Comparative proteomics analysis for identifying the lipid metabolism related pathways in patients with Klippel-Feil syndrome
Introduction
Klippel-Feil syndrome (KFS) is a relatively rare and complicated condition that is characterized as congenital fusion of two or more cervical vertebrae with or without additional spinal or extraspinal manifestations (1,2). It is estimated to occur one in every 40,000 to 42,000 births (3). However, the prevalence of KFS may be much higher because its diagnosis can be missed due to heterogeneity in phenotypic expression between patients (4-6). Patients with KFS show the clinical triad of short neck, low posterior hairline, and limited cervical range of motion (7). Congenital cervical fusion deformity often alters the kinematics of the cervical spine, leading to acceleration of degenerative manifestations, hypermobility and instability, neurologic symptoms, and the potential for neurological spinal injury after major or even minor trauma (8,9). As a consequence, KFS may result in serious physical and mental problems in patients. Studies have shown that, in KFS, the congenital fusion of the cervical spine is associated with failure of formation and segmentation embryologically (8). Mutated genes such as GDF6, MEOX1, GDF3, MYO18B, and RIPPLY2 encode proteins involved in somite development via transcription regulation and signaling pathways (10-14). Although significant progress has been made in understanding the process of cervical vertebra fusion, there is a wide gap in understanding of the detailed mechanisms of KFS because of its rarity, heterogeneity, small pedigrees, and the broad spectrum of anomalies (15).
Thus, it is essential to explore early specific biomarkers and design measures to prevent potentially fatal outcomes in KFS patients. Data-independent acquisition (DIA) based quantitative proteomics analysis is a powerful mass spectrometric (MS) technique to perform both protein identification and quantification of complex protein samples (16,17). Furthermore, DIA method has been performed in biomarker studies to understand the pathogenesis and underlying mechanisms of congenital diseases (18-20).
Therefore, proteomics by DIA coupled with Q-Exactive mass spectrometry was used to compare the serum protein profiles of patients with KFS and healthy controls as well as patients with KFS and patients with congenital scoliosis (CS; patients with known syndromes and cervical deformity were excluded).
We present the following article in accordance with the MDAR checklist (available at http://dx.doi.org/10.21037/atm-20-5155).
Methods
Patients and blood collection
We consecutively recruited eight patients of Chinese Han ethnicity with KFS, who had fulfilled inclusion criteria of the congenital fusion of at least two cervical vertebrae, in the Peking Union Medical College Hospital from October 2018 to May 2019. Four were male and four were female, and the mean age at diagnosis was 21.0±13.4 years. Seven healthy control participants and five patients with CS were recruited with the sex and age matched. The patients with CS were presented with congenital fusion of the thoracic or lumbar spine and had no known syndrome and cervical deformity. The healthy controls had no congenital skeletal malformations which were confirmed by spine X-ray.
Blood samples were drawn from all the participants and centrifuged at 16,000 ×g (4 °C) for 10 min. The serum from each sample was transferred into new Eppendorf tubes and stored at −80 °C until used. Demographic information, clinical symptoms, detailed comorbidities, and radiological assessments of all the participants also were obtained (Table S1).
The study was conducted in accordance with the Declaration of Helsinki (as revised in 2013). The study was approved by ethics board of the Peking Union Medical College Hospital (No. JS-098) and informed consent was taken from all individual participants.
Sample preparation
ProteoPrep Blue Albumin and an IgG Depletion kit (PROTBA, Sigma-Aldrich Company, Darmstadt, Germany) were used to deplete serum IgG and albumin. We used 10 µL serums for the depletion, and 1 mL was eluted at the end of the procedure. The Bradford method was used to measure the protein concentration after depletion and vacuum concentration according to the manufacturer’s instructions. Next, 40 µg protein lysate was reduced with 25 mM DTT at 60 °C for 60 min and alkylated with 50 mM iodoacetamide in the dark for 30 min. After alkylation, FASP digestion was performed for each sample using an ultrafiltration filter (10 kDa cutoff, Sartorius, German). Trypsin was added in a 1:100 ratio (enzyme:protein) at 37 °C for 14–16 h, after which the samples were centrifuged at 20,000 ×g (4 °C) for 10 min. The peptides were desalted using Ziptip C18 pipette tips (Merck KGaA, Darmstadt, Germany). After drying, the peptides were resuspended in 0.1% formic acid. Then, 20 µg protein lysate was taken out of each sample and used to build the DIA Spectral Library. The Biognosys’ iRT kit was added to the rest of the samples according to the manufacturer’s instructions (required for DIA analysis using Biognosys’ Spectronaut).
