Machine learning models to predict red blood cell transfusion in patients undergoing mitral valve surgery
Introduction
Blood transfusion is widely used in surgery. Cardiac surgery uses the largest amount of blood products among surgeries, with a rate of blood transfusion ranging from 40% to 90% (1-3). However, this treatment is a double-edged sword. Transfusion has been associated with high rates of morbidity and mortality in critically ill patients (4). Some recent studies have shown worse outcomes incurred by blood transfusion, including increased renal failure and infection, as well as respiratory, circulatory, and neurological complications after cardiac surgery (5,6). A review of studies on cardiac surgery have shown that red blood cell (RBC) transfusion is associated with an increased risk of postoperative infections and mortality (7-9). It is widely acknowledged blood products are often wasted, where this may reflect a lack of application of evidence-based measures to blood transfusion (10-12). In some instances, the patient’s need for RBC transfusion is based on personal experience, and this leads to a waste of blood and burdens the medical staff (13). Transfusion is now recognized as among the most overused treatments in modern medicine (14). Owing to the large amount of blood used and the many factors affecting blood transfusion in cardiac surgery, few studies have used models of blood transfusion in cardiac surgery to examine the issue. Related research used traditional statistical models to predict the relevant factors that affect large volume blood transfusion (LVBT) results in thoraco-abdominal aortic aneurysm (TAAA) surgery. However, only few independent predictors are available for clinical practice (15).
Artificial intelligence (AI) is increasingly being used to aid diagnosis, treatment, automatic classification, and rehabilitation in medicine. The machine learning algorithm is an AI technique designed to simulate human intelligence by discovering patterns of reasoning about the available data (16). Given basic data, machine learning algorithms can be used to predict the relevant information, such as whether blood transfusion is needed. Because patients who have had mitral valve disease have good homogeneity, mitral valve disease has a set of standard procedures for diagnosis and treatment, the comparison of patient data is comparable and are easier to operate on, the amount of bleeding does not change significantly with surgeon during the operation. We use machine learning models to explore the risk-related factors that influence blood transfusion during mitral valve surgery, and accurately provide the boundaries of these factors to guide the surgeon’s assessment of patients’ need for intraoperative blood transfusion. We present the following article in accordance with the STROBE reporting checklist (available at http://dx.doi.org/10.21037/atm-20-7375).
Methods
The study was conducted in accordance with the Declaration of Helsinki (as revised in 2013). This study was approved by the Ethical Committee of Zhongshan Hospital affiliated to Fudan University (No. B2020-218) and individual consent for this retrospective analysis was waived.
Database
The data was drawn from details of 698 patients undergoing isolated mitral or simultaneous tricuspid valve surgery at the Department of Cardiology of Zhongshan Hospital from January to December of 2019, where the surgeries included conventional and minimally invasive approaches. The data included demographic characteristics and the relevant variables before, during, and after surgery. The data extraction techniques included system extraction and manual collection. As usual, the missing values of the measurement data were inserted using the average value while those of counting data were inserted according to the most frequently occurring value.
Patients
Patients who had the maze operation, aortic valve surgery, and atrial septal repair were excluded, as were patients with a history of heart surgeries (excluding interventional therapy). Postoperative complications related to sepsis were defined as those with pathogens that occur in two blood cultures. Preoperative patients were categorized as suffering from mild (HB >90 g/L, but lower than normal), moderate (HB =60–89 g/L), severe (HB =30–59 g/L), and extremely severe anemia (HB <30 g/L) (17). Acute kidney injury (AKI) was defined as an increase in absolute serum creatinine ≥0.3 mg/dL (≥26.5 mol/L) within 48 hours of surgery (18). The severity of all intraoperative valve stenosis or insufficiency was determined according to the results as interpreted by an echocardiographic physician.
Dependent and independent variables
The primary endpoint of this study was “intraoperative red blood cell (RBC) infusion”. Intraoperative RBC infusion refers to the amount of allogeneic RBCs initiated to be injected during the operation, excluding autologous and postoperative blood transfusion (15).
Given the aim of establishing a predictive model, the independent variables were chosen by considering the baseline characteristics of the patients in the context of preoperative and intraoperative variables (Table 1).

Full table
AI model algorithm
The CatBoost algorithm was used to build the AI model. Yandex company proposed and tested the approach using oblivious decision trees as base predictors in 2017 (19) as well as a special method to deal with the characteristics of classification. CatBoost alleviates the problem of overfitting, and improves the generalization ability and robustness of the model, which is particularly suitable for small sample sizes and unbalanced data.
