Nomogram for predicting the survival rate of primary pulmonary mucoepidermoid carcinoma patients: a retrospective study based on SEER database
Introduction
Mucoepidermoid carcinoma is the most common malignant salivary gland tumor in children and adults (1). Most mucoepidermoid carcinoma identified in the head and neck can also occur in other sites, such as lung, skin, breast, liver, and so forth (1-3). Pulmonary mucoepidermoid carcinoma (PMEC) is rare, which originates from the submucosal glands of the tracheobronchial tree (4,5). It was first defined in 1952 by Smetana et al. (6) and accounted for only 0.1–0.2% of all primary pulmonary malignant tumors (7).
PMEC is composed of mucinous cells, intermediate cells, and squamous cells arranged in solid, cystic, or glandular shape (8). According to the degree of cell differentiation, atypia, and mitotic figures, PMEC was classified as low and high-grade (9). The majority of high-grade PMEC is the solid squamous cell with strong invasiveness, presenting with regional lymph node metastasis, and distant metastasis (10). Low-grade PMEC is mostly cystic components, with a favorable prognosis. However, due to the lack of specificity in PMEC patients’ clinical manifestations, the grade classification and diagnosis of PMEC depends on bronchoscopy and lung biopsy (11,12). In addition, the low incidence of PMEC and limited data makes it challenging to characterize epidemiologically. Furthermore, the traditional AJCC TNM staging system is backward owing to its timeliness and lack of individuality.
Nomogram based on cox regression is a graphical calculating tool that involved patients’ characteristics in estimating the personalized survival time and providing individualized risk prediction (13). Compared to other estimation models, nomogram has superior accuracy, utility, and risk classification (14). Based on specific samples, it can more effectively estimate patients’ survival rate with individualized baseline characteristics, applied in the prognosis estimation of malignant tumors.
Whereas there is no study to construct a prediction model to forecast the individual survival time of primary PMEC patients. Our study addressed this gap, attempting to construct and validate authentic nomograms to estimate the survival outcome of PMEC patients. We present the following article in accordance with the TRIPOD reporting checklist (available at http://dx.doi.org/10.21037/atm-20-6555).
Methods
Data source
- This retrospective study was performed based on the SEER database (http://www.seer.cancer.gov), provided by the National Cancer Institute, which summarized 18 registries of the US from 1975–2016 and covered more than 30% of the US population. SEER*Stat 8.3.6 software was used to access information from the database. We identified the study population from the SEER cancer registry followed: (I) Diagnosis of MEC (ICD-O-3, Hist/behave: 8430/3 and primary sites: C34.1, C34.2, C34.3, C34.8, C34.9);
- Only primary cancer;
- Age of diagnosis ≥18 years;
- Active follow-up.
Those who had incomplete characteristics or only with a death certificate or autopsy were excluded.
The study was conducted in accordance with the Declaration of Helsinki (as revised in 2013).
Data extraction
We recorded eligible patients’ demographics, clinicopathological features, and follow-up information, covering age at diagnosis, sex, race, marital status, tumor stage, differentiated grade, tumor laterality, primary tumor site, tumor size (T, the largest diameter of the primary tumor), lymph node metastases status (N), tumor distant metastases status (M), surgery, radiation and chemotherapy. These continuous variables, including age and tumor size were translated into categorical variables using the X-tile 3.6.1 program (Yale University, New Haven, CT), according to the minimum P value of the survival time. The age was divided into ≤44 years old, 44–69 years old, and ≥69 years old.
Owing to the traditional AJCC TNM system had multiple versions in the SEER database and did not apply to all patients, we created a customized TNM system. Collecting tumor size, lymph metastases status, and tumor distant metastases status from different periods, and divided into N0/N+, M0/M1. Tumor size was divided into two groups: ≤2.7 centimeters, 2.7–4.8 centimeters, and ≥4.8 centimeters by X-tile. Survival analysis indicated no survival difference was observed among N1, N2, and N3 (P=0.067). Hence, we redefined the positive lymph node metastasis (N1, N2, or N3) as one group named N+.
