Deep learning for differentiating novel coronavirus pneumonia and influenza pneumonia
Introduction
In December 2019, a cluster of idiopathic pneumonia cases emerged in Wuhan, China. These cases were eventually identified as novel coronavirus pneumonia (NCP), namely coronavirus disease 2019 (COVID-19). In late January 2020, the outbreak was declared a global health emergency by the World Health Organization. The virus responsible for NCP, severe acute respiratory syndrome coronavirus 2 (SARS-CoV-2) (1), can be transmitted from person to person, and in severe cases, can progress rapidly, leading to sepsis or multiple organ failure (2). The human health crisis that has resulted from the spread of SARS-CoV-2 is unprecedented.
Emerging seasonal influenza viruses are also a critical cause of contagious respiratory disease across the globe that could potentially lead to hospitalization and mortality (3,4). As well as sharing non-specific onset symptoms, such as sudden fever, cough, sore throat, and headache, patients with viral pneumonia (VP) caused by SARS-CoV-2 and influenza infection have similar laboratory findings; consequently, the viruses have been widely considered to be clinically indistinguishable (5,6). Therefore, early diagnosis and differentiation between NCP and influenza pneumonia (IP) are of the utmost importance.
Real-time reverse transcriptase-polymerase chain reaction (RT-PCR) was initially used to confirm the clinical diagnosis of NCP; however, it was reported to have a high false negative rate, owing to a low viral load at the early stage of infection or possibly genetic mutations, and further testing using specimens from multiple sites was usually required for confirmation (7-9). Meanwhile, some early-onset NCP patients who had presented with abnormal findings on chest computed tomography (CT) were found to return negative results on the initial nucleic acid test. Therefore, early differentiation of IP patients from NCP patients based on chest CT would provide another diagnostic approach that could potentially reduce mortality rates and the risk of cross infection while patients await laboratory confirmation.
Imaging is routinely performed to help detect and differentiate disease in patients suspected of having VP, with common radiological features being unilateral or patchy bilateral areas of consolidation, nodular opacities, bronchial wall thickening, and lobar consolidation (10). However, the radiologic manifestations of VP are nonspecific, and it is challenging for clinicians to differentiate NCP from IP due to their similar imaging features. Despite its high sensitivity (97%) in screening for NCP, chest CT has a poor performance in differential diagnosis, with a specificity of 25% (7).
The problem of differentiating between NCP and IP could potentially be alleviated by deep learning, a technique that was formerly used to automatically detect pneumonia based on chest X-ray images and to discriminate usual interstitial pneumonia from nonspecific interstitial pneumonia based on chest CT images (11-13). Deep learning is considered to be worthwhile in terms of differentiation, as it can achieve expert-level performance in medical image analysis with minimal demands on time and labor, thus optimizing the allocation of medical resources.
In this study, we developed and validated an integrated deep learning framework on chest CT images for the automatic detection of NCP, particularly focusing on differentiating NCP from IP at the early stage to ensure prompt implementation of isolation. An intrinsic difference was found in deep learning classification models trained by different devices from multiple centers (13,14). To solve the problem, we proposed a deep learning model (the Trinary scheme) to discriminate IP lesions from NCP lesions by learning image features that reflect differences among devices or hospitals. We present the following article in accordance with the STARD reporting checklist (available at http://dx.doi.org/10.21037/atm-20-5328).
Methods
Patients
The study was conducted in accordance with the Declaration of Helsinki (as revised in 2013). This study was reviewed and approved by the Ruijin Hospital Ethics Committee (2017-186). The requirement for written informed consent was waived due to the retrospective nature of this study.
From January 11, 2020 to February 23, 2020, NCP patients with a confirmed positive result of SARS-CoV-2 via laboratory testing of respiratory secretions by nucleic acid in double swab tests through RT-PCR from 4 hospitals were consecutively enrolled in this study. All included participants had undergone CT scans within 4 days of admission to hospital. Patients with negative CT findings for pneumonia were excluded. Finally, 148 NCP patients were recruited. Among them, 15 patients from one hospital underwent 2 CT scans within 4 days due to rapid disease progression, while the rest of the patients underwent CT scans only once. Chest abnormalities for NCP patients included bilateral pulmonary parenchymal ground-glass opacity and consolidation, sometimes presenting as rounded morphology and peripheral lung distribution (15). Each CT scan was considered as a case. Therefore, there were 163 cases (148 patients) analyzed.
From May 2015 to February 2020, patients confirmed with influenza infection by RT-PCR from 4 hospitals were enrolled as the IP group. Patients who presented negative pneumonia findings on CT and/or co-infection with other virus or bacteria were excluded. Finally, 194 IP patients were included in this study. All IP patients underwent CT scanning once.
The clinical indices collected for all participants included sex, age, and symptoms (including cough and fever). All the patients who participated in the training and tuning of the deep learning algorithms for classification were randomly divided into a training set, a validation set, and a testing set.
To further validate the generalization of the deep learning framework, 57 NCP patients from 8 hospitals and 50 IP patients from 1 hospital were enrolled as an external validation set.
The patient recruitment process is shown in Figure 1. The average time intervals between CT scans and positive RT-PCR test results for all NCP patients and IP patients were 10.8±3.2 and 3.3±0.8 days, respectively. Figure 2 shows a flowchart of this study.

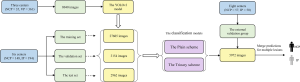
Computation process overview
First, lesion level computation was performed, followed by patient-level computation based on the lesion level results. The lesion level computation comprised 4 parts: (I) lesion detection using YOLOv3; (II) lesion classification using a modified VGGNet model; (III) a Trinary training scheme was introduced, which had the aim of lessening the influence of CT machines on the model and improving lesion classification; (IV) a widely used transfer learning method that was adopted to achieve better model performance by fine-tuning a pre-trained classification model on public non-medical images. Each part is described in detail in the following sections.
