcellation in the whole-brain network analysis. Thus, nodes were defined as the parcellated 90 brain regions. Subsequently, Pearson’s correlations of the meantime series between all pairs of the 90 nodes were characterized as the edges.
For the sub-network analysis, preprocessed rs-fMRI data were decomposed into independent components (ICs) using GIFT software (Group ICA of fMRI Toolbox, version 4.0, http://icatb.sourceforge.net) to construct the sub-networks. Generally, the group ICA for this multi-subject analysis utilized a concatenation approach plus back-reconstruction (17). The images were first dimensionally reduced using principal component analysis (PCA), then temporally concatenated and reduced to extract 40 spatial components using the expectation-maximization algorithm at a group level. In addition, we performed the infomax ICA algorithm in ICASSO for 100 repetitions to validate its robustness. After the aggregated spatial maps were estimated, subject-specific spatial patterns and time courses were extracted through the back-reconstruction approach. A time course for all subjects as well as spatial weight maps were produced, which revealed the possibility of one voxel belonging to one particular component. Then we thresholded these maps at group level after a Z-transformation of the spatial weight map.
We selected meaningful ICs through spatial sorting and visual inspection. Four ICs-of-interest were identified in this study: the sensorimotor network (SMN) (Figure 1A), frontoparietal network (FPN) (Figure 1B), dorsal default mode network (dDMN) (Figure 1C), and ventral default mode network (vDMN) (Figure 1D). For these sub-networks, we specified each voxel as the node and functional connectivity of the voxel–voxel as the edge. Besides, we resampled the data and adjusted the voxel size to 6×6×6 mm3 to decrease the large scale of the connectivity matrix, which reduced the computational work significantly.
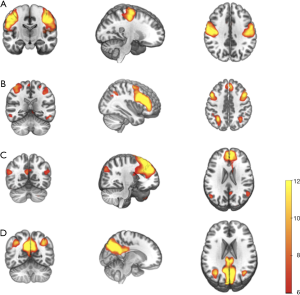
After the functional connectivity matrices were obtained, we calculated the network metrics of the whole-brain and the sub-networks separately using a pre-selected sparsity value (the ratio of the number of actual edges divided by the maximum possible number of edges in a network) to ensure the relative network organization. Specifically, the topological organization of whole-brain networks was analyzed over a wide range of network sparsity (0.05–0.45) (19), where the small-world metrics were analyzed (20). Network density was applied to each adjacent matrix with an increment of 0.01 for the whole-brain network and 0.02 for the sub-network metrics to reduce the computational dimension.
Network analysis
Global properties of the whole-brain network and sub-networks
Graph theory analysis is a suitable method to characterize the brain network topology properties (12,21). The commonly used network metrics, including the small-world property (clustering coefficient Cp, characteristic path length Lp, normalized clustering coefficient γ, normalized characteristic path length λ and small-worldness σ), network efficiency (local efficiency Elocal and global efficiency Eglobal), assortativity r, hierarchy β and synchronization S), were calculated in this study. All the calculations of network metrics were completed with GRETNA. Table 2 gives detailed descriptions of the above metrics (15,22).
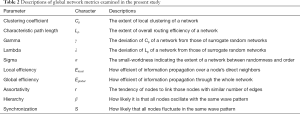
Full table
The small-world parameters hold on both modularized/specialized and distributed/integrated information processing and greatly amplify the efficiency of information transfer at a low-cost. Therefore, small-world networks have a shorter Lp than regular networks (high Cp and long Lp) but greater Elocal than random networks (low Cp and short Lp). To characterize the small-world properties of the target network, we calculated its Cp and Lp with the corresponding value, which derive from the average of 100 random networks with the equal scale of nodes and degree distribution (23). Compared with the random networks, small-world networks have a relatively high normalized clustering coefficient γ (Cp/Cprand) >1 and relatively low normalized characteristic path length λ (Lp/Lprand) ≈1 (24).
Global efficiency measures the efficiency of information transfer at a network level, which is the inverse of the harmonic mean of the minimum path length (25). While the local efficiency of the network measures the fault tolerance in a network, showing how the efficient communication exchanges when the first neighbors of a given node is phased out (25).