Statistical analysis
OS was the primary outcome and cancer-specific survival (CSS) as the secondary outcome in this study. CSS was defined as the time from diagnosis to death caused by MEC; alive or death from other diseases was considered as censored cases. We identified 438 eligible cases in this study. In order to construct and validate the nomograms, we divided these eligible cases into training cohort (n=308, 70%) and validation cohort (n=130, 30%) randomly by 7:3. All categorical variables of baseline characteristics (age, sex, race, marital status, tumor stage, differentiated grade, tumor laterality, primary tumor site, T, N, M, surgery, radiation, and chemotherapy) were compared using the χ2 test. In the training cohort, univariate and multivariate analyses were used to identify the independent prognostic variables of nomogram construction used to build a risk classification system-Only variables with significant (P<0.05) in univariate analyses can be retained into multivariate analyses. Considering the small sample size of CSS, we used OS independent factors to build a nomogram.
Validation cohorts were devoted to examine the accuracy of the nomograms. Internal validation was evaluated under bootstrapping validation with 1,000 resamples. The calibration curve and concordance index (C-index) were applied to reflect the conformance between predicting survival and reality survival. All patients in the training cohort were divided into two groups according to their risk scores for examining the nomograms’ discriminability. Scores above the average are considered a high-risk group, while below-average account for the low-risk group. The survival curves of risk groups were evaluated by the log-rank test and shown by the Kaplan-Meier plot. IDI was used to compare our nomogram with other models.
All statistical analyses were conducted using SPSS version 26.0 (SPSS Chicago, IL) and rms, foreign, survival, and survival ROC packages in R version 3.6.1 software (R Foundation for Statistical Computing, Vienna, Austria, http://www.r-project.org/). All tests with two sides P value less than 0.05 was considered a significant difference.
Results
Characteristics
A total of 438 patients with primary PMEC were reviewed in this study. According to age, all patients were divided to ≤44 years old (n=185, 42.2%), 44–69 years old (n=177, 40.4%) and ≥69 years old (n=76, 17.4%). The whole sample had the similar distribution of gender and laterality: males (n=234, 53.4%) and females (n=204, 46.6%), left (n=211, 48.2%) and right (n=227, 51.8%). The majority of race was white (n=338, 77.2%).
For differentiation degree, tumors with well, moderately, poorly, and undifferentiated accounted for 23.5%, 34.7%, 9.6%, and 8.7% of all patients, respectively. Of all cases, more than half were negative lymph node metastases (n=251, 57.3%) and negative distant metastases (n=289, 66.0%). Moreover, most of patients received surgery (n=339, 77.4%) and did not accept chemotherapy (n=377, 86.1%). Only 20.1% of patients underwent radiation. There is no significant discrepancy between the training cohort and validation cohort. The detailed baseline characteristics were shown in Table 1.
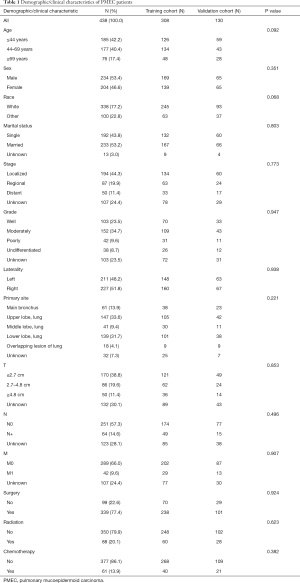
Full table
Independent prognostic factors of nomogram construction
Prognostic factors in predicting OS
Univariate and multivariate Cox proportional hazards regression models were conducted to identify prognostic factors. As shown in Table 2, in univariate analyses, we noticed except for sex (P=0.364), race (P=0.609), marital status (P=0.385), and tumor laterality (P=0.715), the other variables were shown a significant association with OS. Multivariate analyses suggested that age, tumor stage, differentiated grade, T, N, M, surgery, and radiation as the independent prognostic factors for OS.