High pH reversed-phase fractionation
High pH reversed-phase chromatography was applied to further fractionate the digests and 30 µg of the digest was combined. The RIGOL L-3000 system (RIGOL, Beijing, China) was applied to the reverse-phase chromatography column to separate the peptides. The peptide mixtures were dissolved in 100 µL mobile phase A (2% (v/v) acetonitrile, 98% (v/v) ddH2O; pH 10) and then centrifuged at 14,000 ×g for 20 min. The supernatant was loaded into the column and eluted by continuously injecting mobile B (98% (v/v) acetonitrile, 2% (v/v) ddH2O; pH 10) at a flow rate of 700 µL/min. A step gradient (1.5 min per step) of mobile phase B was used to elute and collect the fractions.
Mass spectrometric acquisition
Each sample was analyzed using the analytical column (75 µm × 250 mm; 2 µm) in a nanoliquid chromatography system (EASY-nLC 1000 System, Thermo Scientific, Waltham, MA, USA) connected to a Q Exactive HF mass spectrometer (Thermo Scientific). A binary solvent system with 99.9% H2O, 0.1% formic acid (phase A) and 80% ACN, 19.9% H2O, 0.1% formic acid (phase B) was applied to elute the peptide mixtures. The following linear gradient was applied: 13–28% B in 93 min, 28–38% B in 11 min, 38–100% B in 4 min, and washed at 100% B for 8 min. The eluent was added directly into the Q-Exactive HF mass spectrometer via an EASY-Spray ion source. Source ionization parameters were as follows: spray voltage 2.2 kV, capillary temperature 320 °C, and declustering potential 100 V. One full-scan MS from 300 to 1,600 m/z, then 20 MS/MS scans were continuously acquired for data-dependent acquisition (DDA) LC-MS/MS analysis. The resolution was set to 60,000 for MS and 30,000 for MS/MS. For high-energy collisional dissociation, the isolation window was set to 2 m/z and the normalized collision energy was applied as 28%.
The DIA LC-MS/MS method relied on the MS1 scan from 300 to 1,100 m/z (AGC target of 36 or 80 ms injection time). DIA segments were collected at 30,000 resolutions (AGC target 2e5 and auto for injection time). The spectra were recorded in profile mode with collision energy 28%. The default charge state for the MS2 was set to 3. The raw mass spectrometric data, the spectral libraries, and the quantitative data tables have been deposited to the ProteomeXchange Consortium via the PRIDE partner repository.
Mass spectrometric data analysis and protein identification
DIA analysis was performed on Spectronaut Pulsar, the mass spectrometer vendor-independent software from Biognosys. The false discovery rate was evaluated using the mProphet approach and was set to 1% at the peptide level. Protein inference was performed on the principle of parsimony using the ID picker algorithm implemented in Spectronaut. For the DIA analysis, the RAW files were converted to the Spectronaut file format and calibrated in the retention time dimension based on the global spectral library. The recalibrated files were used for the targeted data analysis without new recalibration of the retention time dimension.
Proteome Discoverer 2.1 analysis software with default settings (Trypsin/P, two missed cleavages) was applied for the DDA spectra. Search criteria included carbamidomethylation of cysteine as a fixed modification and oxidation of methionine and acetyl (protein N terminus) as variable modifications. The parent ion tolerance was 10 ppm and the fragment ion mass tolerance was 0.02 Da. The DDA files were searched against the human Swiss-Prot fasta database (state 15.03.2018, 20,240 entries) and the Biognosys iRT peptides fasta database (uploaded to the public repository).
Procedures for sample preparation, high pH reversed-phase fractionation, LC-MS/MS and data analysis were shown in Figure 1.
Bioinformatics and statistical analyses
The Perseus software was used to analyze the differences among the proteomic inventories in the two comparisons and the data were subjected to an analysis of variance test (two-sample t-tests). The cutoff values were set as 1.4-fold for increased and 0.71-fold for decreased abundance and the differential changes were considered significant at P≤0.05. The three main Gene Ontology (GO) categories, biological process, molecular function, and cellular component, were analyzed with OmicsBox (1.2.4) and pathway analysis was performed using the Ingenuity Pathway Analysis (IPA) database.
