A predictive model for postthrombotic syndrome in proximal deep vein thrombosis patients
Introduction
Postthrombotic syndrome (PTS) is the most common long-term complication of deep vein thrombosis (DVT). The incidence of PTS ranges from 20% to 50% in DVT patients (1,2). Surgical treatment of PTS is attempted when conservative treatment fails. However, there is a lack of evidence to support that the existing treatment options improve the quality of life and costs of care of patients with PTS (3-8). Therefore, prevention is a key measure for managing PTS. Due to the development of endovascular techniques, a large proportion of proximal DVT patients undergo endovascular therapy to prevent PTS development (9,10), and proximal DVT accounts for the vast majority of hospitalized DVT patients who undergo endovascular therapy (11).
Predictive models have been accepted as reliable tools to quantify risk for prognostic analyses. Although numerous predictors have been identified as risk factors for PTS (12-15), confirming the combined factors to develop a prediction model that predicts the development of PTS after DVT remains a challenge. Because of the high proportion of proximal DVT among hospitalized DVT patients, we believe that the derivation of an easy-to-use PTS predictive model for patients with proximal DVT who are hospitalized may be beneficial in daily clinical practice. Furthermore, we externally validated our model and existing PTS prediction rules to evaluate the calibration, discrimination and clinical utility of these predictive models. We present the following article in accordance with the STROBE reporting checklist (available at http://dx.doi.org/10.21037/atm-20-3239).
Methods
Sampling design
This study was a retrospective, single-center, case-control study. The data used in our model were retrospectively collected in a prospective registry database. This registry was launched in June 2016 and prospectively collected consecutive patients who were diagnosed with DVT at the Vascular Center of Shanghai Jiaotong University (Shanghai Ninth People’s Hospital Affiliated to Shanghai Jiao Tong University School of Medicine). The study was conducted in accordance with the Declaration of Helsinki (as revised in 2013). The study was approved by the registration number of Medical Ethics Committee of Shanghai Ninth People’s Hospital (No.: SH9H-2020-T314-2) and individual consent for this retrospective analysis was waived. The DVT registry at the Vascular Center of Shanghai Jiaotong University collected the demographics of patients, treatment details, preoperative and postoperative laboratory tests, and postdischarge follow-ups, including telephone follow-up and/or outpatient follow-up. The missing data in the database was excluded.
The inclusion criteria were adult inpatients with a first diagnosis of proximal DVT. Proximal DVT was defined as a thrombus involving the iliac and/or common femoral veins, with or without extension to the inferior vena cava and with or without concomitant PE. There were no exclusion criteria. The included cases were randomly divided into derivation and validation sets according to a 7:3 ratio. The presence of PTS was defined as a Villalta score of ≥5 (16). A Villalta score at 6 months post-DVT was used to assess the incidence of PTS, as determined by a medical doctor.
Predictor variables
A standardized data form was created to retrieve all relevant information on demographics [sex, age, and body mass index (BMI)], potential DVT risk factors (hospitalization for surgery, active cancer, trauma/fracture, nursing home confinement, hospitalization due to nonsurgical illness, and idiopathic DVT), comorbidities [hypertension, heart disease, diabetes, neurological disease, prior varicose vein, and pulmonary embolism (PE)], classification of DVT (limbs of DVT, acute/chronic DVT), and signs or symptoms of DVT at baseline (pain, cramps, heaviness, pruritus, paresthesia, edema, skin induration, hyperpigmentation, venous ectasia, redness, pain during calf compression, venous ulcers, and the number of signs and symptoms). There were 30 variables in the study, and 18 candidate predictors were finally analyzed. For instance, 10 venous signs and symptoms were combined to a candidate predictor of “number of signs and symptoms”. The selection of candidate predictors was based on previous studies, and we also considered clinically observed variables with potential impact. The potential DVT risk factors mentioned above are defined as factors that occurred within 3 months before the onset of DVT. Acute DVT is defined from onset to treatment <14 days, and chronic DVT is defined from onset to treatment ≥14 days. As there were too many signs or symptoms of DVT at baseline, we used the number of signs and symptoms as a variable.