Prediction migration is often a problem in modeling. In each iteration of the gradient boosting decision tree (GBDT), the loss function uses the same dataset to obtain the gradient of the model to train the base learner. This leads to a deviation in the estimated gradient, which in turn leads to the problem of overfitting. CatBoost replaces the method of gradient estimation of the traditional algorithm with order boosting, which reduces bias and improves the generalization ability of the model.
The SHapley Additive exPlanations (SHAP) evaluator proposed by Lundberg and Lee (20) can be used to explain the predictions produced by a model. Following model training, a partial dependency graph (PDP or PD graph) is used to calculate the SHAP value of each feature to allow clinicians to make more accurate predictions. In this way, the impact of each feature on the model can be represented using Shapley values (21). Using these calculations, a matrix of SHAP values can be obtained to provide a visualization of the contribution of each feature to the model predictions. This helps explain the role of each feature in the model in an intuitively understandable way.
Statistical analysis
SPSS 25.0 as well as Python 3.6, with the Python packages Scikit-learn, SHAP (feature analysis), and matplotlib (visualization), were used in. We described the continuous variables of the normal distribution using the mean and standard deviation (SD), the continuous variables of the non-normal distribution using the median and quartile values, and the categorical variables using proportion. The Student’s t-test and was used to identify statistically significant differences between the means of groups. The chi-square test was used to identify any significant association between variables. Mann-Whitney U test was used to compare non-normally distributed variables and variables of ranked data between two groups. First, the variables needed to be screened through univariate analysis to choose the ones with positive results. Second, the screened variables were used for multivariate analysis. We used logistic regression to build a model to mine the relationship between the variables and the outcomes. Third, the machine learning algorithm was used to construct the prediction model for intraoperative blood transfusion. The dataset is randomly divided into training set (70%) and testing set (30%), the training set is implemented to build up a model using 10-fold cross-validation, while the testing set is to validate the model built using the area under the ROC curve (AUC). According to its results, the characteristic values and risk factors were mined to analyze the effects of the latter on the outcome-related variables.
Results
RBC consumption
Of the 677 patients considered, 166 (24.52%) had received intraoperative RBC transfusion, where the amount of RBC transfusion had varied from 2 to 10 Units. The average RBC consumption was 0.71±1.43 Units.
Building traditional models of RBC transfusion
Univariate analysis was performed on all independent variables, and P<0.1 was used for screening. Independent variables with P<0.1 were shown in Table 2.
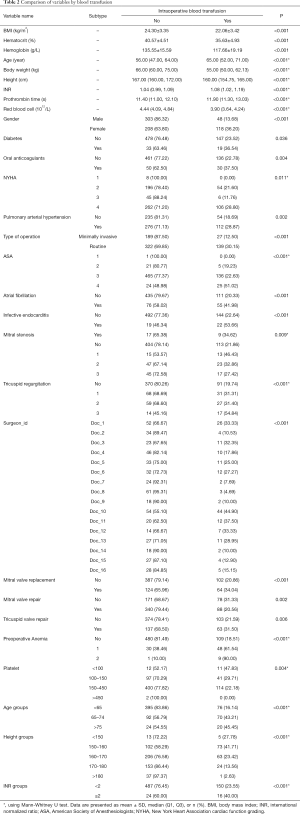
Full table
Variables screened by a single factor were entered into the logistic model and screened backward. Variables were eliminated when P>0.1. Those with lower HCT, lower body mass index (BMI), longer PT, females, diabetics, those undergoing routine surgery, patients with atrial fibrillation, severe mitral stenosis, preoperative anemia, and older patients had increased likelihood of the need for of RBC transfusion. In addition, different surgeons are associated with the need for RBC transfusion (Table 3).
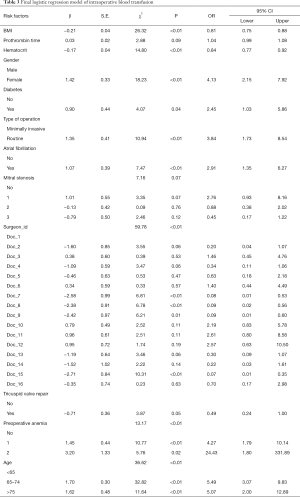
Full table
AI model
The 70% database was used as the training set and 30% as the testing set. Thirteen machine learning algorithms were used for calculation. The training set used 10-fold cross-validation, and the results are shown in the table below. The CatBoost model delivered the best performance with an AUC of 0.888 (95% CI: 0.845–0.909) (Table 4).
