Standardization of pleural effusion-based tumor mutation burden (TMB) estimation using capture-based targeted sequencing
Introduction
The development of immune checkpoint inhibitors (ICIs), which target inhibitory receptors on T cells, has begun to revolutionize the treatment landscape for numerous cancers. Monoclonal antibodies that reverse tumor-induced immunosuppression by targeting immune checkpoint proteins CTLA-1 or PD-1 (or its ligand PD-L1) have been approved as first- or second-line therapy for a growing list of malignancies (1). However, only a fraction of patients benefited from ICI treatment, even in generally-responsive tumor entities. Many patients develop resistance shortly after the initiation of the treatment or even at baseline (2). Therefore, there is a clear need to identify predictive markers for response to ICI treatment.
Multiple biomarkers have been explored, including, but not limited to, microsatellite instability (MSI) status, PD-L1 expression, tumor mutation burden (TMB), and mutation status of certain genes such as STK11 and TP53. Although PD-L1 expression, TMB, and MSI status have been approved as predictor biomarkers in certain cancer types for certain ICIs, controversy remains around their value in non-small cell lung cancer (NSCLC). MSI-H is a rare event in NSCLC, whereby less than around 1% NSCLC harbor MSI-H. Many patients with microsatellite-stable NSCLC show enhanced TMB and subsequently respond favorably to immunotherapy. PD-L1 expression level determined by immunohistochemistry (IHC) has been shown to identify patients with a higher likelihood of response to ICI in NSCLC, melanoma, and bladder cancer. Unfortunately PD-L1 expression yields limited sensitivity and specificity in NSCLC potentially for the following reasons: (I) PD-L1 expression can be transient, therefore, intra-patient and intra-tumor heterogeneity in PD-L1 expression is possible; and (II) there is a lack of consistency in the thresholds used for defining PD-L1 positivity. Another promising pan-cancer measure of genomic instability is TMB, which is purported to represent the likelihood of neoantigen production. High TMB, representing genomic instability, has the potential to induce neoantigen production and subsequent enhancement in immunogenicity (3,4). Numerous studies have demonstrated a positive correlation between high TMB and favorable response to ICIs in a number of cancers, including, but not limited to, melanoma, lung cancer, and urothelial carcinoma (5-8).
To date there has not been a universal agreement on certain key aspects of TMB evaluation, a process susceptible to variations in both wet lab processing and bioinformatic analysis. There is diversity as to how TMB is used in guiding immunotherapy decisions. For instance, studies differ in thresholds for distinguishing “TMB-high” from “TMB-low” tumors, algorithms for TMB computation, and scales for conversion between TMB estimates derived from WES and from targeted panels. An ideal solution is a large-scale clinical trial comparing TMB levels determined by all mainstream approaches of the same specimens and examining the value of the standardized TMB estimates in ICI efficacy prediction. A standard protocol for TMB evaluation is key in the clinical utility of immunotherapy, as trials based on which can be developed to resolve current inconsistencies regarding the relationship between TMB, efficacy of immune checkpoint inhibitor, and other markers such as PD-L1 expression. Despite lack of comprehensive consensus, it is often derived from the number of non-synonymous somatic mutations. Whole-exome sequencing (WES) is considered the gold standard for determining TMB; however, implementation of WES is limited by a number of factors, including the need for large sample quantities, high costs, and extensive data management. On the other hand, capture-based sequencing has established a firm footing in oncology practice in a host of scenarios, including detecting the full spectrum of actionable targets, determining MSI status, and identifying molecular biomarkers of prognosis and/or treatment response. Importantly, panel-based sequencing has also been actively explored as a more practical alternative in TMB estimation. Using WES-derived TMB as reference, a number of studies have confirmed the feasibility of estimating TMB using a defined gene panel coupled with targeted sequencing (9). Although initial studies of TMB primarily focused on relative levels, absolute definitions are being pursued by several commercial providers with the goal of selecting patients who are more likely to benefit from ICI treatment. Clearly, accuracy in both TMB measurement and establishment of cutoff values for patient classification—such as 10 mutations/Mb, proposed by Foundation Medicine—bears weight in informing therapeutic decisions. Given the diversity of commercially-available panels, it is necessary to conduct a comparison of their performance in TMB estimation.
