DNA damage response as a prognostic indicator in metastatic breast cancer via mutational analysis
Introduction
Breast cancer is recognized as the most common female malignant tumor, and is the leading cause of death from metastasis (1). A growing number of studies have reported mutational characteristics of metastatic breast cancer (MBC), indicating genomic evolution and high tumor heterogeneity in this disease (2-7). However, useful prognostic biomarkers that could reliably pinpoint the MBC patients most likely to benefit from specific and tailored treatment have yet to be identified. A circulating tumor DNA (ctDNA) analysis of MBC with largest scale in China was undertaken in the current study, to comprehensively interpret the genomic features and reveal the prognostic relevance of somatic mutations in MBC.
Recently ctDNA assays have been used in metastatic and recurrent cancers. Given the intra-tumor heterogeneity of breast cancer, the genomic map of tissue specimens may not represent the whole tumor (8). Additionally, it is difficult to obtain tissue samples of metastatic foci in clinical practice, as MBC commonly involves other metastatic organs such as the liver, lung, bone, or brain. Recently, ctDNA has been proposed as an accurate means to identify mutations, assess tumor burden, and dynamically monitor treatment response with minimally invasive procedures (9-12). In this study, 958 blood samples of MBC were collected, ctDNA was extracted, and target capture sequencing was performed. Survival analysis was subsequently carried out to explore the prognostic relevance of somatic alterations. We present the following article in accordance with the STROBE reporting checklist (available at http://dx.doi.org/10.21037/atm-20-2137).
Methods
Patient selection
The study was approved by the Institutional Review Board and Human Ethics Committee of at National Cancer Center/ National Clinical Research Center for Cancer/Cancer Hospital, Chinese Academy of Medical Science and Peking Union Medical College (No. CH-BC-052). All patients provided written informed consent prior to study enrollment. The study was conducted in accordance with the Declaration of Helsinki (as revised in 2013).
A total of 958 female MBC patients were enrolled at the time of diagnosis (TNM stage: M1) between June, 2015, and August, 2019. Hormone receptor (HR), including estrogen receptor (ER), progesterone receptor (PR), and human epidermal growth factor receptor 2 (HER2) expressions were assessed by two independent pathologists in accordance with standard clinical practice.
- DNA Extraction and target capture sequencing:
Blood samples were obtained and the plasma was separated from peripheral blood cells via centrifugation. Cell-free DNA was extracted from the plasma with QIAamp Circulating Nucleic Acid Kits (Qiagen, Hilden, Germany). Genomic DNA was isolated from peripheral blood cells with QIAamp DNA Blood Mini Kits (Qiagen, Hilden, Germany. Capture probes were designed, covering coding sequences and hot exons of 1021 genes. DNA sequencing was performed with the HiSeq 3000 Sequencing System (Illumina, San Diego, CA, USA). All procedures were conducted according to the manufacturers’ instructions (13). - Sequencing data analysis:
After removing the terminal adaptor sequences and low-quality data, the reads were mapped to the human reference genome. Variant calling was performed using Genome Analysis ToolKit (https://www.broadinstitute.org/gatk/, GATK) for single nucleotide variants (SNVs), small insertions and deletions (indels) in somatic DNA by filtering peripheral blood sequencing data. All final candidate variants were manually verified using the Integrative Genomics Viewer (IGV) Browser. The method of sequencing has been previously described by Hu et al. (14). - Next generation sequencing (NGS) datasets and enrichment analysis:
The NGS dataset comprised 128 non-metastatic breast cancer cases (NMBC, M0 stage), processed with the same panel as the MBC cohort. The public dataset which included MBC information from Memorial Sloan Kettering Cancer Center (MSK) was selected for comparison. These datasets were age-matched to the study group and of a similar HR expression profile. Enrichment analysis was performed via Metascape (15). This web-based tool was used to integrate functional enrichment, interactome analysis, gene annotation, and protein-protein networks (http://metascape.org/gp/index.html#/main/step1). - Driver mutations analysis:
OncodriveFML (http://www.intogen.org/oncodrivefml) was used to identify driver mutations for pattern analysis of somatic mutations across tumors and elucidate their involvement in carcinogenesis. OncodriveFML integrated different scoring frameworks and predicted the impact of mutations on gene function (16). - Tumor mutation burden (TMB) and mutant-allele tumor heterogeneity (MATH) calculation:
TMB and MATH have been frequently used to assess somatic mutations due to their established association with tumor heterogeneity. TMB was calculated using the number of non-synonymous somatic mutations per mega-base in coding regions (14). To analyze the effect of intra-tumor heterogeneity, MATH was the normalized width distribution of mutant-allele fractions among tumor-specific mutated loci (17). - Statistical analysis:
Differences of categorical and numerical variables were performed using Fisher’s and Mann–Whitney U tests, respectively. The relationship between two variables was assessed using Pearson’s or Spearman’s correlation test. Statistical significance was considered when P<0.05. PFS was assessed using a Kaplan-Meier survival plot. All statistical analyses were performed using GraphPad Prism 5 (GraphPad Software, San Diego, CA) or R (18).
