Identification of drug compounds for keloids and hypertrophic scars: drug discovery based on text mining and DeepPurpose
Introduction
Keloids (KL) and hypertrophic scars (HS) are fibroproliferative disorders caused by abnormal wound healing following dermal injury. These scars form due to fibroblast proliferation and are characterized by excessive collagen accumulation (1). There is great variation in the epidemiology of KL and HS depending on the study population; for instance, the incidence among African and Hispanic populations ranges from 4.5–16%, compared with only 0.09% in England (2). Aside from the unpleasant symptoms of HS, such as itching, pain, erythema, and functional damage, its unsightly appearance can cause psychological pain for patients, affecting their quality of life (3).
Currently, treatments for KL and HS include drug injections, surgical excision, laser therapy, radiotherapy, pressure therapy, and cryotherapy. However, corticosteroid injections can produce side effects such as skin atrophy and telangiectasia. Furthermore, the rate of recurrence among keloid patients treated with surgical excision combined with radiotherapy has been reported to be 21%, with none in craniofacial locations (4). Other therapies may also cause side effects and have unsatisfactory effectiveness (5). However, the molecular mechanism underlying scar formation still needs to be elucidated, and successful treatment of KL and HS remains a challenge.
It takes more than 10 years to discover and develop a new drug, at an average cost exceeding 2.6 billion US dollars. However, new therapeutic purposes for existing drugs may be discovered through drug repositioning (6,7). Drug-target interactions (DTIs) measure the binding affinity of drug molecules to protein targets (8). Therefore, computational methods that can obtain knowledge about the interaction between compounds and target proteins are important in drug research and discovery (R&D). Computer simulation methods can speed up the drug research and development process by systematically prioritizing the most effective compounds. Recently, deep learning (DL) technology has been demonstrated to have the potential to predict compound–protein interactions on a large scale by learning from limited data, and it has been successfully applied in the R&D of new drugs, in which it significantly shortened the time and cost (9,10).
Our previous studies demonstrated that drug discovery using in silico text mining and pathway analysis tools may be a method to explore candidate drugs targeting the genes and pathways associated with certain diseases. In this study, we utilized DeepPurpose, a powerful Python toolkit, which presented the most likely drug candidates based on our previous work. DeepPurpose processes the input target amino acid sequences and candidate drug codes by feeding the data into multiple latest deep learning models pre-trained on DAVIS, BindingDB-Kd, and kinase inhibitor bioactivity (KIBA) datasets (11-13). The prediction results are then integrated by DeepPurpose to generate a ranked list, with the drug candidates with the highest predicted binding scores positioned at the top. The top-ranked drug candidates are considered to possess the potential for experimental verification.
DeepPurpose presents the DTI model as an encoder-decoder framework to predict drug-target interactions. Taking the simplified molecular-input line-entry system (SMILES) format of the drug and the target amino acid sequence pair as input, DeepPurpose outputs the score of the binding affinity between the drug and the molecule. For drug molecules, DeepPurpose provides 8 encoders: Morgan, PubChem, Daylight, RDKit 2D, convolutional neural network (CNN), convolutional recurrent neural network (CNN+RNN), Transformer encoders, and Message-Passing Neural Network (MPNN). For protein targets, DeepPurpose provides 7 encoders: amino acid composition (AAC), PseACC, Conjoint Triad, Quasi Sequence, CNN, CNN+RNN, and Transformer (14).
In this study, we investigated new drug therapies for KL and HS by employing computational methods. First, we performed text mining, biological process and pathway analysis, and protein-protein interaction (PPI) analysis to explore the target genes and pathways highly relevant to KL and HS. DTI analysis was then performed to obtain candidate drugs. Finally, DeepPurpose was used to predict the interaction of candidate drugs and gene targets, and the drugs with the highest predicted binding affinity from a ranked list were obtained.
We present the following article in accordance with the MDAR checklist (available at http://dx.doi.org/10.21037/atm-21-218).
