Diabetes management before and after cancer diagnosis: missed opportunity
Introduction
Because of advances in early detection and treatment methods, the overall cancer death rate has steadily declined since the early 1990s and the 5-year survival rate is now 68%, up from 49% in the 1970s (1). In 2013, the cancer survivor population in the US was estimated to be 14 million, and a great number of them have comorbid conditions. Comorbid conditions such as diabetes are major threats to life for breast and colorectal cancer survivors (2,3). If diabetes is not well controlled, it can lead to stroke, heart disease, and disabilities, such as vision problems, kidney failure, or amputations of the foot or leg. A systematic review found that breast and colorectal cancer patients with diabetes also had worse clinical outcomes than those without diabetes (2). In addition, pre-existing diabetes is more prevalent in individuals with breast and colorectal cancer than other Americans (4,5).
There is well-founded concern that cancer-related services may compete with ongoing delivery of primary health care services, such as diabetes care, as patients with cancer often undergo treatment that includes multiple services over weeks or months delivered by medical specialties (6). This care pattern may disrupt usual care by overriding health concerns or from specialists “taking over” responsibility for a patient’s health care while cancer is being managed. However, it can also be postulated that since cancer patients have more interactions with the healthcare system after cancer diagnosis than pre-diagnosis, they would have additional opportunities to receive recommended diabetic management services (6-8). A recent study found that diabetic cancer patients received similar diabetic care relative to diabetic patients without cancer in a health maintenance organization (6). Another study found that survivors with diabetes enrolled in traditional fee-for-service Medicare were significantly less likely than diabetic patients without cancer to have regular diabetes examinations (7). These results potentially indicate that the health care delivery model may play a role in coordinating diabetes care.
This study examined the influence of cancer care delivery, such as characteristics of the hospital where the patient was treated, and the patient’s overall reliance on that hospital for their cancer on diabetes care among cancer patients with Medicare insurance. We expected that physicians at different hospitals would have different levels of motivation and capacity to recommend management services to cancer patients with diabetes, which could produce heterogeneity in diabetes care among survivors. In the present study, we compared the quality of diabetes management delivered to diabetic patients who had cancer surgery in small hospitals and patients treated in large cancer centers, and considered the effects of care fragmentation and distance on the outcome. The present study provides new insights by using a population-based sample of Medicare beneficiaries diagnosed with breast or colorectal cancer to examine the effect of several characteristics of the hospital where the patient was treated on measures of diabetes management quality outcomes before and after diagnosis.
Methods
Data and study cohort
This study is a part of a large project to study the pattern and quality of cancer care in breast and colorectal cancer patients living in Appalachian counties in North Carolina, Ohio, Kentucky, and Pennsylvania (9,10). This project obtained state central cancer registry data on breast and colorectal cancer incidence for 2006-2008. Patient data were linked to Medicare enrollment files using patients’ social security numbers, first and last names, gender, and dates of birth. These data are likely to have complete claims during 2005-2009 for patients not enrolled in a health maintenance organization. Data that were similarly linked have been used extensively in cancer care research (11-14).
Patients age 21 or older diagnosed with a first primary breast or colorectal cancer in 2006-2008 were identified in the linked data. The cancer registry data recorded the sequence number of reported tumors of each patient. We included those enrolled 1 year before and 1 year after diagnosis in both Medicare parts A (hospital insurance) and B (medical insurance). Cases with enrollment in Medicare Advantage or HMO involvement during the 2-year time frame were excluded. Cases with prior cancer diagnosis were excluded. Autopsy cases and cases where death was recorded within 1 year from diagnosis were excluded. Of these, 2,907 patients were diagnosed with diabetes 1 year before their cancer diagnosis and received surgery 6 months after cancer diagnosis. We then identified the facility where colorectal cancer surgery was conducted using the reporting hospital fields from the cancer registries data together with the “class of case” variable indicating if the reporting facility was the treatment facility. In addition we supplemented this identification by searching for treatment facility using Medicare claims files (Table S1). This resulted in 2,707 patients in the final sample (Figure 1).
