Development and validation of an autophagy-related prognostic signature in esophageal cancer
Introduction
Esophageal cancer (ESCA) ranks as the sixth most common cause of cancer deaths globally (1). Despite the improved diagnostic and treatment procedures, including endoscopic resection, targeted therapy, and immunotherapy, the overall survival (OS) of ESCA patients is still poor. The 5-year survival rate for patients with advanced ESCA is less than 20% (2). Improving early diagnosis and identifying new prognostic markers and therapeutic targets are important in improving the prognosis of patients with ESCA.
Autophagy, known as type II programmed cell death, plays an important role in maintaining cell homeostasis. Abnormal autophagic levels are involved in the carcinogenesis of various organs, including ESCA (3,4). Previous studies indicated that autophagy played a dual role in cancer development, depending on stage and type of cancers (5). Studying the relationship between autophagy and tumorigenesis may facilitate the discovery of new targets for prognosis and treatment.
Various prognostic models based on gene expression data, such as long non-coding RNA (lncRNA) and microRNA (miRNA) expression, have been developed for different cancers (6,7); however, prognostic models based on autophagy-related genes (ARGs) in ESCA have not been reported. In the present study, we examined the associations between expression profiles of ARGs and clinical outcomes in ESCA patients using the database of The Cancer Genome Atlas (TCGA). We developed an ARG prognostic signature that was proved to be an independent predictor of OS in ESCA patients. Our findings provide an effective multi-dimensional biomarker strategy that predicts the prognosis of ESCA patients. We present the following article in accordance with the TRIPOD reporting checklist (available at http://dx.doi.org/10.21037/atm-20-4541).
Methods
The study conformed to the provisions of the Declaration of Helsinki (as revised in 2013).
Data extraction from the TCGA database
The transcriptome data and clinical information for ESCA patients were obtained from TCGA (https://portal.gdc.cancer.gov/). RNA-sequencing (RNA-Seq) was performed using HTSeq-FPKM (version: July 19, 2019), which is a simple expression level normalization method. A total of 158 primary ESCA patients with gene expression data and clinical follow-up information were included in the current study. The ARGs were acquired from The Human Autophagy Database (HADb, http://www.autophagy.lu/index.html).
Differentially expressed ARGs and functional pathways analysis
The “Limma” package in R software was employed to identify the differentially expressed ARGs between tumor samples and non-tumor samples from patients with ESCA. The cut-offs were |logFold change (FC)| >0.5 and P value <0.05. The “org.Hs.eg.db” package was utilized to extract the Entrez ID of each ARG. The “clusterProfiler”, “enrichplot” and “ggplot2” packages were applied for Gene Ontology (GO) and Kyoto Encyclopedia of Genes and Genomes (KEEG) analyses as well as for visualization of the enrichment terms.
Construction and validation of an ARG-related prognostic signature
The ESCA patients in the entire TCGA dataset were randomly separated into a training dataset and a testing dataset by the “caret” package of R. The clinical information related to the TCGA-ESCA cohort was downloaded, and the OS was defined as the time from diagnosis to death or the last follow-up date. ARGs that were significantly associated with OS were selected using a univariate Cox regression analysis. To identify ARGs that independently predicted OS in the training dataset, a multivariate Cox regression analysis was performed on the ARGs selected in the univariate regression analysis. A prognostic signature was developed based on these ARGs. A risk score was established based on the prognostic ARGs signature using a linear combination of the gene expression levels weighted by the regression coefficients derived from the Cox regression analysis. This model was utilized to estimate the survival of each patient in the training dataset and testing dataset. The patients in each dataset were assigned to a high-risk or a low-risk group using the median risk score as a cutoff. The predictive potential of the ARGs signature was evaluated via area under the curve (AUC) value of the receiver operating characteristic (ROC) curve at 1-year using the “survivalROC” package.
PPI network construction and module screening
The differentially expressed ARGs were submitted to the STRING database (Search Tool for the Retrieval of Interacting Genes/Proteins, http://www.string-db.org/, version 11.0) to identify protein-protein interaction (PPI) information. The Cytoscape 3.7.2 software was applied to further construct and visualize the PPI network. Important modules and genes were selected in the PPI network by applying the Molecular Complex Detection (MCODE) plug-in with node counts higher than 10.