Additionally, we evaluated the hierarchical nature of networks using the β parameter (26), which defines the size of the power–law relationship between the clustering coefficient (Cp) and degree (k): Cp ≈ k-β (27). In a network with hierarchy organizations, some high-degree related nodes form a densely connected cluster. These generated clusters act as elements in the next level of the network and merge into a larger-scale interconnected cluster (28). We computed the parameter β for the network through the plot of log(C) versus log(k) applied with regression line fitting.
In addition, assortativity reflects the tendency of nodes to link those nodes with a similar number of edges, which measures the correlation between the node degree and the mean degree of its adjacent neighbor (29). Positive assortativity indicates that the densely connected nodes are more likely to associate with other nodes with the same degree. Synchronization, which is described as the ratio of the second smallest eigenvalue to the largest eigenvalue of the coupling matrix of the network, measures the possibility of the fluctuation of all nodes in the same wave pattern.
Regional properties of the whole-brain network
For nodal metrics, we also calculated the regional properties, including the nodal degree, nodal efficiency, and betweenness centrality of the whole-brain network. The nodal characteristics of the brain networks may demonstrate the importance of specific areas in brain network by measuring the extent to which a given node connects to all other nodes of a network (25,30).
Data analysis
The area under the curve (AUC) over the sparsity range was used to conduct group comparisons of the metric. Statistical tests of topological measures between the groups were performed using permutation testing to assess alterations of the topological parameters (10,000 permutations) for each topological parameter over a wide range of connection densities. For each permutation, participants were randomly assigned to patients and HCs with the same size as the original two groups. Next, the randomized groups difference of the metric was measured to obtain a null distribution. Depended on the generated null distribution, a P value was charged to evaluate the extent of alteration between groups. A P value of <0.05 was considered statistically significant (31). In the nodal properties analysis, a correction of false discovery rate (FDR) for multiple comparisons was performed at a q value of 0.05 (32).
Results
Demographic and clinical characteristics
The patients with PFPS (females; age: 33.61±8.55 years) and 19 HC (females; age: 33.21±6.75 years) were included in the statistical analysis. We observed non-significant differences between PFPS and HC concerning age (P=0.86) and gender (P=0.11). Demographic and clinical characteristics of these subjects is provided in Table 1. One patient was excluded because his head-motion beyond 3 mm or 3°.
Alterations in whole-brain organization of the functional connectome
In the initial test of global topological parameters, we found λ ≈1, γ >1, and σ = γ /λ >1, which indicates the networks are of small-worldness property. As displayed by Figure 2, the topological metrics in whole-brain propagation between groups were non-significant. In the nodal analysis, none of the nodes in the regional properties’ analysis (including nodal degree, nodal efficiency, and betweenness centrality) were significant after FDR correction. That means, there was no significant difference between the patient group and the HCs on the whole-brain scale.
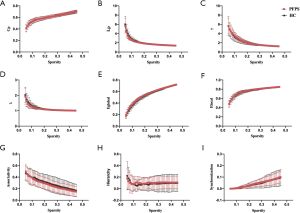
Alterations in sub-network organization of the functional connectome
We identified four subnetworks from the resting-state data after the ICA procedure (Figure 1): SMN (component A), FPN (component B), dDMN (component C), and vDMN (component D). A comparison of component A between groups revealed that γ (10,000 permutations, P=0.048) and synchronization (10,000 permutations, P=0.022) within the SMN progressively decreased in patients with PFPS (Figure 3). A comparison of components B and C found a non-significant difference between the groups. The FPN and dDMN show analogous patterns in PFPS and HC (Figures 4,5). For component D, significant differences were found in γ (10,000 permutations, P=0.019), Elocal (10,000 permutations, P=0.008), and hierarchy (10,000 permutations, P=0.011) between the groups. The PFPS group showed lower γ, Elocal, and hierarchy of the vDMN than did the HCs (Figure 6).
