Comprehensive serum metabolic and proteomic characterization on cognitive dysfunction in Parkinson’s disease
Introduction
Parkinson’s disease (PD) is the second-most common neurodegenerative disorder; its prevalence is increasing, and it currently affects 2–3% of the population ≥65 years of age (1). Cognitive dysfunction is one of the most frequent and troublesome non-motor issues in PD. Cognitive dysfunction and other non-motor symptoms can be preceded by a prodromal phase of years or even decades (2,3). Also, the clinical manifestations of PD usually lag behind changes in the brain or blood (4). It is not realistic to utilize the postmortem human brain tissue for proteomics and metabolomics analyses. Instead, analysis of proteins and metabolites in biofluids, such as serum, are relatively easy to obtain information and promote the understanding of the specific biological system and regulatory mechanisms in PD, which is conducive to identify the biomarkers and characteristics of PD patients with cognitive dysfunction. The precise mechanism of cognitive dysfunction in PD remains elusive. Identifying the α-synuclein as the first target etiological biomarker was especially relevant considering the pathophysiology of PD (5). Numerous attempts have been made to explore the proteomics and metabolomics of biological fluids such as cerebrospinal fluid (CSF), serum, blood, and urine. In a previous proteomics study, synaptic proteins in CSF showed the potential to detect impairments in synaptic function and neurotransmitter transmission (6). Moreover, metabolites have been considered a potential reflection of physiologic and pathologic conditions.
The emergence of novel methods and methodologic improvement promised a bright future geared toward identifying and quantifying metabolites and proteins in biological samples (7). Specific and sensitive biomarkers can assess disease risk during the early phase. For example, glutamatergic dysfunction was identified as a contributor to neurodegenerative disease pathology (8), and glutamine is significantly higher in PD patients than the non-PD population (9). Some PD indicators are known, and evidence exists for others, however, some have yielded contradictory findings that makes their true relationship with non-motor symptoms unclear. One study reported that decreased total tau (t-tau) and phosphorylated tau (p-tau) in CSF can predict cognitive impairment in PD (10). However, subsequent prospective longitudinal studies failed to show associations between t-tau and p-tau levels and cognitive decline (11,12). There remains a need to establish sensitive, specific, and reliable biomarkers for PD.
Due to each-omics technique’s specificity and the limitations of employing a single-omics strategy, it is important to use complementary and powerful techniques to identify more precise biomarkers; this approach reduces the bias inherent from a single method. Our research’s primary objective was to use systematic and comprehensive technology to reveal the characteristics of metabolism and protein in serum biology of PD with significant cognitive impairment. Our findings supported significant differences in the proteomic and metabolic profiles of PD exhibiting an inflammatory and lipid metabolism profile alterations. Meanwhile, we hope to find a potential biomarker with instructive significance. This could profoundly impact PD diagnosis. Additionally, the findings of altered metabolic pathways in PD patients will further the understanding of the pathophysiological mechanisms underlying cognitive impairments in PD. We present the following article following the TRIPOD reporting checklist (available at http://dx.doi.org/10.21037/atm-20-4583).
Methods
The study was conducted in accordance with the Declaration of Helsinki (as revised in 2013). The study was approved by clinical research ethics committee of Xuzhou Medical University Affiliated Hospital (XYFY2017-KL047-01, XYFY2017-KL047-02, XYFY2017-KL047-03) and informed consent was taken from all individual participants.
Participants
We enrolled 57 participants with PD.
Inclusion and exclusion criteria
Patients were included if they met the following criteria: (I) PD diagnosis confirmed by the UK Brain Bank Criteria (13), (II) aged between 50 and 75 years, (III) Hoehn and Yahr (H-Y) stage <5; (IV) serum material available for analysis; and (V) provided written informed consent. Participants were excluded if they had any history of: (I) severe vascular encephalopathy or normal-pressure hydrocephalus, (II) head injury, (III) metabolic disease, (IV) severe psychiatric illness, or (V) medication-induced PD.
Clinical evaluation (behavioral and cognitive assessments) and potential study confounders
We collected participant demographic and clinical characteristics including age, sex, years of education, age at onset, occupation, anthropometrics, social habits, alcohol and tobacco use. The Unified Parkinson’s Disease Rating Scale (UPDRS) and H-Y stage were used to classify movement disorder severity. Structured questionnaires including the Mini-Mental State Examination (MMSE) and Montreal Cognitive Assessment (MoCA) were used to assess global cognitive function. The cognitive status diagnosis in patients with PD was assigned by consensus among 2 neurologists on the basis of a neuropsychological battery (MMSE, MoCA) and the physician-administered neurologic examination and physician-visits on daily living status.