Sample size
According to the literature, the incidence of PTS is 20–50% (1,2); thus, we estimated the incidence of PTS to be 35% for the sample size calculation. We first selected 100 samples and found that 7 variables were significant after univariate analysis. We followed the suggested sample size calculation for logistic regression, where p is the smallest of the proportions of negative or positive cases in the population and k is the number of independent variables; then, the minimum number of cases to include is N=10 k/p. The minimum number of cases required of the derivation set is therefore N=10 * 7/0.35 = 200.
Statistical analysis
All model creation steps were based only on the derivation set. First step, univariate logistic regression was used to evaluate the factors associated with PTS. Second step, a multivariate logistic regression model was performed with selected variables that were significant in the univariate analysis (P<0.05). The final multivariable model was constructed by removing variables with a P value >0.1. The odds ratio (OR) for each independent variable was determined with a 95% confidence interval (CI). To facilitate the ease of use in the clinical setting, a nomogram was generated on the basis of the final predictive model.
We externally validated our predictive model, the SOX-PTS predictive model, and the SWITCO-PTS predictive model in our set. We calculated the score or the predicted probabilities based on the score and the model coefficients presented in the original publication. A receiver operating characteristic (ROC) curve of each predictive model was generated using a validation set, and predictive discriminations were evaluated by calculating the area under the curve (AUC) (17). The overall accuracy and calibration of each predictive model were visualized by calibration curves that compared the predicted versus actual probabilities, including a bias correction for overfitting (18). A decision curve analysis (DCA) was performed to assess the clinical utility of using each of these 3 predictive models to guide the decision (19,20). Data were analyzed with R version 3.5.3.
Results
In total, 349 consecutive patients with proximal DVT were registered in our registry database from June 2016 to June 2018. We excluded patients without 6 months of follow-up (n=32) and those who died before the 6-month visit (N=17). Finally, 300 patients were enrolled in this study. A total of 210 patients were used as the derivation set and 90 as the validation set. The characteristics of the patients in the derivation set and validation set are presented in Table 1. Among those in the derivation set, 126 patients were diagnosed with PTS after 6 months, accounting for 42.0%. Participation at each stage is shown in a flow diagram (Figure 1).
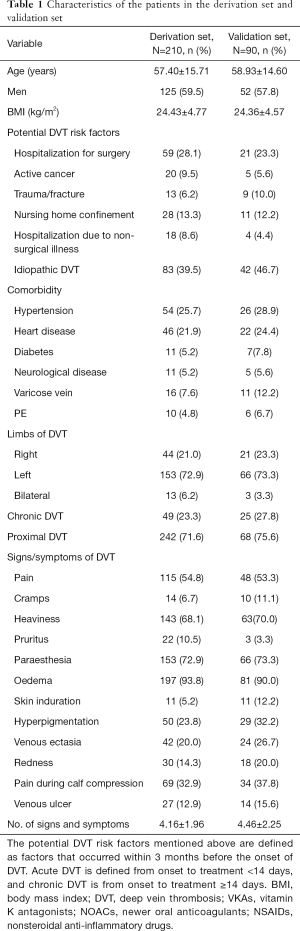
Full table
Derivation of the predictive model
Table 2 presents the characteristics of the derivation set. The univariable associations between PTS and potential predictors are listed in Table 2. Of the 18 candidate predictors, 6 were significantly associated with PTS in our final multivariable model (Table 3). The independent predictors of PTS based on the derivation set were the number of signs and symptoms (OR 1.33, 95% CI: 1.17 to 1.53, P<0.001), male sex (OR 1.79, 95% CI: 1.07 to 3.06, P=0.028), varicose vein history (OR 3.02, 95% CI: 1.04 to 7.60, P<0.001), BMI (OR 1.06, 95% CI: 1.00 to 1.12, P=0.052), and chronic DVT (OR 2.66, 95% CI: 1.49 to 4.79, P<0.001). A nomogram incorporating the 5 independent predictors in the derivation set was established (Figure 2).