Statistical analysis
A Cox regression analysis was conducted using the “survival” package. The “Limma” package was utilized to conduct the normalization and differential expression analyses. Wilcoxon rank-sum test, a non-parametric statistical hypothesis test, was used for comparing two groups. All statistical analyses were implemented using the R software (version 3.6.3). A P value <0.05 was considered to be significant.
Results
Differentially expressed ARGs in ESCA
The ESCA RNA-seq data download from the TCGA database included 159 tumor samples and 10 non-tumor samples from the patients. Among these patients, a total of 158 primary ESCA patients with gene expression data and clinical follow-up information were included in the current study. We analyzed the expression of 232 ARGs in ESCA tumor samples and non-tumor tissues using the Wilcoxon signed rank test in R. Using the criteria of |log2 FC| >0.5 and P<0.05, 69 ARGs were extracted, including 9 down-regulated genes and 60 up-regulated genes. The expression patterns of these differentially expressed ARGs were visualized using the “limma” package of R (Figure 1A,B). A box plot was generated to display the expression patterns of the 69 ARGs in ESCA and non-tumor tissues (Figure 2).
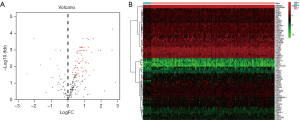
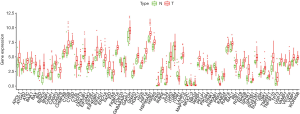
Functional enrichment analysis of the differentially expressed ARGs
The GO annotations for the differentially expressed ARGs were determined. The most enriched GO terms for biological processes were autophagy, process utilizing autophagic mechanism, and neuron death. The cellular component analysis showed enrichment of vacuolar membrane, endosome membrane, and autophagosome in the differentially expressed ARGs. In the molecular function analysis, ubiquitin-like protein ligase binding, ubiquitin protein ligase binding, and cytokine receptor binding (Figure 3A,B) were enriched in the differentially expressed ARGs. The results of the KEGG pathway analysis showed enrichment of the apoptosis, autophagy-animal, and Kaposi sarcoma-associated herpes virus infection pathways (Figure 3C,D). More importantly, the functional analysis showed that the enriched genes were up-regulated in the most significant KEGG pathways (e.g., apoptosis and autophagy-animal).
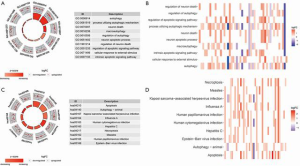
PPIs among differentially expressed ARGs
To understand the interaction among the differentially expressed ARGs, a PPI network was constructed using STRING (Figure 4A). The PPI network was composed of three modules, including 670 nodes and 335 edges. For the ease of description, we named these modules as CASP3, VEGFA, and SQSTM1 modules. In the CASP3 module (Figure 4B), 26 edges involving 11 nodes were formed, of which the most remarkable nodes were CASP3, GAPDH, CASP8, EIF4G1, HSPA5, and DDIT3. In the VEGFA module (Figure 4C), apoptosis and necroptosis-related genes were assigned to the center of the module, including CASP1, FAS, FADD, and TNFSF10. The SQSTM1 module had the most nodes associated with autophagy and ubiquitin-like proteins (Figure 4D).
