Development and validation of magnetic resonance imaging-based radiomics models for preoperative prediction of microsatellite instability in rectal cancer
Introduction
Rectal cancer is one of the most common cancers worldwide and has high rates of morbidity and mortality (1). In the last decade, significant progress in the treatment of locally advanced rectal cancer has been achieved via neoadjuvant chemoradiotherapy (2), followed by total mesorectal excision (TME) (3). However, responses to chemotherapy and prognoses of rectal cancer patients are quite different, even for patients in the same pathological stage. The most plausible explanation for these multifarious clinical outcomes is associated with the strong heterogeneity both between and inside tumors (4).
Microsatellite instability (MSI), which is frequently analyzed by testing the loss of one or more mismatch repair (MMR) proteins by immunohistochemistry (IHC), serves not only as a predictive biomarker for responses to chemotherapy but also as a prognostic biomarker for clinical outcomes (5-7). To date, there is considerable evidence that suggests that patients with stage II rectal cancer with high-frequency MSI (MSI-H) obtain no benefit from 5-fluorouracil (5-FU)-based adjuvant therapy (8,9), and these patients have shown a significantly better prognosis than those characterized by microsatellite stability (MSS) (10,11). Therefore, since 2016, the National Comprehensive Cancer Network (NCCN) guidelines have recommended MSI evaluation for all patients with stage II rectal cancer (12). Furthermore, since MSI determines whether rectal cancer patients respond well to immunotherapy, the European Society for Medical Oncology (ESMO) also recommended MSI evaluation for cancer immunotherapy in 2019 (13).
Microsatellite status is currently assessed by genetic or IHC analyses of biopsies; these procedures are considered the gold standard in clinical practice but still present two challenges. First, since tumors have spatial and temporal heterogeneities (14), the results of MSI evaluation may vary depending on where and when the specimens are obtained. Second, the risks of invasive sampling and potential complications limit the application of these methods for real-time monitoring of disease progression and biological characteristics of the tumor (15). Thus, the development of a method that is noninvasive, displays convenient repeatability, and reflects intratumoral heterogeneity to help identify microsatellite status is crucial to provide an adjunct to histological assessment in real time.
Radiomics analysis, in which several high-throughput quantitative features are extracted from radiological images, provides valuable information associated with tumoral heterogeneity (16). This method has recently gained attention as a promising tool for predicting prognosis and guiding clinical decision-making (17,18). Recent studies on rectal cancer have provided encouraging evidence that radiomics can be applied to predict characteristics including therapeutic responses (19,20), lymph node metastasis (21,22), and KRAS mutations (23,24). Three recent studies, including all left- and right-sided colorectal cancers, reported that radiomics analysis based on computed tomography (CT) data could predict MSI in colorectal cancer (25-27); however, MSI prevalence in colorectal cancer is not evenly distributed, and it gradually varies from the proximal colon to the rectum (28). Furthermore, magnetic resonance imaging (MRI) has been recommended by both NCCN (29) and ESMO (30) guidelines as the preferred imaging examination for rectal cancer in clinical practice. However, to the best of our knowledge, no study to date has reported whether the MRI-based image signature is associated with the MSI status in rectal cancer.
We hypothesize that if MRI-based imaging features associated with MSI status can be determined, they could provide noninvasive clinical predictors in patients with rectal cancer. Therefore, this retrospective study performed a radiomics analysis to identify MRI-based image biomarkers of MSI in rectal cancer patients.
We present the following article in accordance with the TRIPOD reporting checklist (available at http://dx.doi.org/10.21037/atm-20-7673).
Methods
Study population
This study was conducted in accordance with the Declaration of Helsinki (as revised in 2013), and was approved by the Medical Ethics Committee of West China Hospital (No. 2019-1159). Individual informed consent for this retrospective analysis was waived. Between January 2016 and May 2019, 715 consecutive patients with histopathologically confirmed rectal cancer who underwent preoperative MRI examinations were initially retrieved. Of these, 224 patients were excluded for the following reasons: (I) without MSI evaluation (n=87); (II) receiving chemoradiotherapy before MRI (n=82); (III) small tumors (<5 mm) that are difficult to identify on images (n=9); (IV) insufficient T2-weighted imaging quality to draw the region of interest (ROI), such as an obvious motion artifact caused by respiration or intestinal peristalsis (n=18); and (V) mucinous adenocarcinoma (n=28). Finally, 491 patients were retrospectively enrolled in this study.