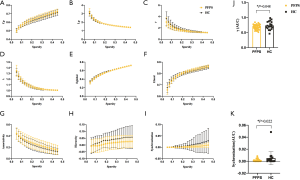
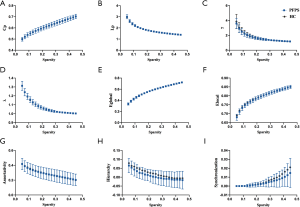
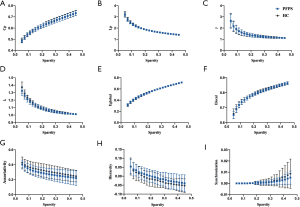
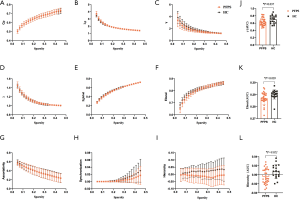
Discussion
In recent years, cortical plasticity in PFPS has been extensively discussed (33,34). To date, most work has attempted to identify neuro markers that reveal mechanisms and neurophysiological processes that are significant in PFPS (9,35). Considering that the experience of synkinesis has diverse influences, from a deficit in control to social context, neuro marker candidates will be found to reflect all aspects of involuntary movements in all contexts and help to afford clinical prognostic indicators (36-38). We utilized a graph theory analysis to examine the resting-state fMRI data to characterize the global and local features after facial synkinesis, instead of localization information. Beyond regional activity, PFPS in our study was found to influence intrinsic brain networks (also known as resting-state networks), mainly the somatosensory networks, and default mode networks, which subserve homeostatic, attentional, cognitive, executive, and sensory functions together and alter their functional connectivity. Therefore, facial synkinesis is a major complication of facial paralysis while it does not induce extensive plasticity of the whole brain. By contrast, it only leads to limited topological changes in some functional sub-networks.
Our study shows that the activity induced by synkinesis is related to many brain regions that belong to different functional brain networks, rather than the dedicated areas within the brain. These components of networks belong to the default mode, and somatosensory networks that control the cortical and subcortical representations of synkinesis movements, which have been clarified in large-scale resting-state studies, extensively overlap with the regions related to PFPS (39). The functional network of the brain is complicated and includes areas associated with coding information regarding muscular control and reactions to muscular control, the recognition and modulation of spatial information, and somatosensory information and attention (40). This might be the reason for the deactivated SMN, which plays an important role in motor control and consists of somatosensory (post-central gyrus) and motor (pre-central gyrus) regions, and extends to the supplementary motor areas. The vDMN, which is spontaneously active during passive performance and associated with effective processing, memory, and self-projective thinking (41,42), consists of the posterior cingulate cortex, medial prefrontal cortex, angular gyrus, hippocampus, parahippocampus, retrosplenial cortex, and posterior inferior parietal lobe. Consequently, these intrinsic networks and dedicated connectomes may be engaged during facial synkinesis. The primary aim of this study was to define the scale affected by facial synkinesis.
Although regular networks and random graphs are both useful idealizations, many real networks lie somewhere between order and randomness. Small-world networks have been extensively researched since the fundamental work by Watts and Strogatz, who indicated that random mutation of a few connections in a regular or lattice network extensively lessened the mean minimum path length between any pair of nodes while holding strong local connectivity or “cliquishness” (43). The coexistence of integration and separation further reflects the small worldness characteristics of the networks.
The local efficiency of the network evaluates how efficient communication is among the first neighbors of a given node when it is eliminated. A lower Elocal in SMN and vDMN means that the possibility of “off track” increases, suggesting a trend of “separation” of these functional sub-networks. The decrease in Elocal also indicates the randomization of the sub-networks, reflecting the decrease in overall efficiency and change of the integration-separation balance in different neural functional sub-networks. Hierarchy is the tendency of hub regions to connect with nodes outside the hubs (44). In this study, it was found that the subjects’ functional complex sub-networks had small-world attributes, and the efficiency of regional information transmitting in SMN and vDMN decreased. It is suggested that patients with facial synkinesis can cause changes in the mode of brain information processing.
The declined propagation characteristics in the sub-network represent less active neural transmitting activity in facial paralysis. Therefore, the connection of the subnetworks is diminished. The results imply a disruption of the efficiency of local information in the sub-networks, which is a manifestation of maladaptive alteration in facial synkinesis. According to previous studies, facial synkinesis seems to be a facial mismatch on neural activity rather than deactivation (39,45). We found that facial synkinesis tends to reduce the cluster efficiency to lower than normal, which may illustrate the effects on cortical plasticity from the perspective of neural mechanisms. Furthermore, we discovered many measurements, including network efficiency and synchronization, that showed neither significant nor trending differences in any sub-network. Therefore, we believe that they may not be involved in the neuroplasticity of facial synkinesis. Such interpretations are not difficult as post-facial paralysis synkinesis only causes changes in topological properties to a limited extent. This would not be significant if the contrast were executed on a global scale. These findings should be confirmed with a larger sample size in the future.