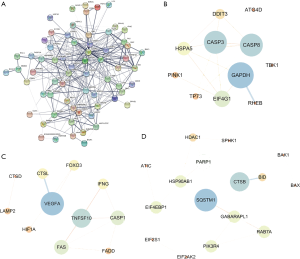
Construction of ARG prognostic signature and prediction in the training dataset
The entire group (N=158) with complete survival information and RNA-seq expression profiles was randomly separated into the training dataset (N=80, Table S1) and testing dataset (N=78, Table S2). The summary information of ESCA patients is shown in Table 1. The detailed characteristics and population demographics of the tissue samples is shown in Table S3. Seven ARGs, including DDIT3 (DNA damage-inducible transcript 3), HSP90AB1 (heat shock protein 90 alpha family class B member 1), FKBP1A (FKBP prolyl isomerase 1A), DNAJB1 (DnaJ heat shock protein family (Hsp40) member B1), BNIP1 (BCL2 interacting protein 1), VAMP7 (vesicle-associated membrane protein 7) and TBK1 (TANK binding kinase 1) were significantly associated with OS (P<0.05). These genes were selected as prognostic candidate genes using a univariate Cox regression analysis. Based on the multivariate Cox regression analysis, four genes including DNAJB1, BNIP1, VAMP7 and TBK1, were selected to construct the OS prediction model. Finally, a prognostic risk score based on the prognostic signature was established as follows: (0.61184 × expression value of DNAJB1) + (1.59675 × expression value of BNIP1) + (0.90984 × expression value of VAMP7) + (0.96329 × expression value of TBK1). Here, 0.61184, 1.59675, 0.90984 and 0.96329 are regression coefficients for the respective genes derived from the Cox regression analysis. Using the median value of the risk score as the cutoff, we divided the ESCA cases into high- and low-risk groups. Kaplan-Meier curves indicate that the high-risk group had a lower survival probability, compared to the low-risk group in the training dataset [hazards ratio (HR) =1.508, 95% confidence interval (CI): 1.201–1.894, P=1.346e−05; Figure 5A]. The ROC curve for the ARGs signature model is shown in Figure 5B, and the AUC for 1-year survival was 0.746. Figure 5C,D,E show the distribution of patient risk scores, survival time, and the expression of risk genes in the training dataset.
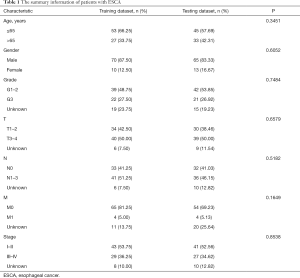
Full table
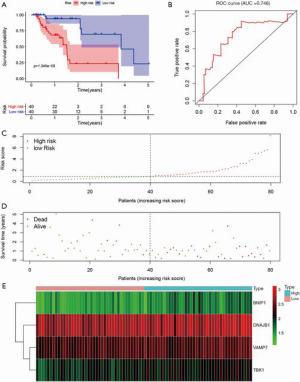
Construction of a nomogram based on the four ARGs
To develop a quantitative method for the prediction of ESCA prognosis, we used the prognostic signature derived from the four ARGs to construct a nomogram. Based on the multivariate Cox analysis results, we drew a horizontal line to determine the points for each autophagy prognosis gene and calculated the total score of each patient by adding the points for all variables. The points were normalized to a 0 to 100 distribution. By calculating the total score, we could estimate the 1-, 2-, and 3-year survival of each ESCA patient (Figure 6A). Additionally, we evaluated the relationship between different clinical characteristics and the prognosis of ESCA by performing a Cox regression analysis. We found that tumor stage, distant metastasis, lymph node involvement, and risk score were associated with OS of ESCA patients (P<0.01; Figure 6B). Importantly, as shown in Figure 6C, risk score remained as an independent risk predictor.
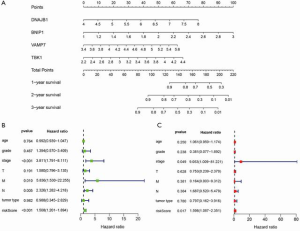
Validation of the ARG prognostic signature
To examine the robustness of the four-ARGs signature, the prognostic value of the ARGs signature was validated in the testing dataset. Based on the expression value of the four prognostic ARGs, the prognostic risk score was calculated for patients in the testing dataset. Each patient was marked as a high- or low-risk based on the median risk score obtained from the training dataset. The Kaplan-Meier survival curves for the high- and low-risk groups in the testing dataset were significantly different (P=6.876e−03; Figure 7A). The AUC for the ROC curve of the OS-related predictive signatures in the testing dataset was 0.691 (Figure 7B). The distribution of risk scores, survival status, and expression for the four prognostic ARGs in the testing dataset are shown in Figure 7C,D,E, respectively.