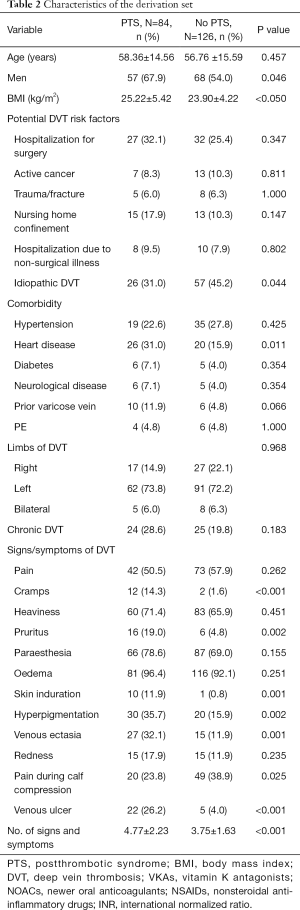
Full table
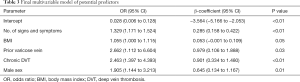
Full table
External validation and comparison with other models
The validation set was used in the external validation of our predictive model, the SOX-PTS predictive model, and the SWITCO-PTS predictive model. The discriminative ability of the models is represented as the AUC. The AUC was highest for our predictive model (AUC 0.724) (Figure 3); the AUC for the SOX-PTS and SWITCO-PTS predictive models was 0.606 and 0.579, respectively. The difference between AUC of our model and SOX-PTS model was not statistically significant (Z=1.707, P=0.088), and the difference between AUC of our model and SWITCO-PTS model was statistically significant (Z=2.556, P=0.011). The calibration curves are presented in Figure 3, showing good overall agreement between the predicted and observed risk of PTS in our predictive model, whereas the risk of PTS was systematically overestimated in both the SOX-PTS predictive model and SWITCO-PTS predictive model (Figure 4). The clinical utility of our predictive model, the SOX-PTS predictive model, and the SWITCO-PTS predictive model is presented in a DCA (Figure 5). The DCA graphically shows the clinical usefulness of each model based on a continuum of potential thresholds for PTS risk (x axis) and the net benefit of using the model to risk stratify patients (y axis) relative to assuming that no patient will have a PTS. In this analysis, our predictive model was associated with the highest net benefit within threshold probabilities of 20%-95% for the prediction of PTS.
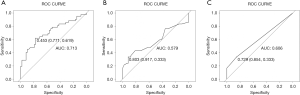
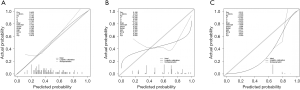
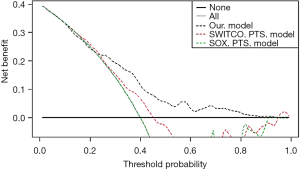
Discussion
PTS is a common, late complication of DVT that reduces the quality of life and increases the financial burden of patients (1-3). Due to the lack of effective prevention and treatment, PTS occurs in 20% to 50% of DVT patients, even with effective anticoagulation (1,2). Previous studies have revealed a number of risk factors for PTS (12-15,21), but identifying patients at a high risk of PTS by a single risk factor is difficult. Several previous models of PTS have attempted to predict the development of PTS by combining risk factors (12-14). However, most of these studies adopted cohorts from randomized controlled trials, excluding young people and chronic cases, among others, populations that are common in clinical practice. Therefore, whether these predictive models have practical value and repeatability in clinical work is debatable. Additionally, with recent advances in endovascular technology, many endovascular interventions have been attempted in DVT patients, especially patients with proximal DVT, to reduce the development of PTS (9-11). Previous randomized controlled trials have revealed that endovascular therapy is associated with a PTS reduction compared with anticoagulation alone. Accordingly, there is a great demand for surgical decision-support tools in clinical work for patients with DVT who receive endovascular therapy. Our predictive model was derived by using a registry database of hospitalized proximal DVT patients. Our model was established by easily available variables such as information on demographics, potential DVT risk factors, comorbidities, classification, and signs or symptoms of DVT at baseline.
Factors such as male sex, chronic DVT, BMI, signs and symptoms at baseline, and prior varicose vein were incorporated into our predictive model as independent risk factors. These variables were also revealed to be independent risk factors in a previous analysis. In addition, previous PTS predictive models did not consider the risk factors of DVT included in our analysis. These variables are easily available in the electronic medical records of hospitalized patients, enhancing the ease of use of our predictive model.
