Biomarkers and key pathways in atrial fibrillation associated with mitral valve disease identified by multi-omics study
Introduction
Atrial fibrillation (AF) is one of the most common arrhythmias (1,2). It is known that electrical, contractile, and structural remodeling play important roles in the initiation and maintenance of AF (3). Patients with mitral valve disease (MVD) and AF are associated with an increased risk of thromboembolic events. A recent study (4) reported that the prevalence of persistent or permanent AF in MVD is as high as 87.1% in mitral regurgitation and 86.6% in aortic regurgitation, highlighting the importance of AF in MVD. Despite efforts to discover plasma biomarkers in MVD (5) and to identify the role of ion channels (6), the molecular mechanism of AF in MVD remains unclear. Recently, proteomics studies were performed to explore the mechanisms of AF (6-10). These studies identified the differential proteins (DPs) in the right and left atrial appendage of rheumatic MVD patients (6,7,9), or pathways including ketone body (8) or glutathione (10) metabolism and the peroxisome proliferator-activated receptor (PPAR) pathway (9,10), which are related to postoperative AF (8-10).
We recently performed multi-omics studies in MVD (5,6,9), congenital heart disease (11-14), and coronary artery disease (15). In human atrial tissues from patients with rheumatic MVD in persistent AF compared to sinus rhythm (SR) using a combined transcriptomic and proteomic approach, we found that intracellular chloride channels (6) as well as PPARα, β, and γ pathways play an important role in the development of AF (9). However, for persistent and chronic AF in MVD, no combined proteomics and metabolomics studies have been reported. In this study, we used multi-omics methods to investigate plasma samples from patients with MVD in AF or in SR undergoing heart valve replacement surgery to identify pathways and biomarkers associated with AF. We present the following article following the Strengthening the Reporting of Observational Studies in Epidemiology (STROBE) reporting checklist (available at http://dx.doi.org/10.21037/atm-20-3767).
Methods
Patient recruitment and sample collection
From January 2013 to December 2017, 100 consecutive patients with MVD who underwent mitral valve replacement surgery at TEDA International Cardiovascular Hospital were enrolled and grouped into either chronic AF (n=70) or SR (n=30). AF was diagnosed by history, physical examination, and a 12-lead electrocardiogram. For comparison, patients in SR were screened to ensure that they had no AF by direct questioning about symptoms suggestive of AF and by analysis of 12-lead electrocardiograms. Patients with infective endocarditis, sick sinus syndrome, familial paroxysmal AF (lone AF/familial AF), pulmonary heart disease, cardiomyopathy, hyperthyroidism, chronic kidney diseases, coronary artery disease, and those under the age of 18 years in both groups were also excluded to reduce prevalence-incidence bias. The demographic characteristics of patients are shown in Tables S1,S2.
This study was approved by the Ethics Committee of the TEDA International Cardiovascular Hospital {No. [2019]-0315-1}. Written informed consent was obtained from all patients enrolled in the study and conforms to the principles outlined in the Declaration of Helsinki (as revised in 2013). Blood samples were collected from all patients the day before surgery and were then centrifuged at 3,500 rpm for 10 min to separate the plasma from blood cells.
Proteomics analysis
A discovery-validation workflow was utilized in the proteomics analysis, and isobaric tags for relative and absolute quantitation (iTRAQ) were used for the proteomics study. As eight isobaric tags (m/z 113, 114, 115, 116, 117, 118, 119, and 121) were applied in the study, the plasma samples from 24 patients were divided into six groups (three AF groups and three SR groups). Each of these groups contained the plasma samples from four patients. Also, plasma samples from 12 AF or 12 SR patients were used as controls (Figure S1). Thus, the eight isobaric tags were concomitantly labeled in the plasma samples for the iTRAQ study (16).
In the validation phase, DPs identified by the above iTRAQ study were validated in plasma samples taken from a new cohort of patients using enzyme-linked immunosorbent assays (ELISA).
Metabolomics analysis
Plasma samples were thawed at room temperature and were then prepared for gas chromatography-mass spectrometry (GC-MS) analysis (Figure S1). ChromaTOF software (v4.34, LECO, St. Joseph, MI, USA) and the Fiehn database were used to process the raw data and to provide qualitative information about the metabolites. The Kyoto Encyclopedia of Genes and Genomes (KEGG) pathway database was used to generate significant pathways in the metabolomics dataset (17).