Results
Identification and functional analysis of DAPs between patients with KFS and healthy controls
We performed proteomic profiling of eight patients with KFS and seven healthy controls. A total of 493 proteins were identified and 49 of them were differentially abundant in the patients with KFS compared with the controls (ratio ≥1.42 or ≤0.70; P<0.05). Among the differentially abundant proteins (DAPs), 27 were significantly decreased and 22 were significantly increased. Details of the 49 DAPs are presented in Table S2 and their distribution is shown in the volcano plot in Figure 2A. A protein-protein interaction network was constructed using the DAPs that exhibited specific and extensive interactions and a score was calculated to assess the probability of protein-protein interactions that occurred by random chance (Figure 2B). The highest scoring network (score =18) included 10 target proteins related to cellular development, cellular growth and proliferation, and cellular movement. The DAPs were functionally annotated with GO terms under the three main categories: biological process, molecular function, and cellular component. Under biological process, the most enriched terms were related to regulation of cellular process, cellular response to stimulus, establishment of localization, and immune response. Under molecular function, the most enriched terms were protein binding, antigen binding, and ion binding. Under cellular components, the most enriched terms were extracellular space, plasma membrane, and cell periphery (Figure 2C).
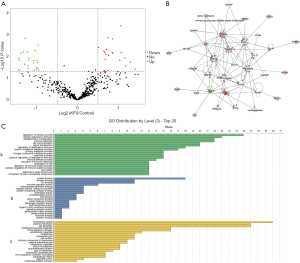
Identification and functional analysis of DAPs between patients with KFS and patients with CS
To further explore the correlated pathogenesis between KFS and CS and provide possible reasons for the deformity located at cervical segments, we performed proteomic profiling of patients with KFS and patients with CS. A total of 462 proteins were identified and 192 DAPs were differentially abundant in patients with KFS compared with patients with CS. Among them, 118 decreased and 74 increased in abundance. Details of the 192 DAPs are presented in Table S3. The DAPs were functionally annotated with GO terms. Under biological process, the most enriched term was regulation of cellular process, followed by organic substance metabolic process, primary metabolic process, cellular metabolic process, and nitrogen compound metabolic process. Under molecular function, the most enriched terms were protein binding and ion binding. Under cellular component, the most enriched terms were extracellular space and membrane-bounded organelle. The protein-protein interaction network with the highest score (score =37) included 25 target molecules associated with cancer, hematological disease, and inflammatory disease. The volcano plot, GO functional annotation, and protein-protein interaction network of these DAPs are shown in Figure 3.
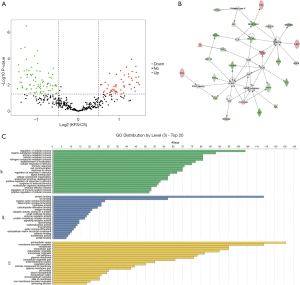
Common DAPs in the two comparisons
We selected 15 DAPs that were detected in both comparisons as candidate markers of KFS (Table 1). They included glyceraldehyde-3-phosphate dehydrogenase, CD109 antigen, cadherin-5, peroxiredoxin-1, galectin-3-binding protein, membrane primary amine oxidase, noelin, and eight immunoglobulins. A cluster analysis of the abundance levels of the 15 DAPs in patients with KFS, controls, and patients with CS showed that the abundance levels of eight immunoglobulins decreased significantly in patients with KFS or CS compared with the controls. Moreover, the decline in the abundance levels of immunoglobulins in patients with CS was more significantly than that in patients with KFS (Figure 4).
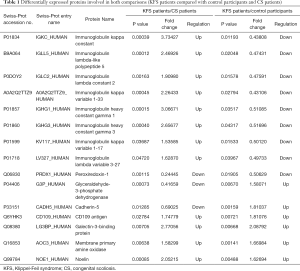
Full table
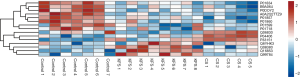
Common canonical pathways in the two comparisons
The distribution of the DAPs in the canonical pathways was calculated using the IPA software package. Pathways were considered to be significantly enriched for P<0.05. The DAPs detected in the patients with KFS vs. controls comparison were involved in primary immunodeficiency signaling, hematopoiesis from pluripotent stem cells, communication between innate and adaptive immune cells, autoimmune thyroid disease signaling, phagosome formation, and LXR/RXR and FXR/RXR activation. The DAPs detected in the patients with KFS vs. patients with CS comparison were involved in crucial lipid metabolism-related pathways of LXR/RXR and FXR/RXR activation (Figure 5). The abundance levels of three key differentially abundant lipoproteins, apolipoprotein C-III (APOC3), apolipoprotein A-IV (APOA4), and apolipoprotein F (APOF), were highly elevated in patients with KFS vs. controls, whereas the abundance levels of four other key lipoproteins, apolipoprotein A-II (APOA2), apolipoprotein E (APOE), apolipoprotein H (APOH: beta-2-glycoprotein I), and apolipoprotein D (APOD) were differentially abundant in the patients with KFS vs. patients with CS comparison; APOA2, APOE, and APOH were significantly reduced and APOD was highly elevated. All the DAPs involved in the LXR/RXR and FXR/RXR activation pathways, including the seven differentially abundant apolipoproteins, are shown in Table 2.