The discrimination of our PTS predictive model, as highlighted by the AUC value, was higher than those of the SOX-PTS predictive model and the SWITCO-PTS predictive model, which were both derived from data from a prospective cohort. The calibration curve demonstrated very good agreement between the predicted and actual risk of PTS, which assures the repeatability and reliability of our PTS predictive model. At the same time, the calibration curves of previous predictive models showed either a systematic overestimation or systematic underestimation. Moreover, we used DCA to evaluate the clinical utility of the predictive models. In DCA, the net benefit is determined by calculating the difference between the expected benefit and the expected harm associated with each predictive model (19,20). The DCA for our validation set showed that the net benefit was maximized with threshold probabilities of 0–25% by the “predict all” approach; with threshold probabilities of 25–90%.
Our study may support clinical decision making. Our model may offer personalized patient PTS risk estimates and facilitate clinical counseling for both patients and doctors. Identifying subgroups of DVT patients at different risk for PTS might have an impact on treatment or care options. Furthermore, this predictive model may also provide information for patient stratification in the design of clinical studies, gaining better equivalence between study arms.
As with any observational population-based study, our study has some limitations. First, our model was derived from data for hospitalized DVT patients and did not include outpatient DVT patients. Moreover, our institution is a famous venous center in China, and we received many DVT patients who were referred from local institutions, and these patients may have had severe DVT. Therefore, when applying this model in specific centers with different DVT populations, some inconsistencies between the predicted and actual PTS risks may be observed. Finally, future predictive models with better methodologies are needed to assist in clinical decision making and to guide future research.
Acknowledgments
The authors wish to thank Meirong Xi for her important contribution to data collection.
Funding: This work was supported by National Natural Science Foundation of China (81601621, 81701842, 81570432, 81600205), Shanghai Municipal Health Bureau Project (202040434), Shanghai Science and Technology Innovation Action Plan (20Y11909600), Clinical Research Program of Shanghai Ninth People’s Hospital (JYLJ202010), Shanghai Ninth People’s Hospital Nursing Fund Project (JYHL2020MS01) and Shanghai Jiao Tong University School of Medicine Doctoral Innovation Fund (BXJ201935).
Footnote
Reporting Checklist: The authors have completed the STROBE reporting checklist. Available at http://dx.doi.org/10.21037/atm-20-3239
Data Sharing Statement: Available at http://dx.doi.org/10.21037/atm-20-3239
Conflicts of Interest: All authors have completed the ICMJE uniform disclosure form (available at http://dx.doi.org/10.21037/atm-20-3239). The authors have no conflicts of interest to declare.
Ethical Statement: The authors are accountable for all aspects of the work in ensuring that questions related to the accuracy or integrity of any part of the work are appropriately investigated and resolved. The study was conducted in accordance with the Declaration of Helsinki (as revised in 2013). The study was approved by the registration number of Medical Ethics Committee of Shanghai Ninth People’s Hospital (No.: SH9H-2020-T314-2) and individual consent for this retrospective analysis was waived.
Open Access Statement: This is an Open Access article distributed in accordance with the Creative Commons Attribution-NonCommercial-NoDerivs 4.0 International License (CC BY-NC-ND 4.0), which permits the non-commercial replication and distribution of the article with the strict proviso that no changes or edits are made and the original work is properly cited (including links to both the formal publication through the relevant DOI and the license). See: https://creativecommons.org/licenses/by-nc-nd/4.0/.
