A deep learning-based quantitative computed tomography model for predicting the severity of COVID-19: a retrospective study of 196 patients
Introduction
Coronavirus disease 2019 (COVID-19), caused by the novel severe acute respiratory syndrome coronavirus 2 (SARS-CoV-2, previously known as 2019-nCoV), has become increasingly prevalent worldwide. As of July 18, 13,876,441 cases had been confirmed globally with 953,087 deaths according to World Health Organization (WHO) statistics (1). With a death toll exceeding that of the severe acute respiratory syndrome coronavirus (SARS-CoV) outbreak in 2002 and 2003, COVID-19 has led to a public health emergency of international concern, putting all health organizations on high alert (2).
COVID-19 can be fatal, with a case fatality rate of 2% (3), making it the third most fatal disease caused by the coronavirus family after SARS-CoV infection (fatality rates of 10%) and Middle East respiratory syndrome coronavirus (MERS-CoV) infection (fatality rates of 37%) (4). Although the fatality rate of COVID-19 is relatively low, it can be widely and quickly transmitted from person-to-person (5), and 17% to 29% of patients with COVID-19 develop severe or even fatal acute respiratory distress syndrome (ARDS) (6-8). However, there are still no efficient treatments or control strategies available today. Therefore, in addition to early diagnosis and quarantine, timely identification of the patients who may become critically ill is pivotal for subsequent active interventions, such as triaging patients, designing treatment protocols and performing follow-up evaluations to improve clinical outcomes.
To date, although several preliminary studies have reported the clinical and imaging features of COVID-19 (6-9), the comprehensive prediction of disease severity is limited. Previous studies have demonstrated that the extent of consolidation or ground-glass opacity (GGO) on chest computed tomography (CT), which is significantly different between ARDS and non-ARDS groups, may play an important prognostic role in viral pneumonias such as influenza A (H1N1), SARS, and MERS (10-12). In recent years, deep learning (DL), one of the major subfields of artificial intelligence (AI), has played a central role in image analysis, and has been proposed as a potential method for the analysis of diffuse lung disease on CT (13,14). DL is more effective in automatically measuring lesion range than the traditional visual evaluation of CT does.
Therefore, we hypothesized that DL-based quantitative CT measurements of lesion extent on admission, together with clinical features, will help predict the severity of COVID-19. The purpose of this study was to characterize these baseline clinico-radiological features and to establish a model for predicting the severity of COVID-19. We present the following article in accordance with the TRIPOD reporting checklist (available at http://dx.doi.org/10.21037/atm-20-2464).
Methods
Study design and participants
The Institutional Review Board of Shanghai Public Health Clinical Center, Fudan University approved the study protocol (No. YJ-2020-S035-01). The requirement for informed consent was waived because of the retrospective nature of the study. The study was conducted in accordance with the Declaration of Helsinki (as revised in 2013). The inclusion criteria were as follows: (I) patients with positive new coronavirus nucleic acid through real-time PCR (RT-PCR) performed by the Centre for Disease Control (CDC), Shanghai; and (II) patients with thin-section chest CT scans demonstrating pneumonia. Ten patients with normal CT images were excluded. A total of 196 patients with COVID-19 were enrolled from January 20 to February 10, 2020 in our centre.
According to the COVID-19 Guidelines (the fifth version) set by the National Health Commission of the People’s Republic of China (15), patients with COVID-19 can be divided into four subtypes: mild, common, severe and critically ill. As the mild subtype with no pneumonia was excluded, the patients enrolled in this study were divided into non-severe (common subtype, 151 cases) and severe (severe and critically ill subtypes, 45 cases). Among the severe group, 28 patients had severe pneumonia, 15 had ARDS, and 2 developed shock. The clinical and laboratory data were reviewed. The pneumonia severity index (PSI) was calculated for all patients (16).
Imaging acquisition
All patients underwent CT examinations with a 64-section scanner (Scenaria, HITACHI, Japan) at full inspiration from the thoracic inlet to the costophrenic angle level with the following parameters: detector width, 64×0.625 mm; pitch, 1.57; tube voltage, 120 kV; automatic tube current with advanced mode (maximum: 400 mA, minimum: 150 mA); rotation time, 0.35 seconds; and matrix, 512×512. The images were reconstructed using a standard lung algorithm with a thickness of 1 mm and an interval of 0.8 mm. The following windows were used: a mediastinal window with a window width of 350 Hounsfield unit (HU) and a window level of 40 HU, and a lung window with a width of 1,200 HU and a level of −600 HU.