Clinical and laboratory variables
Clinical variables comprised age, sex, and T-stage based on MR (MR-T stage). Laboratory analysis included carcinoembryonic antigen (CEA) and carbohydrate antigen 19-9 (CA19-9), which had threshold values of 3.4 ng/mL and 22 U/mL, respectively. IHC for MMR proteins, including mutL homologue 1 (MLH1), mutS homologue 2 (MSH2), mutS homologue 6 (MSH6), and postmeiotic segregation increased 2 (PMS2), was performed using the standard streptavidin–biotin–peroxidase procedure. Tumors displaying loss of an MMR protein were collectively referred as defective mismatch repair (dMMR) and expected to have MSI-H, whereas those with intact MMR proteins were classified as proficient mismatch repair (pMMR) and expected to have MSS or MSI-low (MSI-L). Considering the Bethesda guidelines for colorectal cancers suggest that the terms MSI-H or MSI-L should be discontinued and MSI-L tumors included with MSS tumors (13), we regarded MSI-H as MSI and MSI-L as MSS in our study.
MRI protocol
All of the patients underwent preoperative rectal MRI on a 3T Magnetom Skyra MR scanner (Siemens Healthineers, Malvern, PA, USA) with a phased-array 18-channel body coil in the supine position. MRI protocols did not change during the 41-month study. Two rectal suppository pills were inserted to remove feces at 1 hour before MRI, and 10 mg of racanisodamine hydrochloride was injected intravenously to reduce rectal motility (unless contraindicated) at 30 min before MRI. High-resolution rectal MRI protocol comprised turbo spin echo sagittal, oblique coronal (angulated parallel to the long axis of the rectal tumor), and oblique axial (angulated perpendicular to the long axis of the rectal tumor) T2- and diffusion-weighted imaging using readout-segmented echo-planar imaging of long variable echo trains. The scan parameters used for the oblique axial T2-weighted imaging sequence were as follows: repetition time/echo time, 6,890/100; slice thickness, 3 mm; voxel size, 0.3×0.3×3 mm; field of view, 180 mm; matrix, 384×346; slices, 48; average, 3; total scanning time, 5 min and 5 s; and parallel acquisition technique with generalized auto-calibrating partial parallel acquisition acceleration factor, 2. Fat saturation techniques were not required.
Tumor segmentation
MR images were retrieved from the picture archiving and communication system to a local workstation for image segmentation and analysis. A radiologist with more than 10 years of experience in abdominal imaging manually segmented the tumor region volumetrically on all oblique axial (angulated perpendicular to the long axis of the rectal tumor) T2-weighted imaging slices using the ITK-SNAP software (v3.6.0, http://www.itksnap.org). When the boundary was uncertain, another radiologist with 20 years of experience in abdominal diagnosis was consulted for a final decision. Both radiologists were blinded to all clinical and pathological findings. Meanwhile, the necrosis area, intraluminal air regions, and uninvaded rectal wall were excluded from the ROIs with caution.
Radiomics analysis
Feature extraction
The radiomics features from the manually segmented tumor region were automatically extracted using an open-source Python package (PyRadiomics version 2.1.2, https://github.com/Radiomics/pyradiomics) (31). Three groups of radiomics features were extracted: (I) shape features (n=14); (II) first-order intensity statistics features (n=288); and (III) texture features [Gray Level Co-occurrence Matrix (n=336), Gray Level Dependence Matrix (GLDM, n=224), Gray Level Run Length Matrix (GLRLM, n=256), Gray Level Size Zone Matrix (n=256), and Neighboring Gray Tone Difference Matrix (n=80)]. Ultimately, 1,454 radiomics features were extracted from the manually-labeled ROI regions; all of these features had generally been used in previous radiomics studies (32,33).
Inter-observer reproducibility evaluation
To ensure the reproducibility and accuracy of radiomics features extracted from MR imaging, 50 MR scans were randomly selected for double-blind manual segmentation by two radiologists. The radiomics features from the 50 paired segmentation results were automatically extracted, and the inter- and intra-observer agreement was evaluated using intraclass correlation coefficients (ICC). In related research, an ICC of >0.75 was interpreted as being indicative of almost perfect agreement (34).
