Temporal dynamic changes of intrinsic brain activity in Alzheimer’s disease and mild cognitive impairment patients: a resting-state functional magnetic resonance imaging study
Introduction
Alzheimer’s disease (AD) is the most common form of dementia worldwide as well as the fifth leading cause of disability in people over the age of 65 (1). Clinically, AD manifests as memory impairment, cognitive degradation, and the deterioration of the individual’s ability to carry out daily living activities (2). Although some drugs can slow the progression of AD, this disease cannot currently be prevented or cured. Mild cognitive impairment (MCI) is considered to be a precursor for AD. Its onset is followed by progressive cognitive decline, but the basic everyday abilities of patients can largely be preserved (3). Many MCI patients eventually progress to AD, with an annual conversion rate of 10–15% (4). Therefore, it is essential that the pathological mechanisms of AD and MCI are illuminated and that individuals in the precursor stage of AD can be identified. Noninvasive methods based on positron emission tomography (PET), structural magnetic resonance imaging (sMRI), magnetic resonance spectroscopy (MRS), diffusion tensor imaging (DTI), task functional MRI(t-fMRI), and resting-state functional MRI (rs-fMRI) have provided diversified imaging tools for discriminating MCI from AD. PET has high sensitivity and specificity in the diagnosis of AD, but it is expensive. sMRI can provide an accurate index to evaluate the brain atrophy of patients, but the ability to accurately assess the severity of dementia is limited, the associations between clinical disease stages and brain tissue loss are not linear (5). While t-fMRI and rs-fMRI studied two completely different states of the brain, rs-fMRI has no request for subjects except to stay still, so it's especially suitable for older people who can't cooperate with task. Resting-state functional magnetic resonance imaging (rs-fMRI) is a widely recognized method for investigating intrinsic brain activity (IBA) and functional connectivity networks in AD (6). Rs-fMRI internal brain activity analysis can help diagnose early stages of diseases before brain atrophy occurs. By analyzing the correlation between brain activity changes and cognitive behavior (7), it helps to clarify the pathophysiological mechanism of AD.
The amplitude of low-frequency fluctuation (ALFF) measures the gross power of oscillations within a certain frequency range to detect changes of spontaneous activity of regional signals (8). Fractional ALFF (fALFF) is the ratio of the power spectrum of low-frequency (0.01–0.08 Hz), which can reduce or eliminate the effects of physiological noise (9). ALFF and fALFF have been widely used to explore IBA in AD (10-12). Ren et al. reported that MCI patients showed lower ALFF than healthy individuals in the anterior cingulate cortex, superior frontal gyrus, and medial prefrontal cortex after cognitive tasks (13), and Han et al. (14) showed that MCI patients had reduced ALFF and fALFF activity in the basal ganglia. Recently, Pan (12) observed that the posterior default mode network (DMN) and visual networks of MCI patients were hyperactivated, while and the spontaneous low-frequency brain activity of the salience network (SN) was decreased. Greicius et al. (15) suggested that the resting-state activity in the DMN was reduced in AD patients. Furthermore, Liu et al. (16) showed that AD patients had decreased ALFF values in the posterior cingulate cortex and inferior parietal lobe, and increased ALFF values in the hippocampus, and the middle and inferior temporal gyri. They believed the decline in ALFF values to be associated with memory impairment, while the increase in ALFF was interpreted as being a compensatory mechanism to improve memory. Nevertheless, previous studies on ALFF and fALFF are limited and have produced inconsistent results, rs-fMRI results are not enough to be shown to assess the severity of AD. We hope to find a more sensitive rs-fMRI marker to evaluate disease.
During MRI scanning, the dynamic features of brain activity are ignored by the static-state analysis of ALFF and fALFF. To date, few studies have focused on the local spontaneous neuronal activity and substantial fluctuation in cognitive dysfunction. Recent studies have reported that the regional dynamic characteristics of brain activity can be captured more effectively using the dynamic sliding window method throughout the scanning procedure (17). Compared to the static measurement tool, abnormal brain function can be examined more easily through dynamic analysis (18-21). The dynamic ALFF (dALFF) and dynamic fALFF (dfALFF) methods are recommended for measuring the variance of ALFF and fALFF over time. The dALFF and dfALFF methods provide a new way to show local brain activity during scanning (22), and dALFF has been applied in patients with schizophrenia, anxiety, depression, and sleep disturbance (20,21,23,24). However, further research on abnormal dynamic local brain activity in AD and MCI patients is required.