Grouping based on cognition function level
First of all, based on the MMSE results, the subjects who scored more than 26 were deemed to be no cognitive impairment preliminarily. Then the MoCA assessment was further combined for further cognition examination. In addition, clinicians’ daily follow-up and inquiry of patients’ daily cognitive performance is also a big determinant for cognitive evaluation. Synthesizing the above three aspects, the presence or absence of cognitive impairment of PD patients was judged by the clinician. After that, researchers in lab began to analyze the cognitive characteristics of these two groups and reveal the comprehensive serum metabolic and proteomic characterization of these two groups. Subjects were grouped by clinician judgment and then data was analyzed by researchers to avoid the occurrence of selective bias in our study. During the grouping cognitive analysis, we found that one group subjects who have relative good cognitive performance, hardly any impact on life, so defined as cognitively healthy patients with PD group (PD-N). On the contrary, subjects who scored less than 26 of MMSE and were also poor performance in MoCA scale and daily life were regarded as cognitively impairment patients with PD group (PD-CI).
Serum sample preparation
On the morning of the patient’s enrollment (7:00–9:00), vacuum tubes (BD Biosciences, Franklin Lakes, NJ, USA) were used to collect peripheral venous blood in a fasting state. The supernatant was subjected to centrifugation at 3,000 rpm for 15 minutes and was then collected and maintained at −80 °C until analysis.
Proteomic analysis
Serum high-abundance protein depletion
Serum samples were processed to deplete the top two [albumin, immunoglobulin G (IgG)] high-abundance proteins using the ProteoExtractTM Albumin/IgG Removal Kit (Calbiochem, La Jolla, CA, USA).
Isobaric tags for relative and absolute quantitation (iTRAQ) labeling
According to the bicinchoninic acid assay quantitative results, 100 µg of protein from each sample was moved to a 1.5-mL tube, 8 M urea [0.1% sodium dodecyl sulfate (SDS)] and ultra-pure water (0.1% SDS) was added, and 4.5 L of triethylammonium bicarbonate (1 M) was added. The total volume was up to 100 µL, and the urea concentration was 4 M. We added 5 µL 200 mM tris(2-carboxyethyl) phosphine, and the sample was incubated at 55 °C for 1 h. The sample was acetylated with 5 µL of 375 mM iodoacetamide, followed by 660 µL of acetone overnight. The precipitation was washed with acetone, and then 2.5 µg trypsin was added for overnight enzyme digestion. The proteins were labeled with iTRAQ reagent (catalog no. 4390812; AB SCIEX, Framingham, MA, USA) (14). Reverse-phase liquid chromatography separation and mass spectrometry (Q Exactive mass spectrometer; Thermo Fisher Scientific, Waltham, MA, USA) were performed by CaptialBio Technology (Beijing, China).
Mass spectrometry and bioinformatic analyses
Protein identification was performed using the Mascot search engine (version 2.3.0; Matrix Science, London, UK) against the Uniprot_Human Database containing 216,686 sequences. All proteins were submitted for database searching and quantitative analysis using Protein Pilot v 3.0 (Applied Biosystems, Foster City, CA, USA). The conditions for screening differentially expressed proteins were as follows: fold change ≥1.5 and P value ≤0.05; fold change ≤2/3 and P value ≤0.05. Blast2GO software (www.blast2go.org) was used to obtain Gene Ontology (GO) annotations. The Kyoto Encyclopedia of Genes and Genomes (KEGG) was also used to identify pathways (data not shown).
Non-targeted metabolomics analysis
Sample preparation
For ultra-high-performance liquid chromatography linked to tandem mass spectrometry (UHPLC-Q-TOF/MS), 400 µL of MeOH/acetonitrile (ACN) (1:1, v/v) containing 2-chloro-L-phenylalanine (1,000:5, 1 mg/mL stock in dH2O) were added to 100 µL of each serum sample, and the samples were vigorously vortexed for 30 s. The solutions were then centrifuged at 12,000 rpm for 15 min at 4 °C. Next, 425 µL of the supernatant was transferred to a clean vial and dried in a vacuum concentrator. Dried samples were subsequently reconstituted with 100 µL of ACN/H2O (1:1, v/v) and vortexed for 30 s. A 60 µL aliquot of the supernatant was then subjected to UHPLC-Q-TOF/MS. Additionally, 10 µL of each sample was taken and pooled as quality control (QC) samples.
Chromatography and mass spectrometry
UHPLC-Q-TOF/MS analysis was performed using a UHPLC system (1,290 series, Agilent Technologies, Santa Clara, CA, USA) coupled to a quadrupole time-of-flight (TOF) mass spectrometer (Triple TOF 5600, AB SCIEX). The Triple TOF mass spectrometer was used for its ability to acquire mass spectrometry (MS/MS)/MS spectra on an information-dependent basis during a liquid chromatography (LC)/MS experiment with acquisition software (Analyst TF 1.7, AB SCIEX) that continuously evaluates the full scan survey of MS data as it collects and triggers MS/MS spectra acquisition depending on preselected criteria. Electrospray ionization source conditions were set as follows: ion source gas 1, 60 Psi; ion source gas 2, 60 Psi; curtain gas pressure, 35 Psi; source temperature, 650 °C; ion spray voltage floating, 5,000 V in the positive mode or −4,000 V in the negative mode; collision energy, 30 eV.
Quality control
The same concentration and amount of internal standard substance L-2-chlorophenylalanine were added to each sample detected by UPLC-QTOF-MS to monitor instrument performance or correct the data.