Methods
Text mining
In this study, text mining, a process in which high-quality information is derived from biological literature, was performed using pubmed2ensembl (http://pubmed2ensembl.ls.manchester.ac.uk/) (15). The following terms were used as search input: “keloid”, “hypertrophic scar”, “hyperplastic scar”, and “scar hypertrophy”. We chose “Homo sapiens” as the species dataset, then selected “Ensembl Gene ID” and “Associated Gene Name” under GENE. “Search for PubMed IDs” and “filter on Entrez: PMID” drop-down menus were chosen in the search of every query. The intersection of the 4 derived gene lists was used for the next step. The study was conducted in accordance with the Declaration of Helsinki (as revised in 2013).
Biological process and pathway analysis
GeneCodis (http://genecodis.cnb.csic.es/) was used to perform enrichment analysis on genes closely related to KL and HS (16). First, the genes identified through text mining were subjected to Gene Ontology (GO) biological process analysis. The most significantly enriched genes in biological processes were selected for Kyoto Encyclopedia of Genes and Genomes (KEGG) pathway analysis. The most significantly enriched KEGG pathways were selected, and genes associated with the selected pathways were used for further analysis.
Protein-protein interaction network
We used the STRING (Search Tool for the Retrieval of Interacting Genes/Proteins) database (http://string-db.org) to construct a protein-protein interaction (PPI) network in order to visualize the genes from the previous step (17). The genes were input under the “Multiple proteins” menu, and “Homo sapiens” was selected as the species dataset. To obtain the genes with strong interactions, we set a high confidence score of 0.700, and the PPI network of the target genes was generated. Then, the CentiScape plugin in Cytoscape was used to determine the centrality parameters of the PPI network (18). “Degree” and “Betweenness” were chosen as the parameters for the selection of key genes in this study. Degree represents the total number of edges incident to the node, and betweenness refers to the number of shortest paths through the node.
Drug-gene interactions
Drugs targeting the genes highly related to KL and HS were searched for using Pharmaprojects (https://pharmaintelligence.informa.com/) (19). Each gene query returned a drug list detailing the global status, disease, mechanism of action, delivery route, target, chemical structure (SMILES format), and other information about drugs. Drugs with “launched”, “phase I/II/III clinical trial”, “pre-registration”, or “registered” as the global status were screened out, and those with the delivery route of “oral” or “oral, swallowed” were also excluded. These criteria allowed us to obtain candidate drugs with targeting ability, quick onset of action, and few side effects. Drugs derived from the DTI analysis may be candidates for KL and HS treatment.
DeepPurpose
In order to utilize DeepPurpose, we first translated the target proteins into amino acid sequences and the potential drugs into SMILES fingerprints. Taking the sequences and fingerprints as input, we used the pre-trained models provided by DeepPurpose to predict the binding affinity between each paired drug molecule and protein target of interest. As DeepPurpose provides 15 pre-trained models, we predicted the binding affinity score for each pre-trained model individually and screened the potential drug-target interaction by setting appropriate thresholds. We validated the results using the validation set we collected. We also calculated aggregated binding affinity scores with the aggregation schema proposed by DeepPurpose. Finally, the differences in the predicted binding affinity scores obtained using single models and aggregate models were analyzed.
Statistical analysis
Statistical analyses were carried out using machine learning algorithm in DeepPurpose.
Results
Results of text mining, biological process, and KEGG pathway analysis
Through the data mining process described in Figure 1, 135 genes relating to “scar hypertrophy”, “keloid”, “hypertrophic scar”, and “hyperplastic scar” were found. After deleting the duplicates, we were left with 69 genes (Figure 2). In the analysis of enriched GO biological process annotations, the P value cutoff (P=1.00e-11) was set to select the most enriched biological processes relevant to the pathology of KL and HS, which resulted in 7 sets of annotations containing 39 genes (Table 1). The 5 most enriched biological process annotations were: “positive regulation of epithelial to mesenchymal transition” (P=1.41E-13), the “transforming growth factor beta receptor signaling pathway” (P=2.67E-13), the “cytokine-mediated signaling pathway” (P=4.17E-13), “wound healing” (P=4.42E-12), and “pathway-restricted SMAD protein phosphorylation” (P=1.54E-11). For the KEGG pathway analysis, the P value cutoff was set to P=1.00e-14, which resulted in 25 genes in 7 pathways above the cutoff (Table 2). The top 3 most enriched biological process annotations were: the “AGE-RAGE signaling pathway in diabetic complications” (P=1.71E-21), “pathways in cancer” (P=5.43E-16), and the “TGF-beta signaling pathway” (P=8.08E-16).
