Heart rate fluctuation predicts mortality in critically ill patients in the intensive care unit: a retrospective cohort study
Introduction
Elevated resting heart rate (HR) has been widely associated with mortality across a broad spectrum of diseases (1-3). Circadian rhythms, which drive the sleep-wake cycle, are involved in the physiological process of HR variation to adapt to the external environment (4). Except sleep-wake cycle, demographic factors, such as age and sex, and lifestyles, such as physical activity, alcohol and smoking, were also showed to be involved in the physiological process of HR variation (5). Recently, HR variability has been the focus of increased attention. In particular, decreased HR variability, which is associated with impaired automatic nervous function, has been shown to predict the risk of cardiovascular and all-cause mortality in community-based populations (6,7). In the intensive care unit (ICU), the circadian rhythms of critically ill patients are more easily disrupted by altered light-dark cycles and a different external environment (8). HR fluctuation between the maximum and minimum HR in a 24-hour period is an accessible marker representing circadian changes in HR. In the ICU, it was reported that several heart rate dynamics, including mean HR and its fluctuation, could be used to predict transfusion requirement (9). While the association between HR fluctuation and mortality in the ICU remains unknown. Several circadian variation factors, such as blood pressure and blood glucose level, were demonstrated to be associated with mortality (10,11). Thus, we hypothesized that disrupted HR fluctuation, as one of the accessible circadian variation factors, may also have predictive value for prognosis in ICU patients.
A U-shaped relationship between higher mortality and both high HR and low HR was reported in a hypertensive cohort, indicating that HR above or below a suitable level may suggest poor prognosis (12). Adequate HR control has been shown to be a beneficial treatment for fast HR disorders (13). However, unlike mean HR control, evidence for recommendations to control HR fluctuation to a target range remains limited.
In this study, we aimed to elucidate the association between HR fluctuation and risk of mortality, as well as the appropriate HR fluctuation target with lowest risk of death in the ICU. We designed and conducted this retrospective cohort study by using multivariate Cox proportional hazards regression modeling and restricted cubic spline modeling based on the Medical Information Mart for Intensive Care (MIMIC-III) database. Briefly, MIMIC-III is a large, public, and free database comprising deidentified health-related records associated with more than 30,000 patients who stayed in ICU of the Beth Israel Deaconess Medical Center between 2001 and 2012 (14). Based on the MIMIC-III database, a diverse range of analytic studies spanning epidemiology, clinical decision improvement, and electronic tool development were carried out. Our study may be helpful for providing novel evidence to optimize HR control strategies.
We present the following article in accordance with the STROBE reporting checklist (available at http://dx.doi.org/10.21037/atm-20-7897).
Methods
Data source
The data for this retrospective cohort study were extracted from the MIMIC-III database. The MIMIC-III database consists of comprehensive and de-identified clinical data from 53,423 distinct ICU stays for 38,597 adult patients during the period of 2001 to 2012. A relational database was established with demographic data, laboratory tests, discharge summaries, electrocardiography, imaging examinations, diagnostic information such as the International Classification of Diseases, Ninth Revision (ICD-9) codes, and in-hospital and out-of-hospital mortality. The use of the MIMIC-III database was approved by the review boards of the Massachusetts Institute of Technology and Beth Israel Deaconess Medical Center (14). The study was conducted in accordance with the Declaration of Helsinki (as revised in 2013).
Study cohort
Structure Query Language was applied for data extraction (15). Study inclusion criteria were as follows: (I) age ≥18 years; (II) length of ICU stay >1 day; and (III) only the first ICU admission was included for patients with multiple ICU admissions. A total of 27,814 ICU stay records were extracted after exclusion of patients with censored age, no HR records, or no weight records (Figure 1). All characteristics were collected in the initial 24-hour documentation following admission. The following variables for further statistical analysis were included: (I) basic demographics, including age, sex, and weight; (II) HR, use of sedatives, ventilation, vasopressors, simplified acute physiology score (SAPS), sequential organ failure assessment (SOFA) score; and (III) comorbidities, including arrhythmia, congestive heart failure (CHF), diabetes, renal failure, liver disease, and metastatic cancer. Missing values were fulfilled with predictive values by random forest using miss Forest package in R (16).