Results
Mutational landscape of MBC
Mutations were identified in 801 of 958 blood samples from female MBC patients, comprising 494 HR+ cases (luminal A and B), 130 HER2+ cases, and 177 triple-negative breast cancer (TNBC) cases. All patients were diagnosed at the M1 stage, and the cohort ranged in age from 22–76 years old, with a median age of 46. The involved distant organs included the lung, liver, bone, and brain. Extracted ctDNA was assayed for somatic mutations by target capture NGS with a panel of 1,021 genes. The patient’s characteristics of the identified MBC cases are summarized in Table 1.
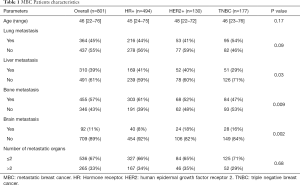
Full table
There were 5,829 nonsynonymous mutations identified in 663 genes, including 4,590 missense mutations, 474 nonsense mutations, 370 deletions of small fragment, and 182 insertions of small fragment in coding sequence. The most frequently altered genes were tumor protein p53 (TP53, 54%), phosphatidylinositol-4,5-bisphosphate 3-kinase, catalytic subunit alpha (PIK3CA, 41%), estrogen receptor 1 (ESR1, 12%), myeloid/lymphoid or mixed-lineage leukemia protein 3 (MLL3, 11%), DNA (cytosine-5)-methyltransferase 3A (DNMT3A, 10%), erb-b2 receptor tyrosine kinase 2 (ERBB2, 10%), GATA binding protein 3 (GATA3, 8%), FAT atypical cadherin 1 (FAT1, 7%), phosphatase and tensin homolog (PTEN, 6%), and mitogen-activated protein kinase kinase kinase 1 (MAP3K1, 6%) (Figure 1). The frequency of mutations observed in this current study was concordant with tissue-based results from the matched MSK dataset (R2=0.8395) (Figure S1, Table S1). Despite the lower mutation frequency, the variant allele frequency (VAF) of PTEN was higher than that of PIK3CA (Figure 2A). Moreover, the TMB per sample ranged from 1-111, with a median of 5. Mutated TP53 significantly co-existed with PIK3CA, neurofibromatosis type 1 (NF1), myeloid/lymphoid or mixed-lineage leukemia protein 2 (MLL2), ERBB2, and retinoblastoma protein (RB1). Meanwhile, ESR1, GATA3, and DNMT3A were revealed to be mutually exclusive genes (Figure 2B).
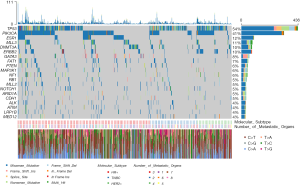
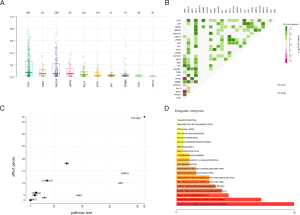
Analysis of our data in the Oncogenic Signaling Pathways in The Cancer Genome Atlas (19) revealed that the affected genes were mostly involved in the RTK-RAS, PI3K/Akt and Notch pathways. It was also noted that the p53 pathway was associated with the highest frequency of affected genes (Figure 2C). A total of 16 druggable genes including PTEN, TP53, ESR1, RB1, notch receptor 1 (NOTCH1), ALK receptor tyrosine kinase (ALK), ERBB2, ATM serine/threonine kinase (ATM), PIK3CA, GATA3, mediator complex subunit 12 (MED12), cadherin 1 (CDH1), DNMT3A, MAP3K1, NF1, and AT-rich interaction domain 1A (ARID1A) were identified and classified into 20 target categories, based on Drug Gene Interaction database (Figure 2D). The mutated genes were associated with a pathway in cancer (hsa05200), disease of signal transduction (R-HSA-5663202), and EGFR tyrosine kinase inhibitor resistance (hsa0521) (Figure S2).