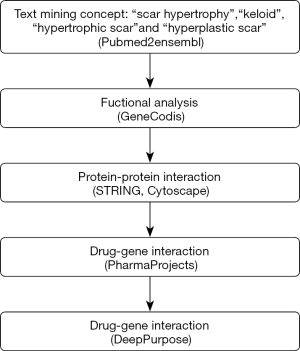
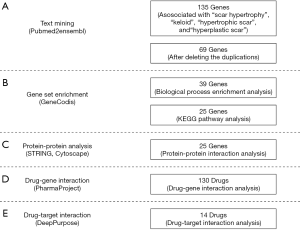
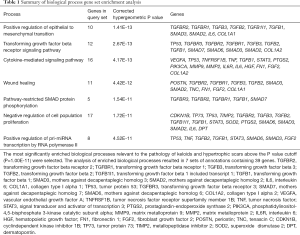
Full table
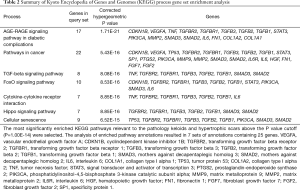
Full table
Results of PPI network analysis
The PPIs of the 25 target genes were analyzed using the STRING database (Figure 3). Data from STRING were then input into Cytoscape to generate the PPI network (Figure 4). In CentiScaPe, the average values of the 2 important centrality parameters, degree and betweenness, were 10.00 and 15.44, respectively. The final gene list included “CDKN1B”, “VEGFA”, “TNF”, “TGFBR1”, “TGFBR2”, “TGFB1”, “TGFB2”, “TGFB3”, “STAT3”, “PIK3CA”, “MMP2”, “SMAD2”, “SMAD3”, “IL6”, “IL6R”, “FN1”, “COL1A1”, “COL1A2”, “TP53”, “SP1”, “PTGS2”, “MMP9”, “HGF”, “FGF2”, and “FGF7”.
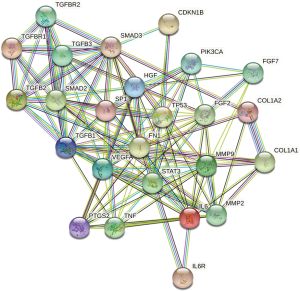
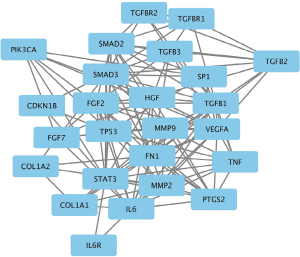
Results of drug-gene interaction analysis
A total of 130 drugs targeting the final gene list were initially selected as possible treatments for KL and HS. These drugs included 30 vascular endothelial growth factor A (VEGFA) receptor antagonists, 27 prostaglandin-endoperoxide synthase 2 (PTGS2) inhibitors, 15 tumor necrosis factor alpha (TNF-α) antagonists, 14 transforming growth factor beta 1 (TGF-β1) antagonists, 8 hepatocyte growth factor (HGF) receptor agonists, 8 interleukin (IL)-6 antagonists, 7 IL-6 receptor (IL-6R) antagonists, 5 fibroblast growth factor (FGF2) agonists, 5 TGF-β1 antagonists, 5 PI3 kinase inhibitors, 4 STAT 3 inhibitors, 1 matrix metalloproteinase-9 (MMP-9) inhibitor and 1 TGF-β3 antagonist.
Results of DeepPurpose analysis
DeepPurpose requires drug molecules to be in the SMILES format, so 34 pharmaceutical compounds with SMILES structure were selected for DeepPurpose analysis. Subsequently, each pre-trained model in DeepPurpose generated a ranked list showing the predicted binding affinity between the drugs and molecules (Table 3). A threshold of pKd ≥7.0 was used for models based on the DAVIS and the BindingDB datasets, while for models based on the KIBA dataset, the threshold was set to 12.1.