TMB assessment has been primarily performed on tissue samples. The challenges and pitfalls associated with tissue biopsy have been well documented, primarily pertaining to its static nature and invasiveness. Less invasive alternatives, such as blood cell-free DNA (cfDNA), have been investigated, although the predictive value of blood TMB is even more controversial than that of tissue TMB (10,11). An alternative liquid biopsy is to use malignant pleural effusion (MPE), a complication that results from tumor invasion of the pleural space causing disrupted drainage of the pleural fluid (12,13). Although MPE is frequently observed in an array of advanced malignancies, lung cancer is the most common cause, with MPE ultimately affecting 40% of patients during the course of the disease (14). In relation to potential utility in immune checkpoint therapy, there is evidence that MPE shows high concordance with tumor tissues in PD-L1 expression. Furthermore, MPE has been shown to be amenable for mutational analysis with both PCR-based and panel-based approaches (15-17). Currently little is known regarding the use of MPE for TMB evaluation in lung cancer or the level of concordance among estimates derived from WES and panel-based approaches. In this study, we evaluated the performance of five gene panels in TMB estimation by analyzing 32 pleural effusion (PE) samples from patients with advanced NSCLC. This study provides real-world data on the performance of five commercially-available targeted gene panels. Furthermore, our work also offers a general framework for TMB evaluation using MPE samples, establishing a basis for future efforts in the harmonization of panel-based TMB assessment.
We present the following article in accordance with the MDAR checklist (available at http://dx.doi.org/10.21037/atm-20-7702).
Methods
Patients and study design
Thirty-two patients diagnosed with advanced NSCLC (stage IV) accompanied by the development of PE from May 2018 to March 2019 in Shanghai Chest Hospital were prospectively enrolled in the study (NCT registration number 03546452). Both PE and blood samples were collected from each patient. CfDNA from the supernatant of PE and genomic DNA from white blood cells (WBCs) were subjected to both WES and capture-based targeted sequencing. Written informed consent was obtained from all patients under an institutional review board-approved protocol. The study was conducted in accordance with the Declaration of Helsinki (as revised in 2013) and approved by the ethics committee of Shanghai Chest Hospital of Shanghai Jiao Tong University (No. KS1856).
DNA extraction
DNA was extracted from PE using QIAamp Circulating Nucleic Acid Kit (Qiagen, CA, USA) according to the manufacturer’s protocol. DNA quantification was performed using the Qubit dsDNA HS Assay Kit (Life Technologies, CA, USA).
DNA library construction
DNA was fragmented by Covaris M220 Focused-ultrasonicator (Covaris Inc., Woburn, MA, USA) followed by end repair, phosphorylation, dA addition, and adaptor ligation for library construction. The DNA library was then purified with Agencourt AMPure XP beads (Beckman Coulter, Fullerton, CA, USA). Samples with at least 50 ng of DNA were used for library construction.
Capture-based targeted sequencing and WES
Capture-based targeted sequencing was performed using five commercially-available panels. Table 1 outlines the number of genes and lengths of genomic regions targeted by each panel. Agilent SureSelect Human All Exon V5 (50 M) (Agilent, Santa Clara, CA, USA) was used for WES. Libraries were sequenced on Illumina NextSeq 500 System (Illumina, Inc., San Diego, CA, USA) with pair-end reads.
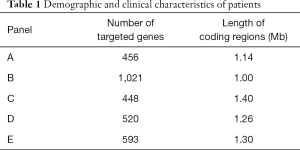
Full table
Sequencing data analysis
The raw sequencing data were preprocessed using Trimmomatic 0.36 for trimming adaptor, low-quality reads and reads less than 50 base pairs. Preprocessed sequencing data were mapped to the human genome (hg19) using Burrows-Wheeler Aligner 0.7.10 and Genome Analysis Toolkit 3.2 (Broad Institute, Cambridge, MA, USA). Variant calling was performed using VarScan, and variants were annotated with ANNOVAR and SnpEff v3.6. The allelic frequency (AF) of mutations was calculated. Mutations were then filtered against common single nucleotide polymorphisms found in 1,000 Genomes ExAC, dbSNP, and ESP6500SI-V2, ClinVar, databases.