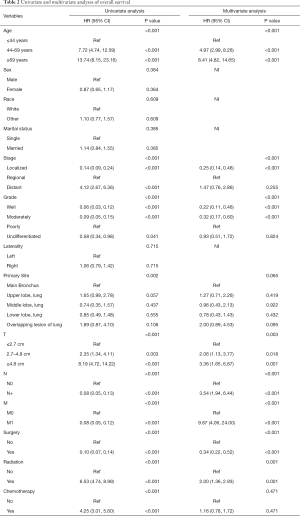
Full table
Prognostic factors in predicting CSS
Of 175 cases. 136 patients died of MEC, while the other 39 patients were ascribed to non-MEC causes. As shown in Table S1, tumor stage, T, M, surgery, and radiation were analyzed as the prognostic factors predicting CSS of MEC patients.
Construction of nomograms
We constructed two nomograms based on independent prognostic factors identified by Cox proportional hazards analyses, including age, tumor stage, differentiated grade, T, N, M, surgery, and radiation. The eight variables were enrolled in nomograms building for 3-, 5- and 10-year OS and CSS of MEC patients in this study. Each variable had a corresponding score on the point scale, locating and summing up each score according to the baseline characteristic; the total scores were obtained. The total scores represent the probabilities of 3-, 5- and 10-year OS and CSS. Particulars score of each variable in the nomograms were shown in Figures 1,2.
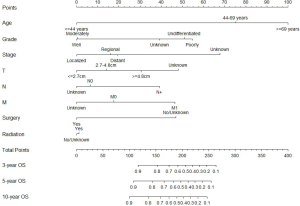
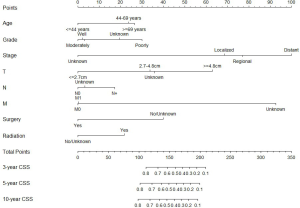
Calibration and validation of nomogram
OS and CSS nomograms had the acceptance C-index in training cohort, 0.887 (95% CI: 0.863–0.911) and 0.764 (95% CI: 0.713–0.815), respectively. In internal validation, the nomograms of OS (Figure 3) and CSS (Figure S1) had considerable C-index values, 0.912 (95% CI: 0.883–0.941) and 0.794 (95% CI: 0.727–0.861), respectively. The calibration curves of 3-, 5- and 10-year OS (Figures 4,5) and CSS (Figures S2,S3) indicated considerable coherence between estimation and actual in the training and validation cohort.
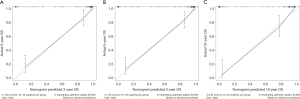
Compared to the TNM system, our nomograms have better accuracy in estimating survival time in the training cohort. The IDI (Table 3) of our nomogram comparing with the TNM system is 0.167 for 3-year OS (P<0.001). For 5-, 10-year OS and 3-,5-, 10-year CSS, the IDI indicated that our models are superior to the TNM system significantly.
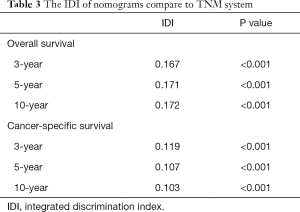
Full table
Risk stratification of OS and CSS
According to each case’s total scores, we further explored risk stratification for OS and CSS based on this study’s training cohort. All MEC training cohort patients were divided into a high-risk group or low-risk group. As Figure 6 shown, both OS and CSS, the Kaplan-Meier curves, indicated that the low-risk group had a significantly better survival (P<0.001 and P<0.001, respectively).
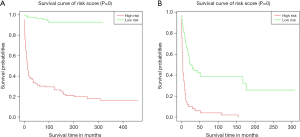
Clinical use
Scores of each variable were obtained by drawing a vertical line to the top points row; then, the sum of the points of each variable is located on the total points row, and a vertical line is drawn from the total point row to 3-, 5-, 10-year OS or CSS row to acquire the survival probability of patients (Figure 1). For example, one patient was a 47-year-old, undifferentiated PMEC with 1.8 cm and N+M0 regional stage, and received surgical and radiation therapy; the total score is 200 points with corresponding 3-, 5-, 10-year OS of 0.50–0.60, 0.40–0.50, and 0.40–0.50, respectively.