Lesion detection
For lesion detection, 35 NCP patients (50 cases) randomly selected from 3 hospitals and 361 patients (361 cases) with VP were enrolled. The 361 VP patients were diagnosed according to the 2016 Clinical Practice guidelines by the Chinese Thoracic Society (16) and the 2007 Infectious Diseases Society of America (IDSA)/American Thoracic Society (ATS) guidelines (17). The CT parameters are presented in Table 1.
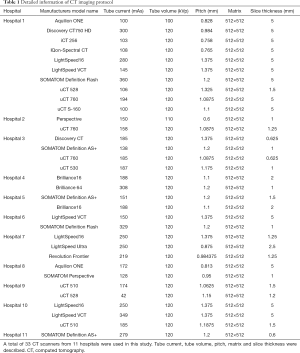
Full table
Annotation
In our study, the lesion regions of each CT image were independently annotated by 2 radiologists individually with more than 10 years of experience in pulmonary-thoracic disease. Both radiologists were aware of the clinical history of infection. All lesion annotations by the 2 radiologists were collected. If a lesion was annotated by only 1 of the radiologists, a consensus was reached between the pair.
Preprocessing
Before the lesions were detected, the CT images were preprocessed through the following steps to remove irrelevant parts. First, an in-house tool was used to segment the lung region. Second, the convex hull of the lung region was calculated independently for each slice of the CT image. Then, the smallest bounding box that could cover all the convex hulls was calculated. Next, the CT image was cropped using the calculated bounding box. Finally, only the slices that contained ≥1 lesions were preserved to train the lesion detection model.
To reduce the influence of CT image spacing, all CT images with a spacing of <5 mm were transformed into 5 mm by taking the average of continuous slices. Specifically, to transform CT images with a spacing of 1.25 mm, the mean of every 4 continuous slices was taken as 1 slice. The spacing distribution was as follows. First, all preprocessed images were resized to 256×256 to fit the data into the YOLOv3 model, and then further randomly placed on a 416×416 template. During training, all the input images were randomly flipped.
Dataset partition
Ten patients with NCP (15 CT series) and 40 patients (40 CT series) with VP were randomly selected as the test set. The remaining patients (24 patients with NCP and 321 patients with VP) were used for training and validation. After the removal of image slices without lesions, there were a total of 8,040 image slices in the training and validation sets, and 10% of them were randomly selected as the validation set. Due to randomness, only 1 NCP patient (1 CT series) was included in the validation set. Therefore, there were 23 NCP patients (35 CT series) in the training set. For NCP and VP, there were 793 and 2,021 images, respectively, in the test set, and 3,277 and 2,695 images, respectively, in the external validation set after preprocessing.
Lesion detection model and training details
YOLOv3, a highly efficient and widely used object detection network in computer vision, was employed to perform lesion detection on the preprocessed images (18). In our dataset, lesions were found in only 34% and 36% of the slices for NCP and VP, respectively. The YOLOv3 model can identify a limited number of candidate regions by ruling out most of the irrelevant healthy regions. Removing slices and regions without lesions can reduce the potential of the model to overfit, especially when the training data are limited, as they were in our case. The YOLOv3 architecture pre-trained to 33% mAP on the COCO dataset was used in this study, and we retrained it on our dataset. The parameters were fine-tuned across all layers with a learning rate of 0.0001, where the learning rate was adjusted by step policy. A decay factor of 0.0005 and a momentum factor of 0.9 were used in our model. Due to the multiple-scale method of feature pyramid networks (FPN), YOLOv3 output feature maps are of 3 different scales. The output of YOLOv3 may contain multiple bounding boxes, which may be false positive results or overlapping results. Therefore, the score threshold was set at 0.2 to reduce the number of bounding boxes of the output.
Lesion level classification
Preprocessing
In our work, a classification model for classifying NCP and IP was built. We randomly selected 80% of patients as the training set, 10% as the validation set, and 10% as the test set. Specifically, 118 patients with NCP (132 CT series) and 157 patients with IP (157 CT series) for training, 14 patients with NCP (15 CT series) and 19 patients with IP (19 CT series) for validation, and 16 patients with NCP (18 CT series) and 18 patients with IP (18 CT series) were incorporated for testing. After preprocessing, there were 27,605 and 3,154 images in the training and validation sets, respectively. There were 2,962 images (4,683 annotated lesions) containing ≥1 lesions in the test set. All lesions in an NCP patient were considered as NCP lesions, and all lesions in an IP patient were considered to be IP lesions. For an annotated lesion, a random 128×128 patch was cropped from the CT image around the lesion so that the lesion was always in the cropped patch. If the lesion was larger than 128×128, the CT image was magnified before cropping.
Classification model
Due to the limited number of training samples, VGGNet was chosen as the classification model (19). The neural network structure VGGNet is relatively old and simple, and can be improved using AlexNet. The network consisted of 5 convolution groups and 1 max pooling layer, followed by 3 fully connected layers and 1 softmax layer. The entire network used the same convolution kernel size (3×3) and maximum pooling size (2×2).
To better address our problem, some modifications were made to the original VGGNet. Specifically, first, the 3 fully connected layers and one softmax layer were removed, followed by the max pooling layer. Then, 1 convolution layer with rectified linear unit (ReLU), 1 fully connected layer with ReLU, and 1 softmax layer were added. ReLU is the most commonly used activation function in deep learning methods and can be written as:
[1]
where x is the input to a neuron.
These added modules are denoted as “head” (Figure 3).
Trinary classification scheme
To distinguish NCP and IP, the classification model was trained using NCP and IP image patches. This training process is referred to as the Plain scheme herein. We found that the Plain scheme may have been influenced by device-specific features and did not generalize well. Therefore, a novel training scheme, referred to as the Trinary scheme, was introduced as follows.
In the Trinary scheme, we started by collecting random regions from the CT images and then generated random bounding boxes. These random regions were assumed to share the same device-specific features with lesions from the same device, and the randomly generated bounding box regions were not overlapping with lesions with a high probability. The only difference between the random regions and the lesions was whether a lesion was present. Then, the binary classification problem was revised to a trinary classification problem, and the 3 classes corresponded to NCP lesions, IP, lesions, and random regions.