Statistical analyses
The R-3.6.0 (R Foundation for Statistical Computing) statistics package was used to perform statistical analysis. ANOVA was used to test for statistically significant differences between panel-derived MAFs and TMBs. Correlations between TMB estimations derived by WES and each of the five panels were assessed by Pearson correlation analysis.
Results
Patient characteristics
This study prospectively enrolled 32 patients with advanced lung adenocarcinoma (stage IV) who had developed PE (NCT 03546452). Both PE and plasma samples were obtained from each patient (Figure 1). CfDNA obtained from the PE supernatant (PE-cfDNA) and genomic DNA from white blood cells (WBC-gDNA) were subjected to respective targeted sequencing. The cohort had a median age of 60 (range, 43–77) with 63.6% males (Table 2). There were 14 ‘never smokers’ and 18 current or former smokers. Table S1 presents further clinical information for each patient.
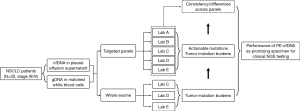
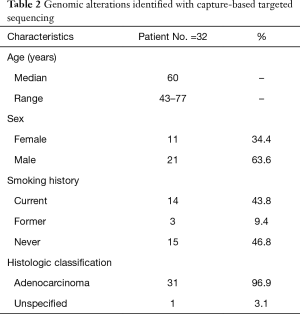
Full table
Various panels revealed generally consistent somatic mutation profiles
Genomic mutation profiling of PE-cfDNA was performed with five commercially-available panels at laboratories of their respective vendors. Key indices measuring DNA quality were summarized in Table S2. Collectively, 349 single nucleotide variations (SNVs), 75 short insertions and deletions (indels), 11 fusions, and 230 copy number variations (CNVs) were identified (Table 3). There was a high level of agreement among the five panels with respect to detection of SNVs and indels. All 32 patients were found to carry at least one mutation. All patients, except one (P29), carried at least one mutation with established clinical significance specified in guidelines issued by the US Food and Drug Administration and/or National Comprehensive Cancer Network, attesting to the sensitivity of the five gene panels under evaluation. Figure 2A shows a detailed distribution of identified mutations with known clinical significance in NSCLC. Specifically, 17 patients harbored mutations at a total of 18 SNV loci, among which nucleotide substitution occurring at 13 loci (72.2%) was detected by all five panels, three loci by four panels, and three loci by only one panel (Figure 2A). The SNV loci consistently detected were KRAS p.G12C, KRAS p.G12V, PTEN p.H93R, TP53 p.T248W, and TPP3 p.R273H. Regarding indel events, a total of 13 loci were identified in 11 patients, with nine loci in seven sites detected across all five panels (Figure 2B). These seven indel sites were EGFR p.K745_A750del, EGFR p.E746_A750del, EGFR p.E746_A750delELREA, EGFR p.E746_T751delinsVP, EGFR p.E746_S752delinsV, EGFR p.L747_S752del, and EGFR p.L747_T751del (Figure 2C).
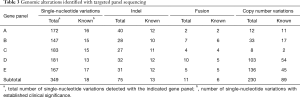
Full table
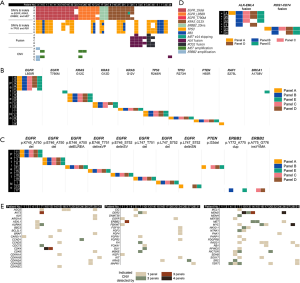
By contrast, detection of fusion and CNV events appeared less consistent. Of the 11 fusions detected, only six (54.5%) were identified by at least two panels, and two (18.1%) by all five panels (Figure 2D). Detection of copy number changes was least consistent. As shown in Figure 2E, no CNV was detected with all five panels, and of 89 CNV events in 59 genes, only eight (9.0%) were detected by at least three panels and 28 (31.5%) by at least two.
