Aberrant lactate dehydrogenase A signaling contributes metabolic signatures in pancreatic cancer
Introduction
Pancreatic cancer (PC) is currently one of the 10 most commonly diagnosed cancer types in the United States (US), and the death rate of PC is 8%, making it the fourth most fatal cancer after lung, prostate, and colon cancer in men (1,2). The 5-year survival rate of patients with this type of cancer is less than 5% due to presentation at late stages and the absence of early detection and treatment (3). Hence, it is important to explore possible early screening methods and therapies for PC. Many studies have focused on this topic in recent years, and liquid biopsy is a promising field with great value for application.
Liquid biopsy has the advantages of low invasive sampling, operability, fast turnaround time, and suitability for long-term monitoring. Among biomarkers used in liquid biopsy, metabolomics has the advantages of early diagnosis and treatment development. Metabolites play an important role in human cells and organs and are the key components in maintaining physiological function and intercellular signal transduction, which can reflect the state of the body directly and accurately, while genomics and proteomics are more about what may happen in organisms (4). Therefore, the usage of metabolism is viewed as one of the next-generation methodologies of liquid biopsy after genomics (5-7). Tumors have a special metabolism that leads to metabolite alterations in the tumor microenvironment or even bodily status.
Aerobic glycolysis is a metabolic phenomenon that always happens in cancer due to the increased need for energy consumption. Although aerobic glycolysis creates fewer adenosine triphosphates (ATPs) than normal glucose metabolism, cancer cells prefer to use the glycolysis pathway, which is used for energy supply only under anoxic conditions in normal tissue. This progression is known as the “Warburg effect”, and leads to the accumulation of lactic acid (8,9).
Although glycolysis has been explored in many types of cancers, metabolic analysis in serum combined with genetic evidence in PC has not been reported. Here, we present the effect of glycolysis in PC on both sides. We analyzed PC and normal tissue gene expression data from Gene Expression Omnibus (GEO) data repositories and verified the differential expression of glycolysis-related genes. Then, The Cancer Genome Atlas (TCGA) dataset was used to analyze the correlation of these genes with the survival time and hazard. Then, serum metabolites were detected to determine the alterations between cancer patients and healthy controls. Glycolysis-related gene expression status and serum metabolite variety in PC patients were combined to explain the relationship between these 2 aspects. The overall workflow of this article is shown in Figure 1. Our results revealed some biomarkers that can promote earlier diagnosis and monitoring of curative effects. We present the following article in accordance with the MDAR checklist (available at http://dx.doi.org/10.21037/atm-21-295).
Methods
Sample collection
Venous fasting blood samples of the healthy cohort (n=70) and PC cohort (n=36) were collected at the Tianjin Medical University Cancer Institute and Hospital. All healthy controls included in this study were older than 18 years and healthy upon medical examination. Cancer samples were taken from hospitalized patients before operation, and PC status and clinical stages were confirmed by postoperative pathology results.
Blood samples were drawn into serum vacuum tubes and kept at room temperature for 30 minutes before centrifugation (1,200 ×g, 10 minutes), and serum samples were collected into tubes, stored at 80 °C, and shipped in dry ice. The usage of serum samples in this study was reviewed and approved by the Ethics Committee of Tianjin Medical University Cancer Institute and Hospital (Scientific Ethical Approval No. bc2020101) and informed consent was taken from all the patients. The study was conducted in accordance with the Declaration of Helsinki (as revised in 2013).
Gene expression and clinical data of PC patients
The microarray-based gene expression data of 36 tumor samples and 16 normal samples were obtained from GEO datasets (GSE16515; n=52). Gene expression and detailed clinical data for 181 PC (PAAD) patients were obtained from TCGA (https://cancergenome.nih.gov/abouttcga/overview). The overall survival (OS) and other PC-related clinical data for each sample were all available in TCGA.