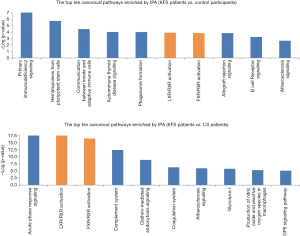
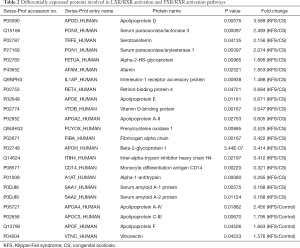
Full table
Discussion
KFS is a congenital cervical fusion malformation caused by segmentation defects in mesodermal somites (8,21,22). In this study, we employed a DIA method to explore 49 DAPs in patients with KFS compared with healthy controls. CS is a complex deformity of the spine caused by vertebral malformations, including defects of vertebral formation (hemivertebra or wedge vertebra) and defects of vertebral segmentation (vertebral bar or block vertebra) (23,24). Although CS and KFS both present with congenital vertebral malformation, whether there are potential pathogenic mechanisms between CS and KFS is largely unknown (25,26). Therefore, we performed a proteomic analysis of patients with KFS and patients with CS to detect predictive protein biomarkers of KFS and to explore the underlying mechanism between KFS and CS in somitogenesis. Overall, eight different immunoglobulins were detected among the 15 common DAPs in the two comparisons.
Immunoglobulins are glycoproteins that play roles in the immune system by specifically recognizing and binding to particular pathogens. Immunoglobulin kappa chain constant region (IGKC) was identified as a candidate gene that encodes a protein that may play a pivotal role in the autoimmune mechanism associated with the etiology of abdominal aortic aneurysm formation (27). In addition, IGKC was described as the B cell-related gene signature in human solid tumors, including breast, lung, and colorectal adenocarcinomas. IGKC is a novel diagnostic marker for risk stratification and the encoded protein may promote the humoral immune response in anticancer therapy (28). Moreover, immunogenetic studies suggested that IGKC and immunoglobulin heavy chain G (IGHG) contributed to the prognosis in breast cancer and to breast tumor-associated antigens in a racially restricted manner (29-31). However, our results are the first to account for the correlation between immunoglobulins and congenital spine deformities. The cluster analysis revealed a significant decline in the abundance levels of immunoglobulins, including IGHG1, IGHG3 and IGKC, in both patients with KFS and patients with CS compared with the healthy controls. These findings indicated that the low abundance of immunoglobulins may contribute to congenital vertebral malformation. We also found that immunoglobulins were more abundant in serum samples of patients with KFS compared with those of patients with CS, which indicated that immunoglobulins may be valuable biomarkers for KFS. We deduced that dysfunction of the immune system may be the underlying pathogenic mechanism of somitogenesis defects in the cervical spine.
One of the 15 common DAPs, membrane primary amine oxidase, showed a more than 1.6-fold increase in patients with KFS compared with the controls. Membrane primary amine oxidase is highly abundant in endothelial cells, smooth muscle cells, and adipocytes (32) and is associated with several amino acid metabolism pathways by catalyzing the oxidative deamination of primary amines to aldehydes (33). Amino acid deficiency in the amniotic fluid was related to the pathogenesis of skeletal dysplasia in pregnant women with skeletal dysplasia fetuses (34). Further, protein tyrosine phosphatases were shown to play important roles in gastrulation and somitogenesis during early embryonic development, osteogenesis, and angiogenesis (35). Therefore, we propose that membrane primary amine oxidase could be a candidate biomarker related to amino acid metabolism in the pathogenesis of KFS. The other common DAPs, galectin-3-binding protein, glyceraldehyde-3-phosphate dehydrogenase, peroxiredoxin-1, CD109 antigen, cadherin-5, and noelin, have not previously been reported to be related to KFS. They require further validation to establish their possible use as biomarkers for the etiological elucidation in KFS.