Enriched mutations in MBC
In the current study (n=801), 10 differentially mutated genes of MBC (Table S1) were recognized when compared to matched NMBCs (Table S2) derived from our ctDNA dataset (n=128) (Figure 3A). Considering the important role of molecular subtypes in the diagnosis and treatment of breast cancer, we compared mutational frequencies of metastatic HR+ (n=494), HER2+ (n=130), and TNBC (n=177) subtypes. TP53, ERBB2, and MSH2 were more prevalently altered in metastatic HER2+ cases; TP53 and PIK3CA were differentially mutated in metastatic TNBCs; and ESR1 and GATA3 occurred more frequently in HR+ subtype (Figure 3B,C,D).
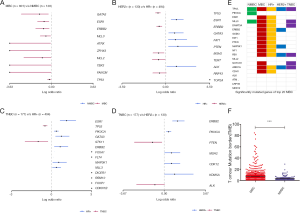
Through OncodriveFML (q<0.1), 56 driver genes were identified in MBC, with only 3 found in NMBC. Of the top 20 frequently mutated genes in MBC, 14 were interpreted as driver genes. It was found that the driving effect of PIK3CA in NMBC was not shown in MBC. Within the molecular subtypes of MBC, 52 driver genes were observed in the HR+ group, 14 in the HER2+ group, and 9 in the TNBC group. TP53, RB1, and ARID1A were shared driver genes, regardless of subtypes. ESR1, GATA3, FAT1, NF1, PTEN, and CDH1 were specific driver genes in the HR+ subtype, while NOTCH1 showed a strong correlation with TNBC cases (Figure 3E). Additionally, TMB was found to be significantly higher in MBC than NMBC (F). An association was observed between the number of metastasis and gene alterations (P=0.0003). Furthermore, a significant difference between mutational burden in lung metastasis and liver metastasis (P=0.0467) (Figure S3) was noted. Through Metascape, it was shown that different pathways were involved with different metastatic sites in MBC (Table S3).
Prognostic relevance of somatic mutations in MBC
The relationship between highly mutated genes and cancer prognosis was investigated. Follow-up data from 126 MBC cases in this study revealed that TP53 or ERBB2 mutations led to shorter PFS (Figure 4A,B). Co-existence of TP53 and PIK3CA mutations was significantly associated with worse prognosis (Figure 4C). Mutations of DNA damage response (DDR) genes have been considered to play a critical role in maintaining genomic stability (19). Our findings identified a total of 85 mutated DDR genes from 570 MBC cases, while 35 altered DDR genes were found in 71 NMBC cases, highlighting a significant difference between these two cohorts (Figure 4D). The distribution of DDR gene mutations varied among different molecular subtypes (Figure 4E). MBC cases with DDR mutations showed higher TMB and MATH scores compared to those without DDR mutations (Figure 4F,G). More importantly, MBC patients with DDR mutations displayed shorter PFS than those without DDR mutations (Figure 4H).
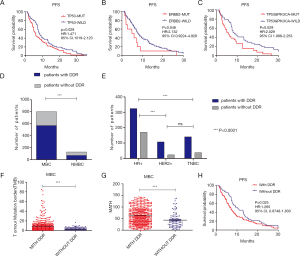
Characteristics of TMB or MATH in MBC
TMB was higher in MBC cases with two or more metastatic organs than those with single distant organ involvement (Figure 5A). Mutations in TP53, PIK3CA, ESR1, MLL3, ERBB2, GATA3, FAT1, PTEN, MAP3K1, and NF1 were closely related with higher TMB (Figure 5B,C,D,E,F,G,H,I,J,K). However, no direct association was found between TMB and PFS (Figure 5L). MATH score was higher in MBC cases with multiple metastatic organs (Figure 5M). TP53, MLL3, ERBB2, and FAT1 mutations were significantly associated with MATH scores (Figure 5N,O,P,Q). No relationship was observed between MATH score and PFS in MBC (Figure 5R).