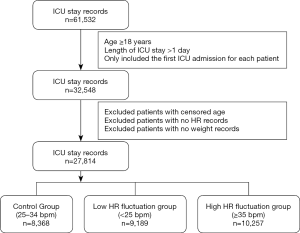
HR fluctuation and outcomes
HR was measured at least once per hour for each subject. HR fluctuation was calculated as the maximum HR minus the minimum HR in the initial 24 hours. According to the level of HR fluctuation, participants were divided into 3 groups: low HR fluctuation [<25 beats per minute (bpm)], control group (25–34 bpm), and high HR fluctuation (≥35 bpm). In the baseline characteristics analysis and Cox regression analysis, these cut-off values of HR were designed to guarantee that each group shared a relatively equal sample size. And in nonlinear association analysis, the target value of HR with lowest risk of mortality was evaluated by restricted cubic spline model. In this study, 28-day mortality and 1-year mortality were defined as the outcome events, which were also available in the MIMIC-III database.
Statistical analysis
Normally distributed continuous variables are presented as the mean ± standard deviation, while non-normally distributed data are presented as the median [interquartile range (IQR)]. Differences among continuous variables were tested using analysis of variance or the rank-sum test, as appropriate. Categorical variables are presented as a number (percentage) and were tested by the chi-square test. Cox regression models with backward process were used to investigate the association between HR fluctuation and outcomes. Adjusted variables included age, male sex, weight, SAPS score, SOFA score, mean HR, ventilation, vasopressors, sedatives, arrhythmias, CHF, hypertension, diabetes, renal failure, and liver disease. Hazard ratios and 95% confidence intervals (CI) for the high and low HR fluctuation groups were calculated and compared with the control group. An adjusted model using restricted cubic spline with 5 knots was constructed to flexibly represent the association between the risk of mortality and HR fluctuation as a continuous variable (17). Further sensitivity analyses were conducted to evaluate the influence of different mean HR or comorbidities on our results.
A two-tailed P value <0.05 was considered statistically significant in our study. SPSS software (version 23.0, IBM, NY, USA) and R (version 3.6.3, R Foundation for Statistical Computing, Vienna, Austria) were utilized for statistical analysis.
Results
Baseline characteristics of enrolled patients
The control group, low HR fluctuation group, and high HR fluctuation group comprised 8,368, 9,189, and 10,257 ICU patients, respectively. Patients in the high HR fluctuation group had the lowest age, ratio of males, and weight, as well as the highest SAPS score, HR fluctuation, and mean HR among the 3 groups. Meanwhile, the high HR fluctuation group showed a significantly higher exposure rate for ventilation, vasopressors, and sedatives compared to the low HR fluctuation and control groups. Additionally, the high HR fluctuation group was most likely to suffer arrhythmias and CHF among the 3 groups, while the low HR fluctuation group had a higher prevalence of hypertension, diabetes, renal failure, and liver disease. No significant difference was found in the prevalence of metastatic cancer among the 3 groups. Importantly, patients in the control group suffered the lowest 28-day and 1-year mortality among the 3 groups (both P<0.001) (Table 1).
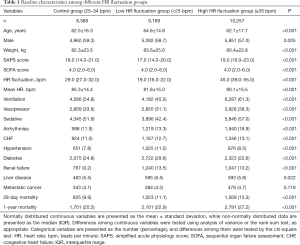
Full table
Association between HR fluctuation and mortality
Compared to the control group, both the high and low HR fluctuation groups were associated with a higher risk of 28-day mortality and 1-year mortality in the crude model (each P<0.05). Even after adjusting for age, sex, weight, SAPS score, SOFA score, mean HR, ventilation, vasopressors, sedatives, arrhythmias, CHF, hypertension, diabetes, renal failure, and liver disease, the higher risk of 28-day mortality and 1-year mortality remained significant in the high HR fluctuation group, with hazard ratios of 1.210 (95% CI, 1.103–1.327) and 1.150 (95% CI, 1.078–1.227), respectively, as well as in the low HR fluctuation group, with hazard ratios of 1.130 (95% CI, 1.035–1.232) and 1.087 (95% CI, 1.022–1.157), respectively (Table 2).