The IPA illustrated that the LXR/RXR and FXR/RXR activation pathways were common significant canonical pathways in the patients with KFS vs. controls and patients with KFS vs. patients with CS comparisons. All seven of the differentially abundant apolipoproteins (APOC3, APOA4, APOF, APOA2, APOE, APOH, and APOD) were involved these pathways. Retinoid X receptors (RXRs) are nuclear receptors that regulate a variety of physiological processes, including lipid metabolism, osteoclastogenesis, inflammatory response, and cell differentiation (36-38). Liver X receptors (LXRs) are ligand-activated transcription factors that form a functional heterodimer with RXR through the LXR reaction element (39,40). LXR/RXR is considered to be involved in lipid metabolism and bile acid metabolism (41,42) and the formation of LXR/RXR heterodimers was shown to inhibit the transcriptional program of osteoclast differentiation (37). Farnesoid X receptors (FXRs) are members of the nuclear receptor family, which is involved in many metabolic pathways (43). FXR/RXR and LXR/RXR activation are involved in regulating osteogenic differentiation of adipose stem cells (44). These biological pathways detected in patients with KFS are consistent with the results of a previous comparative proteomics study by iTRAQ that explored the differential serum protein abundance levels of nine patients with CS who had TBX6 haploinsufficiency and nine healthy controls (45). Several studies have shown that lipid metabolism is related to osteogenesis and musculoskeletal disorders. Nogami et al. reported that increased numbers of lipid droplets were detected in biopsied muscle fibers of two patients with congenital skeletal deformities such as scoliosis (46). van Gastel et al. revealed a role for forkhead box O transcription factors during lipid starvation and defined lipid scarcity as an important determinant of chondrogenic commitment (47). Rendina-Ruedy et al. suggested that lipids in the bone marrow are essential for proper bone remodeling (48). Vasiljevski et al. summarized neurofibromatosis type 1 and autoimmune myopathies (polymyositis, dermatomyositis, and inclusion body myositis) are involved in dysfunction of lipid metabolism (49). As such, our results and other evidence strongly point to disrupted lipid metabolism in KFS.
Apolipoprotein is plasma lipoprotein that is synthesized mainly in the liver (and partially in the small intestine) and transports lipids and stabilizes lipoproteins (50). APOE and APOA1 were shown to be highly abundant in the embryonic yolk syncytial layer and distributed in the form of cell clusters along the spinal cord, an extraembryonic structure implicated in embryonic and larval nutrition (51). It has been demonstrated that APOE was up-regulated by bone morphogenetic protein-2 in the murine mesenchymal progenitor cell and APOE−/− mice had significantly reduced levels of the osteoblastic (RUNX2 and Osterix) and lipoblastic (PPARγ and CEBPα) indicators (52-54). These findings suggested that APOE played significant roles in embryonic differentiation as well as osteoblastic and lipoblastic differentiation and activity. The pathogenesis of congenital cervical fusion malformations have been attributed to the paraxial mesoderm, somites, or central axis at the embryonic stage, possibly because of disruptions in differentiation and segmentation (55). Therefore, differentially abundant apolipoproteins and lipid metabolism-related molecules may play important roles in the development of paraxial mesoderm, somites, and the central axis, and may be involved in the pathogenesis of KFS and related vertebral segmentation defects.
The differentially abundant apolipoproteins and LXR/RXR and FXR/RXR activation pathways related to lipid metabolism led us to strongly speculate that lipid metabolism was involved in the underlying pathogenesis of KFS. Because somitogenesis generates vertebral structures in a rostral-to-caudal direction, we predict that the differentially abundant apolipoproteins detected in patients with KFS and patients with CS (e.g. APOA2, APOD, APOE, and APOH) could be significant biomarkers to investigate the possible mechanism associated with the vertebral segmental abnormalities located at cervical segments.
Conclusions
We detected 15 DAPs that were common in both comparisons as candidate predictive biomarkers of KFS. The lipid metabolism-related canonical pathways of LXR/RXR and FXR/RXR activation together with seven differentially abundant apolipoproteins may play significant roles in the etiology of KFS and provide possible pathogenesis correlation between KFS and CS. The underlying interactions among the 15 DAPs and the progression of KFS require further investigation.
Acknowledgments
The authors would like to thank all the patients and their family members for their help and informed consent.
Funding: This work was supported by the National Natural Science Foundation of China (No. 81871746 to YW, No. 81772299 and 81930068 to ZW, No. 81822030 to NW, No. 81672123 and 81972037 to JZ) and China Postdoctoral Science Foundation (No. 2020TQ0052 to ZL).
Footnote
Reporting Checklist: The authors have completed the MDAR checklist. Available at http://dx.doi.org/10.21037/atm-20-5155
Data Sharing Statement: Available at http://dx.doi.org/10.21037/atm-20-5155
Conflicts of Interest: All authors have completed the ICMJE uniform disclosure form (available at http://dx.doi.org/10.21037/atm-20-5155). The authors have no conflicts of interest to declare.