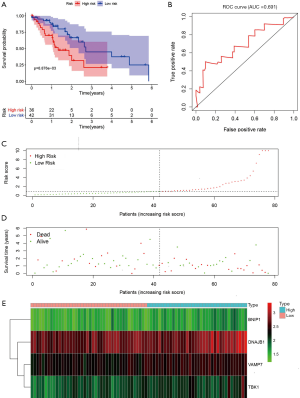
Independence of the prognostic value of the ARGs signature derived from other clinical variables
We evaluated the independence of the prognostic value of the ARGs signature based on other clinical variables. Univariate and multivariate Cox regression analyses were performed using age, grade, stage (T, N, M), tumor type (adenocarcinoma, squamous cell carcinoma), and the prognostic risk score model as covariables. Multivariate Cox analysis revealed that the four-ARG signature was significantly associated with OS in each dataset (training: Figure 6B,C; testing: Figure 8A,B). Thus, the ARGs signature is a potential prognostic marker for ESCA. The AUCs of the ROC curves were 0.709 (risk score), 0.594 (age), 0.583 (grade), 0.655 (stage), 0.611 (T), 0.676 (lymph node status), and 0.509 (distant metastasis) (Figure 9).
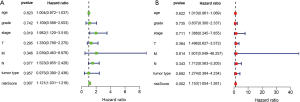
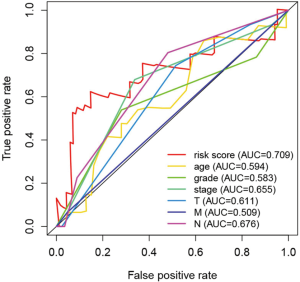
Discussion
ESCA is a fatal malignant tumor, causing approximately 500,000 deaths worldwide in 2018 (8), and the incidence is still growing. Unfortunately, there are no effective molecular biomarkers for monitoring ESCA prognosis. In recent years, the focus of ESCA research has turned to tumor autophagy (9). However, most of the current research on autophagy in ESCA only focuses on a single gene involved in autophagy (10,11). A comprehensive analysis of ARGs has not been conducted to explore the clinical significance of autophagy in ESCA. In the present study, we analyzed the RNA-seq expression profiles and corresponding clinical information of ESCA patients downloaded from TCGA. To obtain the genes essential for ESCA from the perspective of autophagy, we first screened 69 ARGs that were differentially expressed between ESCA tumor and non-tumor tissues. A functional analysis showed that the genes enriched in the most significant KEGG pathway (e.g., apoptosis and autophagy-animal) were upregulated. Based on these results, we speculated that autophagy is activated during the initiation of ESCA. In addition, analysis of the PPI network for ESCA indicated that the main nodules were related to autophagy, apoptosis, and ubiquitin-like protein process. Subsequently, patients were randomly divided into a training dataset and a testing dataset. Univariate Cox regression and multivariate Cox regression analyses were performed in the training dataset. An autophagy-related prognostic signature was constructed based on the four key ARGs. This prognostic signature was then validated in the testing dataset. Kaplan-Meier curves showed the high-risk group had worse OS compared to the low-risk group in the two datasets. Univariate Cox regression and multivariate Cox regression analyses suggested that the four-ARG signature was as an independent risk predictor for patient survival, independent of other clinical factors.
These four ARGs play roles in the progression of various tumors. DNAJB1 is associated with a variety of cellular processes, including the proteasome pathway (12), endoplasmic reticulum stress (13), and viral infection (14). Lately, more attention has been paid to the functions of DNAJB1 in the progression of cancer. DNAJB1 inhibits mitogen-inducible gene 6 (MIG6) stabilization and increases lung cancer cell proliferation (15). On the other hand, DNAJB1 acts as an autophagy-related protein involved in the development of tumors. DNAJB1 also targets programmed cell death 5 (PDCD5) to suppress p53-mediated apoptosis and enhance the proliferation of cancer cells (16). The up-regulation of DNAJB1 correlates with poor prognosis for cholangiocarcinoma patients (17). Bnip1, a member of the BH3-only protein family, influences apoptosis, proliferation, invasion, and migration in cervical cancer cells through the regulation of mammalian target of rapamycin (mTOR) expression (18). Although TBK1 was thought to only play a role in the immune response (19), a recent study showed that TKB1 promotes the oncogenic phenotype in cancer and regulates autophagy (20). As for VAMP7, it was shown to be involved in multiple vesicular transport events, including autophagy (21). However, little is known about the functional mechanism of VAMP7 in ESCA.