Statistical analyses
Differentially expressed genes (DEGs) were defined as more than 2-fold change in cancer patients (both upregulation and downregulation). The P values between cancer and normal cohorts were less than or equal to 0.05, and pathway enrichment analysis was performed with these DEGs.
Kaplan-Meier plots were used to estimate the correlation between OS and gene expression levels. The median expression value of each gene was selected as the grouping criterion, and cancer patients were divided into high-expression and low-expression groups. A significant correlation was judged by P values. Univariate and multivariate Cox regression analyses were all shown in terms of hazard ratios (HRs), estimated 95% confidence intervals (CIs), and P values; P values in the multivariable Cox proportional hazards model were the key indices to identify independent predictors.
The receiver operating characteristic curve (ROC) analysis for the combination of 4 metabolites was calculated using linear regression. The calculated formula was: score (all) =14.143−1.896× (concentrationglucose) −7.812× (concentrationcitrate) −2.818× (concentrationlactate) +68.845× (concentrationpyruvate).
All statistical analyses and data plotting were performed using R software (http:///www.r-project.org). Two-tailed P values were obtained, and a significant difference was defined with a cutoff value of 0.05.
Serum metabolite detection
Serum samples were prepared and detected according to Bruker in vitro diagnostic research standard operating procedures (IVDr SOPs) at ProteinT Biotechnology Co., Ltd. (Tianjin, China). Briefly, samples were thawed at room temperature, 400 µL of serum samples and 400 µL of buffer (phosphate buffer pH 7.4 containing TSP-d4, Bruker) were fully mixed, and 600 µL of the mixture was transferred to a 5-mm nuclear magnetic resonance (NMR) tube pending analysis. The detection was performed on a 600 MHz NMR Avance III HD spectrometer equipped with a BBI probehead and SampleJet autosampler, which was regulated at 6 °C during detection (Bruker Biospin, Rheinstetten, Germany). Before acquisition, automatic tuning and shimming were performed on every sample. The free induction decays (FIDs) were presented as spectra after Fourier transformation, and automatic phase and baseline correction were performed in Topspin software as Bruker IVDr. The concentrations of metabolites were expressed as mmol/L.
Immunohistochemical analysis
Antibody against LDHA (HuaAn Biotechnology, Huangzhou, China) was used to perform immunohistochemical (IHC) staining in the PC tissues and paired adjacent normal tissues. Briefly, tissue sections were heated at 60 °C for 25 minutes, de-paraffinized in xylene, and rehydrated using graded alcohol. Hydrogen peroxide (0.03%) containing sodium azide was used to block endogenous peroxidase before primary antibody incubation. The sections were then incubated with streptavidin-HRP (streptavidin conjugated to horseradish peroxidase in PBS containing an anti-microbial agent) and 3,3’-diaminobenzidine (DAB)-substrate-chromagen respectively. Positive findings of the IHC staining were observed as a brown coloration in the tissue sections under a light microscope.
Results
Glycolysis-related differential gene expression in PC
We analyzed the GEO dataset of PC (GSE16515: 36 cancer samples, 16 normal samples) (10-12) and identified 794 DEGs (fold change (cancer/normal) >2 or <0.5, P<0.05). A total of 186 genes were downregulated, while 608 genes were upregulated in cancer patients (Figure 2A). Then, we performed pathway enrichment analysis and noticed that 9 genes were enriched in the glycolysis pathway: phosphofructokinase (PFKP), pyruvate kinase (PKM), fructose bisphosphate aldolase A (ALDOA), glyceraldehyde-3-phosphate dehydrogenase (GAPDH), phosphoglycerate kinase (PGK1), lactate dehydrogenase-A (LDHA), bisphosphoglycerate mutase (BPGM), enolase 2 (ENO2), and alcohol dehydrogenase (ADH1B). Except for ADH1B, the remaining 8 genes were all overexpressed in cancer (Figure 2B,C,D).