Ethical Statement: The authors are accountable for all aspects of the work in ensuring that questions related to the accuracy or integrity of any part of the work are appropriately investigated and resolved. The study was conducted in accordance with the Declaration of Helsinki (as revised in 2013). The study was approved by ethics board of the Peking Union Medical College Hospital (NO. JS-098) and informed consent was taken from all individual participants.
Open Access Statement: This is an Open Access article distributed in accordance with the Creative Commons Attribution-NonCommercial-NoDerivs 4.0 International License (CC BY-NC-ND 4.0), which permits the non-commercial replication and distribution of the article with the strict proviso that no changes or edits are made and the original work is properly cited (including links to both the formal publication through the relevant DOI and the license). See: https://creativecommons.org/licenses/by-nc-nd/4.0/.
References
- Samartzis D, Kalluri P, Herman J, et al. Cervical scoliosis in the Klippel-Feil patient. Spine 2011;36:E1501-8. [Crossref] [PubMed]
- Mesfin A, Bakhsh WR, Chuntarapas T, et al. Cervical Scoliosis: Clinical and Radiographic Outcomes. Global Spine J 2016;6:7-13. [Crossref] [PubMed]
- Juberg RC, Gershanik JJ. Cervical vertebral fusion (Klippel-Feil) syndrome with consanguineous parents. J Med Genet 1976;13:246-9. [Crossref] [PubMed]
- Xue X, Shen J, Zhang J, et al. Klippel-Feil syndrome in congenital scoliosis. Spine 2014;39:E1353-8. [Crossref] [PubMed]
- Gruber J, Saleh A, Bakhsh W, et al. The Prevalence of Klippel-Feil Syndrome: A Computed Tomography-Based Analysis of 2,917 Patients. Spine Deform 2018;6:448-53. [Crossref] [PubMed]
- Nouri A, Tetreault L, Zamorano JJ, et al. Prevalence of Klippel-Feil Syndrome in a Surgical Series of Patients with Cervical Spondylotic Myelopathy: Analysis of the Prospective, Multicenter AOSpine North America Study. Global Spine J 2015;5:294-9. [Crossref] [PubMed]
- Samartzis D, Kalluri P, Herman J, et al. "Clinical triad" findings in pediatric Klippel-Feil patients. Scoliosis Spinal Disord 2016;11:15. [Crossref] [PubMed]
- Tracy MR, Dormans JP, Kusumi K. Klippel-Feil syndrome: clinical features and current understanding of etiology. Clin Orthop Relat Res 2004.183-90. [Crossref] [PubMed]
- Nouri A, Patel K, Evans H, et al. Demographics, presentation and symptoms of patients with Klippel-Feil syndrome: analysis of a global patient-reported registry. Eur Spine J 2019;28:2257-65. [Crossref] [PubMed]
- Mohamed JY, Faqeih E, Alsiddiky A, et al. Mutations in MEOX1, encoding mesenchyme homeobox 1, cause Klippel-Feil anomaly. Am J Hum Genet 2013;92:157-61. [Crossref] [PubMed]
- Ye M, Berry-Wynne KM, Asai-Coakwell M, et al. Mutation of the bone morphogenetic protein GDF3 causes ocular and skeletal anomalies. Hum Mol Genet 2010;19:287-98. [Crossref] [PubMed]
- Alazami AM, Kentab AY, Faqeih E, et al. A novel syndrome of Klippel-Feil anomaly, myopathy, and characteristic facies is linked to a null mutation in MYO18B. J Med Genet 2015;52:400-4. [Crossref] [PubMed]
- Karaca E, Yuregir OO, Bozdogan ST, et al. Rare variants in the notch signaling pathway describe a novel type of autosomal recessive Klippel-Feil syndrome. Am J Med Genet A 2015;167A:2795-9. [Crossref] [PubMed]
- Tassabehji M, Fang ZM, Hilton EN, et al. Mutations in GDF6 are associated with vertebral segmentation defects in Klippel-Feil syndrome. Hum Mutat 2008;29:1017-27. [Crossref] [PubMed]
- Chacón-Camacho O, Camarillo-Blancarte L, Pelaez-González H, et al. Klippel-Feil syndrome associated with situs inversus: description of a new case and exclusion of GDF1, GDF3 and GDF6 as causal genes. Eur J Med Genet 2012;55:414-7. [Crossref] [PubMed]