Metabolomics analysis
MS raw data (wiff) files were converted to the mzXML format using ProteoWizard and processed by R package XCMS (version 3.2). R package CAMERA was used for peak annotation after XCMS data processing. An in-house MS2 database was used for metabolite identification. SIMCA-P 14.1 (Umetrics, Umeå, Sweden) was employed for multivariable analysis, including principal components analysis (PCA) with mean-centered (ctr) scaling and orthogonal partial least squares discriminant analysis (OPLS-DA) with unit variance scaling. Variables with VIP (variable importance in projection) >1 were considered to have significant contributions to the model. R2 and Q2 were used to characterize the explanatory and predictive ability of the model. Potential metabolic biomarkers with VIP >1 and a Student’s t-test P value <0.05 were selected. Differentially abundant metabolites were cross-referenced to the pathways by further searching commercial databases including KEGG (http://www.genome.jp/kegg/) and MetaboAnalyst (http://www.metaboanalyst.ca/).
Combined proteomics and metabolomics analysis
Two-way orthogonal partial least squares (O2PLS) analysis
O2PLS analysis (15) is an integrative method capable of modeling systematic variation while providing more parsimonious models that aid interpretation (Figure S1). Cross-validation was performed to calculate Q2 values. Loadings from O2PLS are interpreted in the same way as for PCA; specifically, we looked for clusters, outliers, and interesting patterns in the line plots.
Correlation analyses of proteins and metabolites
Spearman algorithm for correlation calculation was used to obtain the correlation coefficient matrix and correlation P value.
KEGG pathway analysis of sphingolipid and arachidonic acid metabolism
Positively correlated metabolomics data obtained as described above were divided into the following categories: sphingolipid, arachidonic acid (AA), and lecithin. Quantitative changes of individual metabolites and proteins and adjacent regulated factors were calculated and graphed on the KEGG-based pathway map as a grade function.
Creation of network pathway maps
Ingenuity pathway analysis (IPA) was used to identify interaction networks among selected candidates from the above data using Cytoscape 3.6.1. Signaling pathway annotations and relevant networks were generated using IPA software (IPA; QIAGEN, Hilden, Germany), which was introduced in 2003 and helps researchers analyze omics data and model biological systems.
Statistical analysis
Group differences in categorical variables (e.g., sex, levodopa use, and smoking status) were assessed with chi-square tests. Group comparisons of all other clinical characteristics (e.g., age, UPDRS score), and relative levels of different indexes, were performed using the Student’s t-tests test if continuous variables follow a normal distribution, otherwise Kruskal-Wallis H test. General linear model analysis with mixed effects was adapted to investigate metabolite level differences. Relationships between metabolites, proteins, and clinical measurements were explored using Spearman correlation coefficients. The results are shown in the form of a matrix. Partial correlation analysis based on a general linear model was used to calibrate correlation analysis results adjusted for age, sex, smoking status, hypertension, diabetes, disease duration, and H-Y grade. Finally, serial analysis of MMSE and MoCA results was carried out using a linear regression method. Logistic regressions were carried out between serum metabolites, baseline characteristics, and clinical diagnosis. Receiver operating characteristic (ROC) curves were constructed and the areas under the ROC curves (AUCs) were calculated to evaluate the characteristics of metabolites that could be sensitive biomarkers to identify the presence or absence of cognitive impairment in PD. All statistical analyses were performed using SPSS22.0 software (IBM Corp., Armonk, NY, USA), and differences were considered significant with a two-sided α=0.05.
Results
Demographic characterization of study subjects
A total of 26 cognitively relatively healthy patients with PD (PD-N) and 31 PD patients with cognitive impairment (PD-CI) were enrolled. Demographic data and clinical data are presented in Table 1. There were no significant differences between the two groups with regards to age (normal distribution and homogeneity of variance, t=−0.732, P>0.05), sex ratio, hypertension, diabetes, cigarette smoking, or alcohol drinking (chi-square test, P>0.05), but there was a difference in education status (Z=−2.607, P=0.009). The average PD disease duration was 5.192 (7.047) years for the PD-N group, which was not notably different from the PD-CI group [6.484 (6.152) years (Z=−1.792, P=0.073)]. For the PD scale, the frequency distribution was distinctly different between the groups (Z=−2.034, P=0.042). PD-CI participants had significantly lower scores than PD-N patients on the MMSE (Z=−5.327, P=0.000) and MoCA (Z=−6.266, P=0.000) assessments.
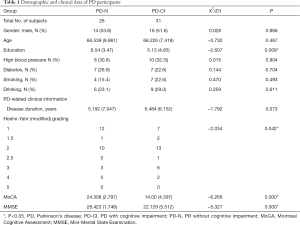
Full table
Identification of differentially serum proteins profiling in PD
Differential serum proteins were compared in PD-CI and PD-N participants using iTRAQ and LC-MS/MS. Serum sample quality was verified by SDS-polyacrylamide gel electrophoresis (Figure S2). We observed that indicates similar expression patterns within the same groups (Figure S3A). We screened for intergroup differentially expressed proteins at a 1% false discovery rate (FDR) by searching the data in the UnitProt Human Database and calculated the p-value and fold change (FC) using a t-test in the R language, and the volcano plots of differential protein expression analysis was showed in Figure S3B. All differentially expressed proteins identified in the iTRAQ study are listed in Table S1. The cluster analysis results showed that the characteristics of samples from the same group were consistent (Figure S3C), which also affirmed the 16 differentially expressed proteins and reinforced the correlation.