The present study investigated the topological structure of functional brain networks between HCs and patients with PFPS. The rs-fMRI images were examined using a graph theory analysis to study the brain functional connectomes. No significant differences in the whole-brain scale were observed between patients with PFPS and HCs. However, patients with PFPS had decreased small-worldness and synchronization in the SMN and decreased small-worldness, local efficiency, and hierarchy structures in the vDMN. Moreover, patients with PFPS had altered network metrics in the SMN and vDMN brain regions, mainly involved in information transport, attention, memory, and emotion.
Conclusions
The current results provide the first evidence that reduced intrinsic connectivity represents regionally restricted information transmission efficiency in the SMNs and vDMNs of patients with PFPS. PFPS produces changes in neuroplasticity in sub-networks rather than the whole brain network. These findings indicate that functional disruption in patients with PFPS may be associated with instabilities in the local topological organization of the brain functional connectome. In addition, this study provides new insights into the pathophysiological alterations to further reaching the mechanism of PFPS in-depth.
Acknowledgments
Funding: This work was supported by the Open Project of Shanghai Key Laboratory of Peripheral Nerve and Microsurgery [Grant Number 17DZ2270500].
Footnote
Reporting Checklist: The authors have completed the MDAR reporting checklist. Available at http://dx.doi.org/10.21037/atm-20-4865
Data Sharing Statement: Available at http://dx.doi.org/10.21037/atm-20-4865
Peer Review File: Available at http://dx.doi.org/10.21037/atm-20-4865
Conflicts of Interest: All authors have completed the ICMJE uniform disclosure form (available at http://dx.doi.org/10.21037/atm-20-4865). All authors report grants from Open Project of Shanghai Key Laboratory of Peripheral Nerve and Microsurgery [Grant Number 17DZ2270500], during the conduct of the study. The other authors have no conflicts of interest to declare.
Ethical Statement: The authors are accountable for all aspects of the work in ensuring that questions related to the accuracy or integrity of any part of the work are appropriately investigated and resolved. The study was conducted in accordance with the Declaration of Helsinki (as revised in 2013), and the Harmonized Tripartite Guideline for Good Clinical Practice from the International Conference on Harmonization. This study was reviewed and approved by the Ethics Committee of the Shanghai Jiao Tong University (approval number 2017-365-T267), and the study protocol was registered in the Chinese Clinical Trial Registry (ChiCTR1800014630). All patients enrolled completed the informed consent form.
Open Access Statement: This is an Open Access article distributed in accordance with the Creative Commons Attribution-NonCommercial-NoDerivs 4.0 International License (CC BY-NC-ND 4.0), which permits the non-commercial replication and distribution of the article with the strict proviso that no changes or edits are made and the original work is properly cited (including links to both the formal publication through the relevant DOI and the license). See: https://creativecommons.org/licenses/by-nc-nd/4.0/.