- Nigjeh EN, Chen R, Brand RE, et al. Quantitative Proteomics Based on Optimized Data-Independent Acquisition in Plasma Analysis. J Proteome Res 2017;16:665-76. [Crossref] [PubMed]
- Reubsaet L, Sweredoski MJ, Moradian A. Data-Independent Acquisition for the Orbitrap Q Exactive HF: A Tutorial. J Proteome Res 2019;18:803-13. [Crossref] [PubMed]
- Parker SJ, Stotland A, MacFarlane E, et al. Proteomics reveals Rictor as a noncanonical TGF-β signaling target during aneurysm progression in Marfan mice. Am J Physiol Heart Circ Physiol 2018;315:H1112-26. [Crossref] [PubMed]
- Kobayashi D, Tokuda T, Sato K, et al. Identification of a Specific Translational Machinery via TCTP-EF1A2 Interaction Regulating NF1-associated Tumor Growth by Affinity Purification and Data-independent Mass Spectrometry Acquisition (AP-DIA). Mol Cell Proteomics 2019;18:245-62. [Crossref] [PubMed]
- Folkesson E, Turkiewicz A, Rydén M, et al. Proteomic characterization of the normal human medial meniscus body using data-independent acquisition mass spectrometry. J Orthop Res 2020;38:1735-45. [Crossref] [PubMed]
- Schieffer KM, Varga E, Miller KE, et al. Expanding the clinical history associated with syndromic Klippel-Feil: A unique case of comorbidity with medulloblastoma. Eur J Med Genet 2019;62:103701. [Crossref] [PubMed]
- Dauer MVP, Currie PD, Berger J. Skeletal malformations of Meox1-deficient zebrafish resemble human Klippel-Feil syndrome. J Anat 2018;233:687-95. [Crossref] [PubMed]
- Liu J, Wu N, Yang N, et al. TBX6-associated congenital scoliosis (TACS) as a clinically distinguishable subtype of congenital scoliosis: further evidence supporting the compound inheritance and TBX6 gene dosage model. Genet Med 2019;21:1548-58. [Crossref] [PubMed]
- Wu N, Ming X, Xiao J, et al. TBX6 null variants and a common hypomorphic allele in congenital scoliosis. N Engl J Med 2015;372:341-50. [Crossref] [PubMed]
- Cho W, Shepard N, Arlet V. The etiology of congenital scoliosis: genetic vs. environmental-a report of three monozygotic twin cases. Eur Spine J 2018;27:533-7. [Crossref] [PubMed]
- Klimo P, Rao G, Brockmeyer D. Congenital anomalies of the cervical spine. Neurosurg Clin N Am 2007;18:463-78. [Crossref] [PubMed]
- Kim DI, Eo HS, Joh JH. Differential expression of immunoglobulin kappa chain constant region in human abdominal aortic aneurysm. J Surg Res 2005;127:118-22. [Crossref] [PubMed]
- Schmidt M, Hellwig B, Hammad S, et al. A comprehensive analysis of human gene expression profiles identifies stromal immunoglobulin κ C as a compatible prognostic marker in human solid tumors. Clin Cancer Res 2012;18:2695-703. [Crossref] [PubMed]
- Pandey JP, Namboodiri AM, Armeson KE, et al. IGHG, IGKC, and FCGR genes and endogenous antibody responses to GARP in patients with breast cancer and matched controls. Hum Immunol 2018;79:632-7. [Crossref] [PubMed]
- Pandey JP, Kistner-Griffin E, Iwasaki M, et al. Genetic markers of immunoglobulin G and susceptibility to breast cancer. Hum Immunol 2012;73:1155-8. [Crossref] [PubMed]
- Oxelius VA, Pandey JP. Human immunoglobulin constant heavy G chain (IGHG) (Fcγ) (GM) genes, defining innate variants of IgG molecules and B cells, have impact on disease and therapy. Clin Immunol 2013;149:475-86. [Crossref] [PubMed]
- Elovaara H, Kidron H, Parkash V, et al. Identification of two imidazole binding sites and key residues for substrate specificity in human primary amine oxidase AOC3. Biochemistry 2011;50:5507-20. [Crossref] [PubMed]
- Carpéné C, Les F, Hasnaoui M, et al. Anatomical distribution of primary amine oxidase activity in four adipose depots and plasma of severely obese women with or without a dysmetabolic profile. J Physiol Biochem 2016;73:475-86. [Crossref] [PubMed]
- Kale E, Kale A. Amniotic fluid amino acid concentrations in fetal skeletal dysplasia. Clin Exp Obstet Gynecol 2014;41:280-2. [PubMed]