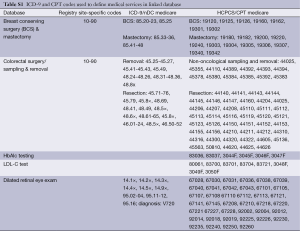
Full table
Diabetes management services
We assessed three measures of diabetes technical quality and clinical outcomes based on definitions proposed by the Diabetes Quality Improvement Program (DQIP) and 2004 Health Plan Employer Data and Information Set (HEDIS): glycated hemoglobin (HbAlc) testing every 6 months, serum cholesterol level (LDL-C) testing every year, and dilated retinal eye exam every year (15,16). For example, healthcare systems, such as Partners HealthCare, also use these three measures to develop their report cards (17). HbA1c is a lab test that shows the average level of blood sugar (glucose) and registry cancer diagnosis variables (Table S1).
Explanatory variables
The main explanatory variables of concordance concerned characteristics of the hospital where surgery was completed. Most patients completed their adjuvant therapy in the hospital or cancer center where surgery was completed or the outpatient clinics of these hospitals. We calculated volume of care variables based on all 2008 national Medicare claims associated with the hospitals in our database. All claims with procedure codes for breast and colorectal cancer surgical codes were singled out, and all non-duplicate unique claims for the hospital were summed. Surgery volume quartiles calculated over the sample were assigned to patients depending on the type of cancer (e.g., colorectal cancer resection volume quartiles for patients with colorectal cancer diagnosis). A volume of diabetes care variable was calculated using all available 2008 Medicare claims associated with facilities surrounding the study region, including claims not associated with patients within the sample. For each facility, all claims during 2008 associated with a diabetes ICD-9 diagnosis initiating with “250” were selected, duplicates were removed, and the number of unduplicated claims was then summed to arrive at a volume variable.
Number of beds, ownership information, and service mix (the number of high tech services such as acute renal dialysis, burn care unit, organ transplant) were obtained from the Provider of Service (POS) file available from the Centers for Medicare and Medicaid Services (CMS) particular to the year 2007. Commission on Cancer (CoC) accreditation status was obtained by consulting accreditation history (particular to 2007) from the web site facility locator (18). The ratio of total Medicaid inpatient days to total inpatient days was extracted from Healthcare Cost Report Information (HCRIS) data (19). We used the 2010 American Hospital Association survey data to extract information of the organizational structure of the hospital (centralized, centralized physician/insurance, decentralized, freestanding, independent, and moderately centralized). Patients’ residential distance from the surgical site was calculated as driving distance from the listed patient residential address to the surgical facility location. The addresses of both the patients and facilities were obtained from the Cancer Registry patient data and Medicare Provider of Service file. Driving distances between the addresses were geocoded and calculated with ArcGIS using the ESRI Street Map Pro network file.
Patient level variables were extracted from the cancer registry data and included age at diagnosis, gender, race/ethnic status, derived America Join Committee on Cancer (AJCC) stage grouping, tumor size, cancer site, surgery, and state of residence. A two-level diabetes severity score was calculated. Patients with low risk diabetes did not have complications or use insulin. Patients on insulin and patients with diabetes complications and diabetic ketoacidosis hospital episodes were classified as intermediate to high risk. A comorbidity score was calculated using the ACE-27 measure (excluding diabetes from the metric) as it captures more comorbidities than the Charlson comorbidity index (20). The four-level overall score ranges from 0 (no comorbidity burden) to 3 (severe comorbidity burden). In addition, patients were also classified by whether or not they received surgery at the facility where their cancer was diagnosed. (Patient’s pattern of use of facilities for cancer care were determined from NAACCR coding on ‘class of case’ reported for the surgical facility, and from CMS POS data where place of service was identified. Patients were initially defined as have ‘sole source’ cancer care if they were coded as class of case “1” (Diagnosis at the accessioning facility, and all or part of the first course of treatment was performed). CMS claims data were used to reclassify patients with class of case =1 as having fragmented care if they received chemotherapy services from a location other than the surgical facility site.) County level contextual variables where assigned to each patient based on residence at time of diagnosis. Data of contextual variables were drawn from the American Community Survey, Area Resource Files, and Appalachian Regional Commission (21-23). Important variables of socioeconomic status, such as family income and educational level, were not accessible due to data limitations.