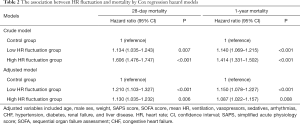
Full table
The survival curves consistently showed that the high HR fluctuation group was significantly associated with the highest risk of 28-day and 1-year mortality, followed by the low HR fluctuation group and control group (log rank P<0.001) (Figure 2).
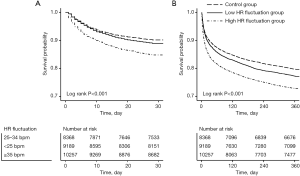
Moreover, a typical U-type curve was also observed in restricted cubic splines, representing a nonlinear association between HR fluctuation and 28-day and 1-year mortality, with an HR fluctuation of 30 bpm conveying the lowest risk (Figure 3).
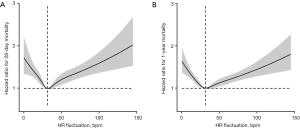
Sensitivity analyses
To evaluate the influence of different mean HR or comorbidities on mortality, all patients were divided into subgroups for further sensitivity analysis. For 28-day mortality, the association between HR fluctuation and mortality remained significant in a subgroup with mean HR ≥85 bpm, acute kidney injury, or sedative use (each P<0.05) but not in a subgroup with hypertension or arrhythmias (Figure 4). For 1-year mortality, the influence of HR fluctuation on mortality was weakened but remained significant in a subgroup with sedative use (P<0.05) (Figure 5).
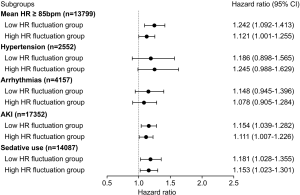
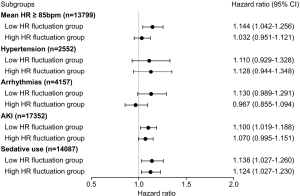
Discussion
This retrospective cohort study included 27,814 ICU patient records, which were divided into a control group, low HR fluctuation group, and high HR fluctuation group based on different HR fluctuation levels during the first 24 hours following admission. In brief, we found that both 28-day mortality and 1-year mortality were significantly increased in the high HR fluctuation group and low HR fluctuation group when compared with the control group. These results were also reflected by the survival curves. Importantly, there was a typical U-type curve in the restricted cubic splines, depicting a nonlinear association between HR fluctuation and 28-day and 1-year mortality, with the lowest risk at an HR fluctuation of 30 bpm. To the best of our knowledge, this is the first retrospective cohort study to clearly reveal that daily HR fluctuation could predict prognosis in ICU patients, highlighting the importance of daily HR fluctuation control.
Daily HR variation, similar to blood pressure variation, is determined by neural regulation for systemic adaption to the external and internal environment (18). Patients admitted to the ICU are commonly exposed to disordered rhythmic light-dark cycles, sedative use, and other conditions that largely differ from the normal environment, resulting in impaired physiological homeostasis and circadian rhythm disorders (8,19). Our results showed more common usage of ventilation, vasopressors, and sedatives in the high HR fluctuation group compared to the low HR fluctuation and control groups, indicating severe illness in the high HR fluctuation group. Patients with high HR fluctuation were more likely to suffer arrhythmias and CHF, while the highest prevalence of diabetes, hypertension, renal failure, and liver disease occurred in the low HR fluctuation group. In a community population, it was reported that HR in 24 hours was 75.8±9.4 bpm, with a fluctuation of nearly 18.8 bpm (20). And in a CHF patients’ population, average HR fluctuation was 47 bpm (IQR, 36–59) (21). These results support the notion that HR fluctuation might be associated with different diseases or severity of illness, and marked heterogeneity existed among the different HR fluctuation groups.