Image interpretation
The presence and distribution of pulmonary opacities were analysed, including the following four types (Figure 1): type I, pure ground-glass opacity (pGGO), which manifested as a hazy opacity without obscuring the underlying vessels; type II, GGO with consolidation, which was defined as an area of opacification obscuring the underlying vessels in the GGO; type III, GGO with interlobular septal thickening or reticulation, which was defined as a crazy-paving sign; and type IV, consolidation. The number of pulmonary segments involved was counted. If the number of inferior lobe segments involved was more than half of the total involved segments, this was defined as inferior lobe preference. The outer one-third of the lung was defined as peripheral, and the rest was defined as central.
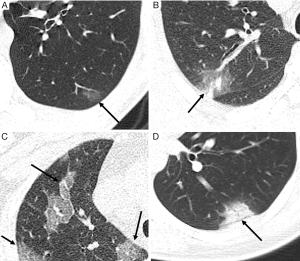
Air bronchograms, focal pulmonary fibrosis (including reticulation and liner opacity), pleural effusion, and mediastinal lymphadenopathy (>1 cm in short-axis diameter) were also noted. All the terms were defined according to the Fleischner Society (17). The images were analysed independently by two radiologists (Weiya Shi and Fei Shan, with 12 and 19 years of experience in chest radiology, respectively). In cases of disagreement, the results were determined by consensus.
Quantitative CT analysis
The infected regions on CT were quantified by uAI-Discover-NCP (beta version) developed by Shanghai United Imaging Intelligence Inc., China. The software utilized the V-shaped neural network (V-Net) (Figure 2), which is a convolutional neural network (CNN) (18), and combined it with transfer learning to segment the lung fields and infected regions on images. The procedure was carried out in two steps. In the training step, the lung fields and infected regions were manually annotated by senior radiologists. These data served as training data for the neural network model. In the application step, the trained model was used to automatically extract lung fields and infected regions from new chest CT images, and the volume of the infected regions can be calculated.
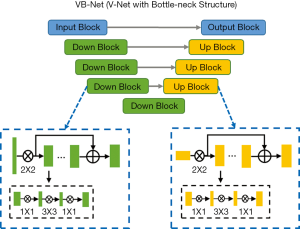
The infected regions were mainly composed of GGOs and consolidations. An opacity observed within the range of −750 to −300 HU was defined as a GGO, and that observed within the range of −300 to 50 HU was defined as consolidation (19). The percentage of infection (POICT) was calculated as the infected volume divided by the whole lung volume. The percentage of consolidation was calculated as the volume of consolidation divided by the whole infected volume. The mass of infection (MOICT) in the whole lung was also included in the quantitative analysis. Mass was calculated according to the following equation (20): M = V * [(Amean+1000) * 0.001], where M was the mass in milligrams per millimetre, V was the infected volume, and Amean was the mean attenuation in Hounsfield units.
Statistical analysis
The continuous data are expressed as the median and interquartile range (IQR, 25th and 75th percentiles) because a majority of the data did not follow a normal distribution. The Wilcoxon rank-sum test and Fisher’s exact test were used to compare quantitative and categorical variables, respectively, between the two groups.
Variables found to be significant in univariate analysis were inputted into the least absolute shrinkage and selection operator (LASSO) logistic regression analysis to determine the optimal subset of clinico-radiological features for prediction. By incorporating these significant predictors, a nomogram model was established by five-fold cross-validation. The nomogram was calibrated by performing a calibration curve analysis. Receiver operating characteristic (ROC) analyses were conducted, and the areas under the receiver operating characteristic curve (AUCs) of the nomogram model, quantitative CT parameters that were significant in univariate analysis, and PSI were compared using the Delong test to evaluate the effects of the classifier when comparing the severe group with the non-severe group. The cut-off values were defined based on the maximal Youden index.
Statistical analysis was performed with R version 3.6.1 (R Project for Statistical Computing, Vienna, Austria). The nomogram construction and calibration plots were performed using the “rms” package. A two-tailed α less than 0.05 was considered statistically significant.