Cross-entropy, which characterizes the distance between 2 probability distributions and is commonly used in multi-classification problems, was used as the classification loss function (20):
[2]
Where pi is the output probability for class i, y is the benchmark label from {0, 1, 2}, and δ(·) is the Dirac’s delta function. For hard instance mining, we used focal loss (21):
[3]
Where (1−pi)y is a modulating factor, y is a focusing parameter, and we set y to 2. Focal loss suppressed the weight of samples that are easy to classify during training calculation loss, so that the model could focus on learning to distinguish those samples that could not be distinguished easily. The final loss function is:
[4]
Transfer learning
Transfer learning was used based on previous reports. The training of the modified VGGNet model was divided into two steps (22,23). Firstly, the preserved original VGGNet (5 convolution groups and 1 max pooling layer) was frozen (without back propagation or updated parameters), and only the “head” module was trained. An Adam optimizer with a learning rate of 0.0001 was used. Secondly, after 70 iterations, the entire network was fine-tuned. Because the training sets for NCP or IP were imbalanced, the NCP lesion images were up-sampled to balance the training data. For the Trinary scheme, 1 random region was inserted for every 3 NCP or IP lesions.
Patient-level classification
Taking the Plain model as an example, the classification model outputs two probabilities corresponding to NCP and IP for each lesion. To obtain patient-level prediction from lesion level predictions, the lesion level probabilities of a patient were averaged. For Trinary scheme, the probabilities for NCP and IP were further normalized such that they were summed to be one.
The rationale behind taking the average was as follows. If each lesion classification was considered to be the result of a weak classifier for the patient (24), taking the average provides an efficient ensemble method of obtaining a strong classifier. Many popular machine learning methods have utilized such an ensemble method. For instance, the well-known random forest classification model would take the average of the probabilities of multiple decision trees to achieve a better performance than a single decision tree (25). In our experiments, the patient-level classification was also observed to have higher accuracy than the lesion-level classification.
Comparison with experts on the external validation set
To compare the performances of the deep learning framework and radiologists in the external validation set, a panel of 10 radiologists from two groups (group 1, 5 specialist-level radiologists with more than 15 years of experience; group 2, 5 resident radiologists with 3–5 years of experience) in thoracic imaging were recruited. The radiologists were completely blinded to the clinical information and histological findings of the participants (they were aware that the patients had either NCP or IP but were completely blinded to the location and distribution). Each radiologist had access to digital imaging and communications in medicine (DICOM) series. All cases were anonymized, randomly assigned case numbers from 1 to 107, and randomly divided into two groups with 54 cases and 53 cases respectively. The 10 radiologists interpreted 1 group of images each time. Each reading session was separated by 1 day. To mimic the routine diagnostic process, each session was required to be finished within 1 day. The evaluation was repeated after 1 week with disorganized groups different from the first time to avoid possible interobserver variation. The radiologists were instructed to independently provide a classification decision of NCP or IP each time. The final decision was not made until a consensus was reached between the two assessments. The performance of both individual radiologists and the radiologists as a group were compared with that of the Plain scheme and Trinary scheme. The consistency between the radiologist group and the schemes was also recorded.
To determine which scheme was more akin to the judgement of human experts, we also performed the lesion re-classification process by radiologists, for there was no standard for reference. First, any lesion with inconsistent classification between the two schemes was selected for further evaluation. To eliminate potential interference factors (e.g., other lesions), only an individual lesion, instead of the whole image, was selected. Second, the lesions were re-assessed by two senior specialists, who had the highest area under the curves (AUCs) and were blinded to the results of the algorithms. The classification of NCP or IP was recorded for all selected lesions, and the final decision for lesion triage was made by consensus of the specialists. Lastly, the consistency was calculated between human experts and the algorithms.
Statistical analysis
Clinical indices were analyzed based on the variable type. Continuous variables were measured by means or medians and were compared using two-sided independent t tests or Wilcoxon rank-sum tests. Categorical variables were measured as proportions and were compared using chi-square tests or Fisher’s exact tests. The classification metrics used included AUC, sensitivity, specificity, accuracy, precision, and F1 score. 95% confidence interval (CI) was used to handle indeterminacy. The intraclass correlation coefficient (ICC) was used to evaluate interobserver agreement among radiologists. The mean values of the classification metrics were computed across the specialist group as well as the resident group. A statistically significant difference was indicated when results with two-sided P<0.05. Data analysis was performed using Python (version 3.7.4, http://www.r-project.org) and MedCalc (version 1.13.1, MedCalc Software Ltd., Ostend, Belgium). The deep learning models were implemented based on Tensorflow (version 1.13.1, https://www.tensorflow.org) framework.
Results
Patient information
Among the 148 patients with NCP, 53.7% were men, which showed no significant difference with the IP patients (57.7%, P=0.43). With a median age of 51.5 years (IQR, 42.5–63.0), the NCP patients were significantly younger than the IP patients, (P<0.01). Fewer NCP patients than IP patients exhibited coughing (50% vs. 73.2%, P<0.01). The information for the external validation set is presented in Table 2.
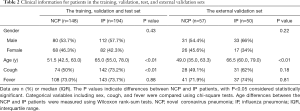
Full table
Comparison of imaging features between IP and NCP
A joint analysis was further performed of the imaging features of annotated lesions from 148 NCP patients and 194 IP patients. Because a three-dimensional lesion may appear in multiple layers of the CT images, to perform comparison, the annotated lesions that had overlapped bounding boxes were merged. There were 1,669 and 1,568 NCP and IP merged lesions, respectively. To simplify the description, ‘merged lesion’ is simplified as ’lesion’ in this section.