Previous cohort studies have concluded that increased mean HR upon admission was independently associated with elevated all-cause mortality and in-hospital mortality in the general population and among patients with acute coronary syndrome (3,22). Furthermore, beat-to-beat HR variability can also predict sudden cardiac death and all-cause mortality (23-25). The risk of cardiac mortality for a low standard deviation of normal-to-normal RR intervals was significantly elevated in both young adults and the elderly (24). Usual leisure activities and walking helped improve specific HR variability indices, indicating enhanced autonomic function in older adults, including normalized circadian fluctuations (26). Combined with our results, these findings stress that appropriate HR fluctuation should be fully considered in HR control strategies for ICU patients for better prognosis. Due to the high risk of mortality for patients with high HR fluctuation, active treatment of primary disease was well warranted. Besides, close vital sign monitoring and strategies for choosing different times of the day at which to perform some medical procedures or drugs might also be beneficial to these patients (27).
Increased resting HR has been widely validated to be linearly associated with a higher risk of all-cause mortality in the general population and among patients with hypertension (28-30). Interestingly, a U-type curve was fitted by restricted cubic splines in our study, which revealed a nonlinear association between HR fluctuation and the risk of 28-day or 1-year mortality, with the lowest risk at an HR fluctuation of 30 bpm. Current HR control is effective in the treatment of fast HR disorders, including atrial fibrillation and CHF, with an appropriate HR target of approximately 80 bpm for minimizing the risk of cardiovascular events (13,31,32). Our results provide a critical point for optimizing HR control by targeting 30 bpm for proper HR fluctuation control, which could be helpful to maintain stable HR circadian rhythms and reduce the risk of all-cause mortality in critically ill patients.
There were some limitations to this study. First, since this was a real-world data-based retrospective study in which different intervals between HR monitoring measurements for each patient might have existed, we calculated the HR fluctuation between the maximum and minimum HR across the initial 24 hours, with further adjustment for multiple covariates in the regression models to reduce their potential impact on the risk of mortality. Second, there was inevitable selection bias from the retrospective study design. Thus, future external validation will help enhance the credibility of our results. Third, sensitivity analyses showed that the association between HR fluctuation and mortality was impaired in the subgroup with hypertension or arrhythmia. These results might be due to the small sample sizes of these subgroups, as well as the HR-limiting therapy in these patients, which could influence the level of HR fluctuation.
Conclusions
In conclusion, this retrospective cohort study revealed an independent association between HR fluctuation and 28-day and 1-year mortality in ICU patients, with the lowest risk associated with an HR fluctuation of 30 bpm. HR fluctuation might be an accessible and reliable predictor for the risk of mortality in critically ill patients, which should be considered and further validated in future studies on HR control strategies.
Acknowledgments
We would like to thank the participants, developers and investigators associated with the Medical Information Mart for Intensive Care (MIMIC)-III database.
Funding: This study was funded by grants from the National Natural Science Foundation of China (Grant No. 81870170, 81970388, 82070237), the Guangdong Basic and Applied Basic Research Found (Grant No. 2019A1515011682), the Guangzhou Regenerative Medicine and Health Guangdong Laboratory (Grant No. 2019GZR110406004), the Guangzhou Science and Technology Plan Project (Grant No. 201704020044, 201803040010) and Yat-sen Start-up Foundation (No. YXQH202014).
Footnote
Reporting Checklist: The authors have completed the STROBE reporting checklist. Available at http://dx.doi.org/10.21037/atm-20-7897
Conflicts of Interest: All authors have completed the ICMJE uniform disclosure form (available at http://dx.doi.org/10.21037/atm-20-7897). The authors have no conflicts of interest to declare.