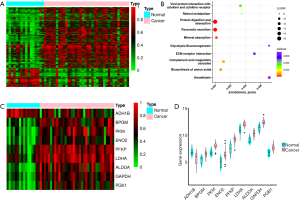
Glycolysis-related genes are predictive of prognostic potential
To determine the relationship between the expression of these 9 genes and survival time in the glycolysis pathway, we analyzed the TCGA PC dataset (181 PC samples). The median expression value of each gene was selected as the grouping criterion, and cancer patients were divided into high-expression and low-expression groups. Kaplan-Meier plots were generated, resulting in a significant correlation between the high expression of 4 genes and lower OS: LDHA (P<0.0001), ALDOA (P=0.012), GAPDH (P=0.033), and PKM (P=0.012), as shown in Figure 3. The expression of the other 5 genes was not significantly related to survival probability, as shown in Figure S1.
Moreover, we chose the clinical data in TCGA that are associated with PC risk, as reported (13), such as gender, age, family history of cancer, and history of diabetes, among others. We performed univariate and multivariate Cox regression analyses on these clinical data and 4 survival-related genes in glycolysis (LDHA, ALDOA, GAPDH, PKM). In univariate Cox analysis, age, neoplasm histologic grade, primary therapy outcome success, and the expression of LDHA and PKM were significantly associated with survival time. After multivariate regression analyses, age, primary therapy outcome success, and the expression of LDHA remained remarkable, while neoplasm histologic grade and the expression of PKM were no longer significant (as shown in Table 1). These results identified LDHA expression as an independent predictor of OS in PC patients.
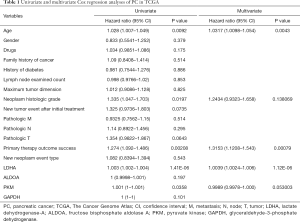
Full table
Serum metabolites may reflect glycolysis status in PC
We tried to determine the metabolite changing characteristics in the serum of PC participants, which would lead to the possibility of early detection or monitoring of cancer recurrence. The detection of metabolites was performed using an NMR spectrometer (Bruker Biospin, Rheinstetten, Germany) in healthy and cancer cohorts. We identified 39 metabolites in serum. The detection values of some metabolites were below the limit of detection (LOD) in more than 80% of the samples, so we used the remaining 24 metabolites for the following analysis (shown in Table S1). Figure 4A shows the profiling variation of these metabolites, and most were upregulated in cancer. Then, we performed principal component analysis (PCA) on the dataset, and the results showed that the normal and cancer serum samples had distinctive metabolomic profiles (Figure 4B).
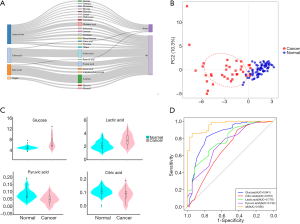
Glycolysis-related metabolite concentrations showed upregulation of glucose and lactic acid and downregulation of pyruvic acid and citric acid in cancer patients (Figure 4C). We performed ROC analysis on these 4 metabolites alone and their combinations in cancer and healthy cohorts (Figure 4D). The areas under the curve (AUCs) of these metabolites were 0.670 (citric acid), 0.732 (pyruvic acid), 0.778 (lactic acid), 0.841 (glucose), and was best for the combination score of 0.956 (all). The calculated formula of the combination score was: score (all) =14.143−1.896× (concentrationglucose) −7.812× (concentrationcitrate) −2.818× (concentrationlactate) +68.845× (concentrationpyruvate). The score was significantly lower (with more negative values) in cancer patients compared with the healthy cohort (shown in Table 2).