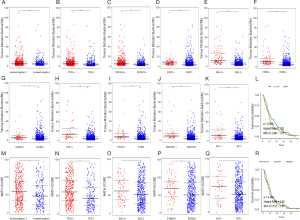
Discussion
This study exploited the multiple advantages of using liquid biopsies as a means of conducting large-scale genomic profiling of MBC via ctDNA analysis. TP53 and PIK3CA alterations appeared most prevalent, followed by PTEN, which warrants attention. It has been reported that PTEN mutations frequently occur in various cancers, particularly in the breast (20) and participate in the PI3K/AKT/mTOR and P53 pathways (21). In the current study, PTEN showed significant correlation with TP53, displaying a co-existing pattern.
Based on our data, the spectrum of genes identified in MBC during the analysis was differed from NMBC, indicating an evolutionary process and tumor heterogeneity. The differentially mutated genes were reported to be strongly associated with tumor progression and poor prognosis. RB1 mutation was associated with poor outcomes in the HR+ subtype, resulting in a low response to cyclin-dependent kinase 4 (CDK4) inhibitors (6,22), thereby warranting new target therapies (23). Interestingly, this study showed RB1 to co-occur with set domain containing 2, histone lysine methyltransferase (SETD2) and MLL2. NF1, which displayed a relationship with low density lipoprotein receptor-related protein 1B (LPR1B) and FAT atypical cadherin 2 (FAT2), has also been involved in resistance to endocrine therapy (24). MAP3K1 was reported to be responsible for regulating the transcription of important cancer genes including c-Myc, c-Elk1, c-Jun, and c-Fos (25). Based on our data, MAP3K1 was strongly associated with the HR+ subtype. NOTCH1, one of the key receptors in the Notch signaling pathway, could promote proliferation, invasion and metastasis of cancer cells (26). It was reported that the inhibition of NOTCH1 may prevent the pathogenesis and metastasis of breast cancer (27). NOTCH1 mutations were mainly found in the TNBC subtype in this study. Unlike TP53, ERBB2, or DDR alterations, no prognostic relevance was shown between RB1, NF1, MAP3K1, and NOTCH1 and MBC according to our data; further research with larger cohorts might be needed.
Compared to normal cells, cancer cells have attenuated DNA repair capacity, driving tumor formation (28). Recent studies have found that DDR alterations are independently associated with response to PD-1/PD-L1 blockade (29). In this study, the proportion of mutated DDR genes in MBC was higher than that in NMBC. The distribution of DDR mutations varied with different subtypes of MBC. DDR mutations were significantly associated with TMB, MATH score, and PFS, suggesting a relationship with heterogeneity and prognosis (30).
It is much argued that ctDNA has a similar mutational profile to gDNA. Given the occurrence of tumor heterogeneity and difficulties in obtaining samples of distant metastasis in clinical practice, ctDNA analysis may therefore provide a convenient way to identify mutations and assess tumor burden in MBC. The frequency of mutations observed in this study was concordant with tissue-based results from the matched MSK dataset (R2=0.8395). However, the validation of ctDNA application needs further and more comprehensive investigation. The potential target genes identified in the current study were evaluated in relation to the existing Genome-Wide Association Studies (GWAS). Most risk variants detected through GWAS were found in non-coding regions (31,32), highlighting the importance of using large-scale genomic data.
We investigated the genomic characteristics and prognostic indicators, based on a large MBC cohort with ctDNA analysis. The mutational profile of MBC was shown to be different from early stage of breast cancer and varied across multiple subtypes, including driver genes. TP53, ERBB2, or coexisting TP53/PIK3CA mutations in MBC contributed to shorter PFS. Furthermore, DDR mutations in MBC were significantly associated with TMB and MATH score, as well as unfavorable prognosis.
Acknowledgments
Funding: This work was supported by National Key R&D Program of China (2018YFC1312101) CAMS Initiative for Innovative Medicine (CAMS-12M-1-010, 2017-12M-3-004), Major Project of Beijing Municipal Science and Technology Commission (D161100000816004), National Science and Technology Major Project of the Ministry of Science and Technology, China (2015ZX09101007).