Some prognostic signatures have been derived from ARGs in several tumor types, including glioblastoma (22), hepatocellular carcinoma (23), and lung cancer (24). Our results indicated that an autophagy-related prognostic signature based on the four ARGs can be employed for the prognostic prediction of survival in ESCA patients. This prognostic tool may be the basis for establishing personalized treatment procedures, based on patient risk, for improved therapy of ESCA. We explored and validated the prognostic value of ARGs in ESCA. However, our research has several limitations. (I) Due to the relatively small number of ESCA tumor and non-tumor samples in the TCGA database, the present study inevitably has the problem of period. (II) Although we have verified the model in the testing dataset, further investigations in vitro, in vivo, and in independent ESCA cohorts are needed to confirm the accuracy of the model. (III) Furthermore, other potential prognostic variables associated with OS in ESCA, such as lymphovascular invasion (LVI) and neutrophil-to-lymphocyte ratio (NLR) need to be explored. (IV) Finally, the functional mechanisms of the four ARGs should be further investigated in ESCA.
Conclusions
In summary, we constructed and verified a novel ARG prognostic signature that could improve the individualized outcome prediction for patients with ESCA. For those ESCA patients with high risk scores, a more comprehensive neoadjuvant/adjuvant therapy or closer follow-up are indicated for an improved prognosis.
Acknowledgments
The results of this study are based on the data from TCGA (https://www.cancer.gov/tcga). We thank all the authors who provided the data for this study. Meanwhile, we would aslo like to thank Prof Qingyi Wei from Duke Cancer Institute for his scientific editing.
Funding: This study was supported by Shanghai Municipal Commission of Health and Family Planning Outstanding Academic Leaders Training Program (2017BR055).
Footnote
Reporting Checklist: The authors have completed the TRIPOD reporting checklist. Available at http://dx.doi.org/10.21037/atm-20-4541
Peer Review File: Available at http://dx.doi.org/10.21037/atm-20-4541
Conflicts of Interest: All authors have completed the ICMJE uniform disclosure form (available at http://dx.doi.org/10.21037/atm-20-4541). The authors have no conflicts of interest to declare.
Ethical Statement: The authors are accountable for all aspects of the work in ensuring that questions related to the accuracy or integrity of any part of the work are appropriately investigated and resolved. The study conformed to the provisions of the Declaration of Helsinki (as revised in 2013).
Open Access Statement: This is an Open Access article distributed in accordance with the Creative Commons Attribution-NonCommercial-NoDerivs 4.0 International License (CC BY-NC-ND 4.0), which permits the non-commercial replication and distribution of the article with the strict proviso that no changes or edits are made and the original work is properly cited (including links to both the formal publication through the relevant DOI and the license). See: https://creativecommons.org/licenses/by-nc-nd/4.0/.
References
- Domper Arnal MJ, Ferrández Arenas Á, Lanas Arbeloa Á. Esophageal cancer: Risk factors, screening and endoscopic treatment in Western and Eastern countries. World J Gastroenterol 2015;21:7933-43. [Crossref] [PubMed]
- Torre LA, Siegel RL, Ward EM, et al. Global Cancer Incidence and Mortality Rates and Trends--An Update. Cancer Epidemiol Biomarkers Prev 2016;25:16-27. [Crossref] [PubMed]
- Poillet-Perez L, White E. Role of tumor and host autophagy in cancer metabolism. Genes Dev 2019;33:610-9. [Crossref] [PubMed]
- Saxena R, Klochkova A, Murray MG, et al. Roles for Autophagy in Esophageal Carcinogenesis: Implications for Improving Patient Outcomes. Cancers (Basel) 2019;11:1697. [Crossref] [PubMed]