Discussion
Primary mucoepidermoid carcinoma of pulmonary salivary gland cancer is a scarce histologic type, which accounts for 0.1-0.2% in all malignant lung cancers generally (15). Owing to the small sample size, precedent reports in the literature were case presentations and case series, while the study based on a large population is lack, and knowledge of PMEC patients’ survival is limited. Moreover, its pathological staging still depends on the traditional TNM system based on general lung cancer. We hypothesized that one of the reasons for poor prognosis was the lack of a specific model specifically for PMEC patients. To provide new ideas for the treatment of PMEC, we established the nomograms and the risk classifications to predict individual PMEC patients’ survival probabilities.
Our nomograms were validated with the internal cohort, C-index, ROC curve, and calibration plots, suggesting that our models were steady and accurate as the predictive device to estimate survival rate and select treatment options. We also established risk stratification as the supplement of nomograms. The TNM system is a generalized and straightforward tool to forecast survival and guide treatment. An individual predicting model should be developed to narrow further the difference between the predicted survival and the actual survival.
Based on univariate and multivariate analyses, we recognized these independent prognosis factors, including age, tumor stage, differentiated grade, T stage, N stage, M stage, surgery, and radiation. Most of the above factors are consistent with the previous study (16,17). Previous studies reported that young people are more likely to have PMEC, while for the past few years, the morbidity of PMEC aging (16). Like the precedent conclusion (18,19), sex was no significant relation to PMEC patients’ prognosis. Our study exclusively examined PMEC samples, which is probably more representative than the precedent outcomes.
Higher degree differentiation of the PMEC enhanced the survival rate of patients. 255 (58.2%) patients are a higher differentiation degree (well and moderately) and 80 (18.3%) samples are a lower differentiation degree (poorly and undifferentiated). In both groups, there was less patients response to radiation or chemotherapy (P=0.001 and 0.471, respectively). In this study, most PMEC was moderate grade; this finding is consistent with the discovery by Chen et al. (20), in which 47.7% of mucoepidermoid carcinoma were moderate grade.
Complete surgical resection is essential for PMEC patients, and it will guide postoperative chemoradiotherapy according to pathological biopsy (21). Besides, bronchoplasty procedures are efficient methods for preserving pulmonary parenchyma. Several published studies regarded that surgical resection can prolong survival time (18,19,22). Endobronchial intervention can maintain airway patency, commonly applied to obstructive pneumonia patients (23,24). However, endobronchial intervention therapy does not remove the tumor entirely; surgical resection is still preferred (25).
Nevertheless, high-grade tumors are challenging to resection entirely owing to aggressiveness; these patients require postoperative chemoradiotherapy (26). For these patients, adjuvant therapy should be performed when surgical resection is not radical, including chemotherapy and radiation (27). The precedent report considered that adjuvant chemotherapy is useful for high-grade PMEC but not presented to low-grade; the combination of cisplatin and vinorelbine or docetaxel is generally used. However, the benefit of adjuvant radiotherapy is still controversial (28). In this study, we noticed that patients with adjuvant therapy had poor prognosis than those without chemotherapy and (or) radiation. The reasonable explanation is that traditional adjuvant therapy is not conducive to reconstructing immune function, and the painful physical and mental also reduces the survival time. Besides, some patients can not tolerate surgery or other reasons; they received chemotherapy and (or) radiation instead of surgical in our study samples. Although our nomograms are precisely models, there are also many shortcomings to noticed.
- the data come from the SEER database, and this database can not provide complete variables, for instance, smoking status, surgery procedure, chemotherapy regimens.
- The AJCC 8th edition system can not apply to previous patients; we transformed the 432 PMEC patients’ TNM status prudently, and some errors are inevitable.
- We failed to obtain sufficient cases as an external validation cohort to further demonstrate our nomograms’ consistency.