Feature selection
Extracted radiomics features that met the criteria of having an ICC of >0.75 were used for further analysis. To prevent overfitting of the model, we applied a two-step feature selection method. First, we used the two-sample t test to select differential features between MSS and MSI groups in the training cohort (35). Key radiomics features that were most closely associated with the determination of colorectal cancer MSI status were then selected from the differential features using the Least Absolute Shrinkage and Selection Operator (LASSO) algorithm (36). Finally, six features were selected after dimension reduction, including textural (two GLRLM features and three GLDM features), and first-order statistics (one feature) features. The textural features included regular texture features such as GLDM and GLRLM, and first-order statistics reflected the distribution of voxel intensities within the region.
The feature extraction and selection process were implemented using Python 3.6.0 (www.python.org).
Development of the radiomics models
Based on the selected radiomics features and clinical variables, we used the XGBoost classifier (37) to develop the radiomics clinical model (RC model), the radiomics imaging model (RI model), and the combined radiomics model (RIC model), incorporating both the radiomics signature and independent clinical characteristics. The development of the RC model was conducted with the following five clinical variables: sex, age, MR-T stage, CEA, and CA19-9. The RI model was constructed with six selected radiomics features. The RIC model was established with the combination of six radiomics features plus five clinical variables. Since there were considerably more MSS patients than MSI patients in this study (440 MSS vs. 51 MSI), the weights of MSI and MSS class were adjusted according to their inversely proportional prevalence to minimize the class imbalance problem. The detailed parameters of model development are summarized in the supplementary files (Appendix 1). The development and validation of these models were performed using InferScholar platform version 3.1 (InferVision, Beijing, China).
Statistical analysis
To evaluate the capacity of the predictive models for the discrimination of the MSI from MSS tumors in the training and validation cohorts, a receiver operating characteristic (ROC) curve was plotted for the calculation of sensitivity and specificity, and the area under the curve (AUC) was also quantified. Sensitivity was determined by TP/(TP + FN) and specificity was computed by TN/(FP + TN), where TP, FP, FN, and TN refer to true positive, false positive, false negative, and true negative, respectively. The maximum value of the AUC is 1.0, indicating a perfect discrimination, while 0.5 indicates a very poor model with a random chance of correctly discriminating the outcome. Generally, an AUC of >0.7 indicates a good model (38).
The Mann-Whitney U test was performed to evaluate the differences in variables with a continuous distribution across categories. The association between categorical variables was accessed using the Chi-square test or Fisher’s exact test. Delong’s test was used to compare the diagnostic performance of the different models (39). All tests were two-sided, and P<0.05 was considered statistically significant. All analyses were performed using Prism 5 for Windows (Version 5.01) and MedCalc (Version 18.11.3).
Results
Study design and patient characteristics
Among the 491 patients, there were 318 men and 173 women with a median age of 61 years (range, 21–91 years). Based on the analysis of the MMR protein, the patients were classified into two groups: an MSI group (n=51) and an MSS group (n=440). The prevalence of MSI was 10.39% (51/491). The patients were randomly divided into a training cohort (291 MSS and 36 MSI-H, n=327) and a validation cohort (149 MSS and 15 MSI-H, n=164). The RC, RI, and RIC models were applied in the training cohort, and their performances were evaluated in the validation cohort (Figure 1).
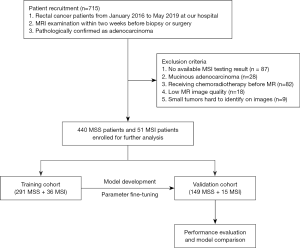
There were no significant differences between the two cohorts in terms of MSI-H prevalence (11.01% and 9.15% in the training and validation cohorts, respectively, P=0.733). Furthermore, there were no notable differences in sex, age, MR-T category, CEA, and CA199 between the MSI and MSS groups in the training and validation cohorts (all P values >0.05). The patients’ demographic and clinical data are shown in Table 1.
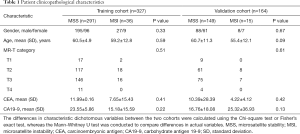
Full table
Radiomics feature selection and model construction
The study framework is shown in Figure 2A, and the architecture of the combined radiomics model incorporating both the radiomics signature and independent clinical characteristics is shown in Figure 2B. Briefly, radiomics features were first extracted from the manual segmentation region following inter-observer reproducibility evaluation. The two-sample t test and LASSO regression methods were applied to determine the most useful predictive features that were commonly applied in the regression of high-dimensional data, and the six most important features were selected to build the radiomics models (Figure 3). Finally, the diagnostic efficiency of the RC, RI, and RIC models were evaluated by analyzing the ROC curve. The six selected radiomics features showed excellent inter-reader agreement, with ICCs ranging from 0.8924 to 0.9607 (Table 2), and the feature heatmap was plotted according to the normalized radiomics feature values (Figure 4).