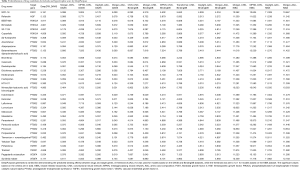
Full table
For the generation of the final outcomes, DeepPurpose proposed 3 aggregation schemas—the mean, max, and average of the max and mean—to combine the predictions from different models. We applied these schemas separately on the models trained on the same dataset, which gave us 9 additional ranked lists of binding affinity scores. The chosen thresholds were also used to screen potential drug-target pairs (Table 4). The final drug list consisted of 14 drugs, including 2 PI3K inhibitors, 10 PTGS2 inhibitors, and 2 VEGFA antagonists (Table 5).
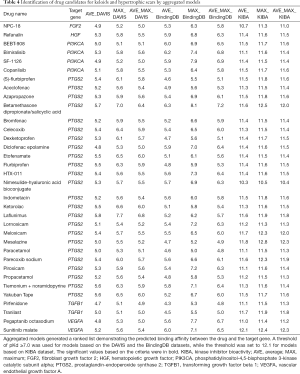
Full table
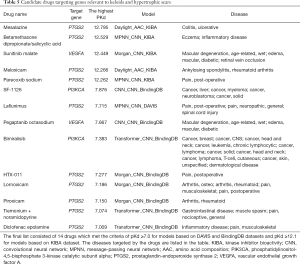
Full table
Discussion
Keloids (KL) and hypertrophic scars (HS) are common dermal fibroproliferative disorders, which place a burden on the health of individuals worldwide. However, the pathogeneses of KL and HS have not been elucidated, and current therapeutic approaches have limited effectiveness. Through gene set enrichment analysis, this study identified 25 genes closely related to the pathology of KL and HS, and a list of 14 drugs targeting 3 of the key genes was compiled using DeepPurpose. Potential drugs can be divided into PI3K inhibitors, PTGS2 inhibitors and VEGFA antagonists.
Prostaglandin-endoperoxide synthase 2 encoded by the PTGS2 gene, also known as cyclooxygenase-2 (COX-2), is the rate-limiting enzyme of prostaglandin biosynthesis (20). The involvement of COX-2 in the pathogeneses of scar lesions has been evidenced. Studies have demonstrated that COX-2 is significantly overexpressed in KL and HS tissues, while down-regulation of COX-2 may reduce KL and HS formation (21-24). After tissue injury, COX-derived prostaglandin E2 (PGE2) promotes the recruitment of inflammatory cells, which release TGF-β or platelet-derived growth factors; thereby, extracellular matrix and fibroblast activation is enhanced, leading to fibroblast proliferation and collagen production (25). The reduction of KL and HS formation in patients using nonsteroidal anti-inflammatory drugs and COX-2 inhibitors has suggested that COX-2 inhibitors may serve as a therapeutic strategy for KL and HS, which is consistent with our findings. Diprosalic, one of the PTGS2 inhibitors found to hold promise in this study, is a combination of betamethasone dipropionate and salicylic acid. It is currently used to treat psoriasis and inflammatory diseases like dermatitis and eczema, as well as to manage subacute and chronic hyperkeratotic and dry dermatoses that are responsive to corticosteroid therapy (26,27). Other COX-2 inhibitors include meloxicam, lornoxicam, piroxicam, mesalazine, parecoxib sodium, HTX-011, tiemonium noramidopyrine, and diclofenac epolamine, the indications for which are postoperative pain and arthritis. These drugs may represent promising treatments for KL and HS.