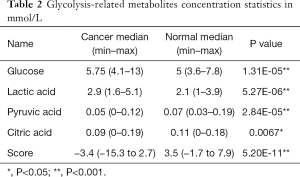
Full table
LDHA overexpression and metabolites alternation in PC specimens
We chose another 3 PC patients, and performed IHC staining in the PC and adjacent normal tissues with LDHA antibody. The clinical parameters and contrast-enhanced computed tomography (CT) scans are shown in Figure 5A. Briefly, pre-operative CT scans showed the tumor location and rough tumor size (the exact size of tumors were measured post-operatively); intraoperatively, the frozen section showed that the mass had features of a tumor of the pancreas with negative resection margins; the CT scans after operation confirmed no cancer was remaining; the PC diagnosis was confirmed by histopathology and IHC after operation. The clinical parameters of these 3 participants are shown in Table 3.
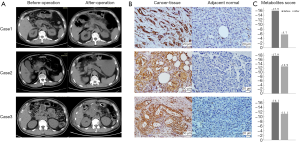
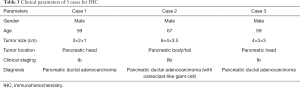
Full table
The results of IHC indicated that LDHA was strongly overexpressed in all 3 cancer cases compared with their adjacent normal tissues (Figure 5B). In addition, we collected the serum samples of these 3 participants before and 1 week after their operations, NMR metabolite detection was also performed on these paired samples. The metabolites scores of preoperative samples were all less than −15, which could be grouped in the cancer cohort, and the scores showed some improvement in postoperative samples (Figure 5C).
Discussion
Aerobic glycolysis and the Warburg effect have been well studied in recent decades, and their high rates in PC create a hypoxic and nutrient-poor microenvironment (14,15). Glycolysis in PC regulates vigorous tumor growth, invasion, and migration via glycolytic enzymes (16,17). The overexpression of glycolysis enzymes has been reported in many cancer types (18,19). The gene PFKFB4 was suggested to be a promoter of cancer metastasis in breast (20) and bladder cancer (21). Glycolysis induced by TP53 and apoptosis regulator (TIGAR) is associated with cancer progression and poor prognosis in lung cancer (22,23). The overexpression of ALDOA was also associated with poorer prognosis and possibility of metastasis (24), and the silencing of ALDOA in PAN-1 cells decreased proliferation and metastasis, which indicated that ALDOA may become a drug target (25,26). In this article, we found that 9 genes in glycolysis were overexpressed in PC, 4 of which are associated with patient OS. After multivariate Cox regression analyses with PC risk factors, LDHA was identified as an independent predictor of PC.
In glycolysis, LDHA is a very important rate-limiting enzyme. It is the final step in the glycolysis pathway, catalyzing the formation of lactic acid from pyruvic acid, and has been reported to be overexpressed in many cancers; a decrease in LDHA suppresses proliferation and leads to cell death (27,28). There have been more studies on inhibiting LDHA than ALDOA (29,30). Small-molecule inhibitors, such as FX11 [3-dihydroxy-6-methyl-7-(phenylmethyl)-4-propylnaphthalene-1-carboxylic acid] and some novel LDHA inhibitors, were used alone or combined with gemcitabine in cell and mouse models, and the results showed that LDHA is a promising therapeutic target for cancer energy metabolism (31-33).
We tested the serum metabolites of cancer patients using NMR spectrometry and found that pyruvic acid and lactic acid were significantly downregulated and upregulated in cancer patients, respectively, which coincided with the results of LDHA overexpression (Figure 6). Furthermore, we found that citric acid, the first metabolite in tricarboxylic acid (TCA) that is converted from pyruvic acid, was simultaneously downregulated. At the beginning of glycolysis, the glucose in the serum of our cancer participants was remarkably upregulated. Serum glucose concentration was positively associated with PC (34), and there were reports that high blood glucose levels of PC patients were also associated with poorer outcomes and increased mortality hazard (35,36). The ROC results using these glycolysis-related metabolites indicated that these serum metabolites could be good identifiers of PC; the combinative metabolites score was calculated using lactic acid, pyruvic acid, citric acid, and glucose, and was shown to be significantly higher in healthy cohorts, which may indicate potential as biomarkers for monitoring the curative effects of LDHA inhibitors.