- Yazdani HO, Huang H, Tsung A. Autophagy: Dual Response in the Development of Hepatocellular Carcinoma. Cells 2019;8:91. [Crossref] [PubMed]
- Gu JX, Zhang X, Miao RC, et al. Six-long non-coding RNA signature predicts recurrence-free survival in hepatocellular carcinoma. World J Gastroenterol 2019;25:220-32. [Crossref] [PubMed]
- Siriwardhana C, Khadka VS, Chen JJ, et al. Development of a miRNA-seq based prognostic signature in lung adenocarcinoma. BMC Cancer 2019;19:34. [Crossref] [PubMed]
- Bray F, Ferlay J, Soerjomataram I, et al. Global cancer statistics 2018: GLOBOCAN estimates of incidence and mortality worldwide for 36 cancers in 185 countries. CA Cancer J Clin 2018;68:394-424. [Crossref] [PubMed]
- Liu H, Zhao J, Fu R, et al. The ginsenoside Rk3 exerts anti-esophageal cancer activity in vitro and in vivo by mediating apoptosis and autophagy through regulation of the PI3K/Akt/mTOR pathway. PLoS One 2019;14:e0216759. [Crossref] [PubMed]
- Ma Z, Chen C, Tang P, et al. BNIP3 induces apoptosis and protective autophagy under hypoxia in esophageal squamous cell carcinoma cell lines: BNIP3 regulates cell death. Dis Esophagus 2017;30:1-8. [Crossref] [PubMed]
- Wu J, Zhang D, Li J, et al. MACC1 induces autophagy to regulate proliferation, apoptosis, migration and invasion of squamous cell carcinoma. Oncol Rep 2017;38:2369-77. [Crossref] [PubMed]
- Yamazaki S, Uchiumi A, Katagata Y. Hsp40 regulates the amount of keratin proteins via ubiquitin-proteasome pathway in cultured human cells. Int J Mol Med 2012;29:165-8. [PubMed]
- Lenna S, Farina AG, Martyanov V, et al. Increased expression of endoplasmic reticulum stress and unfolded protein response genes in peripheral blood mononuclear cells from patients with limited cutaneous systemic sclerosis and pulmonary arterial hypertension. Arthritis Rheum 2013;65:1357-66. [Crossref] [PubMed]
- Batra J, Tripathi S, Kumar A, et al. Human Heat shock protein 40 (Hsp40/DnaJB1) promotes influenza A virus replication by assisting nuclear import of viral ribonucleoproteins. Sci Rep 2016;6:19063. [Crossref] [PubMed]
- Park SY, Choi HK, Seo JS, et al. DNAJB1 negatively regulates MIG6 to promote epidermal growth factor receptor signaling. Biochim Biophys Acta 2015;1853:2722-30. [Crossref] [PubMed]
- Cui X, Choi HK, Choi YS, et al. DNAJB1 destabilizes PDCD5 to suppress p53-mediated apoptosis. Cancer Lett 2015;357:307-15. [Crossref] [PubMed]
- Ren H, Luo M, Chen J, et al. Identification of TPD52 and DNAJB1 as two novel bile biomarkers for cholangiocarcinoma by iTRAQ-based quantitative proteomics analysis. Oncol Rep 2019;42:2622-34. [Crossref] [PubMed]
- Li FH, Xiang L, Ran L, et al. BNIP1 inhibits cell proliferation, migration and invasion, and promotes apoptosis by mTOR in cervical cancer cells. Eur Rev Med Pharmacol Sci 2019;23:1397-407. [PubMed]
- Xiao Y, Zou Q, Xie X, et al. The kinase TBK1 functions in dendritic cells to regulate T cell homeostasis, autoimmunity, and antitumor immunity. J Exp Med 2017;214:1493-507. [Crossref] [PubMed]
- Durand JK, Zhang Q, Baldwin AS. Roles for the IKK-Related Kinases TBK1 and IKKε in Cancer. Cells 2018;7:139. [Crossref] [PubMed]
- Fader CM, Aguilera MO, Colombo MI. ATP is released from autophagic vesicles to the extracellular space in a VAMP7-dependent manner. Autophagy 2012;8:1741-56. [Crossref] [PubMed]
- Wang QW, Liu HJ, Zhao Z, et al. Prognostic Correlation of Autophagy-Related Gene Expression-Based Risk Signature in Patients with Glioblastoma. Onco Targets Ther 2020;13:95-107. [Crossref] [PubMed]
- Mao D, Zhang Z, Zhao X, et al. Autophagy-related genes prognosis signature as potential predictive markers for immunotherapy in hepatocellular carcinoma. PeerJ 2020;8:e8383. [Crossref] [PubMed]
- Liu Y, Wu L, Ao H, et al. Prognostic implications of autophagy-associated gene signatures in non-small cell lung cancer. Aging(Albany NY) 2019;11:11440-62. [Crossref] [PubMed]