Of the NCP lesions, 96.3% were >1 cm, were significantly more NCP lesions than IP lesions >1 cm (P<0.01). However, NCP was more likely to form moderate-sized lesions, and only 28.8% of these lesions were >3 cm. There was a similar distribution of abnormal features in the lower lobes between NCP (42%) and IP (42.8%). Lesions with an intensity <–500 Hu accounted for 80.4% of NCP lesions. In comparison, 40.1% of IP patients had a focus density >–500 Hu, indicating that NCP had less consolidation than IP. Significantly more extensive distribution was demonstrated by NCP than IP, with 86.5% of patients presenting bilateral pulmonary invasion and 35.0% showing involvement of 5 lobes. Of 1568 IP lesions, 4.4% were nodules (Hu >0), of which 60.9% had CT values >40 Hu, and 10 (0.6%) IP nodules were >5 mm. In contrast, only 0.4% (6/1,669) of NCP lesions presented as nodules (Table 3). Examples of differences in CT imaging features between an NCP patient and an IP patient are shown in Figures 4 and 5.
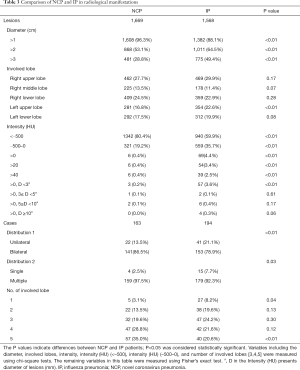
Full table
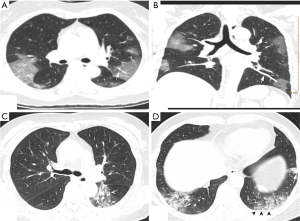
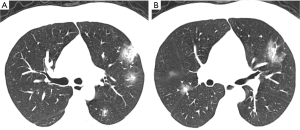
Lesions could be effectively detected by YOLOv3
To evaluate the YOLOv3 model, intersection over union (IOU) was used as the match measure for measuring the detection accuracy. IOU is an evaluation metric which computes the intersection over the union of two bounding boxes. For a lesion annotated by specialists, if its bounding box was overlapped with a predicted bounding box with an IOU ≥0.2, this lesion was considered as having been detected. Examples of detected lesions are presented in Figure 6. For each predicted candidate lesion, the model gave a confidence score. Generally, a high confidence score led to a low false positive rate as well as a low recall rate. However, when the confidence score was extremely low, the recall rate also dropped due to highly overlapping predictions (Table 4). The results showed that the detection performance was not sensitive to the confidence score as long as the cutoff for confidence score was in a reasonable range. For example, if the confidence score cutoff was set to 0.1, the false positive rate was 0.216, the recall rate was 0.704, and the F1 was 0.742. If the confidence score was in the range of (0.03–0.25), the F1 score was always >0.7. In the external validation set, the model detected 2,984 and 3,811 NCP and IP lesions, respectively. These detected lesions were used in the following analysis.
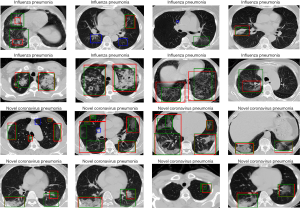
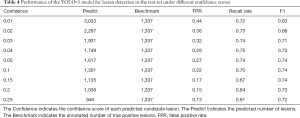
Full table
Lesions could be accurately classified by the modified VGGNet model
The annotated lesions were used to train and evaluate the modified VGGNet classification model. Both Plain and Trinary training schemes were applied. The Trinary scheme (AUC: 0.93) performed better than the Plain scheme (AUC: 0.85) (Figure 7). The F1 scores for the Plain and Trinary schemes were 0.65 and 0.79, respectively (Table 5).
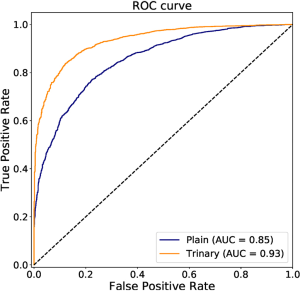
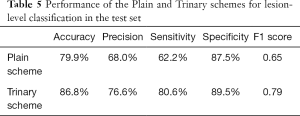
Full table
Trinary scheme was more consistent with specialists on lesion classification
To better understand the difference between the Plain and Trinary schemes, the predicted NCP probability from both schemes was compared using lesions identified in the external validation set. Generally, we found that the Trinary scheme is less influenced by the source of the data. More specifically, for lesions from hospitals in the training set, we found that the Plain scheme may give a very high NCP probability, even if for lesions were not very typical of NCP (Figure 8A). On the contrary, for lesions from hospitals not in the training set, the Plain scheme may give a very low NCP probability, even if the lesions were extremely typical of NCP (Figure 8B). In contrast, the Trinary scheme seemed to give more intuitively reasonable predictions. To quantify the difference, detected lesions on which the absolute difference of predicted NCP probability between the Trinary and Plain schemes was >0.5 were filtered out. These lesions were subsequently annotated by 2 specialists. A lesion was considered as NCP or IP only when it was annotated as such by both specialists. Of 812 filtered lesions, 540 and 102 of them were annotated as NCP and IP, respectively. Out of 540 NCP lesions, the Trinary and Plain schemes correctly identified 366 and 174, respectively. Out of 102 IP lesions, 61 and 41 lesions were correctly identified by the Trinary and Plain schemes, respectively. The differences between the two schemes were statistically significant with P=0.0076 and P<2.2e-16 for IP and NCP lesions, respectively. Therefore, the Trinary scheme was more consistent with specialists than the Plain scheme on lesion-level classification.