This study aimed to explore the dALFF and dfALFF of related brain regions in AD and MCI patients. The relationship between cognitive function and dALFF and dfALFF in the abnormal regions was evaluated by analyzing the correlation of the indicators with assessment scale score. We hypothesized that patients with AD and MCI would show different dALFF/dfALFF patterns compared to HC, and that such abnormalities could be regarded as imaging features that could distinguish patients with AD from individuals with MCI and HC.
We present the following article in accordance with the MDAR reporting checklist (available at http://dx.doi.org/10.21037/atm-20-7214).
Methods
Participants
One hundred and eleven AD patients and 29 MCI patients were recruited from the memory clinic of the Psychiatry Department, Zhejiang Provincial People’s Hospital. Additionally, 73 healthy controls (HC) were recruited from the Health Management Center. Written informed consent was obtained from all subjects before screening. The study was approved by the Ethics Committee of Zhejiang Provincial People’s Hospital (No. 2012KY002) and followed the requirements of the latest revision of the Declaration of Helsinki (as revised in 2013).
All study participants were right-handed and had undergone complete resting-state fMRI scanning and cognitive assessment according to the Mini-Mental State Examination (MMSE) and Montreal Cognitive Assessment (MoCA). None of the subjects had taken any anti-dementia medication previously. Patients with electron implants or various metals in their body, claustrophobia, significant psychiatric and neurological disorders, a history of drug or alcohol abuse, or other severe systemic diseases were excluded.
The AD patients met the diagnostic criteria of the National Institute of Neurologic and Communicative Disorders and Stroke and Alzheimer’s Disease. The MCI patients all met the Petersen’s criteria (25) as follows: a subjective or objective memory complaint; a slight decline in the ability to carry out activities of daily living; and mild abnormal cognitive function, rather than dementia. HC without any psychiatric or neurological disorders or cognitive complaints, and with MMSE scores ≥28, were recruited.
Acquisition of MRI data
MRI data were obtained using a General Electric 3.0 Tesla MR scanner (Discovery MR750 3.0T; GE Healthcare, Waukesha, WI, USA). High-resolution three-dimensional T1-weighted structural images were obtained using a sagittal magnetization-prepared rapid gradient echo (MPRAGE) sequence. The scanning parameters were as follows: repetition time (TR) =6.7 ms, echo time (TE) =2.9 ms, flip angle =12°, FOV =256×256 mm2, inversion time (TI) =450 ms, slice thickness/gap =1/0 mm, matrix =256×256, and slice number =192. The rs-fMRI data were obtained using the following echo-planar imaging (EPI) sequence: TR =2,000 ms, TE =30 ms, FOV =220×220 mm2, flip angle =90°, slice thickness/gap =3.2/0 mm, and slice number =44. During rs-fMRI, the subjects were instructed to close their eyes and to remain motionless and awake, and to refrain from thinking about a specific thing during the scanning sessions.
Data processing
The fMRI data were processed using the Data Processing and Analysis for Brain Imaging (DPABI) (DPABI V4.2, http://www.rfmri.org/dpabi) and Statistical Parametric Mapping (SPM12, http://www.fil.ion.ucl.ac.uk/spm/), and the custom code was written in MATLAB. The standard preprocessing steps included: elimination of the first 10 images; slice-timing correction and head motion correction; spatial normalization to the Montreal Neurological Institute (MNI) space; and re-sampling to 3×3×3 mm3 using a Gaussian kernel with a full-width at half-maximum of 6 mm for spatial smoothing, bandpass filtering (0.01–0.08 Hz), discarding of linear and quadratic trends, regression of covariates including the six head motion parameters, white matter, and cerebrospinal fluid signals. If head movement surpassed 2 mm and 2°, the data were excluded.
Estimation of dALFF and dfALFF
The temporal dynamic analysis toolbox of DPABI was used to analyze dALFF and dfALFF (17). Temporal dynamic patterns were analyzed with sliding window correlation (SWC). Previous studies have reported that the outcome of SWC is influenced by the selection of window length (26,27). To minimize spurious fluctuations, the target frequency interval should be 0–1/w Hz and the minimum window length should be above 1/fmin (28). The fmin is interpreted as the minimum frequency of the time series. Thus, a sliding window length of 50 TR (100 s) and a window step size of 5TR (10 s) were selected for the follow-up analysis and ALFF were individually calculated in each window (29). For the computation of ALFF, a fast Fourier transform (FFT) was used to convert each time course to the frequency domain. The square root of the power spectrum at each frequency was averaged across the filtered band (0.01–0.08 Hz). Then, fALFF was calculated by obtaining the ratio of the power spectrum of the low frequency to that of the entire frequency range. Next, window-based ALFF and fALFF maps were made from each time window. Based on the ALFF and fALFF maps, the mean and standard deviation were calculated for each voxel, and the standard deviation was divided by the mean to obtain the corresponding coefficient of variation (CV). The CV was used as the dALFF/dfALFF for more accurate temporal variability of absolute energy consumption in low-frequency regional brain activity between different individuals (24).