Results
Clinical and laboratory features
The median age of the 196 enrolled patients was 52 years old (IQR, 38–65; range, 16–88), and 105 (53.6%) were men (Table 1). The median age of the severe group (45 patients) was older than that of the non-severe group (151 patients) (65 vs. 46 years, P<0.001). More non-severe patients had a history of exposure in an outbreak area than severe patients (102/151, 67.5% vs. 19/45, 42.2%, P=0.003). Comorbidities were more frequent in the severe group (25/45, 55.6% vs. 47/151, 31.1%, P=0.004), particularly cardiovascular disease (7/45, 15.6% vs. 6/151, 4.0%, P=0.012).
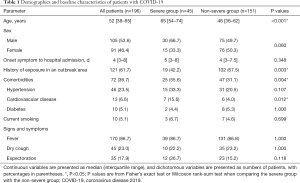
Full table
There were numerous differences in laboratory findings between the two groups (Table 2), including higher neutrophil count and higher serum levels of D-dimer, aspartate aminotransferase, lactate dehydrogenase (LDH), serum troponin I, serum myoglobin, procalcitonin, and C-reactive protein (CRP), as well as lower levels of lymphocyte count, cluster of differentiation 4 (CD4)+ T cell count, and albumin in the severe group (all P<0.001).
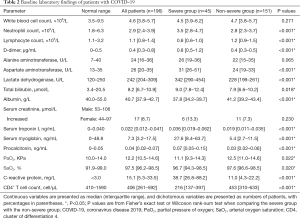
Full table
The severe group had a higher PSI than the non-severe group [66 points (IQR, 54–82) vs. 48 points (IQR, 35–61.5), P<0.001].
CT characteristics
The morphological CT features and quantitative CT characteristics of the 196 patients are shown in Table 3. The most common types of pulmonary opacities were type III (140/196, 71.4%) and type II (139/196, 70.9%). The lesions were distributed more peripherally (173/196, 88.3%) than centrally, usually with inferior lobe predominance (121/196, 61.7%). No significant differences were found in the frequency of opacities and the distribution preference in the inferior and peripheral parts of the lung between the two groups. More pulmonary segments were involved in the severe group [15 (IQR, 12–18) vs. 7 (IQR, 3–13.5), P<0.001]. Bilateral lung involvement, air bronchograms and pleural effusion were more frequent in the severe group (43/45, 95.6% vs. 121/151, 80.1%, P=0.012; 39/45, 86.7% vs. 105/151, 69.5%, P=0.022; 5/45, 11.1% vs. 4/151, 2.6%, P=0.031).
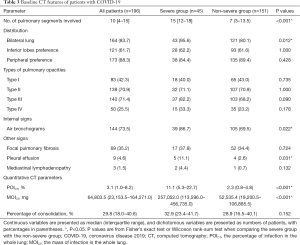
Full table
In terms of quantitative CT parameters, the POICT and MOICT were higher in the severe group [11.1% (IQR, 5.3–22.7%) vs. 2.3% (IQR, 0.8–4.8%), P<0.001; 257,052.0 mg (IQR, 113,596.0–456,735.0) vs. 52,535.4 mg (IQR, 19,200.5–106,885.5), P<0.001] (Figures 3,4). There was no significant difference in the percentage of consolidation between the two groups.
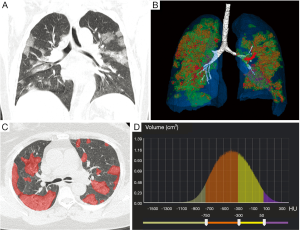
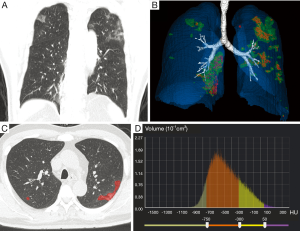
Comparison of ability to predict the severity of COVID-19
LASSO logistic regression analysis was applied to identify the most valuable clinico-radiological features when the optimal value of log(λ) was –2.582 according to 10-fold cross-validation. At this value, 24 clinico-radiological features were reduced to 5 potential predictors with non-zero coefficients (Figure 5).