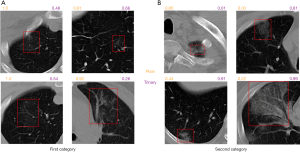
Performance of patient-level classification by human experts
For the external validation set, an average of 8.1 h was required for 10 radiologists to differentiate NCP and IP in all 107 patients. For the specialist group, the average time was 6.8 h (5.3–7.4 h). For the resident group, the average time was 8.9 h (7.5–10.2 h). For the specialist group, micro-average sensitivity and specificity of 71.2% (95% CI, 57.8–84.7%) and 89.2% (95% CI, 80.5–97.9%) were reached, respectively. For the resident group, the mean sensitivity and specificity were 57.2% (95% CI, 51.3–63.1%) and 76.8% (95% CI, 68.8–84.8%), respectively. The mean AUC of the specialist group was 0.802, which was significantly better than that of the resident group (AUC 0.67, P<0.0001). The mean F1 scores for the specialist group and the resident group were 0.79 and 0.64, respectively (Table 6). Both the specialist group and the resident group were found to have good consistency, with ICCs of 0.899 and 0.798, respectively. The correlation for 10 radiologists is presented in Table 7.
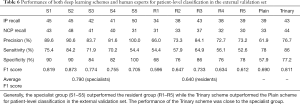
Full table
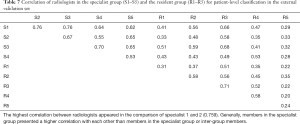
Full table
Trinary scheme outperformed the specialist group on patient-level classification
For the Plain and Trinary schemes, it took an average of 10 seconds to detect and classify all detected lesions for a single patient. The receiver operating characteristic (ROC) curves for the test set and the external validation set of both training schemes are shown in Figure 9. For the test set, the Plain and Trinary schemes achieved AUCs of 0.86 and 0.95, respectively (Figure 9A). The AUCs were similar those for the lesion level. For the Plain and Trinary schemes, the sensitivities were 72.2% and 88.9%, respectively, and the specificities were 72.2% and 94.4%, respectively (Table 8).
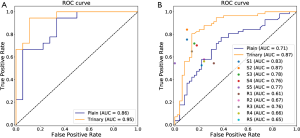

Full table
For the external validation set, the sensitivity and specificity of the Plain scheme were 78% and 57.9%, respectively, compared with 86% and 77.2%, respectively, for the Trinary scheme. Compared with the mean sensitivity (71.2%) and specificity (89.2%) of 5 specialists, the deep learning model achieved higher sensitivity but lower specificity. The F1 scores for the Trinary scheme and Plain scheme reached 0.690 and 0.811, respectively, and the Trinary outperformed 9 human radiologists. Generally, the Trinary scheme achieved specialist-level performance while the Plain scheme achieved resident-level performance (Figure 9B). Our Trinary scheme correctly classified 13 (22.8%) participants with NCP that were misdiagnosed by at least 3 specialists. Among them, 2 patients displayed CT findings less frequently reported in other NCP cases, such as a small mixed ground-glass opacity in the center, or solitary consolidation. These patients were misdiagnosed by 5 specialists (Figure 10). The results indicated that both of the schemes achieved human expert level (Table 6). The Trinary scheme achieved an F1 score of 0.811, which was higher than that of the Plain scheme (0.690), and also achieved a similar level of performance to the human experts (specialist group, average 0.790; resident group, average 0.640) (Table 6).
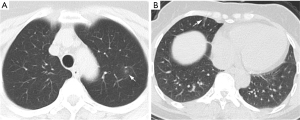
The correlations between the predictions of the two schemes and radiologists were further examined in the external validation set (Table 9). The Trinary scheme outperformed the Plain scheme, as higher correlations were observed in all radiologists. Generally, both the Plain and the Trinary scheme were better correlated with 5 experienced specialists, especially with specialist 1 and specialist 2 in both categories. Specialists 1 and 2 performed best in differential diagnosis for the external validation set; therefore, the Trinary scheme was better correlated with specialists in the external validation set.
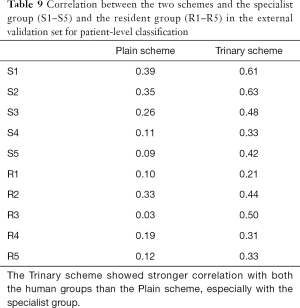
Full table
Discussion
In this study, we established an integrated artificial intelligence (AI) framework for differential diagnosis between NCP and IP, which showed a good performance in the automatic detection of disease lesions, classification of lesions, and differential diagnosis of patients even at the early stage. This AI effort was driven by the phenomenon of misdiagnosis of influenza in COVID-19 deaths in multiple countries during the escalating pandemic. The similarities in clinical symptoms between these two types of VP, along with the shortage and high false negative rate of nucleic acid detection kits, make the differential diagnosis difficult (26-28) and has prompted the search for new diagnostic methods. Meanwhile, the high sensitivity of chest CT gives it an advantage in diagnosing NCP at an early stage, which encouraged the Hubei Provincial Government to adopt characteristic chest CT findings as an important criterion for diagnosis of NCP at the peak of outbreak. In the present climate, our AI framework indicated improvements in accuracy and speed in identifying specific lesions on chest CT images, and thus provides another predominant diagnostic tool to assist clinicians and radiologists.
Recent studies have reported the value of deep learning in differentiating NCP from various other types of pneumonia (29-31). In this study, we focused on discriminating NCP and pneumonia infected with influenza virus, which present extremely similar symptoms and demonstrations. Both viruses can be quickly transmitted through person-to-person contact, droplets, and fomites. The serial interval for SARS-CoV-2 is estimated to be 6–9 days, compared with 3 days for influenza (32,33). Further, pre-symptomatic viral transmission is possible in both types of viruses, which increases the difficulty of precautionary measures. While there are several clinical trials and more than 20 vaccines in development for SARS-CoV-2, there is currently no licensed vaccine or antiviral medication for NCP. On the contrary, medicaments and vaccines are available for influenza, but some patients who have been vaccinated can still be infected by influenza, and early use of antivirals is essential (34). The high transmissibility, lethality, and difficulty in treating both viruses make the early clinical identification particularly important.