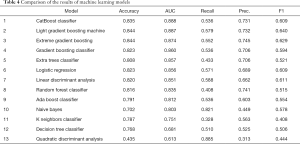
Full table
Further analysis was performed using the CatBoost model, and the importance of the features was analyzed using their SHAP values (Figure 1). The main effects of each factor and the outcome variables are shown in Figure 2. Different surgeons also influenced this probability (Figure 3).
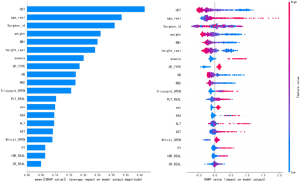
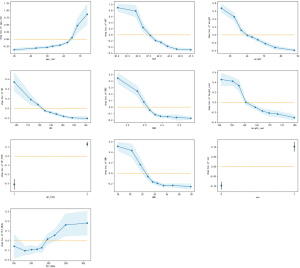
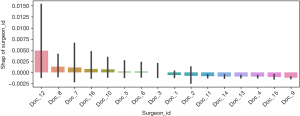
Further analysis using the CatBoost model revealed that hematocrit (<37.81%), age (>64 y), body weight (<59.92 kg), BMI (<22.56 kg/m2), hemoglobin (<122.6 g/L), type of surgery (median thoracotomy surgery), height (<160.61 cm), platelet (>194.12×109/L), RBC (<4.08×1012/L), and gender (female) were the main factors influencing the likelihood of blood transfusion (Figure 4).
Figure 2 shows that platelet was positively correlated with RBC transfusion. But its size was related to the coagulation function of the patient. The higher the platelet was, the better the coagulation function was, and the smaller the amount of intraoperative bleeding that occurred. This reduced the probability of transfusion. This variable is further analyzed in Figure 5, which shows that the relationship between platelet and RBC transfusion was stratified. When platelet was less than 194.5×109/L, platelet was positively correlated with RBC transfusion. When platelet was greater than 203.5×109/L, its correlation was negative.
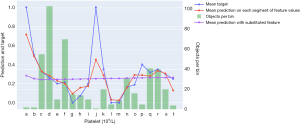
Results of prediction and analysis of RBC transfusion models
A total of 204 patients were tested, with an AUC of 0.922 (95% CI: 0.883–0.956), 177 of whom were predicted accurately (86.8%) and 10 were too large (the patient did not receive a blood transfusion) and 17 were too small (the patient did receive a blood transfusion) (Table 5).
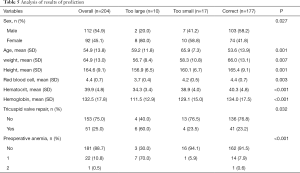
Full table
The group that was predicted more accurately had more females (80% vs. 41.8%), higher age (mean ± SD, 59.2±11.8 vs. 53.6±13.9 years), lower weight (mean ± SD, 56.7±8.4 vs. 66±13.1 kg), lower height (mean ± SD, 156.9±6.5 vs. 165.4±9.1 cm), lower RBC (mean ± SD, 3.7±0.4×1012/L vs. 4.4±0.7×1012/L), lower hematocrit (mean ± SD, 34.3%±3.4% vs. 40.3%±4.8%), lower preoperative hemoglobin (mean ± SD, 111.5±12.9 vs. 134.0±17.5 g/L), more tricuspid valve repair (60% vs. 23.2%), and a higher percentage of patients with preoperative anemia (70% vs. 8.5%).
Discussion
Table 3 shows that the factors influencing blood transfusion extracted from the traditional logistic regression model. Figure 1 shows that the machine learning model identified the influential factors. Thus, the factors excavated by the machine learning model and logistic model were roughly the same. Moreover, the factors identified by our model were consistent with the conclusions of previous studies (13). In addition, the machine learning model accurately gave the specific boundary values of the factors. (Figure 4), which will help clinicians in their judgment of blood transfusion in patients undergoing preoperative surgery.
Research on predicting the need for transfusion in cardiac surgery is rare. Many factors affect the blood used in cardiac surgery, and the traditional model cannot identify all factors influencing this or predict whether patient needs intraoperative blood transfusion (22). We use the machine learning to build a model to predict the need for blood transfusion among patients, and yielded an accuracy of up to 86.8%. The model can play an important role in clinical guidance.