Creation of pathway maps
All of the omics data were used to compare proteins/enzymes with metabolic pathways. Quantitative changes of individual metabolites and proteins were calculated and graphed using the KEGG mapping tools. By combining proteomics and metabolomics data, we were able to identify the common pathways involved in AF.
Statistical analysis
SPSS 23.0 software (SPSS Inc., Chicago, IL, USA) and GraphPad 6.0c software (GraphPad Software, La Jolla, CA, USA) were used for statistical analysis. Data are expressed as mean ± standard error of the mean (SEM). Comparisons of statistical data were performed using either the independent samples t-test or Chi-square tests. A P value of <0.05 was considered statistically significant.
More detailed methods are presented in Appendix 1.
Results
Patient characteristics
The clinical characteristics of the study cohorts are summarized in Tables S1,S2. Patients in the AF group presented with larger left atrium-systolic diameters (LA-Ds), right ventricular-diastolic diameters (RV-Dd), right atrium-systolic diameters (RA-Ds), and lower left ventricular ejection fraction (LVEF) than those in the SR group in both iTRAQ (Table S1) and ELISA validation (Table S2).
Proteomics
A large number of unique peptides were detected by iTRAQ. By matching this data with the theoretical spectrum, 447 reliable proteins were identified. Under the condition of fold change (FC) >1.2 or <0.83 and P value ≤0.05, the number of differentially expressed proteins in the AF group was 57 (Table S3, Figure 1A), including 33 up-regulated and 24 down-regulated (https://cdn.amegroups.cn/static/public/atm-20-3767-1.xlsx).
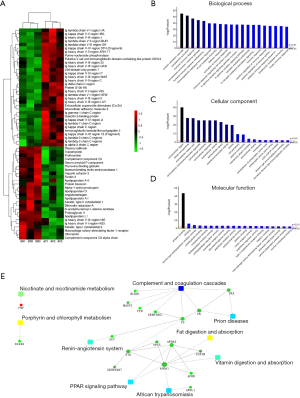
Bioinformatics analyses including Gene Ontology (GO) function and enrichment, KEGG pathway, and protein-protein interaction (PPI) were also performed (https://cdn.amegroups.cn/static/public/atm-20-3767-1.xlsx), and the results are shown in Figure 1B,C,D,E. Biological processes such as immune response (72.7%), defense response (74.5%), receptor-mediated endocytosis (50.9%), protein activation cascade (56.3%), and complement activation (52.7%) were enriched. Cellular component enrichment analysis revealed that most of the differentially expressed proteins were from the extracellular region (94.7%) and that binding (96.3%) was their main molecular function. Furthermore, KEGG pathway analysis demonstrated several pathways, such as the PPAR signaling pathway, renin-angiotensin system, complement and coagulation cascades, cell adhesion molecules, and adherent junction, were enriched. The PPI is shown in Figure 1E.
Protein validation by ELISA
Ten proteins were selected to validate the proteomics result by ELISA: apolipoprotein A-I (ApoA-I), apolipoprotein A-II (ApoA-II), LIM domain only protein 7 (LMO7), prothrombin fragment 1+2, vitronectin (VN), angiotensinogen, ficolin-3, serum amyloid P-component (SAP), macrophage colony-stimulating factor 1 receptor (CSF-1R), and purine nucleoside phosphorylase (PNP) (Figure 2). Six were successfully validated. Of these, the concentration of ApoA-I, angiotensinogen, CSF-1R, and VN 2 was lower in the AF group than those in the SR group, whereas the concentration of LMO7 and prothrombin fragment 1+2 was higher.