The involvement of the phosphatidylinositol-4,5-bisphosphate 3-kinase (PI3K)/protein kinase B (Akt)/mammalian target of rapamycin (mTOR) signaling pathway in the pathogeneses of KL and HS has been reported previously. Activation of the PI3K/Akt/mTOR pathway has been demonstrated to enhance the inflammation, angiogenesis, and deposition of extracellular matrix components in scar formation; thus, it is considered to be related to several fibrous diseases (28). CUDC-907, a dual inhibitor of the PI3K/Akt/mTOR pathway and histone deacetylase (HDAC), was found to reverse the pathological phenotype of KL fibroblasts (29). In this study, we found 2 PI3K inhibitors to have potential as drug therapies. Bimiralisib is a dual inhibitor of PI3K and the mammalian target of rapamycin (mTOR). It has been identified as a clinical candidate with potential antineoplastic activity, including in malignant lymphomas, primary central nervous system lymphoma (PCNSL), head and neck squamous cell carcinoma (HNSCC), advanced solid tumors, and metastatic breast cancer (30,31). Another PI3K inhibitor, SF-1126, which selectively inhibits all PI3K class IA isoforms as well as DNA-dependent protein kinase (DNA-PK) and mTOR, is the focus of current phase I clinical trials for chronic lymphocytic leukemia and advanced or metastatic solid tumors (32). In a phase I clinical trial, this drug showed considerable efficacy against B-cell malignancies and solid tumors with no dose-limiting toxicities or hepatotoxicities (33). However, the incorporation of novel PI3K inhibitors into treatment strategies for KL and HS still requires further experimental research and long-term trials to ascertain their tolerability, efficacy, and safety.
VEGF (or VEGFA, the most abundant VEGF isoform) has been implicated as a crucial participant in pathological wound healing (34). Multiple studies on KL and HS have reported an association of high VEGFA levels with scar formation (35-38). Furthermore, there is experimental evidence that VEGF inhibition may be an approach to reducing deposition of scar tissue (37,39-41). In this study, we identified 2 VEGF antagonists as potential drugs to treat KL and HS. Sunitinib malate, a dual inhibitor of VEGF and PDGF receptors, is a lead injectable sustained-release candidate used in the treatment of wet age-related macular degeneration (AMD) (42). It is also under development for the treatment of diabetic macular edema and retinal vein occlusion (43). Meanwhile, pegaptanib octasodium, a pegylated oligonucleotide aptamer, is a direct inhibitor of VEGF that is used as an anticancer agent and in AMD. However, clinical testing to determine whether VEGF inhibition is an effective anti-scarring strategy will need to be performed.
In this study, we used DeepPurpose to predict the interactions of candidate drugs and gene targets in order to select the drugs with the highest predicted binding scores. In the knowledge of the relevance between candidate drugs and target genes, the identification of interactions between them became our major objective. The potential of machine learning models to predict the binding affinity between new drug-target pairs has been demonstrated in various studies. Bagherian et al. (44) briefly reviewed drug-target interaction prediction by machine learning models. Recently, machine learning methods have been used to search for cures for severe acute respiratory syndrome coronavirus 2 (SARS-CoV-2) (45-47), which has given direction for the promotion of new drug discovery. DeepPurpose, the toolkit we used in the current study, is built on the basis of an encoder–decoder framework. The encoders are generated from novel machine-learning approaches for drug-target interaction prediction to extract features from candidate drugs and target genes, while the decoder is a multi-layer perceptron that uses the extracted features to compute the binding affinity scores. With the 15 pre-trained models and 3 aggregation schemas provided by DeepPurpose, we finally obtained 24 different ranked lists of binding affinity score predictions. Though, we selected all potential drugs that meet the threshold criteria under each model, further analysis of pros and cons of the models may give a better guidance in drug screening with larger datasets. We built a validation set to evaluate these models. For each pair in the validation set, we collect the kinase dissociation constant (
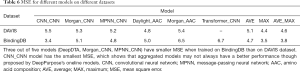
Full table
The results paved the way to obtaining the best drug-target pair. Firstly, the MSEs showed that models trained on larger datasets outperformed those trained on smaller datasets. Three out of 5 models (DeepDTA, Morgan_CNN, MPNN_CNN, and Morgan_ACC) had a smaller MSE when trained on the BindingDB dataset than on the DAVIS dataset. This is often the case for machine learning models: those trained on a larger dataset have better generalizability, since the larger the training set is, the greater opportunity is for the model to learn global patterns. Moreover, by comparing the MSE of the single models and the aggregated models, we found that aggregated models do not always outperform single models, especially when aggregation is applied to models with a considerable variance in performance. However, for models trained on the DAVIS dataset, aggregated models performed better. The model with mean schema had a smaller MSE than most single models, while models with the max and the average of the mean and max schemas outperformed even the best single model. With the BindingDB dataset, however, the aggregated models did not perform as well as the best single model but did outperform most of the single models. This implies that although the use of aggregation schema can, to a certain extent, reduce the limitation and bias of single models, it can also introduce additional errors by aggregating the results of poor models.