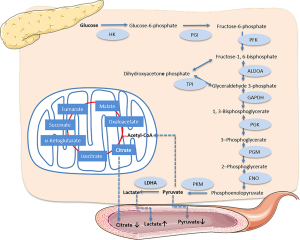
To validate LDHA overexpression and the metabolite results of our study, we collected the surgical tissue sections of another 3 hospitalized patients to perform IHC staining using an LDHA antibody. The IHC results showed that LDHA was significantly overexpressed in 3 cancer tissues when compared to their counterparts. We also tested the serum metabolites of these 3 patients before and after surgery. The metabolite scores were calculated, and the scores before operation were all less than −15, which was less than the minimum score of the healthy cohort in our previous results. These samples could be grouped into the cancer cohort via their glycolysis related metabolite characters; the scores of postoperative samples showed improvement, but had still not recovered to normal status. These results validated the overexpression of LDHA and the glycolysis-related metabolite characteristics revealed in our study.
In this study, we chose the methodology of NMR spectrometer using IVDr system (Bruker Biospin, Rheinstetten, Germany), which has the advantages of excellent reproducibility and accuracy, absence of contamination, high throughput, and lower cost per sample for better preparation and support of clinical screening (37).
Conclusions
Our results showed that upregulation of lactic acid and downregulation of pyruvic acid coincided with overexpression of LDHA in glycolysis, and the combination of 4 related metabolites (glucose, lactic acid, pyruvic acid, and citric acid) has excellent distinguishing power for PC and can be used as a biomarker for early diagnosis and monitoring of curative effects. However, the sample volume of our research was limited, and the use of these metabolites to monitor curative effects still requires validation through further study.
Acknowledgments
Funding: This work was supported by the National Natural Science Foundation of China (81402174), the Natural Science Foundation of Tianjin (18JCZDJC32600), the National Natural Science Foundation of China (81802432).
Footnote
Reporting Checklist: The authors have completed the MDAR checklist. Available at http://dx.doi.org/10.21037/atm-21-295
Data Sharing Statement: Available at http://dx.doi.org/10.21037/atm-21-295
Conflicts of Interest: All authors have completed the ICMJE uniform disclosure form (available at http://dx.doi.org/10.21037/atm-21-295). The authors have no conflicts of interest to declare.
Ethical Statement: The authors are accountable for all aspects of the work in ensuring that questions related to the accuracy or integrity of any part of the work are appropriately investigated and resolved. The usage of serum samples in this study was reviewed and approved by the Ethics Committee of Tianjin Medical University Cancer Institute and Hospital (Scientific Ethical Approval No. bc2020101) and informed consent was taken from all the patients. The study was conducted in accordance with the Declaration of Helsinki (as revised in 2013).
Open Access Statement: This is an Open Access article distributed in accordance with the Creative Commons Attribution-NonCommercial-NoDerivs 4.0 International License (CC BY-NC-ND 4.0), which permits the non-commercial replication and distribution of the article with the strict proviso that no changes or edits are made and the original work is properly cited (including links to both the formal publication through the relevant DOI and the license). See: https://creativecommons.org/licenses/by-nc-nd/4.0/.