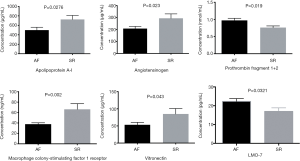
Metabolomics analysis in chronic AF
In the comparison of the plasma metabolite profile between 30 AF patients and 26 SR patients, 322 metabolites were discovered, including 55 differential metabolites (DMs) (Table S4) between AF and SR patients under the condition of variable importance in projection (VIP) >1 in the partial least-squares discriminant analysis (PLS-DA) model and P values <0.05 (https://cdn.amegroups.cn/static/public/atm-20-3767-2.xlsx). The heatmap of these metabolites is displayed in Figure 3. The sucrose concentration, cellobiose, lactose, raffinose, and galactinol was elevated in AF, along with several unsaturated fatty acids (such as arachidonic acid, palmitic acid, and linoleic acid). Furthermore, bioinformatics analysis was performed, and metabolic pathways including galactose metabolism, biosynthesis of unsaturated fatty acids, linoleic acid metabolism, ATP-binding cassette (ABC) transporters, and starch and sucrose metabolism were enriched, demonstrating a close connection between AF and these pathways.
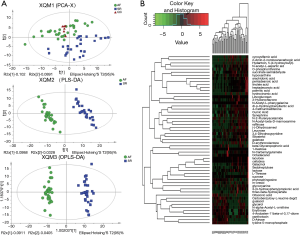
Combined proteomics and metabolomics analysis in chronic AF
We integrated the proteomics and metabolomics datasets to generate a global view of AF patients’ plasma signatures. The global cross-correlation analysis of identified metabolites and proteins demonstrated the recapitulation of known metabolome-proteome reaction models. As a result, the connection between identified proteins, metabolites, and several known enzymatic reaction models were established in Figure 4. On the other hand, KEGG mapping tools were used to integrate the above proteomics and metabolomics datasets into the human metabolic reference map (hsa01100) to provide a unified metabolic signature. As shown in Figure 4, each node represents a metabolite, the concentration of which was altered between the two groups. There were concurrent changes in both metabolites and proteins in the PPARα pathway and nucleotide metabolism. Notably, ELISA validation of PNP, an enzyme that regulates several purine metabolism reactions, revealed that the concentration of PNP was not significantly different between the two groups. Interestingly, proteomics and metabolomics analyses revealed that two proteins in the PPARα pathway, ApoA-I, and ApoA-II, were down-regulated in the AF patients.
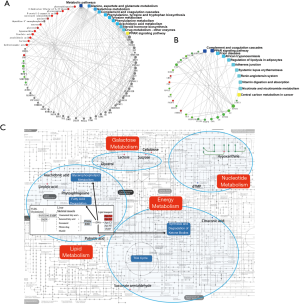
Discussion
In the present combined study of proteomics and metabolomics on the plasma of patients with heart valve disease, we found that: (I) among the 447 proteins and 322 metabolites detected, 57 proteins and 55 metabolites were differentially expressed between AF patients and SR patients; (II) by using bioinformatics tools, several pathways, particularly the PPARα pathway, were enriched; (III) other enriched pathways included the metabolic pathway, renin angiotensin aldosterone system (RAAS), galactose metabolism, biosynthesis of unsaturated fatty acid, and linoleic acid metabolism; and (IV) several DPs and metabolites, such as ApoA-I, ApoA-II, and LMO7, may be further developed as biomarkers for AF in patients with MVD in order to monitor disease progress. The results from our integrated omics study in the plasma of MVD patients with AF compared to MVD patients in SR have not been previously reported (see Table S5 for published studies). Figure 5 is a schema that briefly demonstrates our findings. The findings of this study complement the results of other studies regarding AF (18,19). However, our study provides important insights into multi-omics changes in MVD-associated AF.
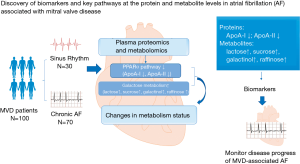
In this study, down-regulation of ApoA-I was observed in the AF patients. In comparison, the change of ApoA-II was only found in the proteomics study. ApoA-I and ApoA-II are the major components of high-density lipoprotein cholesterol (HDL-C), and the genes encoding these two proteins are activated by PPARα via direct transcriptional control (20). PPAR is a major transcriptional regulator of fatty acid metabolism (21). The PPAR pathway regulates the expression of various genes, including proteins involved in fatty acid degradation, glycerophospholipid metabolism, synthesis and degradation of ketone bodies, etc. (16).