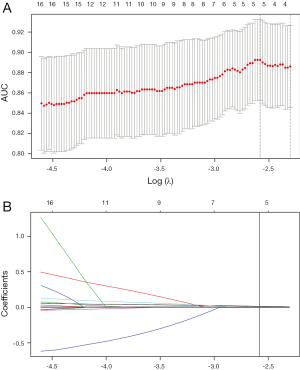
By incorporating the five clinico-radiological features including age, LDH, CRP, CD4+ T cell count, and MOICT, a nomogram model was constructed (Figure 6). The nomogram calibration plot showed predictive ability (Figure 7). The predicted results paralleled the real observations, almost exactly correlating with the 45-degree line on the graph.
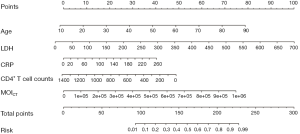
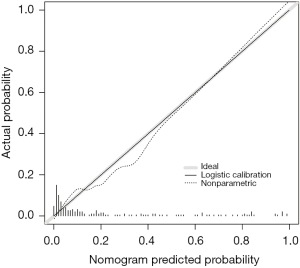
The abilities of the nomogram model, MOICT, POICT, and PSI for predicting the severity of COVID-19 were compared by the Delong test (Table 4). The AUC values of the nomogram model and the other three parameters were 0.900, 0.813, 0.805, and 0.751, respectively. When the cut-off value of the nomogram was 136.5 points, the sensitivity, specificity, and accuracy were 86.1%, 80.0%, and 84.7%, respectively, and the cut-off values for MOICT, POICT, and PSI were 107,720.0 mg, 5.9%, and 63.5 points, respectively. The nomogram model performed significantly better than MOICT, POICT, and PSI (P=0.003, P=0.001, and P<0.001, respectively) (Figure 8). There were no significant differences in AUCs among the other three parameters.
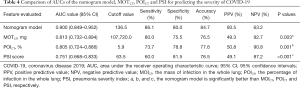
Full table
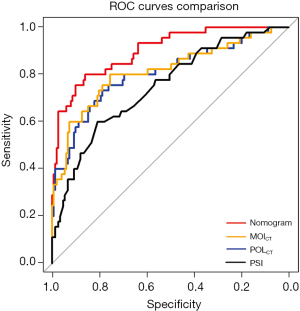
Discussion
The COVID-19 pandemic is a global public health issue threatening millions of lives worldwide. Here, we report the clinico-radiological characteristics of a cohort of 196 patients with COVID-19 admitted to our hospital in Shanghai, China. We compared the baseline clinico-radiological features between the severe and non-severe groups and determined whether a DL-based quantitative CT model can predict the severity of COVID-19.
Current research (21) suggests that some clinical presentations are unique to COVID-19, and are rarely observed in other respiratory viral infections, such as severe lymphopenia, extensive pneumonia with lung tissue damage, cytokine storms leading to ARDS and multiorgan failure. Elevated levels of acute phase reactants, such as interleukin-6 (22), CRP (22,23), tumour necrosis factor-α (24), and LDH (25), are early predictors of severe disease. In critically ill patients, a decrease in the absolute value of CD4+ T cells is correlated with the volume of lung lesions. In our cohort, there were also numerous differences in laboratory findings between the two groups, such as decreases in CD4+ T cells and increases in LDH and CRP in the severe group. These abnormal laboratory indexes can be used as predictors for the severity of COVID-19, which is consistent with current studies.
The PSI is an important score consisting of four parts: demographic, comorbidity, physical, and laboratory factors. This index can be used to evaluate community-acquired pneumonia, as well as viral pneumonia (16). In this study, the severe group had a higher PSI (P<0.001), suggesting that the PSI can be used to assess the severity of COVID-19. However, the scale does not assess CT findings, which may affect its accuracy.
It has been reported that the main CT findings include GGOs with or without consolidation or intralobular lines, with a predominantly peripheral distribution (26,27). Bilateral involvement and air bronchograms were more common in the severe group (P=0.012 and P=0.022, respectively), which may suggest a wider range of lesions in the severe group. Pleural effusion was more frequent in the severe group (P=0.031), consistent with previous studies that the presence of pleural effusion on CT suggests disease progression (28,29). Ichikado et al. analysed the pulmonary lesions of 44 patients with ARDS and found that their appearance on CT has prognostic value for predicting mortality, the number of days without mechanical ventilation, and the 28-day risk of barotrauma in patients with a clinically early stage of ARDS that developed from diverse causes (30). This result is consistent with other reports regarding prognoses of viral pneumonias such as H1N1, SARS, and MERS (10-12). In this study, more pulmonary segments were involved in the severe group (P<0.001), indicating that the extent of lesions on CT may reflect the severity of the disease, as reported in previous studies.