References
- Siegel RL, Miller KD. Cancer statistics, 2020. CA Cancer J Clin 2020;70:7-30. [Crossref] [PubMed]
- Gupta R, Amanam I, Chung V. Current and future therapies for advanced pancreatic cancer. J Surg Oncol 2017;116:25-34. [Crossref] [PubMed]
- Bausch D, Thomas S, Mino-Kenudson M, et al. Plectin-1 as a novel biomarker for pancreatic cancer. Clin Cancer Res 2011;17:302-9. [Crossref] [PubMed]
- Wishart DS. Emerging applications of metabolomics in drug discovery and precision medicine. Nat Rev Drug Discov 2016;15:473-84. [Crossref] [PubMed]
- Hanash SM, Ostrin EJ, Fahrmann JF. Blood based biomarkers beyond genomics for lung cancer screening. Transl Lung Cancer Res 2018;7:327-35. [Crossref] [PubMed]
- Singhal S, Rolfo C, Maksymiuk AW, et al. Liquid Biopsy in Lung Cancer Screening: The Contribution of Metabolomics. Results of A Pilot Study. Cancers (Basel) 2019;11:1069. [Crossref] [PubMed]
- Yu L, Li K, Zhang X. Next-generation metabolomics in lung cancer diagnosis, treatment and precision medicine: mini review. Oncotarget 2017;8:115774-86. [Crossref] [PubMed]
- Koppenol WH, Bounds PL, Dang CV. Otto Warburg's contributions to current concepts of cancer metabolism. Nat Rev Cancer 2011;11:325-37. [Crossref] [PubMed]
- Warburg O, Wind F, Negelein E. THE METABOLISM OF TUMORS IN THE BODY. J Gen Physiol 1927;8:519-30. [Crossref] [PubMed]
- Pei H, Li L, Fridley BL, et al. FKBP51 affects cancer cell response to chemotherapy by negatively regulating Akt. Cancer Cell 2009;16:259-66. [Crossref] [PubMed]
- Li L, Zhang JW, Jenkins G, et al. Genetic variations associated with gemcitabine treatment outcome in pancreatic cancer. Pharmacogenet Genomics 2016;26:527-37. [Crossref] [PubMed]
- Ellsworth KA, Eckloff BW, Li L, et al. Contribution of FKBP5 genetic variation to gemcitabine treatment and survival in pancreatic adenocarcinoma. PLoS One 2013;8:e70216. [Crossref] [PubMed]
- Vincent A, Herman J, Schulick R, et al. Pancreatic cancer. Lancet 2011;378:607-20. [Crossref] [PubMed]
- Sancho P, Burgos-Ramos E, Tavera A, et al. MYC/PGC-1α Balance Determines the Metabolic Phenotype and Plasticity of Pancreatic Cancer Stem Cells. Cell Metab 2015;22:590-605. [Crossref] [PubMed]
- Yang Y, Ishak Gabra MB, Hanse EA, et al. MiR-135 suppresses glycolysis and promotes pancreatic cancer cell adaptation to metabolic stress by targeting phosphofructokinase-1. Nat Commun 2019;10:809. [Crossref] [PubMed]
- Tsutsumi S, Yanagawa T, Shimura T, et al. Autocrine motility factor signaling enhances pancreatic cancer metastasis. Clin Cancer Res 2004;10:7775-84. [Crossref] [PubMed]
- McDonald OG, Li X, Saunders T, et al. Epigenomic reprogramming during pancreatic cancer progression links anabolic glucose metabolism to distant metastasis. Nat Genet 2017;49:367-76. [Crossref] [PubMed]
- Qin Y, Cheng C, Lu H, et al. miR-4458 suppresses glycolysis and lactate production by directly targeting hexoki-nase2 in colon cancer cells. Biochem Biophys Res Commun 2016;469:37-43. [Crossref] [PubMed]
- Li HM, Yang JG, Liu ZJ, et al. Blockage of glycolysis by targeting PFKFB3 suppresses tumor growth and metastasis in head and neck squamous cell carcinoma. J Exp Clin Cancer Res 2017;36:7. [Crossref] [PubMed]
- Dasgupta S, Rajapakshe K, Zhu B, et al. Metabolic enzyme PFKFB4 activates transcriptional coactivator SRC-3 to drive breast cancer. Nature 2018;556:249-54. [Crossref] [PubMed]