Non-targeted metabolomics analysis
For UHPLC-Q-TOF/MS, there were 1,737 metabolite features in the positive ion model and 1,255 metabolite features in the negative ion mode. Typical total ion chromatograms of the metabolic profiles of the PD-CI and PD-N groups’ metabolic profiles are provided in Figure S4.
The PCA score plots obtained for PD-CI and PD-N are shown in Figure S5A,B. The parameters of the OPLS-DA score plots were R2X=0.691, R2Y=0.845, and Q2=0.32 in the positive mode and R2X=0.635, R2Y=0.25, and Q2=−0.0232 in the negative mode (Figure S5C,D). The samples in the two groups segregated into two distinct clusters, and the values indicated clear separation between PD-CI and PD-N.
For UHPLC-Q-TOF/MS, a total of 32 different metabolites were selected, with five metabolites in the positive and negative mode, respectively (Table S2). The screening results were visualized with a volcano plot (Figure S6).
Combined proteomics and metabolomics analysis
Serum metabolite and protein associations
Based on differentially expressed proteins and metabolites, Spearman rank correlations were used. The results are shown as thermograms that depict data with correlation P values <0.05 (Figure 1A,B). The metabolic indicators related to the differential proteins were mainly divided into the three categories: sphingolipid [D-erythro-Sphingosine-1-phosphate (S1P)], phosphatidylcholines {1-palmitoyl-2-hydroxy-sn-glycero-3-phosphoethanolamine [LPE(16:0)], 1-palmitoyl-sn-glycero-3-phosphocholine [LPC(16:0)], 1-stearoyl-2-hydroxy-sn-glycero-3-phosphocholine [LPC(18:0)], 1-oleoyl-sn-glycero-3-phosphocholine [LPC(18:1)], and PC(20:5/20:5)}, and long chain fatty acids (pentadecanoic acid, palmitic acid, AA).
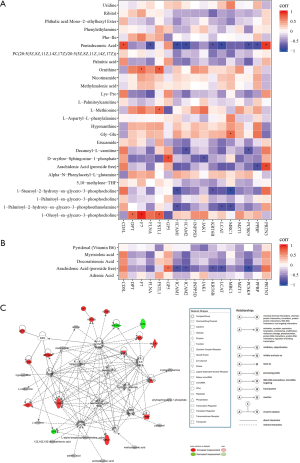
Regulation network analysis by IPA
IPA pathway enrichment analysis (Figure 1C) revealed that the regulatory network associated with PD-CI is primarily related to the immune system. For example, proteins involved in cytokine signaling in the immune system include janus kinase 1 (JAK1), proteinase 3 (PRTN3), inositol polyphosphate-5-phosphatase (INPP5D) and intercellular cell adhesion molecule 1 (ICAM1); those involved in the adaptive immune system term include intercellular cell adhesion molecule 2 (ICAM2), INPP5D, mannose receptor C-type 1 (MRC1), and ICAM1; those involved in the interleukin (IL) signaling term include JAK1, PRTN3, and INPP5D; and those involved in the interferon signaling term include JAK1 and ICAM1. Up- or downstream of these terms, the participating metabolites include palmitic acid, AA, beta-estradiol, hydrogen peroxide, calcium (Ca2+), niacinamide, LPC (16:0), palmitic acid, AA; other participating proteins include epidermal growth factor receptor (EGFR), mitogen activated protein kinase 3 (MAPK3), filamin A (FLNA), and glycoprotein 5 (GP5). Another regulatory network associated with PD-CI is lipid metabolism including proprotein convertase subtilisin/kexin type 9 (PCSK9), INPP5D, and lecithin cholesterol acyltransferase (LCAT); the last is also involved in the glycerophospholipid metabolism. Cytokines (tumor necrosis factor (TNF) and IL-6), metabolites (Ca2+ and hydrogen peroxide), and other proteins (JAK1 and MAPK3) are involved.
Mapping the regulatory and metabolic networks of sphingolipid, AA, and lecithin
A net map was drawn to visualize interactions in the full data set (Figure 2). It shows that 1-acyl-sn-glycero-3-phosohocholine (LPC) was significantly higher in the PD-CI group. There are two ways to generate LPC in vivo: catalyzed by LCAT to form LPC and cholesterol ester or catalyzed by phosphatidylcholine 2-acylhydrolase to hydrolyze the acyl chain at the sn-2 position to form LPC and long-chain fatty acid, which will lead to an increase in LPC. Our research observed increases in both LCAT expression and content of long-chain fatty acid (myristoleic acid, pentadecanoic acid, palmitic acid, AA, adrenic acid, docosatrienoic acid), consistent with the phenomenon of LPC. The concentrations of long-chain fatty acid products fatty acyl carnitine (L-palmitoylcarnitine and decanoyl-L-carnitine) were also significantly increased. On the other hand, LPE (16:0) was significantly elevated, and we speculate that LPE is produced by hydrolysis of phosphatidyl ethanolamine (formed by phosphatidylethanolamine) catalyzed by phosphatidylcholine 2-acyl hydrolase.