Multivariate analysis
Association of post-diagnosis concordance measures with facility characteristics was examined using bivariate and multivariate analysis, in all cases adjusting for pre-diagnosis measures. For bivariate analysis, significance tests were conducted using logistic regression with post-diagnosis concordance as the dependent variable and examining the Wald test P values testing the bivariate association. For the multivariate analysis, all facility variables were included as independent variables in the model and the association with each variable was tested using Wald tests P values. In addition to previous concordance measures, selected demographics, tumor factors, and county level factors were included in the regression model using sequential forward regression and choosing the best fit regression model with the smallest Akaike Information Criteria.
Trend analysis
The follow-up period in the multivariate analyses was relatively short but used a relatively large sample size. In order to examine the pattern of diabetes management care over a relatively long time, we used only those patients who were continually enrolled in traditional Medicare for 3 years to conduct the trend analysis (N=1,665). A generalized estimating equations (GEE) main effect regression was conducted. Independent variables in the model included corresponding management care prior to diagnosis, time period (treated as a categorical variable), age groups, gender, race, comorbidity, diabetes severity, stage at diagnosis, tumor size, and type of surgery. Observations were clustered by patient and the unstructured covariance was chosen. Further, observations with missing data in the covariates were excluded. Expected probabilities of care were calculated by evaluating probabilities predicted by the model at the mean values for continuous variables and balanced rates for categorical variables.
Results
Table 1 shows that the median age of our sample was 74.4 years old. Our sample contained proportionately more female patients (76.5%). Both breast and colorectal cancer patients were predominately white (95.5%). Most patients (78.7%) were diagnosed with tumors smaller than 5 cm in size with early stage diseases (78.7%). Comorbidity burden among these patients was substantial: 90.5% of cancer patients had at least some level of burden, and nearly 19% had ‘severe’ comorbidity burden, which is associated with decreased survival after surgery. Almost 42% of these cancer patients had severe diabetes.
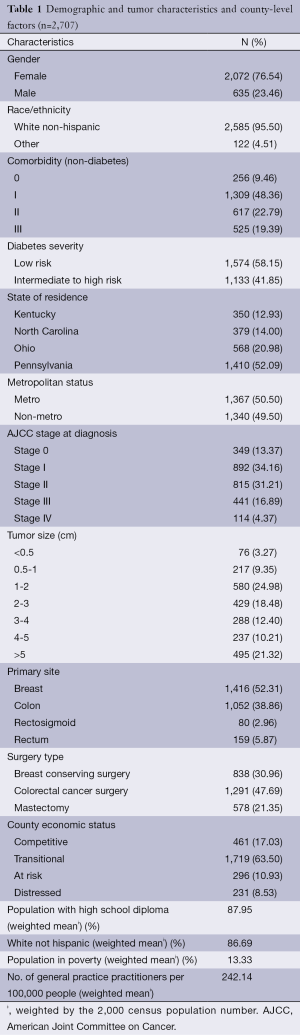
Full table
The overall rates of HbAlc testing, LDL-C testing, and retinal eye examinations before cancer diagnosis were 72.7%, 79.6%, and 57.9%, respectively (Table 2). The rates of HbAlc testing, LDL-C testing, and retinal eye examinations decreased after cancer diagnosis (58.3%, P<0.001; 69.5%, P<0.001; and 55.8%, P<0.05 respectively). In addition, there were no significant differences in guideline-concordant diabetes care before or after cancer diagnosis by characteristics of the hospital where the patient was treated such as CoC designation, number of beds, ownership, the ratio of Medicaid inpatient days, surgery volume, diabetes service volume, medical service mix, and organizational structure. We only found that the distance between the patient’s residence and hospital was negatively related to retinal eye testing (P value =0.0242).