Footnote
Reporting Checklist: The authors have completed the STROBE reporting checklist. Available at http://dx.doi.org/10.21037/atm-20-2137
Data Sharing Statement: Available at http://dx.doi.org/10.21037/atm-20-2137
Conflicts of Interest: All authors have completed the ICMJE uniform disclosure form (available at http://dx.doi.org/10.21037/atm-20-2137). The authors have no conflicts of interest to declare.
Ethical Statement: The authors are accountable for all aspects of the work in ensuring that questions related to the accuracy or integrity of any part of the work are appropriately investigated and resolved. The study was conducted in accordance with the Declaration of Helsinki (as revised in 2013), approved by the Institutional Review Board and Human Ethics Committee of at National Cancer Center/ National Clinical Research Center for Cancer/ Cancer Hospital, Chinese Academy of Medical Science and Peking Union Medical College (No. CH-BC-052) and informed consent was obtained from all the patients.
Open Access Statement: This is an Open Access article distributed in accordance with the Creative Commons Attribution-NonCommercial-NoDerivs 4.0 International License (CC BY-NC-ND 4.0), which permits the non-commercial replication and distribution of the article with the strict proviso that no changes or edits are made and the original work is properly cited (including links to both the formal publication through the relevant DOI and the license). See: https://creativecommons.org/licenses/by-nc-nd/4.0/.
References
- Siegel RL, Miller KD, Jemal A. Cancer statistics, 2019. CA Cancer J Clin 2019;69:7-34. [Crossref] [PubMed]
- Ng CKY, Bidard FC, Piscuoglio S, et al. Genetic Heterogeneity in Therapy-Naive Synchronous Primary Breast Cancers and Their Metastases. Clin Cancer Res 2017;23:4402-15. [Crossref] [PubMed]
- Wang Y, Waters J, Leung ML, et al. Clonal evolution in breast cancer revealed by single nucleus genome sequencing. Nature 2014;512:155-60. [Crossref] [PubMed]
- Gerlinger M, Rowan AJ, Horswell S, et al. Intratumor heterogeneity and branched evolution revealed by multiregion sequencing. N Engl J Med 2012;366:883-92. [Crossref] [PubMed]
- Navin N, Kendall J, Troge J, et al. Tumour evolution inferred by single-cell sequencing. Nature 2011;472:90-4. [Crossref] [PubMed]
- Bertucci F, Ng CKY, Patsouris A, et al. Genomic characterization of metastatic breast cancers. Nature 2019;569:560-4. [Crossref] [PubMed]
- Yates LR, Knappskog S, Wedge D, et al. Genomic Evolution of Breast Cancer Metastasis and Relapse. Cancer Cell 2017;32:169-84 e7.
- Ragoussis J. Regulators of Asymmetric Cell Division in Breast Cancer. Trends Cancer 2018;4:798-801. [Crossref] [PubMed]
- Parsons HA, Beaver JA, Ciminomathews A, et al. Individualized Molecular Analyses Guide Efforts (IMAGE): A Prospective Study of Molecular Profiling of Tissue and Blood in Metastatic Triple-Negative Breast Cancer. Clin Cancer Res 2017;23:379-86. [Crossref] [PubMed]
- Riva F, Bidard F, Houy A, et al. Patient-Specific Circulating Tumor DNA Detection during Neoadjuvant Chemotherapy in Triple-Negative Breast Cancer. Clin Chem 2017;63:691-9. [Crossref] [PubMed]
- Vandekerkhove G, Todenhofer T, Annala M, et al. Circulating Tumor DNA Reveals Clinically Actionable Somatic Genome of Metastatic Bladder Cancer. Clin Cancer Res 2017;23:6487-97. [Crossref] [PubMed]
- Dawson S, Tsui DWY, Murtaza M, et al. Analysis of circulating tumor DNA to monitor metastatic breast cancer. N Engl J Med 2013;368:1199-209. [Crossref] [PubMed]