- Yun SJ, Jo SW, Ha YS, et al. PFKFB4 as a prognostic marker in non-muscle-invasive bladder cancer. Urol Oncol 2012;30:893-9. [Crossref] [PubMed]
- Shen M, Zhao X, Zhao L, et al. Met is involved in TIGAR-regulated metastasis of non-small-cell lung cancer. Mol Cancer 2018;17:88. [Crossref] [PubMed]
- Liu J, Lu F, Gong Y, et al. High expression of synthesis of cytochrome c oxidase 2 and TP53-induced glycolysis and apoptosis regulator can predict poor prognosis in human lung adenocarcinoma. Hum Pathol 2018;77:54-62. [Crossref] [PubMed]
- Cui K, Jin S, Du Y, et al. Long noncoding RNA DIO3OS interacts with miR-122 to promote proliferation and invasion of pancreatic cancer cells through upregulating ALDOA. Cancer Cell Int 2019;19:202. [Crossref] [PubMed]
- Ji S, Zhang B, Liu J, et al. ALDOA functions as an oncogene in the highly metastatic pancreatic cancer. Cancer Lett 2016;374:127-35. [Crossref] [PubMed]
- Youns M, ElKhoely A, Kamel R. The growth inhibitory effect of gambogic acid on pancreatic cancer cells. Naunyn Schmiedebergs Arch Pharmacol 2018;391:551-60. [Crossref] [PubMed]
- Fantin VR, St-Pierre J, Leder P. Attenuation of LDH-A expression uncovers a link between glycolysis, mitochondrial physiology, and tumor maintenance. Cancer Cell 2006;9:425-34. [Crossref] [PubMed]
- Tachtsidis A, McInnes LM, Jacobsen N, et al. Minimal residual disease in breast cancer: an overview of circulating and disseminated tumour cells. Clin Exp Metastasis 2016;33:521-50. [Crossref] [PubMed]
- Xintaropoulou C, Ward C, Wise A, et al. A comparative analysis of inhibitors of the glycolysis pathway in breast and ovarian cancer cell line models. Oncotarget 2015;6:25677-95. [Crossref] [PubMed]
- Boudreau A, Purkey HE, Hitz A, et al. Metabolic plasticity underpins innate and acquired resistance to LDHA inhibition. Nat Chem Biol 2016;12:779-86. [Crossref] [PubMed]
- Rajeshkumar NV, Dutta P, Yabuuchi S, et al. Therapeutic Targeting of the Warburg Effect in Pancreatic Cancer Relies on an Absence of p53 Function. Cancer Res 2015;75:3355-64. [Crossref] [PubMed]
- Maftouh M, Avan A, Sciarrillo R, et al. Synergistic interaction of novel lactate dehydrogenase inhibitors with gemcitabine against pancreatic cancer cells in hypoxia. Br J Cancer 2014;110:172-82. [Crossref] [PubMed]
- Le A, Cooper CR, Gouw AM, et al. Inhibition of lactate dehydrogenase A induces oxidative stress and inhibits tumor progression. Proc Natl Acad Sci U S A 2010;107:2037-42. [Crossref] [PubMed]
- Stolzenberg-Solomon RZ, Graubard BI, Chari S, et al. Insulin, glucose, insulin resistance, and pancreatic cancer in male smokers. JAMA 2005;294:2872-8. [Crossref] [PubMed]
- Xiao Y, Yang H, Lu J, et al. Serum gamma-glutamyltransferase and the overall survival of metastatic pancreatic cancer. BMC Cancer 2019;19:1020. [Crossref] [PubMed]
- Iarrobino NA, Gill BS, Klement RJ, et al. The Impact of Serum Glucose in the Treatment of Locoregionally Advanced Pancreatic Cancer. Am J Clin Oncol 2019;42:692-7. [Crossref] [PubMed]
- Jiménez B, Holmes E, Heude C, et al. Quantitative Lipoprotein Subclass and Low Molecular Weight Metabolite Analysis in Human Serum and Plasma by 1H NMR Spectroscopy in a Multilaboratory Trial. Anal Chem 2018;90:11962-71. [Crossref] [PubMed]