- The data used in our study were retrospective; there is information bias. Further studies need to be performed to improve these deficiencies.
- The small sample size may still affect the stability of this data analysis, though we as far as possible to improve the analysis process.
Conclusions
In conclusion, we built OS (CSS) nomograms that can accurately estimate individualized survival time and identify the risk classification of PMEC. Calibration plots and ROC curves demonstrated the clinical utility of our nomograms.
Acknowledgments
Funding: None.
Footnote
Reporting Checklist: The authors have completed the TRIPOD reporting checklist. Available at http://dx.doi.org/10.21037/atm-20-6555
Peer Review File: Available at http://dx.doi.org/10.21037/atm-20-6555
Conflicts of Interest: All authors have completed the ICMJE uniform disclosure form (available at http://dx.doi.org/10.21037/atm-20-6555). JH serves as an unpaid Editors-in-Chief of Annals of Translational Medicine. The other authors have no conflicts of interest to declare.
Ethical statement: The authors are accountable for all aspects of the work in ensuring that questions related to the accuracy or integrity of any part of the work are appropriately investigated and resolved. The study was conducted in accordance with the Declaration of Helsinki (as revised in 2013).
Open Access Statement: This is an Open Access article distributed in accordance with the Creative Commons Attribution-NonCommercial-NoDerivs 4.0 International License (CC BY-NC-ND 4.0), which permits the non-commercial replication and distribution of the article with the strict proviso that no changes or edits are made and the original work is properly cited (including links to both the formal publication through the relevant DOI and the license). See: https://creativecommons.org/licenses/by-nc-nd/4.0/.
References
- Goode RK, Auclair PL, Ellis GL. Mucoepidermoid carcinoma of the major salivary glands: clinical and histopathologic analysis of 234 cases with evaluation of grading criteria. Cancer 1998;82:1217-24. [Crossref] [PubMed]
- Fonseca FP, Carvalho Mde V, de Almeida OP, et al. Clinicopathologic analysis of 493 cases of salivary gland tumors in a Southern Brazilian population. Oral Surg Oral Med Oral Pathol Oral Radiol 2012;114:230-9. [Crossref] [PubMed]
- de Almeida-Pinto YD, Costa S, de Andrade BAB, et al. t(6;9)(MYB-NFIB) in head and neck adenoid cystic carcinoma: A systematic review with meta-analysis. Oral diseases 2019;25:1277-82. [Crossref] [PubMed]
- Turnbull AD, Huvos AG, Goodner JT, et al. Mucoepidermoid tumors of bronchial glands. Cancer 1971;28:539-44. [Crossref] [PubMed]
- Heitmiller RF, Mathisen DJ, Ferry JA, et al. Mucoepidermoid lung tumors. Ann Thorac Surg 1989;47:394-9. [Crossref] [PubMed]
- Smetana HF, Iverson L, Swan LL. Bronchogenic carcinoma; an analysis of 100 autopsy cases. Mil Surg 1952;111:335-51. [PubMed]
- Stenner M, Klussmann JP. Current update on established and novel biomarkers in salivary gland carcinoma pathology and the molecular pathways involved. European archives of oto-rhino-laryngology: official journal of the European Federation of Oto-Rhino-Laryngological Societies (EUFOS): affiliated with the German Society for Oto-Rhino-Laryngology - Head Neck Surg 2009;266:333-41.