Statistical analyses
The chi-squared test was used to analyze sex differences. Other demographic characteristics and clinical behavior scores were analyzed using analysis of variance (ANOVA). P values of <0.05 were defined as supporting a strong trend towards statistical significance. All whole-brain dALFF and dfALFF data were analyzed by ANOVA to show the difference between the AD, MCI, and HC groups. Then, the abnormal brain regions between the groups were calculated using post-hoc analysis based on a two-sample t-test. Age, sex, and head motion were entered as nuisance covariates. The threshold for significance was set at P<0.05. After alphaSim corrections, the combined height threshold was P<0.005, with a cluster size of >25. Finally, the individual voxel threshold and cluster size were calculated using the Monte Carlo simulation to take consideration of individual differences.
Clinical correlation analyses
SPSS software (version 25.0; IBM, Chicago, IL, USA) was used to carry out clinical correlation analyses in order to explore correlations between the metrics measured and disease severity in patients with cognitive decline. The peak voxels of the abnormal regions were taken as the centers of the spheres, and spherical regions of interest (ROIs) with a radius of 6 mm were constructed. After the normality of the data had been assessed, Pearson’s correlation coefficients were calculated to obtain a better understanding of the relationship between ROIs (abnormal regions with the indices) and behavioral scores (MMSE and MoCA). A P value <0.05 (uncorrected) was considered to be statistically significant.
Results
Demographic and neuropsychological results
Analysis of the participants’ demographic data revealed no significant differences between the AD, MCI, and HC groups in terms of sex (χ2-test, P=0.27), age (Mann-Whitney H-test, P=0.284), or education level (Mann-Whitney H-test, P=0.723) (Table 1). However, the MMSE (Mann Whitney H-test, P<0.001) and MoCA (Mann Whitney H-test, P<0.001) scores of the three groups showed significant differences.
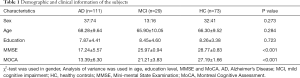
Full table
dALFF and dfALFF variability
The results of ANOVA revealed that participants in the AD, MCI, and HC groups exhibited significant dALFF variability in the bilateral cerebellar posterior lobe and left middle temporal gyrus (Table 2, Figure 1). Compared to the HC, the bilateral cerebellar posterior lobe and the left middle temporal gyrus were the main brain regions that exhibited high dALFF variability in the AD group (Figure 2). No significant differences were found in the dALFF between the MCI group and the HC group, and the same result was observed between the MCI group and the AD group.
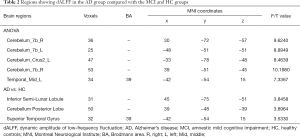
Full table
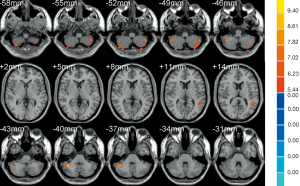
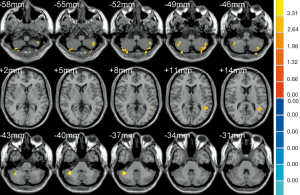
As shown in Table 3, the brain regions with significant dfALFF variability in the three groups were the left calcarine cortex and white matter (Figure 3). Compared to the MCI group, the AD group showed decreased dfALFF in the left calcarine cortex (Figure 4). No significant differences in dfALFF were found between the MCI group and the HC group, or between the AD group and the HC group.
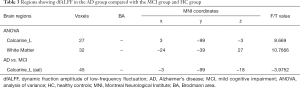
Full table
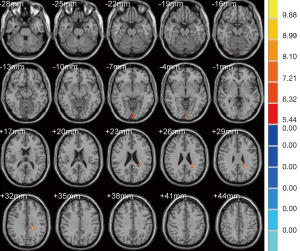
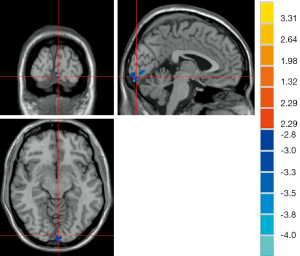
Correlation analysis results
No significant correlation was found between dALFF/dfALFF and clinical behavior scores.
Discussion
This study has pioneered the use of dALFF and dfALFF for the assessment of temporal variability of brain activity in AD and MCI patients. Compared to HC, patients with AD had increased dALFF variability in the cerebellar posterior lobe and the middle temporal lobe. Compared to the MCI patients, decreased dfALFF was observed in the left calcarine cortex of the AD patients. These results suggested that abnormal activity in specific brain regions could lead to the disruption of normal cognitive balance networks.