The next step involved assessing the effect of inter-vendor variation on maximum allelic frequency (MAF), an overall indicator of the rate of mutation detection. No two laboratories were assigned the same gene panel, therefore MAFs were computed based on genomic alterations that occurred within the coding regions shared by all five gene panels. The resulting median MAFs derived from the five panels fell in a narrow range (0.15–0.25%). There was also no significant difference between the MAFs reported by the five panels (P>0.99) (Figure 3A; Table S3). Rather, MAFs for indicated classic lung cancer driver mutations revealed strong correlations across panels (Figure 3B).
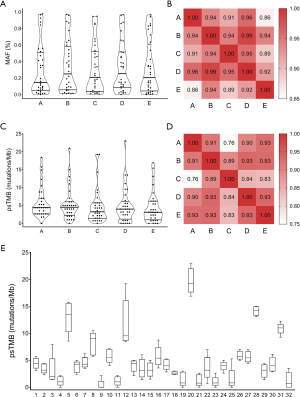
Panel-derived TMB correlated moderately to strongly with those derived from WES
Figure 3C shows that TMB estimations by panel sequencing (psTMB) were comparable across all five panels (P=0.77), with median TMBs ranging from 3.1 to 4.5 mutations/Mb. Table S4 shows psTMB for each patient. Overall, Pearson correlation analysis showed high correlation psTMB between profiles, despite inter-panel and inter-laboratory variations (Figure 3D). Furthermore, a closer look at the psTMB distribution by patient shows a reasonable range of psTMB estimates by the five panels for most patients (Figure 3E).
As WES remains the gold standard for TMB measurement, sets of psTMB estimates were compared against WES-derived TMBs (wesTMBs) to evaluate the accuracy with which each panel gauged TMB. Figure 4 shows strong correlations between wesTMB and psTMB estimates from panels B, D, and E, with Pearson coefficients of no less than 0.78. For panels A and C, weaker but moderate correlations were observed between WES-panel pairs with respective correlation coefficients of 0.73 and 0.68.
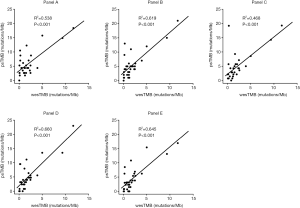
Patient classification based on psTMB estimations
We subsequently simulated the clinical scenario and compared the performance of the gene panels, classifying patients into two- and three-tier systems based on psTMB estimations, with wesTMB serving as the reference standard. In the two-tier system, the cutoff point between “low” and “high” TMB groups was 2.17 mutations/Mb, and the three-tier approach classified patients into “low” (<0.77 mutations/Mb), “intermediate” (0.77–3.44 mutations/Mb) and “high” (>3.44 mutations/Mb) TMB groups. Concordance rates between the classifications made using psTMB- and wesTMB-based systems were then calculated (Figure 5). The two- and three-tier approaches achieved concordance of 74% and 63%, respectively. For the three-tier approach, a total of 25% “low” TMB patients in WES-based grouping were classified as “high” TMB by psTMB-based systems. For instance, a singular pattern of discrepancy was seen with patient P8, who was misclassified as “high” TMB by all five panels. Similar misclassifications also occurred with patient P4, with the exception of panel C. Patient P14 was also misassigned as “high” TMB by panel A (Figure 5B). Notably, despite these overestimations of samples with low TMB levels, no high TMB PE was classified as “low” TMB by the three-tier system.
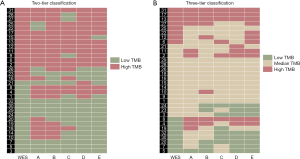
Discussion
Although blood-based liquid biopsies are frequently implemented in clinical practice, especially when tumors are unavailable, detection of ctDNA derived from plasma remains challenging due to the limited amount of ctDNA present in the circulation (18). The potential use of blood samples to assess TMB is currently under evaluation. Alternative media for mutation detection, such as PE, cerebrospinal fluid, and ascites, have been investigated (19,20). Multiple studies have confirmed that PE can be used for mutation detection (21-23). Furthermore, some studies have demonstrated that PE is a superior source to plasma for mutation detection due to higher sensitivity (15). However, a knowledge gap exists regarding the utility of panels in assessing TMB using cfDNA from MPE, which the present study aims to bridge.