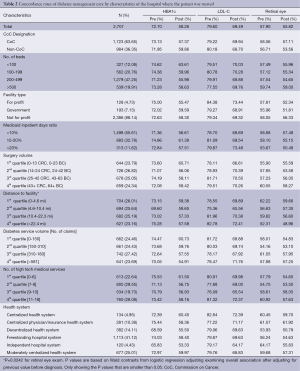
Full table
When patient and tumor characteristics were controlled, the strongest predictor of receiving diabetes care after cancer diagnosis was if the patient had received the same service before cancer diagnosis, which is consistent across three outcome measures (Table 3). For example, patients who received LDL-C testing before cancer diagnosis had five times the odds of having an LDL-C testing after cancer diagnosis when compared to those who didn’t receive an LDL-C testing before cancer diagnosis. There weren’t any significant differences in HbAlc testing, LDL-C testing, and retinal eye exam care before or after cancer diagnosis by hospital characteristics.
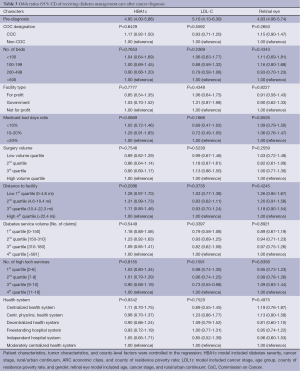
Full table
The trend analyses (Table 4) showed that the predicted probability of HbAlc testing, LDL-C testing, and retinal eye examinations decreased the first year after cancer diagnosis but then increased in the second year after diagnosis. For example, the predicted probability of HbA1c testing decreased from 0.73 before diagnosis to 0.57 at the first 6 months after diagnosis, and gradually increased in the next year and a half (0.64, 0.66, 0.67, P<0.0001). The other two measures followed a similar trend pattern as that for HbAlc testing, but did not reach statistical significance (P=0.0002 for LDL-C testing and P=0.0694 for retinal eye exam).
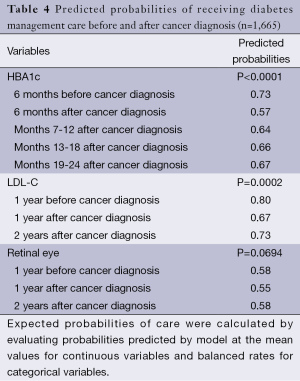
Full table
Discussion
This study provides population-based estimates of diabetes management care before and after cancer diagnosis. Although scientific attention to diabetes care among cancer patients is increasing (2,6-8), this is the first study to compare diabetes management care before and after cancer diagnosis and examine if diabetes management care among cancer patients was associated with the characteristics of the hospital where cancer surgery was performed. We found that cancer patients received fewer diabetes management services after diagnosis than prior to diagnosis. The predicted probability of diabetes management tests decreased at first after diagnosis and gradually increased thereafter. It does not appear that there is a particular advantage to being treated for cancer in a larger or CoC designated hospital or those with higher surgical volumes, at least in terms of concordance with diabetes guidelines. Although we were not able to measure care coordination, the results may suggest that the coordination of care for cancer patients with diabetes is neither better nor worse for cancer patients treated in the more comprehensive cancer treatment hospitals. The overall quality of diabetes care after cancer diagnosis was not associated with hospital ownership, volume, the number of beds, accreditation, safety-net burden, travel distance, diabetes service volume, mix of medical care service, or organizational structure. This may indicate that hospitals where cancer surgery was performed were equally poor in coordinating diabetes care with cancer treatment.
There may be several explanations for the decrease in diabetes management care quality after cancer diagnosis. Cancer patients and their physicians may devote more attention to interventions directly linked to cancer-specific mortality reduction as patients may think cancer is their greatest threat to life (8). So, primary care might be deterred by their intensive cancer treatment. From a provider perspective, cancer care physicians were not trained to coordinate care for non-cancer related health concerns, and the cancer care guidelines did not mention diabetes care (7,24,25). In addition, the Medicare program did not provide financial incentives to health professionals to coordinate non-cancer related healthcare. Although large comprehensive cancer centers were often affiliated with medical centers with enormous healthcare resources, they may fail to connect diabetic cancer patients to diabetes care. Additionally, very few comprehensive cancer centers in the US have a diabetes program to help cancer patients cope with their pre-existing diabetes (26).