In recent years, the rapid development of AI has significantly improved automatic lung segmentation technology, making it possible to quantify lesions automatically. CNNs have been proposed as a potential solution to segmentation problems by identifying the lung boundary of patients with pulmonary diseases and distinguishing between lung tissue and surrounding structures (13,14). As CT images exhibit several prognostic and diagnostic characteristics of COVID-19, the rapid and precise quantification of these characteristics using AI has garnered considerable attentions. Recent studies (31-35) have reported promising results with the use of AI combined with CT imaging. In studies using deep learning for diagnosing COVID-19, U-shaped neural network (U-Net) and its modifications have often been used to segment the lung or lesions on CT images, and residual neural network (ResNet) (2D or 3D) has often been used for classification. In this study, V-Net-based automatic quantitative CT software was used to quantify the regions of infection. The results showed that the POICT was significantly higher in the severe group (P<0.001). This also proves that the extent of lesions is highly correlated with the severity of the disease. The MOICT was also significantly higher in the severe group (P<0.001). This parameter evaluates both extent and attenuation, making it one of the predictive model parameters.
In this study, the parsimonious model containing five features (age, LDH, CRP, CD4+ T cell count, and MOICT) was an ideal measure to predict the severity of COVID-19. LASSO logistic regression analysis not only works better than the conventional method of choosing predictors on the basis of the intensity of their univariate association with outcome, but it also allows researchers to combine the selected features into a model. The nomogram model performed significantly better than MOTCT, POICT, and PSI (P=0.003, P=0.001, and P<0.001, respectively). This is because the model combines DL-based quantifiable CT parameters of lesions with clinical laboratory indicators to comprehensively assess the severity of the disease, rather than as a partial assessment of each patient’s condition.
However, our study has several limitations. Firstly, serum samples were not obtained from patients to evaluate viremia. The viral load is a potentially useful marker associated with the severity of the disease. Secondly, although the nomogram model was established by cross-validation and had a good predictive performance, the proportion of patients with severe disease was relatively low, and there was an imbalance between the sizes of the severe and non-severe group, which may impact the statistical analysis. More data, especially from different geographic areas, are needed to validate the robustness of the model to further improve its prediction accuracy. Thirdly, drug administration during hospitalization was not compared, which may affect the progression of the disease.
Conclusions
Quantitative CT parameters and the PSI can well predict the severity of COVID-19. The DL-based quantitative CT model containing five clinico-radiological features can serve as a more efficient tool for prediction than individual quantitative CT parameters and PSI. External data are needed for further validation.
Acknowledgments
We sincerely thank Dr. Yuhong Tang from Winning Health Technology Group Co., Ltd for assistance with statistical analysis.
Funding: This work was supported by the Novel Coronavirus Special Research Foundation of Shanghai Science and Technology Commission (No. 20441900600).
Footnote
Reporting Checklist: The authors have completed the TRIPOD reporting checklist. Available at http://dx.doi.org/10.21037/atm-20-2464
Data Sharing Statement: Available at http://dx.doi.org/10.21037/atm-20-2464
Peer Review File: Available at http://dx.doi.org/10.21037/atm-20-2464
Conflicts of Interest: All authors have completed the ICMJE uniform disclosure form (available at http://dx.doi.org/10.21037/atm-20-2464). All authors report grants from Shanghai Science and Technology Commission, during the conduct of the study.
Ethical Statement: The authors are accountable for all aspects of the work in ensuring that questions related to the accuracy or integrity of any part of the work are appropriately investigated and resolved. The study was conducted in accordance with the Declaration of Helsinki (as revised in 2013). The Institutional Review Board of Shanghai Public Health Clinical Center, Fudan University approved this retrospective study (No. YJ-2020-S035-01), with a waiver of informed consent.
Open Access Statement: This is an Open Access article distributed in accordance with the Creative Commons Attribution-NonCommercial-NoDerivs 4.0 International License (CC BY-NC-ND 4.0), which permits the non-commercial replication and distribution of the article with the strict proviso that no changes or edits are made and the original work is properly cited (including links to both the formal publication through the relevant DOI and the license). See: https://creativecommons.org/licenses/by-nc-nd/4.0/.