Ethical Statement: The authors are accountable for all aspects of the work in ensuring that questions related to the accuracy or integrity of any part of the work are appropriately investigated and resolved. The application of the MIMIC-III database was approved by the review boards of the Massachusetts Institute of Technology and Beth Israel Deaconess Medical Center. The study was conducted in accordance with the Declaration of Helsinki (as revised in 2013).
Open Access Statement: This is an Open Access article distributed in accordance with the Creative Commons Attribution-NonCommercial-NoDerivs 4.0 International License (CC BY-NC-ND 4.0), which permits the non-commercial replication and distribution of the article with the strict proviso that no changes or edits are made and the original work is properly cited (including links to both the formal publication through the relevant DOI and the license). See: https://creativecommons.org/licenses/by-nc-nd/4.0/.
References
- Kannel WB, Kannel C, Paffenbarger RS Jr, et al. Heart rate and cardiovascular mortality: the Framingham Study. Am Heart J 1987;113:1489-94. [Crossref] [PubMed]
- Mensink GB, Hoffmeister H. The relationship between resting heart rate and all-cause, cardiovascular and cancer mortality. Eur Heart J 1997;18:1404-10. [Crossref] [PubMed]
- Reunanen A, Karjalainen J, Ristola P, et al. Heart rate and mortality. J Intern Med 2000;247:231-9. [Crossref] [PubMed]
- Rana S, Prabhu SD, Young ME. Chronobiological Influence Over Cardiovascular Function: The Good, the Bad, and the Ugly. Circ Res 2020;126:258-79. [Crossref] [PubMed]
- Tegegne BS, Man T, van Roon AM, et al. Determinants of heart rate variability in the general population: The Lifelines Cohort Study. Heart rhythm 2018;15:1552-8. [Crossref] [PubMed]
- Floyd JS, Sitlani CM, Wiggins KL, et al. Variation in resting heart rate over 4 years and the risks of myocardial infarction and death among older adults. Heart 2015;101:132-8. [Crossref] [PubMed]
- Jensen MT, Suadicani P, Hein HO, et al. Elevated resting heart rate, physical fitness and all-cause mortality: a 16-year follow-up in the Copenhagen Male Study. Heart 2013;99:882-7. [Crossref] [PubMed]
- Dessap AM, Roche-Campo F, Launay JM, et al. Delirium and Circadian Rhythm of Melatonin During Weaning From Mechanical Ventilation: An Ancillary Study of a Weaning Trial. Chest 2015;148:1231-41. [Crossref] [PubMed]
- Moss TJ, Clark MT, Lake DE, et al. Heart rate dynamics preceding hemorrhage in the intensive care unit. J Electrocardiol 2015;48:1075-80. [Crossref] [PubMed]
- Mendez CE, Mok KT, Ata A, et al. Increased glycemic variability is independently associated with length of stay and mortality in noncritically ill hospitalized patients. Diabetes Care 2013;36:4091-7. [Crossref] [PubMed]
- Gao Y, Wang Q, Li J, et al. Impact of Mean Arterial Pressure Fluctuation on Mortality in Critically Ill Patients. Critical care medicine 2018;46:e1167-74. [Crossref] [PubMed]
- Salles GF, Cardoso CR, Fonseca LL, et al. Prognostic significance of baseline heart rate and its interaction with beta-blocker use in resistant hypertension: a cohort study. Am J Hypertens 2013;26:218-26. [Crossref] [PubMed]
- Okumura T, Kimura Y, Murohara T. Heart rate control using beta-blockers for heart failure with atrial fibrillation: more than enough is too much. Hypertens Res 2019;42:1826-7. [Crossref] [PubMed]
- Johnson AEW, Pollard TJ, Shen L, et al. MIMIC-III, a freely accessible critical care database. Scientific data 2016;3:160035. [Crossref] [PubMed]