Previous rs-fMRI studies have suggested that the DMN is closely involved in episodic memory processing and regulation of emotion in patients with cognitive decline (30,31). A reduced ALFF/fALFF value in the medial prefrontal cortex (MPFC) (a part of the DMN) indicating cognitive decline (14), the posterior cingulate cortex (PCC) and MPFC exhibit less activity during cognitive tasks than the resting state (32), which means reduced activity in DMN is related to AD progression. However, a study by Wang et al. (33) suggested that MCI patients are characterized by enhanced lateral prefrontal regional homogeneity (ReHo) signaling. Qi et al. (34) reported that MCI patients also showed increased frontal-parietal activity. The above research indicated that decreased activity in some parts of the DMN may cause increased activity in others to compensate for the imbalance of cognitive activity (35). This study revealed the neuroimaging mechanism of AD, including the changes in the DMN and cerebrocerebellar circuits.
For a long time, the cerebellum was thought to engage only in motor and coordinated control; however, it also plays a critical role in the regulation of cognition and emotion. The cognitive cerebellum, which is located in lobules VI and VII in the cerebellar posterior lobe (36), has been found to connect to the critical nodes in the DMN; these include the cerebral cortex association areas, such as MPFC, temporo-parietal junction (TPJ), and hippocampal cortices (37,38). These brain region connections make up the cerebro-cerebellar circuits. Bai et al. found significantly lower FC exist cerebrocerebellar circuits, which is closely related to the decline of episodic memory (39). AD symptoms are associated with significant and anatomically-distinct atrophy in the cerebellum (40), and damage to the cerebellar posterior lobe can result in the clinical cerebellar cognitive affective syndrome (CCAS) (41). Also, a significant reduction of cerebral blood flow and deposits of amyloid plaque have been found in the cerebellum of AD patients (42,43). All types of hypofunction caused by AD are closely related to the cerebellar posterior lobe. Studies have confirmed that language processing and reading tasks activate the right cerebellar posterior lobe (44,45), whereas in spatial processing tasks, the left posterior cerebellum is activated more (46,47). Working memory paradigms activate the bilateral cerebellar posterior regions, particularly in lobules VI and VII (48-50). Complex executive function tasks engage cerebellar lobules VI, Crus I, and VIIB (51,52), and cerebellar activation has also been observed during emotional processing (53,54). Therefore, when cognitive decline, the cerebellum closely related to cognitive function also changes, and showed an increase in dALFF in dynamic indicators.
As part of the DMN, the temporal lobe processes auditory information and is also associated with memory and emotion. The middle temporal gyrus is responsible for language and semantic memory processes (55) and usually is attacked by nerve tangles in patients with early-stage AD (56). Firbank et al. (57) also reported increased apparent diffusion coefficient (ADC) in the left temporal lobe of AD patients, which may indicate damage to microstructures. It can be inferred that pathological damage can lead to the destruction of local microstructures and abnormal compensatory activity. The AD patients in our study showed increased dALFF variability in the cerebellar posterior lobe and the middle temporal gyrus, which suggested that aberrant fluctuations of local brain activity occurred in these regions. These abnormal variabilities may reduce the capacity of the cerebellum to participate in the cerebrocerebellar circuits and DMN in the regulation of cognition and emotion in AD. No significant differences were found between the brain regions of the MCI and HC patients. Abnormally increased cerebellar activities could be recognized as dementia-specific processes.
The calcarine cortex is a component of the visual cortices. In one study, calcium cortex activity was reported to be strongly related to spatial working memory response time (58). Another study found that AD patients showed lower connectivity between the medial prefrontal cortex and the visual cortices (59). Abnormal dfALFF values of the left calcarine cortex were also found between the AD, MCI, and HC groups in our study. Functional connectivity deficits and abnormal dfALFF of the visual cortex may result in a decline in cognitive task processing and lead to memory impairment. Compared to that in MCI patients, the dfALFF value of the left calcarine cortex was observed to be decreased in AD patients. This could be a self-adaptive or decompensation mechanism, more research is needed to explain the mechanism.
Previous rs-fMRI studies have acquiesced that brain activity and the connectivity of networks remain stationary over the whole fMRI scan length. In our study, by using the ALFF and fALFF methods combined with sliding window correlation, we showed that the activity of brain areas, which including the cerebellar posterior lobe, temporal gyrus and the calcarine cortex, have changed throughout the scan in AD patients. A few studies deemed that the temporal variability of brain activity was regarded as the stability of time-varying brain connectivity within a customized time window. Increased temporal variability represented a compensatory mechanism, temporarily maintaining the balance of brain function (21). However, not all compensatory activities are meaningful. Increased temporal variability may also indicate the temporal abnormity and instability in the resting state (60), causing a decline in the ability of the associated brain regions to participate in the normal cognitive loop. As the brain adapts, the compensation decreases later.