References
- World Health Organization. Coronavirus disease 2019 (COVID-19) Situation Report-180. Available online: https://www.who.int/docs/default-source/coronaviruse/situation-reports/20200718-covid-19-sitrep-180.pdf?sfvrsn=39b31718_2. Data as reported by 10AM CET 18 July 2020. Accessed 18 July, 2020.
- World Health Organization. 2019-nCoV outbreak is an emergency of international concern. Available online: http://www.euro.who.int/en/health-topics/health-emergencies/international-health-regulations/news/news/2020/2/2019-ncov-outbreak-is-an-emergency-of-international-concern. Published 31 January, 2020. Acessed 24 February, 2020.
- Schwartz DA, Graham AL. Potential Maternal and Infant Outcomes from (Wuhan) Coronavirus 2019-nCoV Infecting Pregnant Women: Lessons from SARS, MERS, and Other Human Coronavirus Infections. Viruses 2020;12:194. [Crossref] [PubMed]
- Coleman CM, Sisk JM, Mingo RM, et al. Abelson Kinase Inhibitors Are Potent Inhibitors of Severe Acute Respiratory Syndrome Coronavirus and Middle East Respiratory Syndrome Coronavirus Fusion. J Virol 2016;90:8924-33. [Crossref] [PubMed]
- Chan JF, Yuan S, Kok KH, et al. A familial cluster of pneumonia associated with the 2019 novel coronavirus indicating person-to-person transmission: a study of a family cluster. Lancet 2020;395:514-23. [Crossref] [PubMed]
- Wang D, Hu B, Hu C, et al. Clinical Characteristics of 138 Hospitalized Patients With 2019 Novel Coronavirus-Infected Pneumonia in Wuhan, China. JAMA 2020;323:1061-9. [Crossref] [PubMed]
- Huang C, Wang Y, Li X, et al. Clinical features of patients infected with 2019 novel coronavirus in Wuhan, China. Lancet 2020;395:497-506. [Crossref] [PubMed]
- Chen N, Zhou M, Dong X, et al. Epidemiological and clinical characteristics of 99 cases of 2019 novel coronavirus pneumonia in Wuhan, China: a descriptive study. Lancet 2020;395:507-13. [Crossref] [PubMed]
- Shi H, Han X, Jiang N, et al. Radiological findings from 81 patients with COVID-19 pneumonia in Wuhan, China: a descriptive study. Lancet Infect Dis 2020;20:425-34. [Crossref] [PubMed]
- Wong KT, Antonio GE, Hui DS, et al. Thin-section CT of severe acute respiratory syndrome: evaluation of 73 patients exposed to or with the disease. Radiology 2003;228:395-400. [Crossref] [PubMed]
- Kang H, Lee KS, Jeong YJ, et al. Computed tomography findings of influenza A (H1N1) pneumonia in adults: pattern analysis and prognostic comparisons. J Comput Assist Tomogr 2012;36:285-90. [Crossref] [PubMed]
- Das KM, Lee EY, Enani MA, et al. CT correlation with outcomes in 15 patients with acute Middle East respiratory syndrome coronavirus. AJR Am J Roentgenol 2015;204:736-42. [Crossref] [PubMed]
- Pang T, Guo S, Zhang X, et al. Automatic Lung Segmentation Based on Texture and Deep Features of HRCT Images with Interstitial Lung Disease. Biomed Res Int 2019;2019:2045432. [Crossref] [PubMed]
- Park B, Park H, Lee SM, et al. Lung Segmentation on HRCT and Volumetric CT for Diffuse Interstitial Lung Disease Using Deep Convolutional Neural Networks. J Digit Imaging 2019;32:1019-26. [Crossref] [PubMed]
- China National Health Commission. Diagnosis and treatment of pneumonitis caused by new coronavirus (trial version 5). Available online: http://www.nhc.gov.cn/yzygj/s7653p/202002/3b09b894ac9b4204a79db5b8912d4440.shtml. Updated 5 February, 2020. Accessed 5 February, 2020.