- Moran CA. Primary salivary gland-type tumors of the lung. Semin Diagn Pathol 1995;12:106-22. [PubMed]
- Wang YQ, Mo YX, Li S, et al. Low-Grade and High-Grade Mucoepidermoid Carcinoma of the Lung: CT Findings and Clinical Features of 17 Cases. AJR Am J Roentgenol 2015;205:1160-6. [Crossref] [PubMed]
- Roden AC, García JJ, Wehrs RN, et al. Histopathologic, immunophenotypic and cytogenetic features of pulmonary mucoepidermoid carcinoma. Mod Pathol 2014;27:1479-88. [Crossref] [PubMed]
- Li X, Zhang W, Wu X, et al. Mucoepidermoid carcinoma of the lung: common findings and unusual appearances on CT. Clin Imaging 2012;36:8-13. [Crossref] [PubMed]
- Yu Y, Song Z, Gao H, et al. EGFR L861Q mutation is a frequent feature of pulmonary mucoepidermoid carcinoma. J Cancer Res Clin Oncol 2012;138:1421-5. [Crossref] [PubMed]
- Park SY. Nomogram: An analogue tool to deliver digital knowledge. J Thorac Cardiovasc Surg 2018;155:1793. [Crossref] [PubMed]
- Balachandran VP, Gonen M, Smith JJ, et al. Nomograms in oncology: more than meets the eye. Lancet Oncol 2015;16:e173-80. [Crossref] [PubMed]
- Shen C, Che G. Clinicopathological analysis of pulmonary mucoepidermoid carcinoma. World J Surg Oncol 2014;12:33. [Crossref] [PubMed]
- Komiya T, Perez RP, Yamamoto S, et al. Primary lung mucoepidermoid carcinoma: analysis of prognostic factors using surveillance, epidemiology and end results program. Clin Respir J 2017;11:847-53. [Crossref] [PubMed]
- Jiang L, Li P, Xiao Z, et al. Prognostic factors of primary pulmonary mucoepidermoid carcinoma: a clinical and pathological analysis of 34 cases. Int J Clin Exp Pathol 2014;7:6792-9. [PubMed]
- Falk N, Weissferdt A, Kalhor N, et al. Primary Pulmonary Salivary Gland-type Tumors: A Review and Update. Adv Anat Pathol 2016;23:13-23. [Crossref] [PubMed]
- Lee GD, Kang DK, Kim HR, et al. Surgical outcomes of pulmonary mucoepidermoid carcinoma: a review of 23 cases. Thorac Cardiovasc Surg 2014;62:140-6. [PubMed]
- Chen MM, Roman SA, Sosa JA, et al. Histologic grade as prognostic indicator for mucoepidermoid carcinoma: a population-level analysis of 2400 patients. Head Neck 2014;36:158-63. [Crossref] [PubMed]
- Kanemoto A, Oshiro Y, Sugahara S, et al. Proton beam therapy for inoperable recurrence of bronchial high-grade mucoepidermoid carcinoma. Jpn J Clin Oncol 2012;42:552-5. [Crossref] [PubMed]
- Molina JR, Aubry MC, Lewis JE, et al. Primary salivary gland-type lung cancer: spectrum of clinical presentation, histopathologic and prognostic factors. Cancer 2007;110:2253-9. [Crossref] [PubMed]
- Vadasz P, Egervary M. Mucoepidermoid bronchial tumors: a review of 34 operated cases. Eur J Cardiothorac Surg 2000;17:566-9. [Crossref] [PubMed]
- Song Z, Liu Z, Wang J, et al. Primary tracheobronchial mucoepidermoid carcinoma--a retrospective study of 32 patients. World J Surg Oncol 2013;11:62. [Crossref] [PubMed]
- Kitada M, Matsuda Y, Sato K, et al. Mucoepidermoid carcinoma of the lung: a case report. J Cardiothorac Surg 2011;6:132. [Crossref] [PubMed]
- Sonobe S, Inoue K, Tachibana S, et al. A case of pulmonary mucoepidermoid carcinoma responding to carboplatin and paclitaxel. Jpn J Clin Oncol 2014;44:493-6. [Crossref] [PubMed]
- Zhu F, Liu Z, Hou Y, et al. Primary salivary gland-type lung cancer: clinicopathological analysis of 88 cases from China. J Thorac Oncol 2013;8:1578-84. [Crossref] [PubMed]
- Xi JJ, Jiang W, Lu SH, et al. Primary pulmonary mucoepidermoid carcinoma: an analysis of 21 cases. World J Surg Oncol 2012;10:232. [Crossref] [PubMed]