Limitations
This study has several limitations. First, although we recruited three groups of dementia patients, this study inevitably remains cross-sectional. A longitudinal study on dALFF and dfALFF changes in patients with later stages of the disease should be carried out in the future. Recruiting patients with subjective memory complaints, unimpaired cognition, and following up. Second, only the total cognitive level was estimated, and a subanalysis of the cognitive assessment scale scores was not performed. The influence of the defect may cause the subtests to be dropped in the summation of the aggregate score. For future studies, we will assess the cognitive function of patients using more neurocognitive scales. Third, the parametric analysis of dALFF/dfALFF variance was only conducted in the window length of 50 TRs and step size of 5 TRs; therefore, research is needed to identify more potential parameter permutations that could help us to determine the detailed pathophysiological mechanisms of dALFF/dfALFF in AD and MCI patients. Fourth, we only used single technique to investigate this problem. In fact, there are many other noninvasive methods can help us better understand AD and MCI. For example, the combination of rs-fMRI and DTI can comprehensively analyze the functional and structural changes of gray matter and white matter in each brain area. Yan et al. (61) used a machine learning classification method based on a multimodal support vector machine (SVM) to integrate the data information of rs-fMRI and DTI. Finally, they achieved an accuracy of 98.58% in the AD group, 97.76% in the amnestic MCI group, and 80.24% in the subjective cognitive decline (SCD) group. In other studies, rs-fMRI and PET(62), sMRI (63) are combined for research. Based on these neuroimaging modalities have complementary information, multi-modality imaging can be more comprehensive for MCI and AD patients, and its accuracy of disease diagnosis will be improved compared with a single pattern (64). In the foreseeable future, With the rapid development of artificial intelligence (AI), using multi-modality imaging will be a new research trend.
Conclusions
In summary, the enhancive temporal variability of dALFF in the cerebellar and temporal lobes existed in AD patients. These increased dALFF variability suggested that the temporal abnormity and instability in the resting state, which lead to the destruction of related cognitive circuits involving the DMN and the cerebellar circuits. Declined dfALFF variability in AD patients was an indicator of the decompensation of visual cortex function in the later stage of cognitive decline. Our method could be potential neural biomarkers of AD, which could predict the temporal variability of brain activity in AD. By describing dynamic changes of intrinsic brain activity, we offer a novel way of clarifying the pathophysiological mechanism of AD. However, validation with more studies and multimodal data will be necessary to certainly establish a link between the severity of AD and activity within the brain.
Acknowledgments
I thank Dr. Xiaozheng Liu for helping me with data processing and analyses.
Funding: This pilot study was funded by the National Natural Science Foundation of China (No. 81771158), Science Foundation of the Health Commission of Zhejiang Province (Nos. 2019321345).
Footnote
Reporting Checklist: The authors have completed the MDAR reporting checklist. Available at http://dx.doi.org/10.21037/atm-20-7214
Data Sharing Statement: Available at http://dx.doi.org/10.21037/atm-20-7214
Conflicts of Interest: All authors have completed the ICMJE uniform disclosure form (available at http://dx.doi.org/10.21037/atm-20-7214). The authors have no conflicts of interest to declare.
Ethical Statement: The authors are accountable for all aspects of the work in ensuring that questions related to the accuracy or integrity of any part of the work are appropriately investigated and resolved. The study was approved by the Ethics Committee of Zhejiang Provincial People’s Hospital (No. 2012KY002) and followed the requirements of the latest revision of the Declaration of Helsinki (as revised in 2013). Informed consent was obtained from all subjects.
Open Access Statement: This is an Open Access article distributed in accordance with the Creative Commons Attribution-NonCommercial-NoDerivs 4.0 International License (CC BY-NC-ND 4.0), which permits the non-commercial replication and distribution of the article with the strict proviso that no changes or edits are made and the original work is properly cited (including links to both the formal publication through the relevant DOI and the license). See: https://creativecommons.org/licenses/by-nc-nd/4.0/.