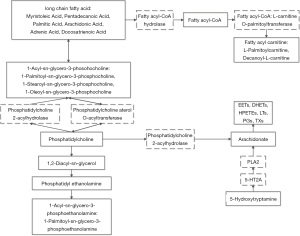
Association of serum metabolites with cognition assessment
We sorted out the targeted metabolites and evaluated related literature to guide our focus to three major substances. Firstly, we generated a statistical description of the serum contents (relative values) of these metabolites (Table S3). PD-CI participants had significantly higher S1P than the controls. Nonparametric testing showed that both indicators were increased in PD-CI. When the raw data were normalized, the t-tests confirmed different levels. This result was somewhat unexpected and prompted additional analyses. Covariance analysis was required to process data (shown in Table 1). By adjusting the different covariates, we found that when education and H-Y were considered as covariates using a general linear model (Model A), LPC (18:1) and PC (20:5/20:5) were significantly different between the groups. Additional adjustments (Model B and Model C, Table 2) confirmed that the significantly different indicators were increased between the groups.
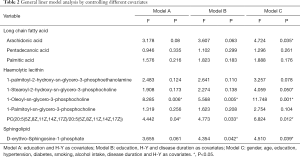
Full table
Next, we investigated metabolites associated with clinical cognition scores using Spearman correlation coefficients and linear regression models. Spearman correlation results showed four metabolites associated with MMSE and three metabolites associated with MoCA (P<0.05, Figure 3A). Given the existence of covariates in our study, partial correlation analysis was used to analyze further the correlations between MMSE and MoCA scores and metabolites parameters among participants. The results revealed a strong negative correlation between MMSE score and LPC (18:1) (b=−0.354, P=0.014). The negative correlation with metabolites is shown in Table S4. The regression models showed that LPC (18:1) and education status were the main factors contributing to cognitive dysfunction (Table S5). We built ROC models for the final selected metabolite for PD-N and PD-CI. LPC (18:1) was the only indicator included in the regression models. The resulting AUC value was 0.660 (P=0.039, Figure 3B).
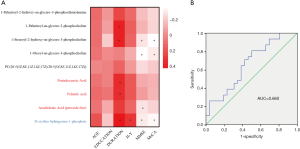
Discussion
This study was to comprehensively characterize the metabolic and proteomics of serum in PD-CI, explore differential metabolites’ association with clinical cognition evaluation, and identify potential early diagnostic biomarkers. Proteomics plays an auxiliary role in illuminating related metabolites’ regulatory mechanism, which also overcomes some of the deficiencies of using just one -omics approach in isolation.
In the present study, correlation and IPA analyses revealed that differences in serum metabolites between the PD-CI and PD-N groups were mainly classified into three categories: sphingolipid, lecithin, and long-chain fatty acids. In our analysis, we mapped the regulatory relationships between proteins and metabolites. We retrospectively assessed clinical data and analyzed the relative quantities of three major substances in the serum samples. After excluding the covariates, a difference in metabolites between the groups was observed. Partial correlation and regression analyses showed that the LPC (18:1) level and education status mostly contributed to cognition evaluation. H-Y grade, LPC (18:1) level, and education status were also implicated in PD cognitive impairment. Also, the amounts of LPC (18:1) alone predicted PD-CI with an ROC AUC of 0.66, suggesting a need to investigate the utility of measuring circulating LPC (18:1) as a biomarker for PD-CI risk and progression.
Associations between cognition and sphingolipid, lecithin, and long-chain fatty acid
The differentially expressed metabolites of PD-CI are mainly involved in lecithin, long-chain fatty acid, and sphingolipid metabolism. Although not all the changes were significant, the biological significance cannot be ignored. Dysfunctional sphingolipid metabolism has been implicated in numerous neurological conditions and is a powerful factor for the occurrence and development of neurodegenerative diseases. The bioactive sphingolipid metabolite S1P affects various neuronal functions including cell fate and is irreversibly degraded by the enzyme S1P lyase, regulating sphingolipid metabolism and apoptosis. It plays important regulatory roles in cell growth, proliferation, adhesion, apoptosis, autophagy (16,17) and intracellular trafficking in the central nervous system (CNS) (18). S1P is one of the most compelling sphingolipids. The S1P-related signaling pathway regulates the synthesis of various neurotrophic factors and pro-inflammatory cytokines, and cellular communication. S1P attenuates free radical generation and increases survival of SH-SY5Y neuronal cells (19). S1P concentration is strongly inversely correlated with tissue Aβ levels and tau hyperphosphorylation in postmortem brain tissue from patients with Alzheimer’s disease (20). Our results indicate that S1P is significantly increased in PD-CI serum, presumably because the substance is destroyed in the brain and metabolites are then found in serum. This process could induce neuronal oxidative stress. In addition, excessive S1P causes cell death accompanied by deoxyribonucleic acid (DNA)fragmentation via the p38 MAPK pathway-mediated release of cytochrome c by neurons. Moreover, sphingolipid metabolites also regulate AA metabolism. A previous study showed that D-erythro-analogs of sphingosine stimulated AA release in PC12 cells. There was synergistic variability between sphingolipid and AA. This suggests that S1P could be an appropriate biomarker of cognitive dysfunction in PD.