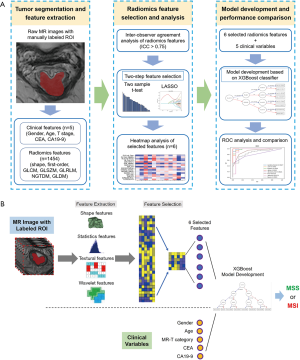
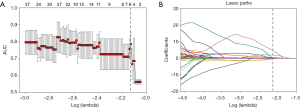
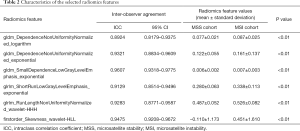
Full table

Development and validation of the radiomics models
ROC analysis was performed to determine the diagnostic performance of the RC, RI, and RIC models with respect to the AUC. The predictive score of the RC, RI, and RIC models for each patient in the validation cohort is shown in Figure 5. The AUC of the RC model was 0.756 [95% confidence interval (CI), 0.705–0.801] and 0.685 (95% CI, 0.608–0.755) in the training and validation cohorts, respectively (Figure 5A), indicating that effective classification of MSS and MSI was not satisfied based on pure clinical information. The six selected features-based RI model discriminated MSI from MSS with an AUC of 0.945 (95% CI, 0.914–0.967) and 0.784 (95% CI, 0.713–0.844) in the training and validation cohorts, respectively (Figure 5B). The RIC model, which incorporated both the radiomics signature and independent clinicopathological characteristics, outperformed the other two models with an AUC of 0.989 (95% CI, 0.970–0.997) and 0.895 (95% CI, 0.838–0.938) in the training and validation cohorts, respectively (Figure 5C). The detailed sensitivity, specificity, and AUC of these predictive models with or without incorporation of clinicopathological characteristics are summarized in Table 3. The results in the validation cohort suggested that the RIC model showed better performance than the RI model, however the difference was not statistically significant (Delong’s test, P=0.204). Compared with the clinical variables alone, integrating the radiomics signature and clinicopathological characteristics could significantly improve the predictive performance (Delong’s test, P=0.015).
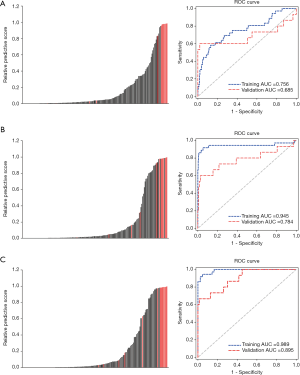
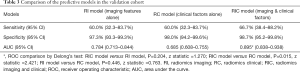
Full table
Discussion
To the best of our knowledge, this is the first study to evaluate the significance of the radiomics approach to predict MSI status in rectal cancer based on preoperative MRI data. Our results showed that radiomics analysis achieved a better predictive performance with a model that combined both imaging features and clinical variables (AUC 0.895) than the models using either imaging features or clinical factors alone (AUC of 0.784 and 0.685, respectively). This suggests that MRI is useful for predicting the MSI status of rectal cancer patients and thus has the potential to aid in the determination of therapeutic strategies.
Literature reviews report a prevalence of MSI of approximately 17% in colorectal cancer (13). The incidence of MSI reported by two previous radiomics studies based on CT data was 25.21% (25) and 32% (26). Our study showed a prevalence of MSI of 10.39% in rectal cancer, which is clearly lower than that reported by the previous studies. A possible explanation for the lower prevalence of MSI in our study compared to those in the aforementioned studies is that these studies enrolled patients with colorectal cancer, including right- and left-sided colon and rectal cancer, whereas our study merely included patients with rectal cancer. Furthermore, it has been reported that the rate of MSI is higher in right-sided colorectal cancer than in left-sided colorectal cancer (28).