References
- Alzheimer’s Association. 2020 Alzheimer's disease facts and figures. Alzheimer's Dement 2020;16:391-460. [Crossref]
- Jaffe RJ, Dave RS, Byrareddy SN. Meningeal lymphatics in aging and Alzheimer's disease. Ann Transl Med 2019;7:S2. [Crossref] [PubMed]
- Langa KM, Levine DA. The diagnosis and management of mild cognitive impairment: a clinical review. JAMA 2014;312:2551-61. [Crossref] [PubMed]
- Eshkoor SA, Hamid TA, Mun CY, et al. Mild cognitive impairment and its management in older people. Clin Interv Aging 2015;10:687-93. [Crossref] [PubMed]
- Filippi M, Agosta F, Frisoni GB, et al. Magnetic resonance imaging in Alzheimer's disease: from diagnosis to monitoring treatment effect. Curr Alzheimer Res 2012;9:1198-209. [Crossref] [PubMed]
- Barkhof F, Haller S, Rombouts SA. Resting-state functional MR imaging: a new window to the brain. Radiology 2014;272:29-49. [Crossref] [PubMed]
- Fox MD, Snyder AZ, Vincent JL, et al. The human brain is intrinsically organized into dynamic, anticorrelated functional networks. Proc Natl Acad Sci U S A 2005;102:9673-8. [Crossref] [PubMed]
- Zang YF, He Y, Zhu CZ, et al. Altered baseline brain activity in children with ADHD revealed by resting-state functional MRI. Brain Dev 2007;29:83-91. [Crossref] [PubMed]
- Zou QH, Zhu CZ, Yang Y, et al. An improved approach to detection of amplitude of low-frequency fluctuation (ALFF) for resting-state fMRI: fractional ALFF. J Neurosci Methods 2008;172:137-41. [Crossref] [PubMed]
- Dai Z, Yan C, Wang Z, et al. Discriminative analysis of early Alzheimer's disease using multi-modal imaging and multi-level characterization with multi-classifier (M3). Neuroimage 2012;59:2187-95. [Crossref] [PubMed]
- He Y, Wang L, Zang Y, et al. Regional coherence changes in the early stages of Alzheimer's disease: a combined structural and resting-state functional MRI study. Neuroimage 2007;35:488-500. [Crossref] [PubMed]
- Pan P, Zhu L, Yu T, et al. Aberrant spontaneous low-frequency brain activity in amnestic mild cognitive impairment: A meta-analysis of resting-state fMRI studies. Ageing Res Rev 2017;35:12-21. [Crossref] [PubMed]
- Ren P, Heffner KL, Jacobs A, et al. Acute Affective Reactivity and Quality of Life in Older Adults with Amnestic Mild Cognitive Impairment: A Functional MRI Study. Am J Geriatr Psychiatry 2017;25:1225-33. [Crossref] [PubMed]
- Han Y, Wang J, Zhao Z, et al. Frequency-dependent changes in the amplitude of low-frequency fluctuations in amnestic mild cognitive impairment: a resting-state fMRI study. Neuroimage 2011;55:287-95. [Crossref] [PubMed]
- Greicius MD, Srivastava G, Reiss AL, et al. Default-mode network activity distinguishes Alzheimer's disease from healthy aging: evidence from functional MRI. Proc Natl Acad Sci U S A 2004;101:4637-42. [Crossref] [PubMed]
- Liu X, Wang S, Zhang X, et al. Abnormal amplitude of low-frequency fluctuations of intrinsic brain activity in Alzheimer's disease. J Alzheimers Dis 2014;40:387-97. [Crossref] [PubMed]
- Yan C-G, Yang Z, Colcombe SJ, et al. Concordance among indices of intrinsic brain function: Insights from inter-individual variation and temporal dynamics. Science Bulletin 2017;62:1572-84. [Crossref]
- Kim J, Criaud M, Cho SS, et al. Abnormal intrinsic brain functional network dynamics in Parkinson's disease. Brain 2017;140:2955-67. [Crossref] [PubMed]
- Zhou Q, Zhang L, Feng J, et al. Tracking the Main States of Dynamic Functional Connectivity in Resting State. Front Neurosci 2019;13:685. [Crossref] [PubMed]
- Cui Q, Sheng W, Chen Y, et al. Dynamic changes of amplitude of low-frequency fluctuations in patients with generalized anxiety disorder. Hum Brain Mapp 2020;41:1667-76. [Crossref] [PubMed]
- Li K, Luo X, Zeng Q, et al. Interactions between sleep disturbances and Alzheimer's disease on brain function: a preliminary study combining the static and dynamic functional MRI. Sci Rep 2019;9:19064. [Crossref] [PubMed]
- Liao W, Li J, Ji GJ, et al. Endless Fluctuations: Temporal Dynamics of the Amplitude of Low Frequency Fluctuations. IEEE Trans Med Imaging 2019;38:2523-32. [Crossref] [PubMed]