References
- Markey JD, Loyo M. Latest advances in the management of facial synkinesis. Curr Opin Otolaryngol Head Neck Surg 2017;25:265-72. [Crossref] [PubMed]
- Shinn JR, Nwabueze NN, Du L, et al. Treatment Patterns and Outcomes in Botulinum Therapy for Patients With Facial Synkinesis. JAMA Facial Plast Surg 2019;21:244-51. [Crossref] [PubMed]
- Cronin GW. The effectiveness of neuromuscular facial retraining combined with electromyography in facial paralysis rehabilitation. Otolaryngol Head Neck Surg 2003;128:534-8. [Crossref] [PubMed]
- Tenney AP, Livet J, Belton T, et al. Etv1 Controls the Establishment of Non-overlapping Motor Innervation of Neighboring Facial Muscles during Development. Cell Rep 2019;29:437-52.e4. [Crossref] [PubMed]
- Watanabe Y, Akizuki T, Ozawa T, et al. Dual innervation method using one-stage reconstruction with free latissimus dorsi muscle transfer for re-animation of established facial paralysis: simultaneous reinnervation of the ipsilateral masseter motor nerve and the contralateral facial nerve to improve the quality of smile and emotional facial expressions. J Plast Reconstr Aesthet Surg 2009;62:1589-97. [Crossref] [PubMed]
- Battistella G, Simonyan K. Top-down alteration of functional connectivity within the sensorimotor network in focal dystonia. Neurology 2019;92:e1843-51. [Crossref] [PubMed]
- Smith SM, Vidaurre D, Beckmann CF, et al. Functional connectomics from resting-state fMRI. Trends Cogn Sci 2013;17:666-82. [Crossref] [PubMed]
- Wang Y, Wang WW, Hua XY, et al. Patterns of cortical reorganization in facial synkinesis: a task functional magnetic resonance imaging study. Neural Regen Res 2018;13:1637. [Crossref] [PubMed]
- Wu JJ, Lu YC, Zheng MX, et al. Motor Control Deficits in Facial Synkinesis Patients: Neuroimaging Evidences of Cerebral Cortex Involvement. Neural Plast 2019;2019:7235808. [Crossref] [PubMed]
- Lee J, Yang J, Li C, et al. Cortical Reorganization in Patients Recovered from Bell’s Palsy: An Orofacial and Finger Movements Task-State fMRI Study. Neural Plast 2016;2016:8231726. [Crossref] [PubMed]
- Song M, Jiang T. A review of functional magnetic resonance imaging for Brainnetome. Neurosci Bull 2012;28:389-98. [Crossref] [PubMed]
- Bullmore E, Sporns O. Complex brain networks: graph theoretical analysis of structural and functional systems. Nat Rev Neurosci 2009;10:186-98. [Crossref] [PubMed]
- VanderWerf F, Reits D, Smit AE, et al. Blink Recovery in Patients with Bell’s Palsy: A Neurophysiological and Behavioral Longitudinal Study. Invest Ophthalmol Vis Sci 2007;48:203. [Crossref] [PubMed]
- Veen MM, Quatela O, Tavares‐Brito J, et al. Patient‐perceived severity of synkinesis reduces quality of life in facial palsy: A cross‐sectional analysis in 92 patients. Clin Otolaryngol 2019;44:483-6. [Crossref] [PubMed]
- Wang J, Wang X, Xia M, et al. GRETNA: a graph theoretical network analysis toolbox for imaging connectomics. Front Hum Neurosci 2015;9:386. [PubMed]
- Pasquini L, Scherr M, Tahmasian M, et al. Link between hippocampus’ raised local and eased global intrinsic connectivity in AD. Alzheimers Dement 2015;11:475-84. [Crossref] [PubMed]
- Calhoun VD, Adali T, Pearlson GD, et al. A method for making group inferences from functional MRI data using independent component analysis. Hum Brain Mapp 2001;14:140-51. [Crossref] [PubMed]
- Damoiseaux JS, Rombouts SA, Barkhof F, et al. Consistent resting-state networks across healthy subjects. Proc Natl Acad Sci U S A 2006;103:13848-53. [Crossref] [PubMed]
- Yu Y, Zhou X, Wang H, et al. Small-World Brain Network and Dynamic Functional Distribution in Patients with Subcortical Vascular Cognitive Impairment. PLoS One 2015;10:e0131893. [Crossref] [PubMed]
- Watts DJ, Strogatz SH. Collective dynamics of ‘small-world’ networks. Nature 1998;393:440-2. [Crossref] [PubMed]
- He Y, Evans A. Graph theoretical modeling of brain connectivity. Curr Opin Neurol 2010;23:341-50. [PubMed]
- Wang JH, Zuo XN, Gohel S, et al. Graph Theoretical Analysis of Functional Brain Networks: Test-Retest Evaluation on Short- and Long-Term Resting-State Functional MRI Data. PLoS One 2011;6:e21976. [Crossref] [PubMed]
- Sporns O, Zwi JD. The Small World of the Cerebral Cortex. Neuroinformatics 2004;2:145-62. [Crossref] [PubMed]
- Achard S. A Resilient, Low-Frequency, Small -World Human Brain Functional Network with Highly Connected Association Cortical Hubs. J Neurosci 2006;26:63-72. [Crossref] [PubMed]
- Achard S, Bullmore E. Efficiency and Cost of Economical Brain Functional Networks. PLoS Comput Biol 2007;3:e17. [Crossref] [PubMed]
- Ravasz E, Barabási AL. Hierarchical organization in complex networks. Phys Rev E [Internet]. 2003. Available online: https://link.aps.org/doi/10.1103/PhysRevE.67.026112
- Supekar K, Musen M, Menon V. Development of Large-Scale Functional Brain Networks in Children. PLoS Biol 2009;7:e1000157. [Crossref] [PubMed]
- Barabási AL, Ravasz E, Oltvai Z. Hierarchical Organization of Modularity in Complex Networks. In: Pastor-Satorras R, Rubi M, Diaz-Guilera A, editors. Statistical Mechanics of Complex Networks. Berlin, Heidelberg: Springer Berlin Heidelberg; 2003:46-65.