Tissue-based WES is the current gold standard and has been routinely used in many trials of ICIs, including CheckMate-032 and KEYNOTE-189 (24,25); however, its relatively high costs and requirement of large quantities of tissue limit its clinical utility. Therefore, we compared the performance of five commercially-available large panels in China for assessing TMB using MPE specimens from patients with advanced NSCLC. To the best of our knowledge, this is the first validation of performance of targeted sequencing in evaluating TMB using malignant PE.
Previous in silico analyses have demonstrated the effect of panel size and coverage on TMB assessment (26,27). All panels analyzed in this study had a minimum panel size of 1 Mb (range, 1.14–1.68 Mb; Table 1), the minimum size recommended for panel-based TMB estimation (27). In this study, we provide further confirmation of the suitability of targeted gene panels covering more than 1 Mb of coding genome for TMB assessment. Moreover, our study also revealed TMB derived from panels B, D, and E showed strong correlation with wesTMB, all with a Pearson correlation coefficient of at least 0.79, whereas the remaining two panels displayed moderate correlation (Figure 4). In addition to differences in panel size, another sizable fraction of inter-vendor variation stems from the set of genes targeted by each panel. It is possible that some genes enriched for somatic mutations in NSCLC are not interrogated, leading to underestimation of TMB. In addition, various wet-lab factors and bioinformatics parameters—including, but not limited to, DNA quality, the amount of cfDNA obtained from PE and germline mutation subtraction—could all contribute to deviation from WES-derived TMB. This performance survey highlights the need for further efforts in standardizing the wet-lab processing and bioinformatic analysis in panel-based TMB estimation.
Previous studies have attempted to categorize TMB into a two- or three-tier system. Here we adopted the same setup and found that the two-tier system (cutoff 2.17 mutations/Mb) resulted in more accurate classification, with 74% agreement observed between WES- and panel-derived TMB compared to 63% agreement using a three-tier system. Interestingly, although the three-tier system allowed for a certain “intermediate” grey zone of TMB, there were still instances where “high” TMB cases determined by WES were misassigned into the “low” TMB group based on panel-based estimations. In particular, one patient (P8) was misclassified by all five panels, as well as patients P4 and P9 by four panels and two panels, respectively. This might have resulted from potential DNA degradation, which would have made certain mutations undetectable. Additionally, no “high” TMB case determined by WES was misclassified as “low” TMB based on psTMB level.
This study presented several limitations, including the limited number of cases and absence of analyses on inter-laboratory comparison of the same panel. Despite this, the five panels under evaluation yielded TMB estimate profiles that showed moderate-to-strong correlation with that derived from the gold standard. To our knowledge, this is the first study that provides evidence for the suitability of capture-based targeted sequencing in assessing TMB in PE. Using real-world specimens from advanced NSCLC cases, our study shows that the five gene panels can produce TMB estimates with reasonable accuracy. Moreover, despite inherent differences—such as sets of genomic regions targeted by different panels—factors like wet-lab processing and bioinformatic analysis could play a role in introducing deviation. In this sense, the data generated in this study could also provide a foundation for understanding the crucial steps and parameters in targeted sequencing analysis with the aim of creating an optimal, standard protocol for assessing TMB in PE. Future studies of larger scale are warranted to validate findings from this study.
Acknowledgments
We would like to express our gratitude to Xiao Zou, Bing Li, Han Han-Zhang, Jianxing Xiang, and Zhou Zhang from Burning Rock Biotechnology, Ziwei Fan and Zhan Huang from Amoy Diagnostics, as well as teams from Geneplus Co., Berry Genomics, and Sinotech Genomics for their valuable assistance in data extraction, statistical analysis, and manuscript preparation.
Funding: This work was supported by funds provided to Dr. Lu by the National Key R&D Program of China (2016YFC1303300), the National Natural Science Foundation of China (81672272), Shanghai Municipal Science & Technology Commission Research Project (17431906103), Shanghai Chest Hospital Project of Collaborative Innovation (YJXT20190105), Clinical Research Plan of SHDC (16CR3005A), and to Dr. Yu by grants from Shanghai Municipal Science and Technology Commission Research Project (16431903200).