Moreover, PPARα is a member of the ligand-activated nuclear receptor superfamily (22), and the dimer binds to PPAR response element (PPRE) on the promoter region of target genes and activates their expression (23). PPARα also serves as a xenobiotic and lipid sensor to regulate fatty acid oxidation, fatty acid storage, lipid uptake, triacylglycerol (TAG) synthesis, glucose oxidation, lipoprotein synthesis, and inflammatory responses, and thus plays a key role in energy combustion (24). The down-regulation of PPARα is most likely attributable to the down-regulation of ApoA-I and ApoA-II. As a result of PPARα down-regulation, lipid metabolism is suppressed, and glucose oxidation is activated to force the heart to utilize more glucose instead of fatty acids. This notion is supported by the present study’s metabolomic findings, as the concentration of plasma unsaturated fatty acids increased, indicating the accumulation of fatty acids in the circulation. Considering these findings, we hypothesize that AF is associated with the heart’s altered metabolic status and PPARα plays an important role in this alternation (Figure 6). Our study suggests that AF may impair the heart’s energy metabolism, and thus, the balance between lipid uptake and utilization is compromised. As a result, metabolic remodeling exists in the heart and is involved in the development of AF.
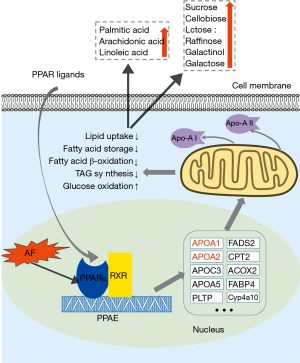
Several other proteins were also validated in the plasma of AF patients. Angiotensinogen is an essential component of the RAAS. It has recently been established that angiotensinogen and RAAS are closely associated with AF initiation and maintenance (25). It was reported that angiotensinogen gene polymorphisms and several other RAAS-related gene polymorphisms were associated with non-familial structural AF (26). The present study demonstrated that angiotensinogen concentration decreased in MVD patients with AF compared to those with SR. However, further exploration is required to understand the role of RAAS in AF better.
LMO7 is a member of the LIM domain-containing protein family and has been linked to the actin cytoskeleton organization. Interestingly, it has been reported that LMO7 is functionally involved in mammalian heart development, especially the development of the conduction system, and that knockdown of the LMO7 gene in zebrafish model may lead to arrhythmia, bradycardia, and delocalization of the heart (27). The present study showed up-regulation of LMO7 in the AF patients, suggesting that LMO7 plays a pathophysiological role in AF development. To our knowledge, this study is the first to reveal the alternation of the LMO7 protein in MVD-associated AF patients. These results suggest that the plasma level of LMO7 may be developed as a biomarker of AF.
VN is a glycoprotein found in serum and tissues, and serves as a cell-to-substrate adhesion molecule. Biological processes, including immune response, complement activation, and vascular endothelial growth factor receptor signaling pathway regulation, are also related to VN (28). VN inhibits the membrane-damaging effect of the terminal cytolytic complement pathway (28). In this study, we observed a down-regulation of VN concentration in AF patients, suggesting that the complement system is activated in MVD-associated AF, and that the plasma level of VN may be a potential biomarker of AF.
In this study, AF’s development was inevitably correlated to some clinical variables, such as left atrium (LA) size (see Tables S1,S2). Nevertheless, our study aimed to identify biomarkers of AF. Therefore, we included all patients who satisfied the study’s selection criteria and divided them into two groups according to the heart rhythm (either sinus or AF). The differences in the protein and metabolite levels between the two groups were then investigated. The above DPs and metabolites were successfully identified as potential biomarkers for AF.
Study limitations
This study has some limitations that should be noted. Both proteomics and metabolomics studies reveal abundant unnamed proteins and metabolites. In order to perform bioinformatics analyses, the corresponding identification (ID) in certain databases is required. In fact, some proteins or metabolites failed to be recognized by these databases, and therefore the data were “invalid” and were unable to be analyzed at this stage. With future advances in technology, more information may be provided.
Conclusions
In conclusion, the present study, for the first time, identified a large number of DPs and metabolites by using multi-omics technologies in MVD-associated AF patients. Our study provides an integrative view with the combined analyses of proteomics and metabolomics, and reveals that the PPAR signaling pathway, and particularly PPARα pathway, plays an important role in AF. Other pathways involved in MVD-associated AF are the RAAS, galactose metabolism, biosynthesis of unsaturated fatty acids, and linoleic acid metabolism. Some identified DPs and metabolites, such as ApoA-I, ApoA-II, LMO7, and VN, may be further developed as biomarkers for MVD-associated AF patients to monitor disease progress.