Conclusions
Our study has demonstrated that drug discovery using in silico text mining and DeepPurpose may be a powerful and effective way to find drugs targeting the genes related to KL and HS. Therefore, our study could provide a theoretical basis for the development of novel targeted therapies for KL and HS.
Acknowledgments
Funding: This work was supported by the National Natural Science Foundation of China (grant No. 81671915).
Footnote
Reporting Checklist: The authors have completed the MDAR checklist. Available at http://dx.doi.org/10.21037/atm-21-218
Conflicts of Interest: All authors have completed the ICMJE uniform disclosure form (available at http://dx.doi.org/10.21037/atm-21-218). The authors have no conflicts of interest to declare.
Ethical Statement: The authors are accountable for all aspects of the work in ensuring that questions related to the accuracy or integrity of any part of the work are appropriately investigated and resolved. The study was conducted in accordance with the Declaration of Helsinki (as revised in 2013).
Open Access Statement: This is an Open Access article distributed in accordance with the Creative Commons Attribution-NonCommercial-NoDerivs 4.0 International License (CC BY-NC-ND 4.0), which permits the non-commercial replication and distribution of the article with the strict proviso that no changes or edits are made and the original work is properly cited (including links to both the formal publication through the relevant DOI and the license). See: https://creativecommons.org/licenses/by-nc-nd/4.0/.
References
- Ogawa R. Mechanobiology of scarring. Wound Repair Regen 2011;19 Suppl 1:s2-9. [Crossref] [PubMed]
- Marneros AG, Norris JE, Olsen BR, Reichenberger E. Clinical genetics of familial keloids. Arch Dermatol 2001;137:1429-34. [Crossref] [PubMed]
- Chiang RS, Borovikova AA, King K, et al. Current concepts related to hypertrophic scarring in burn injuries. Wound Repair Regen 2016;24:466-77. [Crossref] [PubMed]
- Kim S, Choi TH, Liu W, et al. Update on scar management: guidelines for treating Asian patients. Plast Reconstr Surg 2013;132:1580-9. [Crossref] [PubMed]
- Lee HJ, Jang YJ. Recent Understandings of Biology, Prophylaxis and Treatment Strategies for Hypertrophic Scars and Keloids. Int J Mol Sci 2018;19:711. [Crossref] [PubMed]
- Moosavinasab S, Patterson J, Strouse R, et al. 'RE:fine drugs': an interactive dashboard to access drug repurposing opportunities. Database (Oxford) 2016;2016:baw083. [Crossref] [PubMed]
- Mullard A. New drugs cost US$2.6 billion to develop. Nature Reviews Drug Discovery 2014;13:877. [Crossref]
- Mayr A, Klambauer G, Unterthiner T, et al. Large-scale comparison of machine learning methods for drug target prediction on ChEMBL. Chem Sci 2018;9:5441-51. [Crossref] [PubMed]
- Smalley E. AI-powered drug discovery captures pharma interest. Nat Biotechnol 2017;35:604-5. [Crossref] [PubMed]
- Fleming N. How artificial intelligence is changing drug discovery. Nature 2018;557:S55-7. [Crossref] [PubMed]
- Liu T, Lin Y, Wen X, et al. BindingDB: a web-accessible database of experimentally determined protein-ligand binding affinities. Nucleic Acids Res 2007;35:D198-201. [Crossref] [PubMed]
- Tang J, Szwajda A, Shakyawar S, et al. Making sense of large-scale kinase inhibitor bioactivity data sets: a comparative and integrative analysis. J Chem Inf Model 2014;54:735-43. [Crossref] [PubMed]
- Davis MI, Hunt JP, Herrgard S, et al. Comprehensive analysis of kinase inhibitor selectivity. Nat Biotechnol 2011;29:1046-51. [Crossref] [PubMed]
- Huang K, Fu T, Glass L, et al. DeepPurpose: a Deep Learning Library for Drug-Target Interaction Prediction and Applications to Repurposing and Screening. 2020.