Footnote
Reporting Checklist: The authors have completed the MDAR checklist. Available at http://dx.doi.org/10.21037/atm-20-7702
Data Sharing Statement: Available at http://dx.doi.org/10.21037/atm-20-7702
Conflicts of Interest: All authors have completed the ICMJE uniform disclosure form (available at http://dx.doi.org/10.21037/atm-20-7702). The authors have no conflicts of interest to declare.
Ethical Statement: The authors are accountable for all aspects of the work in ensuring that questions related to the accuracy or integrity of any part of the work are appropriately investigated and resolved. The study was conducted in accordance with the Declaration of Helsinki (as revised in 2013). This study was approved by the ethics committee of Shanghai Chest Hospital of Shanghai Jiao Tong University (No. KS1856), and all patients provided written informed consent. All samples were tested at clinical genomics testing laboratories (Burning Rock Biotechnology, Amoy Diagnostics, Geneplus Co., Berry Genomics, and Sinotech Genomics) using protocols approved by the ethics committee of Shanghai Chest Hospital of Shanghai Jiao Tong University.
Open Access Statement: This is an Open Access article distributed in accordance with the Creative Commons Attribution-NonCommercial-NoDerivs 4.0 International License (CC BY-NC-ND 4.0), which permits the non-commercial replication and distribution of the article with the strict proviso that no changes or edits are made and the original work is properly cited (including links to both the formal publication through the relevant DOI and the license). See: https://creativecommons.org/licenses/by-nc-nd/4.0/.
References
- Gong J, Chehrazi-Raffle A, Reddi S, et al. Development of PD-1 and PD-L1 inhibitors as a form of cancer immunotherapy: A comprehensive review of registration trials and future considerations. J Immunother Cancer 2018;6:8. [Crossref] [PubMed]
- Jenkins RW, Barbie DA, Flaherty KT. Mechanisms of resistance to immune checkpoint inhibitors. Br J Cancer 2018;118:9-16. [Crossref] [PubMed]
- Camidge DR, Doebele RC, Kerr KM. Comparing and contrasting predictive biomarkers for immunotherapy and targeted therapy of NSCLC. Nat Rev Clin Oncol 2019;16:341-55. [Crossref] [PubMed]
- Schumacher TN, Schreiber RD. Neoantigens in cancer immunotherapy. Science 2015;348:69-74. [Crossref] [PubMed]
- Snyder A, Makarov V, Merghoub T, et al. Genetic basis for clinical response to CTLA-4 blockade in melanoma. N Engl J Med 2014;371:2189-99. [Crossref] [PubMed]
- Rizvi NA, Hellmann MD, Snyder A, et al. Mutational landscape determines sensitivity to PD-1 blockade in non-small cell lung cancer. Science 2015;348:124-8. [Crossref] [PubMed]
- Hellmann MD, Callahan MK, Awad MM, et al. Tumor Mutational Burden and Efficacy of Nivolumab Monotherapy and in Combination with Ipilimumab in Small-Cell Lung Cancer. Cancer Cell 2018;33:853-61.e4. [Crossref] [PubMed]
- Balar AV, Galsky MD, Rosenberg JE, et al. Atezolizumab as first-line treatment in cisplatin-ineligible patients with locally advanced and metastatic urothelial carcinoma: a single-arm, multicentre, phase 2 trial. Lancet 2017;389:67-76. [Crossref] [PubMed]
- Rizvi H, Sanchez-Vega F, La K, et al. Molecular determinants of response to anti-programmed cell death (PD)-1 and anti-programmed death-ligand 1 (PD-L1) blockade in patients with non-small-cell lung cancer profiled with targeted next-generation sequencing. J Clin Oncol 2018;36:633-41. [Crossref] [PubMed]
- Gandara DR, Paul SM, Kowanetz M, et al. Blood-based tumor mutational burden as a predictor of clinical benefit in non-small-cell lung cancer patients treated with atezolizumab. Nat Med 2018;24:1441-8. [Crossref] [PubMed]