The Patient Protection and Affordable Care Act (PPACA) provides a few opportunities for improving non-cancer related care coordination. The PPACA authorizes grants to support community-based collaborative care networks that must be consortiums of health care providers with a joint governance structure that provides comprehensive coordinated and integrated health care services. The Department of Health and Human Services will provide grants to create community health teams and medical homes that include an interdisciplinary team of health care providers which would provide integrated community-based care for their patients. These policy changes may help small cancer hospitals coordinate with primary care physicians in providing diabetes management care to cancer patients. It does less to ensure the coordination of care if a patient receives cancer treatment in a comprehensive cancer center.
Our study has both strengths and weaknesses. The first strength is that we were able to describe diabetes care before and after cancer diagnosis in the same cohort of patients. Second, the quality of diabetes care before cancer diagnosis was very similar among different patient groups by hospital characteristics in this study. So, patients were probably not selected on the basis of their diabetes care before diagnosis. There are also several limitations. We can only apply these results to elderly cancer patients in a fee-for-service environment. Although we focused on elderly cancer patients from four states by design, some characteristics of healthcare in these four states are shared by other regions of the US. Thus, the importance of our findings certainly reaches beyond these four states. The follow-up time is short in this study, so we were not able to examine long-term diabetes management care quality among cancer survivors. We plan to request additional data to examine if the diabetes care quality improves in the second or third year after cancer diagnosis. Some cancer patients may have received adjuvant therapies outside the hospital where surgery was completed. Our model did not capture the influence of outpatient clinics which are not affiliated with the hospital where surgery was conducted. Our analysis relies on the accuracy of claims data and the cancer registry files. There can be mistakes in the billing data and registry files, but Medicare data are a reliable and efficient source of data to study medical care among elderly cancer patients (9,27), and cancer registries are among the best source of cancer case identification for population research (12,28,29).
In conclusion, diabetes management care for cancer patients declined after diagnosis, regardless of where they received their cancer surgery. This reflects a missed opportunity to connect diabetic cancer patients to diabetes care, particularly among patients treated in large comprehensive cancer centers. This study provides benchmarks against which to measure improvements in comorbidity management among cancer patients.
Acknowledgements
The study was approved by the Institutional Review boards of Pennsylvania State University.
Funding: This research was supported by Grant Number 1R01CA140335 from the National Cancer Institute. Dr. Yao was also supported under a fellowship from the National Cancer Institute (2R25CA093423).
Disclosure: The authors declare no conflict of interest.
References
- American Cancer Society. Cancer Facts & Figures, 2013. Accessed on Oct 16th, 2013. Available online: http://www.cancer.org/research/cancerfactsfigures/cancerfactsfigures/cancer-facts-figures-2013
- Barone BB, Yeh HC, Snyder CF, et al. Long-term all-cause mortality in cancer patients with preexisting diabetes mellitus: a systematic review and meta-analysis. JAMA 2008;300:2754-64. [PubMed]
- Coughlin SS, Calle EE, Teras LR, et al. Diabetes mellitus as a predictor of cancer mortality in a large cohort of US adults. Am J Epidemiol 2004;159:1160-7. [PubMed]
- Larsson SC, Mantzoros CS, Wolk A. Diabetes mellitus and risk of breast cancer: a meta-analysis. Int J Cancer 2007;121:856-62. [PubMed]
- Larsson SC, Orsini N, Wolk A. Diabetes mellitus and risk of colorectal cancer: a meta-analysis. J Natl Cancer Inst 2005;97:1679-87. [PubMed]
- Keating NL, Zaslavsky AM, Herrinton LJ, et al. Quality of diabetes care among cancer survivors with diabetes. Med Care 2007;45:869-75. [PubMed]
- Earle CC, Neville BA. Under use of necessary care among cancer survivors. Cancer 2004;101:1712-9. [PubMed]
- Earle CC, Burstein HJ, Winer EP, et al. Quality of non-breast cancer health maintenance among elderly breast cancer survivors. J Clin Oncol 2003;21:1447-51. [PubMed]
- Anderson RT, Yang TC, Matthews SA, et al. Breast cancer screening, area deprivation, and later-stage breast cancer in Appalachia: does geography matter? Health Serv Res 2014;49:546-67. [PubMed]
- Fleming ST, Mackley HB, Camacho F, et al. Clinical, sociodemographic, and service provider determinants of guideline concordant colorectal cancer care for Appalachian residents. J Rural Health 2014;30:27-39. [PubMed]
- Gross CP, Smith BD, Wolf E, et al. Racial disparities in cancer therapy: did the gap narrow between 1992 and 2002? Cancer 2008;112:900-8. [PubMed]
- Warren JL, Klabunde CN, Schrag D, et al. Overview of the SEER-Medicare data: content, research applications, and generalizability to the United States elderly population. Med Care 2002;40:IV-3-18. [PubMed]
- Gold HT, Dick AW. Variations in treatment for ductal carcinoma in situ in elderly women. Med Care 2004;42:267-75. [PubMed]
- Ballard-Barbash R, Potosky AL, Harlan LC, et al. Factors associated with surgical and radiation therapy for early stage breast cancer in older women. J Natl Cancer Inst 1996;88:716-26. [PubMed]
- McLaughlin S. The diabetes quality improvement project. Diabetes Spectrum 2000;13:5-10.