Acknowledgments
We acknowledge the surgeons and the head nurse (Kai-Jing Wei) and her nursing staff for assistance in collecting samples.
Funding: This work was supported by the National Natural Science Foundation of China (81870288 & 81641017); the Non-profit Central Research Institute Fund of Chinese Academy of Medical Sciences (2017NL31001 & 2018TX31002 & 2019XK310001& 2020-PT310-007); and the Tianjin Science and Technology Commission (18PTZWHZ00060).
Footnote
Reporting Checklist: The authors have completed the STROBE reporting checklist. Available at http://dx.doi.org/10.21037/atm-20-3767
Data Sharing Statement: Available at http://dx.doi.org/10.21037/atm-20-3767
Conflicts of Interest: All authors have completed the ICMJE uniform disclosure form (available at http://dx.doi.org/10.21037/atm-20-3767). All authors report that they have a patent pending for a diagnostic kit of multiple protein biomarkers and its application in atrial fibrillation. The authors have no other conflicts of interest to declare.
Ethical Statement: The authors are accountable for all aspects of the work in ensuring that questions related to the accuracy or integrity of any part of the work are appropriately investigated and resolved. This study was approved by the Ethics Committee of the TEDA International Cardiovascular Hospital {No. [2019]-0315-1} and conforms to the principles outlined in the Declaration of Helsinki (as revised in 2013). Written informed consent was obtained from each patient before being included in the study.
Open Access Statement: This is an Open Access article distributed in accordance with the Creative Commons Attribution-NonCommercial-NoDerivs 4.0 International License (CC BY-NC-ND 4.0), which permits the non-commercial replication and distribution of the article with the strict proviso that no changes or edits are made and the original work is properly cited (including links to both the formal publication through the relevant DOI and the license). See: https://creativecommons.org/licenses/by-nc-nd/4.0/.
References
- Chugh SS, Havmoeller R, Narayanan K, et al. Worldwide epidemiology of atrial fibrillation: a Global Burden of Disease 2010 Study. Circulation 2014;129:837-47. [Crossref] [PubMed]
- Fan K, Lee KL, Chiu CS, et al. Effects of biatrial pacing in prevention of postoperative atrial fibrillation after coronary artery bypass surgery. Circulation 2000;102:755-60. [Crossref] [PubMed]
- Andrade J, Khairy P, Dobrev D, et al. The clinical profile and pathophysiology of atrial fibrillation: relationships among clinical features, epidemiology, and mechanisms. Circ Res 2014;114:1453-68. [Crossref] [PubMed]
- Vinereanu D, Wang A, Mulder H, et al. Outcomes in anticoagulated patients with atrial fibrillation and with mitral or aortic valve disease. Heart 2018;104:1292-9. [Crossref] [PubMed]
- Gao G, Xuan C, Yang Q, et al. Identification of altered plasma proteins by proteomic study in valve heart diseases and the potential clinical significance. PLoS One 2013;8:e72111. [Crossref] [PubMed]
- Jiang YY, Hou HT, Yang Q, et al. Chloride channels are involved in the development of atrial fibrillation - a transcriptomic and proteomic study. Sci Rep 2017;7:10215. [Crossref] [PubMed]
- Liu H, Chen G, Zheng H, et al. Differences in atrial fibrillation associated proteins between the left and right atrial appendages from patients with rheumatic mitral valve disease: a comparative proteomic analysis. Mol Med Rep 2016;14:4232-42. [Crossref] [PubMed]
- Mayr M, Yusuf S, Weir G, et al. Combined metabolomic and proteomic analysis of human atrial fibrillation. J Am Coll Cardiol 2008;51:585-94. [Crossref] [PubMed]
- Chen HX, Li MY, Jiang YY, et al. Role of PPAR pathway in atrial fibrillation associated with heart valvular disease: transcriptomics and proteomics in the human atrial tissue. Signal Transduct Target Ther 2020;5:4. [Crossref] [PubMed]