- Li L, Wang Y, Shi W, et al. Serial ultra-deep sequencing of circulating tumor DNA reveals the clonal evolution in non-small cell lung cancer patients treated with anti-PD1 immunotherapy. Cancer Med 2019;8:7669-78. [Crossref] [PubMed]
- Fenton KN, David Richardson J. Diagnosis and management of malignant pleural effusions. Am J Surg 1995;170:69-74. [Crossref] [PubMed]
- Porcel JM, Gasol A, Bielsa S, et al. Clinical features and survival of lung cancer patients with pleural effusions. Respirology 2015;20:654-9. [Crossref] [PubMed]
- Morgensztern D, Waqar S, Subramanian J, et al. Prognostic impact of malignant pleural effusion at presentation in patients with metastatic non-small-cell lung cancer. J Thorac Oncol 2012;7:1485-9. [Crossref] [PubMed]
- Guo Z, Xie Z, Shi H, et al. Malignant pleural effusion supernatant is an alternative liquid biopsy specimen for comprehensive mutational profiling. Thorac Cancer 2019;10:823-31. [Crossref] [PubMed]
- Liu X, Lu Y, Zhu G, et al. The diagnostic accuracy of pleural effusion and plasma samples versus tumour tissue for detection of EGFR mutation in patients with advanced non-small cell lung cancer: Comparison of methodologies. J Clin Pathol 2013;66:1065-9. [Crossref] [PubMed]
- Shin S, Kim J, Kim Y, et al. Assessment of real-time PCR method for detection of EGFR mutation using both supernatant and cell pellet of malignant pleural effusion samples from non-small-cell lung cancer patients. Clin Chem Lab Med 2017;55:1962-9. [Crossref] [PubMed]
- Rapisuwon S, Vietsch EE, Wellstein A. Circulating biomarkers to monitor cancer progression and treatment. Comput Struct Biotechnol J 2016;14:211-22. [Crossref] [PubMed]
- Li YS, Jiang BY, Yang JJ, et al. Unique genetic profiles from cerebrospinal fluid cell-free DNA in leptomeningeal metastases of EGFR-mutant non-small-cell lung cancer: A new medium of liquid biopsy. Ann Oncol 2018;29:945-52. [Crossref] [PubMed]
- Zhou S, Xu B, Qi L, et al. Next-generation sequencing reveals mutational accordance between cell-free DNA from plasma, malignant pleural effusion and ascites and directs targeted therapy in a gastric cancer patient. Cancer Biol Ther 2019;20:15-20. [Crossref] [PubMed]
- Buttitta F, Felicioni L, Del Grammastro M, et al. Effective assessment of egfr mutation status in bronchoalveolar lavage and pleural fluids by next-generation sequencing. Clin Cancer Res 2013;19:691-8. [Crossref] [PubMed]
- Carter J, Miller JA, Feller-Kopman D, et al. Molecular profiling of malignant pleural effusion in metastatic non-small-cell lung carcinoma the effect of preanalytical factors. Ann Am Thorac Soc 2017;14:1169-76. [PubMed]
- Yang SR, Lin CY, Stehr H, et al. Comprehensive Genomic Profiling of Malignant Effusions in Patients with Metastatic Lung Adenocarcinoma. J Mol Diagn 2018;20:184-94. [Crossref] [PubMed]
- Janjigian YY, Bendell J, Calvo E, et al. CheckMate-032 Study: Efficacy and Safety of Nivolumab and Nivolumab Plus Ipilimumab in Patients With Metastatic Esophagogastric Cancer. J Clin Oncol 2018;36:2836-44. [Crossref] [PubMed]
- Garassino MC, Gadgeel SM, Rodriguez-Abreu D, et al. Evaluation of blood TMB (bTMB) in KEYNOTE-189: Pembrolizumab (pembro) plus chemotherapy (chemo) with pemetrexed and platinum versus placebo plus chemo as first-line therapy for metastatic nonsquamous NSCLC. J Clin Oncol 2020;38:9521. [Crossref]
- Budczies J, Allgäuer M, Litchfield K, et al. Optimizing panel-based tumor mutational burden (TMB) measurement. Ann Oncol 2019;30:1496-506. [Crossref] [PubMed]
- Buchhalter I, Rempel E, Endris V, et al. Size matters: Dissecting key parameters for panel-based tumor mutational burden analysis. Int J Cancer 2019;144:848-58. [Crossref] [PubMed]
(English Language Editor: M. Hawkins)