- National Committee for Quality Assurance. HEDIS 2004 Technical Specifications, 2004. Accessed on Dec 9th, 2013. Available online: http://www.ncqa.org/Portals/0/HEDISQM/HEDIS2008/2008_Measures.pdf
- Partners HealthCare System. Prevention and Chronic Condition Management, 2008. Accessed on Feb 5th, 2014. Available online: http://qualityandsafety.partners.org/measures/overview.aspx?id=16
- American College of Surgeons. Cancer Program Accreditation, 2014. Accessed on Jan 14th, 2014. Available omline: http://www.facs.org/cancerprogram/index.html
- Research Data Assistance Center. Healthcare Cost Report Information System | ResDAC, 2014. Accessed on Jan 14th, 2014. Available online: http://www.resdac.org/cms-data/files/hcris
- Fleming ST, Sabatino SA, Kimmick G, et al. Developing a claim-based version of the ACE-27 comorbidity index: a comparison with medical record review. Med Care 2011;49:752-60. [PubMed]
- Appalachian Regional Commission. Appalachian Maps by Topic: Economic Status, 2014. Accessed on Jan 14th, 2014. Available online: http://www.arc.gov/research/MapsofAppalachia.asp?F_CATEGORY_ID=1
- USDA. USDA ERS - Rural-Urban Continuum Codes, 2014. Accessed on Jan 14th, 2014. Available online: http://www.ers.usda.gov/data-products/rural-urban-continuum-codes/.aspx#.UtVWA7TWv40
- HRSA/Bureau of Health Professions. AHRF: Area Health Resource File, 2013. Accessed on Dec 4th, 2013. Available online: http://arf.hrsa.gov/overview.htm
- American Society of Clinical Oncology. Breast Cancer Guidelines, 2014. Accessed on Feb 6th, 2014. Available online: http://www.asco.org/guidelines/breast-cancer
- National Cancer Institute. Breast Cancer Treatment (PDQ®), 2014. Accessed on Feb 6th, 2014. Available online: http://www.cancer.gov/cancertopics/pdq/treatment/breast/healthprofessional
- MD Anderson Cancer Center. Diabetes Management, 2014. Accessed on 2014 Feb 6th, 2014. Available online: http://www.mdanderson.org/patient-and-cancer-information/cancer-information/cancer-topics/dealing-with-cancer-treatment/diabetes/index.html
- Curtis E, Quale C, Haggstrom D, et al. Racial and ethnic differences in breast cancer survival: how much is explained by screening, tumor severity, biology, treatment, comorbidities, and demographics? Cancer 2008;112:171-80. [PubMed]
- Cress RD, Zaslavsky AM, West DW, et al. Completeness of information on adjuvant therapies for colorectal cancer in population-based cancer registries. Med Care 2003;41:1006-12. [PubMed]
- Warren JL, Harlan LC. Can cancer registry data be used to study cancer treatment? Med Care 2003;41:1003-5. [PubMed]