Unfortunately, the diagnosis of VP still relies heavily on clinician suspicion, which is based on host risk factors, presentation, and exposure, and thus makes different subtypes of viruses rather indistinguishable. Patients who are infected with influenza or SARS-CoV-2 could show a similar range of symptoms, such as sudden onset fever, cough, sore throat, fatigue, and myalgia (1,35). Laboratory findings also show similar results, including normal or lower levels of leucocytes, decreased lymphocyte count, increased C-reactive protein, lower albumin, and higher D-dimer and urea levels (36). Despite some efforts to compare hematological indices between patients with NCP and IP, it is not possible to differentiate based on them due to individual biases. Moreover, patients with severe pneumonia may present with abnormal laboratory findings of damage to multiple organs, including to the heart, liver, or kidney. In a prospective study, clinicians failed to clinically diagnose influenza in approximately 2/3 of influenza-confirmed patients (37), not to mention the difficulty in differentiating it from other types of respiratory viruses on clinical grounds.
Meanwhile, despite the fact that the advent of PCR testing has enormously facilitated the identification of respiratory viruses, the test results usually take days to return, and involve an unavoidable false negative rate (10). In view of that, closer attention to patients’ radiological features is needed from clinicians and radiologists in the search for evidence of influenza or SARS-CoV-2 infection.
Our integrated AI framework provides the possibility for early and differential diagnosis of NCP. Previous research has shown that at the early stage, NCP is difficult to distinguish from IP. Our integrated framework performed well in the automatic detection of disease lesions, the classification of lesions, and patient differential diagnosis, even at the early stage.
Deep learning also suffers from the “black box” problem, which essentially means the results are difficult to explain (38-40). One option is to explain black boxes using various techniques (41), and another is to develop explainable models (42). Although the deep learning pipelines proposed in this work are based on black box models, taking the average of individual lesions to get patient-level prediction obtained some extent of interpretability. The proposed Trinary scheme behaved more similarly to specialists than the Plain deep learning classification model did, providing extra interpretability to the pipeline. The Trinary classification scheme is designed to improve the network from extracting device-specific features during learning. The idea behind the scheme is that if the network only extracted device-specific features, it would lead to a high cost on the random region inputs. Therefore, the scheme forced the trained model to instead extract lesion-specific features. Although it is possible that device-specific features could also be extracted, we demonstrated that such a scheme could clearly improve the classification results and make prediction by the model more consistent with the judgement of experienced specialists. Because the proposed Trinary scheme is task independent, we believe that it can be widely applied to many other medical image classification problems and accelerate the application of deep learning systems to wider clinical usage.
In our study, we found that chest CT images of early-stage NCP manifest a more obvious distribution of ground-glass opacity (GGO) in the lungs, with fewer nodules and consolidation than IP. This result was also confirmed by a recent study comparing CT characteristics between IP and NCP, with NCP showing patchy areas or GGO combined consolidation opacities, with peripheral distribution and balanced lobe predomination, while in IP the lesions were predominantly located in the inferior lobe (7). These findings support the point that chest CT could play an early warning role in the diagnosis and differentiation of NCP, and also provide a basis for further research. At the same time, these subtle differences are quite difficult to uncover through artificial reading and can be easily missed, which makes it necessary to establish an AI model to assist clinical work based on machine learning. We are obliged to admit the fact that the exposure to ionizing radiation is an intrinsic defect of CT. However, compared with other radiological modalities, the role of CT in the detection of NCP is irreplaceable, not only in diagnosis but also in assessing the disease severity, as it provides critical guidance for patients’ treatment. In fact, accumulated evidence has demonstrated the risk related to routine usage of CT in medical care to be low (43,44). Besides, the carcinogenic risk from CT screening for lung cancer, even if non-negligible, should be considered acceptable in light of the substantial reduction in screening-associated mortality (45).
Further analysis of our model results can help us to obtain a better understanding of the pathophysiological origin of NCP. It is presumed that SARS-CoV-2 is able to bind to the ACE-2 receptor in humans, a key component of the renin-angiotensin system (46,47). The ACE-2 receptor is mainly expressed on the surface of alveolar type-2 epithelial cells which, when infected, undergo apoptosis, leading to diffuse alveolar damage and interstitial fluid absorption disorder. This may explain why we found that 80.4% of lesions in NCP were <–500 Hu, 86.5% of patients had bilateral lung damage, and 35.0% of patients had all 5 lung lobes affected. Unlike SARS-CoV-2, influenza viruses primarily damage the tracheal epithelial cells, leading to necrotizing bronchitis and diffuse alveolar damage of the upper respiratory tract (10). This suggests that the trachea and main bronchus should be affected first, resulting in bronchitis and neutrophilic bronchopneumonia at the early stage of infection.
The clinical applicability of our developed AI model was improved by the inclusion of data from multiple centers and machines. In the current study, the training and test data of NCP were from 4 machines in 3 hospitals, and the AI model performed well. The data of our independent verification group were from different machines in 8 hospitals, and both schemes performed well, which suggests that our model has good clinical applicability.
Most of the existing deep learning studies on pneumonia were performed using chest X-ray due to its easy accessibility (11,14,48). However, CT is the major clinical diagnostic method for NCP. A natural concern for the current study was therefore the potentially scarce sample size from CT, which would have supplied insufficient training data for the deep learning models. However, each CT scan can generate multiple images for analysis, and the amount of data can be further increased by manipulations such as image rotations to avoid overfitting. Overall, the performance of our AI system in a multi-center on the independent validation group from multiple centers and machines demonstrates the reliability of our deep learning results.
Conclusions
Currently, SARS-CoV-2 is rampantly spreading around the world, and efficient and accurate diagnosis of NCP is crucial for prevention and control. Our deep learning model potentially supplies an accurate early diagnostic tool for NCP, especially when nucleic acid test kits are in short supply, which commonly happens during outbreaks. This tool could help to reduce the rate of misdiagnosis and diagnosis time, ensure prompt patient isolation and early treatment, improve prognosis, and considerably reduce transmission. The high efficiency of our model in differentiating NCP from IP could be very beneficial to reducing the rate of misdiagnosis and optimizing the allocation of medical resources, particularly in areas with a high prevalence of both NCP and IP. The Trinary scheme not only improved the performance of the model in discriminating NCP from IP, but it also behaved more similarly to specialists than the Plain scheme. Because the proposed Trinary scheme is designed for general use, it could potentially be applied for classifying a wide range of medical images.