We also found that PD-CI patients had significantly increased serum levels of fatty acylcarnitine and long-chain fatty acids compared to PD-N, as well as a strong association between cognition and serum levels of fatty acylcarnitine and long-chain fatty acids (Tables S3-S5). Abnormalities in metabolic intermediates of fatty acid oxidation suggest that PD cognitive dysfunction is likely closely related to fatty acid oxidation. Abnormal regulation of fatty acid oxidation—typically an enzymatic deficiency in fatty acid metabolism (e.g., acyl-CoA dehydrogenase deficiency) or disruption of fatty acid transport across the mitochondrial membrane through defects in the carnitine transport system—primarily results in a metabolic disorder in which fatty acids reach cytotoxic levels (21). Increases in haemolytic lecithin, especially 1-Oleoyl-sn-glycero-3-phosphocholine, is evident in PD-CI patients and are independent of other variables such as age, sex, hypertension, diabetes, smoking, and alcohol consumption (Tables S3,S5). The metabolomics analysis showed consistent results, suggesting that the association of fatty acid β-oxidation disorders with PD is likely real (22). Energy is the basis of neural activity, and normal energy metabolism is required for cognition and memory (23). However, during pathological conditions such as neurodegeneration, hypoxia/ischemia, or post-traumatic brain injury, an insufficient energy supply stimulates the brain to signal the liver to induce fat metabolism to produce ketone bodies (24), which are delivered to the brain to ensure adequate energy (Figure S7). Lipid metabolism is affected in numerous neurodegenerative diseases (25,26). Therefore, increased fatty acid oxidation observed in the serum of PD-CI patients may be an attempt to reduce neuronal cell death related to low energy levels. Collectively, these results suggest that fatty acid oxidation may be an important pathogenetic mechanism of PD cognitive impairment, and metabolic intermediates of fatty acid oxidation may be useful diagnostic biomarkers to distinguish between PD-CI and PD-N.
LPC can reflect cell membrane integrity and is associated with neurodegenerative diseases (27,28). We found that PD-CI patients had significantly elevated serum levels of LPC compared to the PD-N group (Tables S4,S5, model 3). LPC is synthesized from phosphatidylcholine by the enzyme phospholipase A2 (PLA2), which catalyzes the hydrolysis of the sn-2 ester bond of glycerophospholipids to produce free fatty acids and LPC (29). The sn-2 position of phospholipids is mostly occupied by AA, and increased PLA2 activity can hydrolyze phospholipids of cell membranes to produce AA. In this study, LPC and AA levels were significantly increased, presumably due to the increased concentration and activity of PLA2. In addition, LPC can be produced by lecithin; cholesterol acyltransferase (LCAT) catalyzes the transfer of a fatty acyl residue from the sn-2 position of phosphatidylcholine to the 3-beta-hydroxyl group of cholesterol, resulting in the formation of a cholesteryl ester (30). Our proteomics study revealed an increase in serum LCAT activity, and it is speculated that this is also associated with the increase in LPC. Also, phosphatidylcholine can damage other enzymes during reacylation or deacylation. However, due to the lack of literature on the other enzymes’ roles of these other enzymes in PD and PD-like pathology, further enzymatic follow-up studies are needed.
PLA2 products, including LPCs, are involved in various downstream pathways; they induce coordinated signaling through a second messenger that promotes inflammatory mediator biosynthesis and inhibits neurotransmitter release (31). LPCs play a major role in inflammatory signaling, including the release of various cytokines (IL-1β, IL-6, chemokine ligand 2, chemokine ligand 4, and TNF alpha) (32-34). LPC, an endogenous inflammatory phospholipid that is produced under physiological and pathological conditions, has been implicated in the immunomodulatory function of glial cells in the CNS (35,36). LPC is a secondary product of S-adenosylmethionine (SAM)-dependent phosphatidylethanolamine (PTE) methylation and an effective cytotoxin involved in PD pathogenesis (37). SAM injection into the brain results in PD-like changes in rodents (38). In addition, 1-methyl-4-phenylpyridinium (MPP+), a drug that induces PD, increases LPC formation by stimulating the PTE methylation pathway (39). This illustrates the inhibitory effect of LPC on the dopaminergic system. Increased serum LPC in patients with PD-CI suggests that the inflammatory response is involved in PD cognitive dysfunction, while abnormally elevated lysolecithin further inhibits the dopamine system, aggravating cognitive impairment. Serum LCAT was considered a key target based on our multi-omics analysis. The relative levels of metabolites were inversely associated with cognition evaluation. LPC (18:1) was included in the regression equation, suggesting that it was a major contributor to cognition dysfunction.
Limitations and future directions
Although our study revealed the profile of differentially expressed proteins and metabolites in PD-CI, the sample size was relatively small. The associations between indicators and cognition status are worthy of further exploration. Potential confounders such as medications and body mass index should be considered more comprehensively. Nonetheless, measuring sphingolipid, lecithin, and long-chain fatty acid levels will help clarify their metabolism and potential PD mechanisms. Importantly, LPC (18:1) alone was able to predict PD with or without cognition impairment.