- Fu Z, Tu Y, Di X, et al. Characterizing dynamic amplitude of low-frequency fluctuation and its relationship with dynamic functional connectivity: An application to schizophrenia. Neuroimage 2018;180:619-31. [Crossref] [PubMed]
- Yu Y, Li Z, Lin Y, et al. Depression Affects Intrinsic Brain Activity in Patients With Mild Cognitive Impairment. Front Neurosci 2019;13:1333. [Crossref] [PubMed]
- Petersen RC, Smith GE, Waring SC, et al. Mild cognitive impairment: clinical characterization and outcome. Arch Neurol 1999;56:303-8. [Crossref] [PubMed]
- Sakoğlu U, Pearlson GD, Kiehl KA, et al. A method for evaluating dynamic functional network connectivity and task-modulation: application to schizophrenia. Magma 2010;23:351-66. [Crossref] [PubMed]
- Shakil S, Lee CH, Keilholz SD. Evaluation of sliding window correlation performance for characterizing dynamic functional connectivity and brain states. Neuroimage 2016;133:111-28. [Crossref] [PubMed]
- Li R, Liao W, Yu Y, et al. Differential patterns of dynamic functional connectivity variability of striato-cortical circuitry in children with benign epilepsy with centrotemporal spikes. Hum Brain Mapp 2018;39:1207-17. [Crossref] [PubMed]
- Leonardi N, Van De Ville D. On spurious and real fluctuations of dynamic functional connectivity during rest. Neuroimage 2015;104:430-6. [Crossref] [PubMed]
- Raichle ME, MacLeod AM, Snyder AZ, et al. A default mode of brain function. 2001;98:676-82.
- Xie X, Mulej Bratec S, Schmid G, et al. How do you make me feel better? Social cognitive emotion regulation and the default mode network. Neuroimage 2016;134:270-80. [Crossref] [PubMed]
- Greicius MD, Krasnow B, Reiss AL, et al. Functional connectivity in the resting brain: a network analysis of the default mode hypothesis. Proc Natl Acad Sci U S A 2003;100:253-8. [Crossref] [PubMed]
- Wang P, Li R, Yu J, et al. Frequency-Dependent Brain Regional Homogeneity Alterations in Patients with Mild Cognitive Impairment during Working Memory State Relative to Resting State. Front Aging Neurosci 2016;8:60. [Crossref] [PubMed]
- Qi Z, Wu X, Wang Z, et al. Impairment and compensation coexist in amnestic MCI default mode network. Neuroimage 2010;50:48-55. [Crossref] [PubMed]
- Zhao Z, Lu J, Jia X, et al. Selective changes of resting-state brain oscillations in aMCI: an fMRI study using ALFF. Biomed Res Int 2014;2014:920902. [Crossref] [PubMed]
- Stoodley CJ, Schmahmann JD. Evidence for topographic organization in the cerebellum of motor control versus cognitive and affective processing. Cortex 2010;46:831-44. [Crossref] [PubMed]
- Van Overwalle F, Mariën P. Functional connectivity between the cerebrum and cerebellum in social cognition: A multi-study analysis. Neuroimage 2016;124:248-55. [Crossref] [PubMed]
- Iglói K, Doeller CF, Paradis AL, et al. Interaction Between Hippocampus and Cerebellum Crus I in Sequence-Based but not Place-Based Navigation. Cereb Cortex 2015;25:4146-54. [Crossref] [PubMed]
- Bai F, Zhang Z, Watson DR, et al. Abnormal functional connectivity of hippocampus during episodic memory retrieval processing network in amnestic mild cognitive impairment. Biol Psychiatry 2009;65:951-8. [Crossref] [PubMed]
- Guo CC, Tan R, Hodges JR, et al. Network-selective vulnerability of the human cerebellum to Alzheimer's disease and frontotemporal dementia. Brain 2016;139:1527-38. [Crossref] [PubMed]
- Hoche F, Guell X, Vangel MG, et al. The cerebellar cognitive affective/Schmahmann syndrome scale. Brain 2018;141:248-70. [Crossref] [PubMed]
- Hauser T, Schönknecht P, Thomann PA, et al. Regional cerebral perfusion alterations in patients with mild cognitive impairment and Alzheimer disease using dynamic susceptibility contrast MRI. Acad Radiol 2013;20:705-11. [Crossref] [PubMed]
- Sepulveda-Falla D, Matschke J, Bernreuther C, et al. Deposition of hyperphosphorylated tau in cerebellum of PS1 E280A Alzheimer's disease. Brain Pathol 2011;21:452-63. [Crossref] [PubMed]