- Baran J, Gerner M, Haeussler M, et al. pubmed2ensembl: a resource for mining the biological literature on genes. PLoS One 2011;6:e24716. [Crossref] [PubMed]
- Carmona-Saez P, Chagoyen M, Tirado F, et al. GENECODIS: a web-based tool for finding significant concurrent annotations in gene lists. Genome Biol 2007;8:R3. [Crossref] [PubMed]
- Szklarczyk D, Franceschini A, Wyder S, et al. STRING v10: protein-protein interaction networks, integrated over the tree of life. Nucleic Acids Res 2015;43:D447-52. [Crossref] [PubMed]
- Su G, Morris JH, Demchak B, et al. Biological network exploration with Cytoscape 3. Curr Protoc Bioinformatics 2014;47:8.13.1-24.
- Jardim DL, Groves ES, Breitfeld PP, et al. Factors associated with failure of oncology drugs in late-stage clinical development: A systematic review. Cancer Treat Rev 2017;52:12-21. [Crossref] [PubMed]
- Sobolewski C, Cerella C, Dicato M, et al. The role of cyclooxygenase-2 in cell proliferation and cell death in human malignancies. Int J Cell Biol 2010;2010:215158. [Crossref] [PubMed]
- Abdou AG, Maraee A, Saif H. Immunohistochemical evaluation of COX-1 and COX-2 expression in keloid and hypertrophic scar. Am J Dermatopathol 2014;36:311-7. [Crossref] [PubMed]
- Rossiello L, D'andrea F, Grella R, et al. Differential expression of cyclooxygenases in hypertrophic scar and keloid tissues. Wound Repair Regen 2009;17:750-7. [Crossref] [PubMed]
- Louw L. The keloid phenomenon: progress toward a solution. Clin Anat 2007;20:3-14. [Crossref] [PubMed]
- Wilgus TA, Vodovotz Y, Vittadini E, et al. Reduction of scar formation in full-thickness wounds with topical celecoxib treatment. Wound Repair Regen 2003;11:25-34. [Crossref] [PubMed]
- Stratton R, Shiwen X. Role of prostaglandins in fibroblast activation and fibrosis. J Cell Commun Signal 2010;4:75-77. [Crossref] [PubMed]
- Shou M, Galinada W, Wei Y, et al. Development and validation of a stability-indicating HPLC method for simultaneous determination of salicylic acid, betamethasone dipropionate and their related compounds in Diprosalic Lotion. J Pharm Biomed Anal 2009;50:356-61. [Crossref] [PubMed]
- Guenther LC. Fixed-dose combination therapy for psoriasis. Am J Clin Dermatol 2004;5:71-7. [Crossref] [PubMed]
- Wong VW, You F, Januszyk M, et al. Transcriptional profiling of rapamycin-treated fibroblasts from hypertrophic and keloid scars. Ann Plast Surg 2014;72:711-9. [Crossref] [PubMed]
- Tu T, Huang J, Lin M, et al. CUDC 907 reverses pathological phenotype of keloid fibroblasts in vitro and in vivo via dual inhibition of PI3K/Akt/mTOR signaling and HDAC2. Int J Mol Med 2019;44:1789-800. [Crossref] [PubMed]
- Tarantelli C, Gaudio E, Arribas A, et al. PQR309 Is a Novel Dual PI3K/mTOR Inhibitor with Preclinical Antitumor Activity in Lymphomas as a Single Agent and in Combination Therapy. Clin Cancer Res 2018;24:120-9. [Crossref] [PubMed]
- Beaufils F, Cmiljanovic N, Cmiljanovic V, et al. 5-(4,6-Dimorpholino-1,3,5-triazin-2-yl)-4-(trifluoromethyl)pyridin-2-amine (PQR309), a Potent, Brain-Penetrant, Orally Bioavailable, Pan-Class I PI3K/mTOR Inhibitor as Clinical Candidate in Oncology. J Med Chem 2017;60:7524-38. [Crossref] [PubMed]