- Li XY, Hou HT, Chen HX, et al. Preoperative plasma biomarkers associated with atrial fibrillation after coronary artery bypass surgery. J Thorac Cardiovasc Surg 2020. [Epub ahead of print]. [Crossref] [PubMed]
- He GW, Hou HT, Xuan C, et al. Corrective surgery alters plasma protein profiling in congenital heart diseases and clinical perspectives. Am J Transl Res 2020;12:1319-37. [PubMed]
- Xuan C, Gao G, Yang Q, et al. Proteomic study reveals plasma protein changes in congenital heart diseases. Ann Thorac Surg 2014;97:1414-9. [Crossref] [PubMed]
- Zhang X, Hou HT, Wang J, et al. Plasma proteomic study in pulmonary arterial hypertension associated with congenital heart diseases. Sci Rep 2016;6:36541. [Crossref] [PubMed]
- Zhang X, Wang K, Yang Q, et al. Acute phase proteins altered in the plasma of patients with congenital ventricular septal defect. Proteomics Clin Appl 2015;9:1087-96. [Crossref] [PubMed]
- Guo ZP, Hou HT, Jing R, et al. Plasma protein profiling in patients undergoing coronary artery bypass grafting surgery and clinical significance. Oncotarget 2017;8:60528-38. [Crossref] [PubMed]
- Pierce A, Unwin RD, Evans CA, et al. Eight-channel iTRAQ enables comparison of the activity of six leukemogenic tyrosine kinases. Mol Cell Proteomics 2008;7:853-63. [Crossref] [PubMed]
- Kanehisa M, Furumichi M, Tanabe M, et al. KEGG: new perspectives on genomes, pathways, diseases and drugs. Nucleic Acids Res 2017;45:D353-61. [Crossref] [PubMed]
- Wu J, Corr PB. Influence of long-chain acylcarnitines on voltage-dependent calcium current in adult ventricular myocytes. Am J Physiol 1992;263:H410-7. [PubMed]
- Fabritz L, Guasch E, Antoniades C, et al. Expert consensus document: Defining the major health modifiers causing atrial fibrillation: a roadmap to underpin personalized prevention and treatment. Nat Rev Cardiol 2016;13:230-7. [Crossref] [PubMed]
- Lefebvre P, Chinetti G, Fruchart JC, et al. Sorting out the roles of PPAR alpha in energy metabolism and vascular homeostasis. J Clin Invest 2006;116:571-80. [Crossref] [PubMed]
- Khera AV, Millar JS, Ruotolo G, et al. Potent peroxisome proliferator-activated receptor-? agonist treatment in-creases cholesterol efflux capacity in humans with the metabolic syndrome. Eur Heart J 2015;36:3020-2. [Crossref] [PubMed]
- Kliewer SA, Umesono K, Noonan DJ, et al. Convergence of 9-cis retinoic acid and peroxisome proliferator signalling pathways through heterodimer formation of their receptors. Nature 1992;358:771-4. [Crossref] [PubMed]
- Bishop-Bailey D. Peroxisome proliferator-activated receptors in the cardiovascular system. Br J Pharmacol 2000;129:823-34. [Crossref] [PubMed]
- Mukherjee R, Locke KT, Miao B, et al. Novel peroxisome proliferator-activated receptor alpha agonists lower low-density lipoprotein and triglycerides, raise high-density lipoprotein, and synergistically increase cholesterol excretion with a liver X receptor agonist. J Pharmacol Exp Ther 2008;327:716-26. [Crossref] [PubMed]
- Kirchhof P, Fabritz L. Of hammers and screws: renin-angiotensin-aldosterone system inhibition to prevent atrial fibrillation in patients with hypertension. Eur Heart J 2014;35:1169-71. [Crossref] [PubMed]
- Tsai CT, Lai LP, Lin JL, et al. Renin-angiotensin system gene polymorphisms and atrial fibrillation. Circulation 2004;109:1640-6. [Crossref] [PubMed]
- Ott EB, van den Akker NM, Sakalis PA, et al. The LMO7 is important in zebrafish heart development. Dev Dyn 2008;237:3940-52. [Crossref] [PubMed]
- Wang Y, Liu XC, Zhang GW, et al. A new transmyocardial degradable stent combined with growth factor, heparin, and stem cells in acute myocardial infarction. Cardiovasc Res 2009;84:461-9. [Crossref] [PubMed]
(English Language Editor: A. Kassem)