References
- Kahn SR, Shrier I, Julian JA, et al. Determinants and time course of the postthrombotic syndrome after acute deep venous thrombosis. Ann Intern Med 2008;149:698-707. [Crossref] [PubMed]
- Prandoni P, Lensing AW, Cogo A, et al. The long-term clinical course of acute deep venous thrombosis. Ann Intern Med 1996;125:1-7. [Crossref] [PubMed]
- Kahn SR, Comerota AJ, Cushman M, et al. The postthrombotic syndrome: evidence-based prevention, diagnosis, and treatment strategies: a scientific statement from the American Heart Association. Circulation 2014;130:1636-61. [Crossref] [PubMed]
- Raju S. Best management options for chronic iliac vein stenosis and occlusion. J Vasc Surg 2013;57:1163-9. [Crossref] [PubMed]
- Cohen JM, Akl EA, Kahn SR. Pharmacologic and compression therapies for postthrombotic syndrome: a systematic review of randomized controlled trials. Chest 2012;141:308-20. [Crossref] [PubMed]
- Morling JR, Broderick C, Yeoh SE, et al. Rutosides for treatment of post-thrombotic syndrome. Cochrane Database Syst Rev 2018;11:CD005625 [PubMed]
- Kearon C, Akl EA, Ornelas J, et al. Antithrombotic therapy for VTE disease: 7. CHEST guideline and expert panel report. Chest 2016;149:315-52. [Crossref] [PubMed]
- Qiu P, Zha B, Xu A, et al. Systematic Review and Meta-Analysis of Iliofemoral Stenting for Post-thrombotic Syndrome. Eur J Vasc Endovasc Surg 2019;57:407-416. [Crossref] [PubMed]
- Enden T, Haig Y, Kløw NE, et al. Long-term outcome after additional catheter-directed thrombolysis versus standard treatment for acute iliofemoral deep vein thrombosis (the CaVenT study): a randomised controlled trial. Lancet 2012;379:31-8. [Crossref] [PubMed]
- Garcia MJ, Lookstein R, Malhotra R, et al. Endovascular management of deep vein thrombosis with rheolytic thrombectomy: final report of the prospective multicenter PEARL (Peripheral Use of AngioJet Rheolytic Thrombectomy with a Variety of Catheter Lengths) registry. J Vasc Interv Radiol 2015;26:777-85. [Crossref] [PubMed]
- Nyamekye I, Merker L. Management of proximal deep vein thrombosis. Phlebology 2012;27:61-72. [Crossref] [PubMed]
- Rabinovich A, Ducruet T, Kahn SR, et al. Development of a clinical prediction model for the postthrombotic syndrome in a prospective cohort of patients with proximal deep vein thrombosis. J Thromb Haemost 2018;16:262-70. [Crossref] [PubMed]
- Amin EE, van Kuijk SM, Joore MA, et al. Development and validation of a practical two-step prediction model and clinical risk score for postthrombotic syndrome. Thromb Haemost 2018;118:1242-9. [Crossref] [PubMed]
- Méan M, Limacher A, Alatri A, et al. Derivation and validation of a prediction model for risk stratification of post-thrombotic syndrome in elderly patients with a first deep vein thrombosis. Thromb Haemost 2018;118:1419-27. [Crossref] [PubMed]
- Rabinovich A, Kahn SR. The postthrombotic syndrome: current evidence and future challenges. J Thromb Haemost 2017;15:230-41. [Crossref] [PubMed]
- Villalta SB, Bagatella P, Piccioli A, et al. Assessment of validity and reproducibility of a clinical scale for the post-thrombotic syndrome. Haemostasis 1994;24:158a.
- Harrell FE, Lee KL, Mark DB. Multivariable prognostic models: issues in developing models, evaluating assumptions and adequacy, and measuring and reducing errors. Stat Med 1996;15:361-87. [Crossref] [PubMed]
- Steyerberg EW, Vickers AJ, Cook NR, et al. Assessing the performance of prediction models: a framework for some traditional and novel measures. Epidemiology 2010;21:128-38. [Crossref] [PubMed]
- Vickers AJ, Elkin EB. Decision curve analysis: a novel method for evaluating prediction models. Med Decis Making 2006;26:565-74. [Crossref] [PubMed]
- Fitzgerald M, Saville BR, Lewis RJ. Decision curve analysis. JAMA 2015;313:409-10. [Crossref] [PubMed]
- Rabinovich A, Cohen JM, Prandoni P, et al. Association between thrombophilia and the post-thrombotic syndrome: a systematic review and meta-analysis. J Thromb Haemost 2014;12:14-23. [Crossref] [PubMed]