Considering that MSI plays a key role in the prognosis and treatment of rectal cancer and that MSI evaluation by IHC and polymerase chain reaction (PCR) may not be routinely available, recent studies have attempted to identify MSI using methods other than IHC and PCR. Fan et al. (25) and Golia et al. (26) investigated the relationship between imaging features and MSI status in colorectal cancer using radiomics analysis based on conventional CT data. Both of these studies concluded that the combined model of clinical and CT imaging features was more effective in predicting MSI than the independent clinical or radiomics features. A more recent study (27) reported that radiomics analysis based on iodine-based material decomposition images with dual-energy CT imaging provides a relatively high diagnostic value for predicting MSI status in colorectal cancer. All three radiomics studies mentioned above were based on CT data and included all left- and right-sided colorectal cancers. However, significant pathological differences between left and right-sided colorectal cancers, including MSI status, have been recognized (28). In the present study, we focused only on rectal cancer, which may reduce the bias caused by pathological differences between left- and right-sided colorectal cancers. Furthermore, compared to CT, soft-tissue resolution is higher with MRI, which is the preferred imaging method for rectal cancer (29,30), and may therefore provide more valuable data for radiomics analysis via high-throughput extraction of quantitative imaging features. The present study compared the effects of using clinical or radiomics characteristics alone, or a combination of these characteristics to classify MSI status in rectal cancer based on MRI data. The combination model incorporating clinical and radiomics features exhibited superior discerning ability in predicting MSI of rectal cancer, higher than that of the models that included clinical or radiomics features alone. The results of the present study, which utilized MRI data instead of CT images, are consistent with those reported by previous radiomics studies based on CT data, however we obtained relatively higher AUCs. This suggests that radiomics analysis based on MRI data has a better performance than that based on CT for predicting the MSI status in rectal cancer.
As a preliminary study, this research had several limitations. Firstly, although 491 patients with rectal cancer were enrolled in our study, the sample size of the MSI group was significantly smaller than that of the MSS group owing to the low prevalence of MSI in rectal cancer. This potentially reduced the statistical performance, and thus, further data collection and research are needed. Secondly, as a single-center retrospective study, our results need to be validated by future multicenter studies to evaluate or improve the performance of our prediction models. Thirdly, manual segmentation is time-consuming and may be a source of observer variability. Previous study has developed automatic segmentation method for rectal cancer based on CT data (40). But so far, there is no automatic segmentation method for rectal cancer based on MRI data. In the future, automatic segmentation of rectal cancer based on MRI data may help in improving efficiency and eliminating the subjective influence of manual segmentation.
In conclusion, this preliminary study presents a radiomics prediction model that incorporates both MRI features and clinical variables to identify MSI in rectal cancer. This combined analysis of multiple predictors is now regarded as the most promising method to assist clinical practice. The combined prediction model in our study is a potential tool for the noninvasive prediction of MSI in rectal cancer, which is beneficial for individualized treatment and prognosis prediction.
Acknowledgments
Funding: The authors express their gratitude to the Research Grant of National Nature Science Foundation of China (Grant number 81971571).
Footnote
Reporting Checklist: The authors have completed the TRIPOD reporting checklist. Available at http://dx.doi.org/10.21037/atm-20-7673
Data Sharing Statement: Available at http://dx.doi.org/10.21037/atm-20-7673
Conflicts of Interest: All authors have completed the ICMJE uniform disclosure form (available at http://dx.doi.org/10.21037/atm-20-7673). Bin Song serves as an unpaid editorial board member of Annals of Translational Medicine (ATM) from 09/2020 to 08/2021. The other authors have no conflicts of interest to declare.
Ethical Statement: The authors are accountable for all aspects of the work in ensuring that questions related to the accuracy or integrity of any part of the work are appropriately investigated and resolved. This study was conducted in accordance with the Declaration of Helsinki (as revised in 2013), and was approved by the Medical Ethics Committee of West China Hospital (No. 2019-1159). Individual informed consent for this retrospective analysis was waived.
Open Access Statement: This is an Open Access article distributed in accordance with the Creative Commons Attribution-NonCommercial-NoDerivs 4.0 International License (CC BY-NC-ND 4.0), which permits the non-commercial replication and distribution of the article with the strict proviso that no changes or edits are made and the original work is properly cited (including links to both the formal publication through the relevant DOI and the license). See: https://creativecommons.org/licenses/by-nc-nd/4.0/.