- Kao KC, Chang KW, Chan MC, et al. Predictors of survival in patients with influenza pneumonia-related severe acute respiratory distress syndrome treated with prone positioning. Ann Intensive Care 2018;8:94. [Crossref] [PubMed]
- Hansell DM, Bankier AA, MacMahon H, et al. Fleischner Society: glossary of terms for thoracic imaging. Radiology 2008;246:697-722. [Crossref] [PubMed]
- Zhang L, Zhang J, Shen P, et al. Block Level Skip Connections Across Cascaded V-Net for Multi-Organ Segmentation. IEEE Trans Med Imaging 2020;39:2782-93. [Crossref] [PubMed]
- Jacobs C, van Rikxoort EM, Twellmann T, et al. Automatic detection of subsolid pulmonary nodules in thoracic computed tomography images. Med Image Anal 2014;18:374-84. [Crossref] [PubMed]
- Song YS, Park CM, Park SJ, et al. Volume and mass doubling times of persistent pulmonary subsolid nodules detected in patients without known malignancy. Radiology 2014;273:276-84. [Crossref] [PubMed]
- Azkur AK, Akdis M, Azkur D, et al. Immune response to SARS-CoV-2 and mechanisms of immunopathological changes in COVID-19. Allergy 2020;75:1564-81. [Crossref] [PubMed]
- Liu F, Li L, Xu M, et al. Prognostic value of interleukin-6, C-reactive protein, and procalcitonin in patients with COVID-19. J Clin Virol 2020;127:104370. [Crossref] [PubMed]
- Chen W, Zheng KI, Liu S, et al. Plasma CRP level is positively associated with the severity of COVID-19. Ann Clin Microbiol Antimicrob 2020;19:18. [Crossref] [PubMed]
- Chen XY, Yan BX, Man XY. TNFalpha inhibitor may be effective for severe COVID-19: learning from toxic epidermal necrolysis. Ther Adv Respir Dis 2020;14:1753466620926800. [Crossref] [PubMed]
- Wu MY, Yao L, Wang Y, et al. Clinical evaluation of potential usefulness of serum lactate dehydrogenase (LDH) in 2019 novel coronavirus (COVID-19) pneumonia. Respir Res 2020;21:171. [Crossref] [PubMed]
- Chung M, Bernheim A, Mei X, et al. CT Imaging Features of 2019 Novel Coronavirus (2019-nCoV). Radiology 2020;295:202-7. [Crossref] [PubMed]
- Song F, Shi N, Shan F, et al. Emerging 2019 Novel Coronavirus (2019-nCoV) Pneumonia. Radiology 2020;295:210-7. [Crossref] [PubMed]
- Yuan Y, Tao XF, Shi YX, et al. Initial HRCT findings of novel influenza A (H1N1) infection. Influenza Other Respir Viruses 2012;6:e114-9. [Crossref] [PubMed]
- Qureshi NR, Hien TT, Farrar J, et al. The radiologic manifestations of H5N1 avian influenza. J Thorac Imaging 2006;21:259-64. [Crossref] [PubMed]
- Ichikado K, Suga M, Muranaka H, et al. Prediction of prognosis for acute respiratory distress syndrome with thin-section CT: validation in 44 cases. Radiology 2006;238:321-9. [Crossref] [PubMed]
- Ko H, Chung H, Kang WS, et al. COVID-19 Pneumonia Diagnosis Using a Simple 2D Deep Learning Framework With a Single Chest CT Image: Model Development and Validation. J Med Internet Res 2020;22:e19569. [Crossref] [PubMed]
- Li L, Qin L, Xu Z, et al. Using Artificial Intelligence to Detect COVID-19 and Community-acquired Pneumonia Based on Pulmonary CT: Evaluation of the Diagnostic Accuracy. Radiology 2020;296:E65-E71. [Crossref] [PubMed]
- Ni Q, Sun ZY, Qi L, et al. A deep learning approach to characterize 2019 coronavirus disease (COVID-19) pneumonia in chest CT images. Eur Radiol 2020;30:6517-27. [Crossref] [PubMed]
- Wang S, Zha Y, Li W, et al. A fully automatic deep learning system for COVID-19 diagnostic and prognostic analysis. Eur Respir J 2020;56:2000775. [Crossref] [PubMed]
- Zhang K, Liu X, Shen J, et al. Clinically Applicable AI System for Accurate Diagnosis, Quantitative Measurements, and Prognosis of COVID-19 Pneumonia Using Computed Tomography. Cell 2020;181:1423-33.e11. [Crossref] [PubMed]