- Yi Z, Ma F, Li C, et al. Landscape of somatic mutations in different subtypes of advanced breast cancer with circulating tumor DNA analysis. Sci Rep 2017;7:5995. [Crossref] [PubMed]
- Hu Z, Xie N, Tian C, et al. Identifying Circulating Tumor DNA Mutation Profiles in Metastatic Breast Cancer Patients with Multiline Resistance. EBioMedicine 2018;32:111-8. [Crossref] [PubMed]
- Zhou Y, Zhou B, Pache L, et al. Metascape provides a biologist-oriented resource for the analysis of systems-level datasets. Nat Commun 2019;10:1523. [Crossref] [PubMed]
- Mularoni L, Sabarinathan R, Deu-Pons J, et al. OncodriveFML: a general framework to identify coding and non-coding regions with cancer driver mutations. Genome Biol 2016;17:128. [Crossref] [PubMed]
- Mroz EA, Rocco JW. MATH, a novel measure of intratumor genetic heterogeneity, is high in poor-outcome classes of head and neck squamous cell carcinoma. Oral Oncol 2013;49:211-5. [Crossref] [PubMed]
- Mayakonda A, Lin DC, Assenov Y, et al. Maftools: efficient and comprehensive analysis of somatic variants in cancer. Genome Res 2018;28:1747-56. [Crossref] [PubMed]
- Pearl LH, Schierz AC, Ward SE, et al. Therapeutic opportunities within the DNA damage response. Nat Rev Cancer 2015;15:166-80. [Crossref] [PubMed]
- M JR. S V. BMI1 and PTEN are key determinants of breast cancer therapy: A plausible therapeutic target in breast cancer. Gene 2018;678:302-11. [Crossref] [PubMed]
- Leslie NR, Foti M. Non-genomic loss of PTEN function in cancer: not in my genes. Trends Pharmacol Sci 2011;32:131-40. [Crossref] [PubMed]
- Li Z, Razavi P, Li Q, et al. Loss of the FAT1 Tumor Suppressor Promotes Resistance to CDK4/6 Inhibitors via the Hippo Pathway. Cancer Cell 2018;34:893-905.e8. [Crossref] [PubMed]
- Knudsen ES, Wang JY. Targeting the RB-pathway in cancer therapy. Clin Cancer Res 2010;16:1094-9. [Crossref] [PubMed]
- Razavi P, Chang MT, Xu G, et al. The Genomic Landscape of Endocrine-Resistant Advanced Breast Cancers. Cancer Cell 2018;34:427-38 e6.
- Rebbeck TR, DeMichele A, Tran TV, et al. Hormone-dependent effects of FGFR2 and MAP3K1 in breast cancer susceptibility in a population-based sample of post-menopausal African-American and European-American women. Carcinogenesis 2009;30:269-74. [Crossref] [PubMed]
- Chen W, Cao G, Yuan X, et al. Notch-1 knockdown suppresses proliferation, migration and metastasis of salivary adenoid cystic carcinoma cells. J Transl Med 2015;13:167. [Crossref] [PubMed]
- Pal D, Kolluru V, Chandrasekaran B, et al. Targeting aberrant expression of Notch-1 in ALDH(+) cancer stem cells in breast cancer. Mol Carcinog 2017;56:1127-36. [Crossref] [PubMed]
- Jeggo PA, Lobrich M. How cancer cells hijack DNA double-strand break repair pathways to gain genomic instability. Biochem J 2015;471:1-11. [Crossref] [PubMed]
- Teo MY, Seier K, Ostrovnaya I, et al. Alterations in DNA Damage Response and Repair Genes as Potential Marker of Clinical Benefit From PD-1/PD-L1 Blockade in Advanced Urothelial Cancers. J Clin Oncol 2018;36:1685-94. [Crossref] [PubMed]
- Thomas A, Routh ED, Pullikuth A, et al. Tumor mutational burden is a determinant of immune-mediated survival in breast cancer. Oncoimmunology 2018;7:e1490854. [Crossref] [PubMed]
- Ferreira MA, Gamazon ER, Al-Ejeh F, et al. Genome-wide association and transcriptome studies identify target genes and risk loci for breast cancer. Nat Commun 2019;10:1741. [Crossref] [PubMed]
- Escala-Garcia M, Guo Q, Dork T, et al. Genome-wide association study of germline variants and breast cancer-specific mortality. Br J Cancer 2019;120:647-57. [Crossref] [PubMed]