- Qin AC, Li Y, Zhou LN, et al. Dual PI3K-BRD4 Inhibitor SF1126 Inhibits Colorectal Cancer Cell Growth in Vitro and in Vivo. Cell Physiol Biochem 2019;52:758-68. [Crossref] [PubMed]
- Mahadevan D, Chiorean E, Harris W, et al. Phase I pharmacokinetic and pharmacodynamic study of the pan-PI3K/mTORC vascular targeted pro-drug SF1126 in patients with advanced solid tumours and B-cell malignan-cies. Eur J Cancer 2012;48:3319-27. [Crossref] [PubMed]
- Le AD, Zhang Q, Wu Y, et al. Elevated vascular endothelial growth factor in keloids: relevance to tissue fibrosis. Cells Tissues Organs 2004;176:87-94. [Crossref] [PubMed]
- Gira AK, Brown LF, Washington CV, et al. Keloids demonstrate high-level epidermal expression of vascular en-dothelial growth factor. J Am Acad Dermatol 2004;50:850-3. [Crossref] [PubMed]
- van der Veer WM, Niessen FB, Ferreira JA, et al. Time course of the angiogenic response during normotrophic and hypertrophic scar formation in humans. Wound Repair Regen 2011;19:292-301. [Crossref] [PubMed]
- Mogili NS, Krishnaswamy VR, Jayaraman M, et al. Altered angiogenic balance in keloids: a key to therapeutic intervention. Transl Res 2012;159:182-9. [Crossref] [PubMed]
- Ong CT, Khoo YT, Tan EK, et al. Epithelial-mesenchymal interactions in keloid pathogenesis modulate vascular endothelial growth factor expression and secretion. J Pathol 2007;211:95-108. [Crossref] [PubMed]
- Wang J, Chen H, Shankowsky HA, et al. Improved scar in postburn patients following interferon-alpha2b treatment is associated with decreased angiogenesis mediated by vascular endothelial cell growth factor. J Interferon Cytokine Res 2008;28:423-34. [Crossref] [PubMed]
- Salem A, Assaf M, Helmy A, et al. Role of vascular endothelial growth factor in keloids: a clinicopathologic study. Int J Dermatol 2009;48:1071-7. [Crossref] [PubMed]
- Wu WS, Wang FS, Yang KD, et al. Dexamethasone induction of keloid regression through effective suppression of VEGF expression and keloid fibroblast proliferation. J Invest Dermatol 2006;126:1264-71. [Crossref] [PubMed]
- A Depot Formulation of Sunitinib Malate (GB-102) in Subjects With Neovascular (Wet) Age-related Macular De-generation. Available online: https://clinicaltrials.gov/show/NCT03249740
- GrayBug. Available online: https://www.graybug.vision/our-technologies-and-pipeline/#pipeline
- Bagherian M, Sabeti E, Wang K, et al. Machine learning approaches and databases for prediction of drug-target interaction: a survey paper. Brief Bioinform 2021;22:247-69. [Crossref] [PubMed]
- Beck BR, Shin B, Choi Y, et al. Predicting commercially available antiviral drugs that may act on the novel coronavirus (SARS-CoV-2) through a drug-target interaction deep learning model. Comput Struct Biotechnol J 2020;18:784-90. [Crossref] [PubMed]
- Zhang H, Saravanan KM, Yang Y, et al. Deep Learning Based Drug Screening for Novel Coronavirus 2019-nCov. Interdiscip Sci 2020;12:368-76. [Crossref] [PubMed]
- Nand M, Maiti P, Joshi T, et al. Virtual screening of anti-HIV1 compounds against SARS-CoV-2: machine learning modeling, chemoinformatics and molecular dynamics simulation based analysis. Sci Rep 2020;10:20397. [Crossref] [PubMed]
(English Language Editor: J. Reynolds)