Acknowledgments
Funding: This work was supported by National Key R&D Program of China [2017YFC1309701 and 2017YFC1309700 to MZ], Shanghai Key Discipline for Respiratory Diseases [2017ZZ02014 to JQ] and Shanghai Science and Technology Committee [18411953000 to HZ] and Zhejiang University special scientific research fund for COVID-19 prevention and control.
Footnote
Reporting Checklist: The authors have completed the STARD reporting checklist. Available at http://dx.doi.org/10.21037/atm-20-5328
Data Sharing Statement: Available at http://dx.doi.org/10.21037/atm-20-5328
Conflicts of Interest: All authors have completed the ICMJE uniform disclosure form (available at http://dx.doi.org/10.21037/atm-20-5328). The authors have no conflicts of interest to declare.
Ethical Statement: The authors are accountable for all aspects of the work in ensuring that questions related to the accuracy or integrity of any part of the work are appropriately investigated and resolved. The study was conducted in accordance with the Declaration of Helsinki (as revised in 2013). This study was reviewed and approved by the Ruijin Hospital Ethics Committee (2017-186). The requirement for written informed consent was waived due to the retrospective nature of this study.
Open Access Statement: This is an Open Access article distributed in accordance with the Creative Commons Attribution-NonCommercial-NoDerivs 4.0 International License (CC BY-NC-ND 4.0), which permits the non-commercial replication and distribution of the article with the strict proviso that no changes or edits are made and the original work is properly cited (including links to both the formal publication through the relevant DOI and the license). See: https://creativecommons.org/licenses/by-nc-nd/4.0/.
References
- Li Q, Guan X, Wu P, et al. Early Transmission Dynamics in Wuhan, China, of Novel Coronavirus-Infected Pneumonia. N Engl J Med 2020;382:1199-207. [Crossref] [PubMed]
- Wang D, Hu B, Hu C, et al. Clinical characteristics of 138 hospitalized patients with 2019 novel coronavirus-infected pneumonia in Wuhan, China. JAMA 2020;323:1061-9. [Crossref] [PubMed]
- Ly S, Arashiro T. Establishing seasonal and alert influenza thresholds in Cambodia using the WHO method: implications for effective utilization of influenza surveillance in the tropics and subtropics. Western Pac Surveill Response J 2017;8:22-32. [Crossref] [PubMed]
- Livingston E, Bucher K, Rekito A. Coronavirus Disease 2019 and Influenza. JAMA 2020;323:1122. [Crossref] [PubMed]
- Chen L, Han XD, Li YL, et al. Severity and outcomes of influenza-related pneumonia in type A and B strains in China, 2013-2019. Infect Dis Poverty 2020;9:42. [Crossref] [PubMed]
- Kilbourne ED. Influenza pandemics of the 20th century. Emerg Infect Dis 2006;12:9-14. [Crossref] [PubMed]
- Ai T, Yang Z, Hou H, et al. Correlation of Chest CT and RT-PCR Testing in Coronavirus Disease 2019 (COVID-19) in China: A Report of 1014 Cases. Radiology 2020;296:E32-40. [Crossref] [PubMed]
- Lu R, Zhao X, Li J, et al. Genomic characterisation and epidemiology of 2019 novel coronavirus: implications for virus origins and receptor binding. Lancet 2020;395:565-74. [Crossref] [PubMed]
- Wang W, Xu Y, Gao R, et al. Detection of SARS-CoV-2 in Different Types of Clinical Specimens. JAMA 2020;323:1843-4. [Crossref] [PubMed]
- Franquet T. Imaging of pulmonary viral pneumonia. Radiology 2011;260:18-39. [Crossref] [PubMed]
- Hwang EJ, Park S, Jin KN, et al. Development and Validation of a Deep Learning-Based Automated Detection Algorithm for Major Thoracic Diseases on Chest Radiographs. JAMA Netw Open 2019;2:e191095. [Crossref] [PubMed]
- Rajpurkar P, Irvin J, Ball RL, et al. Deep learning for chest radiograph diagnosis: A retrospective comparison of the CheXNeXt algorithm to practicing radiologists. PLOS Med 2018;15:e1002686. [Crossref] [PubMed]
- Walsh SLF, Calandriello L, Silva M, et al. Deep learning for classifying fibrotic lung disease on high-resolution computed tomography: a case-cohort study. Lancet Respir Med 2018;6:837-45. [Crossref] [PubMed]
- Zech JR, Badgeley MA, Liu M, et al. Variable generalization performance of a deep learning model to detect pneumonia in chest radiographs: A cross-sectional study. PLOS Med 2018;15:e1002683. [Crossref] [PubMed]
- Chung M, Bernheim A, Mei X, et al. CT Imaging Features of 2019 Novel Coronavirus (2019-nCoV). Radiology 2020;295:202-7. [Crossref] [PubMed]
- Cao B, Huang Y, She DY, et al. Diagnosis and treatment of community-acquired pneumonia in adults: 2016 clinical practice guidelines by the Chinese Thoracic Society, Chinese Medical Association. Clin Respir J 2018;12:1320-60. [Crossref] [PubMed]
- Mandell LA, Wunderink RG, Anzueto A, et al. Infectious Diseases Society of America/American Thoracic Society consensus guidelines on the management of community-acquired pneumonia in adults. Clin Infect Dis 2007;44 Suppl 2:S27-72. [Crossref] [PubMed]
- Redmon J, Farhadi A. YOLOv3: An Incremental Improvement (serial online) 2018 April (Cited 2020 Mar 19). Available online: https://arxiv.org/abs/1804.02767