Acknowledgments
Thanks to Dr. Deqin Geng and Dr. Guiyun Cui, directors of neurology department of the Xuzhou Medical University Affiliated hospital, for their support in the process of clinical sample collection.
Funding: This work was supported by grants from the National Natural Science Foundation of China (No. 81971006) and the Jiangsu province’s Graduate research innovation project, China (KYCX18_2171, KYCX18_2193, KYCX19_2215).
Footnote
Reporting Checklist: The authors have completed the TRIPOD reporting checklist. Available at http://dx.doi.org/10.21037/atm-20-4583
Data Sharing Statement: Available at http://dx.doi.org/10.21037/atm-20-4583
Conflicts of Interest: All authors have completed the ICMJE uniform disclosure form (available at http://dx.doi.org/10.21037/atm-20-4583). The authors have no conflicts of interest to declare.
Ethical Statement: The authors are accountable for all aspects of the work in ensuring that questions related to the accuracy or integrity of any part of the work are appropriately investigated and resolved. The study was conducted in accordance with the Declaration of Helsinki (as revised in 2013). The study was approved by clinical research ethics committee of Xuzhou Medical University Affiliated Hospital (XYFY2017-KL047-01, XYFY2017-KL047-02, XYFY2017-KL047-03) and informed consent was taken from all individual participants.
Open Access Statement: This is an Open Access article distributed in accordance with the Creative Commons Attribution-NonCommercial-NoDerivs 4.0 International License (CC BY-NC-ND 4.0), which permits the non-commercial replication and distribution of the article with the strict proviso that no changes or edits are made and the original work is properly cited (including links to both the formal publication through the relevant DOI and the license). See: https://creativecommons.org/licenses/by-nc-nd/4.0/.
References
- Poewe W, Seppi K, Tanner CM, et al. Parkinson disease. Nat Rev Dis Primers 2017;3:17013. [Crossref] [PubMed]
- Pont-Sunyer C, Hotter A, Gaig C, et al. The onset of nonmotor symptoms in Parkinson’s disease (the ONSET PD study). Mov Disord 2015;30:229-37. [Crossref] [PubMed]
- Salat D, Noyce AJ, Schrag A, et al. Challenges of modifying disease progression in prediagnostic Parkinson’s disease. Lancet Neurol 2016;15:637-48. [Crossref] [PubMed]
- Trezzi JP, Galozzi S, Jaeger C, et al. Distinct metabolomic signature in cerebrospinal fluid in early parkinson’s disease. Mov Disord 2017;32:1401-8. [Crossref] [PubMed]
- Kingwell K. Zeroing in on neurodegenerative alpha-synuclein. Nat Rev Drug Discov 2017;16:371-3. [Crossref] [PubMed]
- Lleo A, Parnetti L, Belbin O, et al. Has the time arrived for cerebrospinal fluid biomarkers in psychiatric disorders? Clin Chim Acta 2019;491:81-4. [Crossref] [PubMed]
- Ferrer I. Proteomics and lipidomics in the human brain. Handb Clin Neurol 2018;150:285-302. [Crossref] [PubMed]
- Lewerenz J, Maher P. Chronic Glutamate Toxicity in Neurodegenerative Diseases-What is the Evidence? Front Neurosci 2015;9:469. [Crossref] [PubMed]
- Nagesh Babu G, Gupta M, Paliwal VK, et al. Serum metabolomics study in a group of Parkinson’s disease patients from northern India. Clin Chim Acta 2018;480:214-9. [Crossref] [PubMed]
- Olsson B, Lautner R, Andreasson U, et al. CSF and blood biomarkers for the diagnosis of Alzheimer’s disease: a systematic review and meta-analysis. Lancet Neurol 2016;15:673-84. [Crossref] [PubMed]
- Alves G, Lange J, Blennow K, et al. CSF Abeta42 predicts early-onset dementia in Parkinson disease. Neurology 2014;82:1784-90. [Crossref] [PubMed]
- Backstrom DC, Eriksson Domellof M, Linder J, et al. Cerebrospinal Fluid Patterns and the Risk of Future Dementia in Early, Incident Parkinson Disease. JAMA Neurol 2015;72:1175-82. [Crossref] [PubMed]
- Hughes AJ, Daniel SE, Blankson S, et al. A clinicopathologic study of 100 cases of Parkinson’s disease. Arch Neurol 1993;50:140-8. [Crossref] [PubMed]
- Robotti A, Natale M, Albo AG, et al. Acute-phase proteins investigation based on lectins affinity capture prior to 2-DE separation: application to serum from multiple sclerosis patients. Electrophoresis 2010;31:2882-93. [Crossref] [PubMed]