References
- Siegel RL, Miller KD, Fedewa SA, et al. Colorectal cancer statistics, 2017. CA Cancer J Clin 2017;67:177-93. [Crossref] [PubMed]
- Sauer R, Becker H, Hohenberger W, et al. Preoperative versus postoperative chemoradiotherapy for rectal cancer. N Engl J Med 2004;351:1731-40. [Crossref] [PubMed]
- Enker WE, Thaler HT, Cranor ML, et al. Total mesorectal excision in the operative treatment of carcinoma of the rectum. J Am Coll Surg 1995;181:335-46. [PubMed]
- Oh CR, Kim JE, Kang J, et al. Prognostic value of the microsatellite instability status in patients with stage II/III rectal cancer following upfront surgery. Clin Colorectal Cancer 2018;17:e679-85. [Crossref] [PubMed]
- Gupta R, Sinha S, Paul RN. The impact of microsatellite stability status in colorectal cancer. Curr Probl Cancer 2018;42:548-59. [Crossref] [PubMed]
- Sinicrope FA, Sargent DJ. Molecular pathways: microsatellite instability in colorectal cancer: prognostic, predictive, and therapeutic implications. Clin Cancer Res 2012;18:1506-12. [Crossref] [PubMed]
- Aghagolzadeh O, Radpour R. New trends in molecular and cellular biomarker discovery for colorectal cancer. World J Gastroenterol 2016;22:5678-93. [Crossref] [PubMed]
- Sargent DJ, Marsoni S, Monges G, et al. Defective mismatch repair as a predictive marker for lack of efficacy of fluorouracil-based adjuvant therapy in colon cancer. J Clin Oncol 2010;28:3219-26. [Crossref] [PubMed]
- Jover R, Zapater P, Castells A, et al. The efficacy of adjuvant chemotherapy with 5-fluorouracil in colorectal cancer depends on the mismatch repair status. Eur J Cancer 2009;45:365-73. [Crossref] [PubMed]
- He EY, Hawkins NJ, Mak G, et al. The impact of mismatch repair status in colorectal cancer on the decision to treat with adjuvant chemotherapy: an Australian population-based multicenter study. Oncologist 2016;21:618-25. [Crossref] [PubMed]
- Mahasneh A, Al-Shaheri F, Jamal E. Molecular biomarkers for an early diagnosis, effective treatment and prognosis of colorectal cancer: Current updates. Exp Mol Pathol 2017;102:475-83. [Crossref] [PubMed]
- National Comprehensive Cancer Network (NCCN). Clinical practice guidelines in oncology: colon cancer (Version 2.2016). Available online: https://www.nccn.org/professionals/physician_gls/f_guidelines.asp. Accessed 5 Jan 2017.
- Luchini C, Bibeau F, Ligtenberg M, et al. ESMO recommendations on microsatellite instability testing for immunotherapy in cancer, and its relationship with PD-1/PD-L1 expression and tumour mutational burden: a systematic review-based approach. Ann Oncol 2019;30:1232-43. [Crossref] [PubMed]
- Burrell RA, McGranahan N, Bartek J, et al. The causes and consequences of genetic heterogeneity in cancer evolution. Nature 2013;501:338-45. [Crossref] [PubMed]
- Meng X, Xia W, Xie P, et al. Preoperative radiomic signature based on multiparametric magnetic resonance imaging for noninvasive evaluation of biological characteristics in rectal cancer. Eur Radiol 2019;29:3200-9. [Crossref] [PubMed]
- Lambin P, Rios-Velazquez E, Leijenaar R, et al. Radiomics: extracting more information from medical images using advanced feature analysis. Eur J Cancer 2012;48:441-6. [Crossref] [PubMed]
- Gillies RJ, Kinahan PE, Hricak H. Radiomics: images are more than pictures, they are data. Radiology 2016;278:563-77. [Crossref] [PubMed]
- Lambin P, Leijenaar R, Deist TM, et al. Radiomics: the bridge between medical imaging and personalized medicine. Nat Rev Clin Oncol 2017;14:749-62. [Crossref] [PubMed]
- Nie K, Shi L, Chen Q, et al. Rectal cancer: assessment of neoadjuvant chemoradiation outcome based on radiomics of multiparametric MRI. Clin Cancer Res 2016;22:5256-64. [Crossref] [PubMed]
- Shen WC, Chen SW, Wu KC, et al. Predicting pathological complete response in rectal cancer after chemoradiotherapy with a random forest using 18F-fluorodeoxyglucose positron emission tomography and computed tomography radiomics. Ann Transl Med 2020;8:207. [Crossref] [PubMed]