- Simonyan K, Zisserman A. Very Deep Convolutional Networks for Large-Scale Image Recognition (serial online) 2014 Sept (Cited 2020 Mar 19). Available online: http://arxiv.org/abs/1409.1556
- Boer P, Kroese D, Mannor S, et al. A Tutorial on the Cross-Entropy Method. Ann Oper Res 2005;134:19-67. [Crossref]
- Lin TY, Goyal P, Girshick R, et al. Focal Loss for Dense Object Detection. IEEE Trans Pattern Anal Mach Intell 2020;42:318-27. [Crossref] [PubMed]
- Liu X, Faes L, Kale AU, et al. A comparison of deep learning performance against health-care professionals in detecting diseases from medical imaging: a systematic review and meta-analysis. Lancet Digit Health 2019;1:e271-97. [Crossref] [PubMed]
- Toba S, Mitani Y, Yodoya N, et al. Prediction of pulmonary to systemic flow ratio in patients with congenital heart disease using deep learning-based analysis of chest radiographs. JAMA Cardiol 2020;5:449-57. [Crossref] [PubMed]
- Sagi O, Rokach L. Ensemble learning: A survey. Wiley Interdiscip Rev Data Min Knowl Discov 2018;8:e1249. [Crossref]
- Breiman L. Random Forests. Mach Learn 2001;45:5-32. [Crossref]
- Chen N, Zhou M, Dong X, et al. Epidemiological and clinical characteristics of 99 cases of 2019 novel coronavirus pneumonia in Wuhan, China: a descriptive study. Lancet 2020;395:507-13. [Crossref] [PubMed]
- Ruuskanen O, Lahti E, Jennings LC, et al. Viral pneumonia. Lancet 2011;377:1264-75. [Crossref] [PubMed]
- Guan WJ, Ni ZY, Liang WH, et al. Clinical Characteristics of Coronavirus Disease 2019 in China. N Engl J Med 2020;382:1708-20. [Crossref] [PubMed]
- Wang S, Zha Y, Li W, et al. A fully automatic deep learning system for COVID-19 diagnostic and prognostic analysis. Eur Respir J 2020;56:2000775. [Crossref] [PubMed]
- Ni Q, Sun ZY, Qi L, et al. A deep learning approach to characterize 2019 coronavirus disease (COVID-19) pneumonia in chest CT images. Eur Radiol 2020;30:6517-27. [Crossref] [PubMed]
- Li L, Qin L, Xu Z, et al. Artificial Intelligence Distinguishes COVID-19 from Community Acquired Pneumonia on Chest CT. Radiology 2020;296:200905. [Crossref]
- Sanche S, Lin YT, Xu C, et al. High Contagiousness and Rapid Spread of Severe Acute Respiratory Syndrome Coronavirus 2. Emerg Infect Dis 2020;26:1470-7. [Crossref] [PubMed]
- Biggerstaff M, Cauchemez S, Reed C, et al. Estimates of the reproduction number for seasonal, pandemic, and zoonotic influenza: A systematic review of the literature. BMC Infect Dis 2014;14:480. [Crossref] [PubMed]
- Orzeck EA, Shi N, Blumentals WA. Oseltamivir and the risk of influenza-related complications and hospitalizations in patients with diabetes. Clin Ther 2007;29:2246-55. [Crossref] [PubMed]
- Stein J, Louie J, Flanders S. Performance characteristics of clinical diagnosis, a clinical decision rule, and a rapid influenza test in the detection of influenza infection in a community sample of adults. Ann Emerg Med 2005;46:412-9. [Crossref] [PubMed]
- Yun H, Sun Z, Wu J, et al. Laboratory data analysis of novel coronavirus (COVID-19) screening in 2510 patients. Clin Chim Acta 2020;507:94-7. [Crossref] [PubMed]
- Dugas AF, Valsamakis A, Atreyam MR, et al. Clinical diagnosis of influenza in the ED. Am J Emerg Med 2015;33:770-5. [Crossref] [PubMed]
- Lundervold AS, Lundervold A. An overview of deep learning in medical imaging focusing on MRI. Z Med Phys 2019;29:102-27. [Crossref] [PubMed]
- Sahiner B, Pezeshk A, Hadjiiski LM, et al. Deep learning in medical imaging and radiation therapy. Med Phys 2019;46:e1-36. [Crossref] [PubMed]
- Topol EJ. High-performance medicine: the convergence of human and artificial intelligence. Nat Med 2019;25:44-56. [Crossref] [PubMed]
- Wang F, Casalino LP, Khullar D. Deep Learning in Medicine-Promise, Progress, and Challenges. JAMA Intern Med 2019;179:293-4. [Crossref] [PubMed]
- Rudin C. Stop explaining black box machine learning models for high stakes decisions and use interpretable models instead. Nat Mach Intell 2019;1:206-15. [Crossref]
- Kuo W, Ciet P, Tiddens HA, et al. Monitoring cystic fibrosis lung disease by computed tomography. Radiation risk in perspective. Am J Respir Crit Care Med 2014;189:1328-36. [Crossref] [PubMed]
- Marant-Micallef C, Shield KD, Vignat J, et al. The risk of cancer attributable to diagnostic medical radiation: Estimation for France in 2015. Int J Cancer 2019;144:2954-63. [Crossref] [PubMed]
- Rampinelli C, De Marco P, Origgi D, et al. Exposure to low dose computed tomography for lung cancer screening and risk of cancer: secondary analysis of trial data and risk-benefit analysis. BMJ 2017;356:j347. [Crossref] [PubMed]
- Wan Y, Shang J, Graham R, et al. Receptor recognition by novel coronavirus from Wuhan: An analysis based on decade-long structural studies of SARS. J Virol 2020;94:e00127-20. [Crossref] [PubMed]
- Zhao Y, Zhao Z, Wang Y, et al. Single-cell RNA expression profiling of ACE2, the putative receptor of Wuhan 2019-nCov. bioRxiv 2020. doi: [Crossref]
- Kermany DS, Goldbaum M, Cai W, et al. Identifying Medical Diagnoses and Treatable Diseases by Image-Based Deep Learning. Cell 2018;172:1122-31.e9. [Crossref] [PubMed]