- Jansen A, Flöel A, Van Randenborgh J, et al. Crossed cerebro-cerebellar language dominance. Hum Brain Mapp 2005;24:165-72. [Crossref] [PubMed]
- McDermott KB, Petersen SE, Watson JM, et al. A procedure for identifying regions preferentially activated by attention to semantic and phonological relations using functional magnetic resonance imaging. Neuropsychologia 2003;41:293-303. [Crossref] [PubMed]
- Stoodley CJ, Schmahmann JD. Functional topography in the human cerebellum: a meta-analysis of neuroimaging studies. Neuroimage 2009;44:489-501. [Crossref] [PubMed]
- Hanakawa T, Dimyan MA, Hallett M. Motor planning, imagery, and execution in the distributed motor network: a time-course study with functional MRI. Cereb Cortex 2008;18:2775-88. [Crossref] [PubMed]
- Chen SH, Desmond JE. Cerebrocerebellar networks during articulatory rehearsal and verbal working memory tasks. Neuroimage 2005;24:332-8. [Crossref] [PubMed]
- Hayter AL, Langdon DW, Ramnani N. Cerebellar contributions to working memory. Neuroimage 2007;36:943-54. [Crossref] [PubMed]
- Kirschen MP, Chen SH, Schraedley-Desmond P, et al. Load- and practice-dependent increases in cerebro-cerebellar activation in verbal working memory: an fMRI study. Neuroimage 2005;24:462-72. [Crossref] [PubMed]
- Schall U, Johnston P, Lagopoulos J, et al. Functional brain maps of Tower of London performance: a positron emission tomography and functional magnetic resonance imaging study. Neuroimage 2003;20:1154-61. [Crossref] [PubMed]
- Lie CH, Specht K, Marshall JC, et al. Using fMRI to decompose the neural processes underlying the Wisconsin Card Sorting Test. Neuroimage 2006;30:1038-49. [Crossref] [PubMed]
- Bermpohl F, Pascual-Leone A, Amedi A, et al. Dissociable networks for the expectancy and perception of emotional stimuli in the human brain. Neuroimage 2006;30:588-600. [Crossref] [PubMed]
- Hofer A, Siedentopf CM, Ischebeck A, et al. Sex differences in brain activation patterns during processing of positively and negatively valenced emotional words. Psychol Med 2007;37:109-19. [Crossref] [PubMed]
- Cabeza R, Nyberg L. Neural bases of learning and memory: functional neuroimaging evidence. Curr Opin Neurol 2000;13:415-21. [Crossref] [PubMed]
- Piras IS, Krate J, Delvaux E, et al. Association of AEBP1 and NRN1 RNA expression with Alzheimer's disease and neurofibrillary tangle density in middle temporal gyrus. Brain Res 2019;1719:217-24. [Crossref] [PubMed]
- Firbank MJ, Blamire AM, Krishnan MS, et al. Diffusion tensor imaging in dementia with Lewy bodies and Alzheimer's disease. Psychiatry Res 2007;155:135-45. [Crossref] [PubMed]
- Valenzuela MJ, Turner AJ, Kochan NA, et al. Posterior compensatory network in cognitively intact elders with hippocampal atrophy. Hippocampus 2015;25:581-93. [Crossref] [PubMed]
- Zhang HY, Wang SJ, Liu B, et al. Resting brain connectivity: changes during the progress of Alzheimer disease. Radiology 2010;256:598-606. [Crossref] [PubMed]
- Zhou F, Zhan J, Gong T, et al. Characterizing Static and Dynamic Fractional Amplitude of Low-Frequency Fluctuation and its Prediction of Clinical Dysfunction in Patients with Diffuse Axonal Injury. Academic Radiology 2020. [Crossref] [PubMed]
- Yan T, Wang Y, Weng Z, et al. Early-Stage Identification and Pathological Development of Alzheimer's Disease Using Multimodal MRI. J Alzheimers Dis 2019;68:1013-27. [Crossref] [PubMed]
- Marchitelli R, Aiello M, Cachia A, et al. Simultaneous resting-state FDG-PET/fMRI in Alzheimer Disease: Relationship between glucose metabolism and intrinsic activity. Neuroimage 2018;176:246-58. [Crossref] [PubMed]
- Hojjati SH, Ebrahimzadeh A, Khazaee A, et al. Predicting conversion from MCI to AD by integrating rs-fMRI and structural MRI. Comput Biol Med 2018;102:30-9. [Crossref] [PubMed]
- Liu X, Chen K, Wu T, et al. Use of multimodality imaging and artificial intelligence for diagnosis and prognosis of early stages of Alzheimer's disease. Transl Res 2018;194:56-67. [Crossref] [PubMed]