- Hale AJ, Ter Steege E, den Hertog J. Recent advances in understanding the role of protein-tyrosine phosphatases in development and disease. Dev Biol 2017;428:283-92. [Crossref] [PubMed]
- Lefebvre P, Benomar Y, Staels B. Retinoid X receptors: common heterodimerization partners with distinct functions. Trends Endocrinol Metab 2010;21:676-83. [Crossref] [PubMed]
- Menéndez-Gutiérrez MP, Rőszer T, Fuentes L, et al. Retinoid X receptors orchestrate osteoclast differentiation and postnatal bone remodeling. J Clin Invest 2015;125:809-23. [Crossref] [PubMed]
- Rőszer T, Menéndez-Gutiérrez MP, Cedenilla M, et al. Retinoid X receptors in macrophage biology. Trends Endocrinol Metab 2013;24:460-8. [Crossref] [PubMed]
- He Q, Wang F, Fan Y, et al. Differential effects of and mechanisms underlying the protection of cardiomyocytes by liver-X-receptor subtypes against high glucose stress-induced injury. Biochem Biophys Res Commun 2018;503:1372-7. [Crossref] [PubMed]
- Son YL, Lee YC. Molecular determinants of the interactions between SRC-1 and LXR/RXR heterodimers. FEBS Lett 2010;584:3862-6. [Crossref] [PubMed]
- Shen Q, Bai Y, Chang KC, et al. Liver X receptor-retinoid X receptor (LXR-RXR) heterodimer cistrome reveals coordination of LXR and AP1 signaling in keratinocytes. J Biol Chem 2011;286:14554-63. [Crossref] [PubMed]
- Wang B, Tontonoz P. Liver X receptors in lipid signalling and membrane homeostasis. Nat Rev Endocrinol 2018;14:452-63. [Crossref] [PubMed]
- Yang C, Zhou C, Li J, et al. Quantitative proteomic study of the plasma reveals acute phase response and LXR/RXR and FXR/RXR activation in the chronic unpredictable mild stress mouse model of depression. Mol Med Rep 2018;17:93-102. [PubMed]
- Zhao X, Liang M, Li X, et al. Identification of key genes and pathways associated with osteogenic differentiation of adipose stem cells. J Cell Physiol 2018;233:9777-85. [Crossref] [PubMed]
- Zhu Q, Wu N, Liu G, et al. Comparative analysis of serum proteome in congenital scoliosis patients with TBX6 haploinsufficiency - a first report pointing to lipid metabolism. J Cell Mol Med 2018;22:533-45. [Crossref] [PubMed]
- Nogami H, Ogasawara N, Kasai T, et al. Lipid storage myopathy associated with scoliosis and multiple joint contractures. Acta neuropathol 1983;61:305-10. [Crossref] [PubMed]
- van Gastel N, Stegen S, Eelen G, et al. Lipid availability determines fate of skeletal progenitor cells via SOX9. Nature 2020;579:111-7. [Crossref] [PubMed]
- Rendina-Ruedy E, Rosen CJ. Lipids in the Bone Marrow: An Evolving Perspective. Cell Metab 2020;31:219-31. [Crossref] [PubMed]
- Vasiljevski ER, Summers MA, Little DG, et al. Lipid storage myopathies: Current treatments and future directions. Prog Lipid Res 2018;72:1-17. [Crossref] [PubMed]
- Mahley RW, Innerarity TL, Rall SC, et al. Plasma lipoproteins: apolipoprotein structure and function. J Lipid Res 1984;25:1277-94. [Crossref] [PubMed]
- Babin PJ, Thisse C, Durliat M, et al. Both apolipoprotein E and A-I genes are present in a nonmammalian vertebrate and are highly expressed during embryonic development. Proc Natl Acad Sci U S A 1997;94:8622-7. [Crossref] [PubMed]
- Niemeier A, Schinke T, Heeren J, et al. The role of apolipoprotein E in bone metabolism. Bone 2012;50:518-24. [Crossref] [PubMed]
- Zhang L, Li P, Tang Z, et al. Effects of GLP-1 receptor analogue liraglutide and DPP-4 inhibitor vildagliptin on the bone metabolism in ApoE mice. Ann Transl Med 2019;7:369. [Crossref] [PubMed]
- Papachristou NI, Blair HC, Kalyvioti ES, et al. Western-type diet differentially modulates osteoblast, osteoclast, and lipoblast differentiation and activation in a background of APOE deficiency. Lab Invest 2018;98:1516-26. [Crossref] [PubMed]
- Hubaud A, Pourquié O. Signalling dynamics in vertebrate segmentation. Nat Rev Mol Cell Biol 2014;15:709-21. [Crossref] [PubMed]