- Johnson AE, Stone DJ, Celi LA, et al. The MIMIC Code Repository: enabling reproducibility in critical care research. J Am Med Inform Assoc 2018;25:32-9. [Crossref] [PubMed]
- Stekhoven DJ, Bühlmann P. MissForest--non-parametric missing value imputation for mixed-type data. Bioinformatics 2012;28:112-8. [Crossref] [PubMed]
- Ng R, Kornas K, Sutradhar R, et al. The current application of the Royston-Parmar model for prognostic modeling in health research: a scoping review. Diagn Progn Res 2018;2:4. [Crossref] [PubMed]
- Guyton AC. Blood pressure control--special role of the kidneys and body fluids. Science 1991;252:1813-6. [Crossref] [PubMed]
- Oldham M, Pisani MA. Sedation in critically ill patients. Crit Care Clin 2015;31:563-87. [Crossref] [PubMed]
- Johansen CD, Olsen RH, Pedersen LR, et al. Resting, night-time, and 24 h heart rate as markers of cardiovascular risk in middle-aged and elderly men and women with no apparent heart disease. Eur Heart J 2013;34:1732-9. [Crossref] [PubMed]
- Cubbon RM, Ruff N, Groves D, et al. Ambulatory heart rate range predicts mode-specific mortality and hospitalisation in chronic heart failure. Heart (British Cardiac Society) 2016;102:223-9. [Crossref] [PubMed]
- Jensen MT, Pereira M, Araujo C, et al. Heart rate at admission is a predictor of in-hospital mortality in patients with acute coronary syndromes: Results from 58 European hospitals: The European Hospital Benchmarking by Outcomes in acute coronary syndrome Processes study. Eur Heart J Acute Cardiovasc Care 2018;7:149-57. [Crossref] [PubMed]
- Sessa F, Anna V, Messina G, et al. Heart rate variability as predictive factor for sudden cardiac death. Aging (Albany NY) 2018;10:166-77. [Crossref] [PubMed]
- La Rovere MT, Bigger JT Jr, Marcus FI, et al. Baroreflex sensitivity and heart-rate variability in prediction of total cardiac mortality after myocardial infarction. ATRAMI (Autonomic Tone and Reflexes After Myocardial Infarction) Investigators. Lancet 1998;351:478-84. [Crossref] [PubMed]
- Gavish B, Bursztyn M. Blood pressure and heart period variability ratios derived from 24-h ambulatory measurements are predictors of all-cause mortality. J Hypertens 2015;33:491-8; discussion 498. [Crossref] [PubMed]
- Soares-Miranda L, Sattelmair J, Chaves P, et al. Physical activity and heart rate variability in older adults: the Cardiovascular Health Study. Circulation 2014;129:2100-10. [Crossref] [PubMed]
- Crnko S, Du Pré BC, Sluijter JPG, et al. Circadian rhythms and the molecular clock in cardiovascular biology and disease. Nat Rev Cardiol 2019;16:437-47. [Crossref] [PubMed]
- Wang T, Zhang W, Zhang M, et al. Higher heart rates increase risk of diabetes and cardiovascular events: A prospective cohort study among Inner Mongolians. Diabetes Metab 2020;46:20-6. [Crossref] [PubMed]
- Zhao MX, Zhao Q, Zheng M, et al. Effect of resting heart rate on the risk of all-cause death in Chinese patients with hypertension: analysis of the Kailuan follow-up study. BMJ Open 2020;10:e032699. [Crossref] [PubMed]
- Zhang D, Shen X, Qi X. Resting heart rate and all-cause and cardiovascular mortality in the general population: a meta-analysis. CMAJ 2016;188:E53-E63. [Crossref] [PubMed]
- Gillinov AM, Bagiella E, Moskowitz AJ, et al. Rate Control versus Rhythm Control for Atrial Fibrillation after Cardiac Surgery. N Engl J Med 2016;374:1911-21. [Crossref] [PubMed]
- Docherty KF, Shen L, Castagno D, et al. Relationship between heart rate and outcomes in patients in sinus rhythm or atrial fibrillation with heart failure and reduced ejection fraction. Eur J Heart Fail 2020;22:528-38. [Crossref] [PubMed]
(English Language Editor: C. Betlazar-Maseh)