- Bouhaddani SE, Uh HW, Jongbloed G, et al. Integrating omics datasets with the OmicsPLS package. BMC Bioinformatics 2018;19:371. [Crossref] [PubMed]
- Young SA, Mina JG, Denny PW, et al. Sphingolipid and ceramide homeostasis: potential therapeutic targets. Biochem Res Int 2012;2012:248135 [Crossref] [PubMed]
- Spiegel S. Sphingosine 1-phosphate: a prototype of a new class of second messengers. J Leukoc Biol 1999;65:341-4. [Crossref] [PubMed]
- Maceyka M, Spiegel S. Sphingolipid metabolites in inflammatory disease. Nature 2014;510:58-67. [Crossref] [PubMed]
- Pyszko J, Strosznajder JB. Sphingosine kinase 1 and sphingosine-1-phosphate in oxidative stress evoked by 1-methyl-4-phenylpyridinium (MPP+) in human dopaminergic neuronal cells. Mol Neurobiol 2014;50:38-48. [Crossref] [PubMed]
- He X, Huang Y, Li B, et al. Deregulation of sphingolipid metabolism in Alzheimer’s disease. Neurobiol Aging 2010;31:398-408. [Crossref] [PubMed]
- Tein I. Disorders of fatty acid oxidation. Handb Clin Neurol 2013;113:1675-88. [Crossref] [PubMed]
- Burte F, Houghton D, Lowes H, et al. metabolic profiling of Parkinson’s disease and mild cognitive impairment. Mov Disord 2017;32:927-32. [Crossref] [PubMed]
- McDougall M, Choi J, Magnusson K, et al. Chronic vitamin E deficiency impairs cognitive function in adult zebrafish via dysregulation of brain lipids and energy metabolism. Free Radic Biol Med 2017;112:308-17. [Crossref] [PubMed]
- Prins ML. Cerebral metabolic adaptation and ketone metabolism after brain injury. J Cereb Blood Flow Metab 2008;28:1-16. [Crossref] [PubMed]
- Zarrouk A, Debbabi M, Bezine M, et al. Lipid Biomarkers in Alzheimer’s Disease. Curr Alzheimer Res 2018;15:303-12. [Crossref] [PubMed]
- Aditi K, Shakarad MN, Agrawal N. Altered lipid metabolism in Drosophila model of Huntington’s disease. Sci Rep 2016;6:31411. [Crossref] [PubMed]
- Cui Y, Liu X, Wang M, et al. Lysophosphatidylcholine and amide as metabolites for detecting alzheimer disease using ultrahigh-performance liquid chromatography-quadrupole time-of-flight mass spectrometry-based metabonomics. J Neuropathol Exp Neurol 2014;73:954-63. [Crossref] [PubMed]
- Mapstone M, Cheema AK, Fiandaca MS, et al. Plasma phospholipids identify antecedent memory impairment in older adults. Nat Med 2014;20:415-8. [Crossref] [PubMed]
- Murakami M, Kudo I. Phospholipase A2. J Biochem 2002;131:285-92. [Crossref] [PubMed]
- Wells IC, Peitzmeier G, Vincent JK. Lecithin: cholesterol acyltransferase and lysolecithin in coronary atherosclerosis. Exp Mol Pathol 1986;45:303-10. [Crossref] [PubMed]
- Farooqui AA. Lipid mediators in the neural cell nucleus: their metabolism, signaling, and association with neurological disorders. Neuroscientist 2009;15:392-407. [Crossref] [PubMed]
- Cunningham TJ, Yao L, Lucena A. Product inhibition of secreted phospholipase A2 may explain lysophosphatidylcholines’ unexpected therapeutic properties. J Inflamm (Lond) 2008;5:17. [Crossref] [PubMed]
- Liu-Wu Y, Hurt-Camejo E, Wiklund O. Lysophosphatidylcholine induces the production of IL-1beta by human monocytes. Atherosclerosis 1998;137:351-7. [Crossref] [PubMed]
- Goncalves I, Edsfeldt A, Ko NY, et al. Evidence supporting a key role of Lp-PLA2-generated lysophosphatidylcholine in human atherosclerotic plaque inflammation. Arterioscler Thromb Vasc Biol 2012;32:1505-12. [Crossref] [PubMed]
- Jeong H, Kim YH, Lee Y, et al. TRPM2 contributes to LPC-induced intracellular Ca(2+) influx and microglial activation. Biochem Biophys Res Commun 2017;485:301-6. [Crossref] [PubMed]
- Sheikh AM, Nagai A, Ryu JK, et al. Lysophosphatidylcholine induces glial cell activation: role of rho kinase. Glia 2009;57:898-907. [Crossref] [PubMed]
- Crowell BG Jr, Benson R, Shockley D, et al. S-adenosyl-L-methionine decreases motor activity in the rat: similarity to Parkinson’s disease-like symptoms. Behav Neural Biol 1993;59:186-93. [Crossref] [PubMed]
- Lee ES, Soliman KF, Charlton CG. Lysophosphatidylcholine decreases locomotor activities and dopamine turnover rate in rats. Neurotoxicology 2005;26:27-38. [Crossref] [PubMed]
- Lee ES, Charlton CG. 1-Methyl-4-phenyl-pyridinium increases S-adenosyl-L-methionine dependent phospholipid methylation. Pharmacol Biochem Behav 2001;70:105-14. [Crossref] [PubMed]