- Liu L, Liu Y, Xu L, et al. Application of texture analysis based on apparent diffusion coefficient maps in discriminating different stages of rectal cancer. J Magn Reson Imaging 2017;45:1798-808. [Crossref] [PubMed]
- Huang YQ, Liang CH, He L, et al. Development and validation of a radiomics nomogram for preoperative prediction of lymph node metastasis in colorectal cancer. J Clin Oncol 2016;34:2157-64. [Crossref] [PubMed]
- Yang L, Dong D, Fang M, et al. Can CT-based radiomics signature predict KRAS/NRAS/BRAF mutations in colorectal cancer? Eur Radiol 2018;28:2058-67. [Crossref] [PubMed]
- Oh JE, Kim MJ, Lee J, et al. Magnetic Resonance-Based Texture Analysis Differentiating KRAS Mutation Status in Rectal Cancer. Cancer Res Treat 2020;52:51-9. [Crossref] [PubMed]
- Fan S, Li X, Cui X, et al. Computed tomography-based radiomic features could potentially predict microsatellite instability status in stage II colorectal cancer: a preliminary study. Acad Radiol 2019;26:1633-40. [Crossref] [PubMed]
- Golia Pernicka JS, Gagniere J, Chakraborty J, et al. Radiomics-based prediction of microsatellite instability in colorectal cancer at initial computed tomography evaluation. Abdom Radiol (NY) 2019;44:3755-63. [Crossref] [PubMed]
- Wu J, Zhang Q, Zhao Y, et al. Radiomics analysis of iodine-based material decomposition images with dual energy CT imaging for preoperatively predicting microsatellite instability status in colorectal cancer. Front Oncol 2019;9:1250. [Crossref] [PubMed]
- Boland CR, Goel A. Microsatellite instability in colorectal cancer. Gastroenterology 2010;138:2073-87.e3. [Crossref] [PubMed]
- Benson AB, Venook AP, Al-Hawary MM, et al. Rectal Cancer, Version 2.2018, NCCN Clinical Practice Guidelines in Oncology. J Natl Compr Canc Netw 2018;16:874-901. [Crossref] [PubMed]
- Glynne-Jones R, Wyrwicz L, Tiret E, et al. Rectal cancer: ESMO Clinical Practice Guidelines for diagnosis, treatment and follow-up. Ann Oncol 2017;28:iv22-iv40. [Crossref] [PubMed]
- van Griethuysen JJM, Fedorov A, Parmar C, et al. Computational Radiomics System to Decode the Radiographic Phenotype. Cancer Res 2017;77:e104-7. [Crossref] [PubMed]
- Sun H, Qu H, Chen L, et al. Identification of suspicious invasive placentation based on clinical MRI data using textural features and automated machine learning. Eur Radiol 2019;29:6152-62. [Crossref] [PubMed]
- Peeken JC, Spraker MB, Knebel C, et al. Tumor grading of soft tissue sarcomas using MRI-based radiomics. Ebiomedicine 2019;48:332-40. [Crossref] [PubMed]
- Büsing KA, Kilian AK, Schaible T, et al. Reliability and validity of MR image lung volume measurement in fetuses with congenital diaphragmatic hernia and in vitro lung models. Radiology 2008;246:553-61. [Crossref] [PubMed]
- Devore JL, Peck R. Statistics: The Exploration and Analysis of Data. 3rd ed. Duxbury; 1996.
- Sauerbrei W, Royston P, Binder H. Selection of important variables and determination of functional form for continuous predictors in multivariable model building. Stat Med 2007;26:5512-28. [Crossref] [PubMed]
- Chen T, Guestrin C. XGBoost: A scalable tree boosting system. In: Proceedings of the ACM SIGKDD International Conference on Knowledge Discovery and Data Mining (KDD ’16). New York: Association for Computing Machinery, 2016:785-94.
- Liang W, Zhang L, Jiang G, et al. Development and validation of a nomogram for predicting survival in patients with resected non-small-cell lung cancer. J Clin Oncol 2015;33:861-9. [Crossref] [PubMed]
- DeLong ER, DeLong DM, Clarke-Pearson DL. Comparing the areas under two or more correlated receiver operating characteristic curves: a nonparametric approach. Biometrics 1988;44:837-45. [Crossref] [PubMed]
- Gambacorta MA, Luca B, Chiara V, et al. Automatic segmentation software in locally advanced rectal cancer: READY (REsearch program in Auto Delineation sYstem)-RECTAL 02: prospective study. Oncotarget 2016;7:42579-84. [PubMed]
(English Language Editor: A. Kassem)