- Newman MEJ. Assortative mixing in networks. Phys Rev Lett [Internet]. 2002. Available online: http://arxiv.org/abs/cond-mat/0205405
- He Y, Chen Z, Evans A. Structural Insights into Aberrant Topological Patterns of Large-Scale Cortical Networks in Alzheimer’s Disease. J Neurosci 2008;28:4756-66. [Crossref] [PubMed]
- Xu P, Huang R, Wang J, et al. Different topological organization of human brain functional networks with eyes open versus eyes closed. NeuroImage 2014;90:246-55. [Crossref] [PubMed]
- Genovese CR, Lazar NA, Nichols T. Thresholding of Statistical Maps in Functional Neuroimaging Using the False Discovery Rate1. Neuroimage 2002;15:870-8. [Crossref] [PubMed]
- Hu S, Wu Y, Li C, et al. Increasing functional connectivity of the anterior cingulate cortex during the course of recovery from Bell’s palsy. Neuroreport 2015;26:6-12. [Crossref] [PubMed]
- Klingner CM, Volk GF, Brodoehl S, et al. The effects of deefferentation without deafferentation on functional connectivity in patients with facial palsy. Neuroimage Clin 2014;6:26-31. [Crossref] [PubMed]
- Ma J, Hua XY, Zheng MX, et al. Structural remodeling secondary to functional remodeling in advanced-stage peripheral facial neuritis. Neurol Sci [Internet] 2020. Available online: http://link.springer.com/10.1007/s10072-020-04325-5
- Ballesta S, Mosher CP, Szep J, et al. Social determinants of eyeblinks in adult male macaques. Sci Rep 2016;6:38686. [Crossref] [PubMed]
- Hall A. The origin and purposes of blinking. Br J Ophthalmol 1945;29:445-67. [Crossref] [PubMed]
- Smit A, van der Geest J, Metselaar M, et al. Long-term changes in cerebellar activation during functional recovery from transient peripheral motor paralysis. Exp Neurol 2010;226:33-9. [Crossref] [PubMed]
- Klingner CM, Brodoehl S, Witte OW, et al. The impact of motor impairment on the processing of sensory information. Behav Brain Res 2019;359:701-8. [Crossref] [PubMed]
- van den Heuvel MP, Hulshoff Pol HE. Exploring the brain network: a review on resting-state fMRI functional connectivity. Eur Neuropsychopharmacol 2010;20:519-34. [Crossref] [PubMed]
- Buckner RL. The brain’s default network: origins and implications for the study of psychosis. Dialogues Clin Neurosci 2013;15:351-8. [Crossref] [PubMed]
- Otti A, Noll-Hussong M. Acupuncture-Induced Pain Relief and the Human Brains Default Mode Network - an Extended View of Central Effects of Acupuncture Analgesia. Forsch Komplementmed 2012;19:197-201. [Crossref] [PubMed]
- Salvador R, Suckling J, Coleman MR, et al. Neurophysiological Architecture of Functional Magnetic Resonance Images of Human Brain. Cereb Cortex 2005;15:1332-42. [Crossref] [PubMed]
- Bassett DS, Bullmore E, Verchinski BA, et al. Hierarchical Organization of Human Cortical Networks in Health and Schizophrenia. J Neurosci 2008;28:9239-48. [Crossref] [PubMed]
- Diedrichsen J. Neural Correlates of Reach Errors. J Neurosci 2005;25:9919-31. [Crossref] [PubMed]