Based on clinical observations, some researchers have suggested that hematocrit should be maintained at around 30% and hemoglobin concentration at 10 g/dL (23). However, this threshold has been reconsidered due to risks associated with transfusion and a greater appreciation of the importance of varying physiological responses to anemia (24). Due to the particularity of heart surgery, intraoperative extracorporeal circulation requires the heparinization of the patient's blood, and the operating time is long. This increases the risk of intraoperative bleeding. Our machine learning model thus considered cases of intraoperative heparin and the duration of the operation. The dangerous boundary of hemoglobin content was found at 12 g/dL and hematocrit to be 38%.
When platelet was less than 194.5, platelet was positively correlated with RBC transfusion. When platelet was greater than 203.5, its correlation was negative. We think that the increase in platelet was not due to each patient’s thrombocytosis, but perhaps because of acute infection, blood loss, or hemolysis. This suggests that patient might already have lost blood to increase the likelihood of the need for blood transfusion (25).
We used the machine learning model to analyze the surgeons, and found that Doctor 12 (33.3%, 0.76±1.19 U), Doctor 8 (4.7%, 0.22±1.29 U), and Doctor 7 (7.7%, 0.42±1.65 U) were positively correlated with the likelihood of blood transfusion. Subsequent studies can use machine learning models to intervene in the doctors’ choice of administering blood transfusion to reduce the unnecessary use of blood (Figure 3).
Tables 2,3 and Figures 1,2,4 shows that women (36.2%, 1.06±1.62 U) were more likely to receive blood transfusion. Owing to physical blood loss, many women develop anemia during surgery, which was the direct cause of their high intraoperative blood transfusion rate (26). The mode of surgery was also an important factor. The traditional surgical method involves direct midline thoracotomy into the heart while the minimally invasive method involves using the intercostal space to enter the area without requiring midline thoracotomy. Compared with the traditional surgery group (30.2%, 0.88±1.56), minimally invasive surgery (12.5%, 0.36±1.01) can significantly reduce the amount of blood needed, which is consistent with previous reports (27). Tricuspid valve repair was also an important factor influencing blood transfusion in the traditional model and the machine learning model. Functional regurgitation is noted during mitral valve surgery, synchronous repair (class I) is recommended (28). The tricuspid valve regurgitation can cause anemia, thrombocytopenia, coagulation disorders, hepatic failure, and other complications (29), leading to a higher likelihood of the need for blood transfusion.
Although our data were relatively complete and accurate, the analysis was retrospective and covered a single center. Short-term postoperative analysis was carried out for cases of incorrect prediction, but the relevant patients were not followed up with to better understand the impact of blood transfusion on their recovery. Our model is undergoing further development, and does not predict the amount of blood needed for transfusion. Moreover, it can predict the need for blood transfusion for only one type of surgery.
Conclusions
We used machine learning model to predict the need for RBC transfusion in cardiac surgery. It can help guide surgeons in clinic al practice.
Acknowledgments
Funding: This work was supported by Clinical Technology Innovation Project of Shanghai Shenkang Hospital Development Center (SHDC12018615); Science Fund for Management of Zhongshan Hospital affiliated to Fudan University (2019ZSGL07); National Natural Science Foundation of China Youth Science Foundation Project (81801743).
Footnote
Reporting Checklist: The authors have completed the STROBE reporting checklist. Available at http://dx.doi.org/10.21037/atm-20-7375
Data Sharing Statement: Available at http://dx.doi.org/10.21037/atm-20-7375
Conflicts of Interest: All authors have completed the ICMJE uniform disclosure form (available at http://dx.doi.org/10.21037/atm-20-7375). The authors have no conflicts of interest to declare.
Ethical Statement: The authors are accountable for all aspects of the work in ensuring that questions related to the accuracy or integrity of any part of the work are appropriately investigated and resolved. The study was conducted in accordance with the Declaration of Helsinki (as revised in 2013). This study was approved by the Ethical Committee of Zhongshan Hospital affiliated to Fudan University (No. B2020-218) and individual consent for this retrospective analysis was waived.
Open Access Statement: This is an Open Access article distributed in accordance with the Creative Commons Attribution-NonCommercial-NoDerivs 4.0 International License (CC BY-NC-ND 4.0), which permits the non-commercial replication and distribution of the article with the strict proviso that no changes or edits are made and the original work is properly cited (including links to both the formal publication through the relevant DOI and the license). See: https://creativecommons.org/licenses/by-nc-nd/4.0/.
References
- Rogers MA, Blumberg N, Saint S, et al. Hospital variation in transfusion and infection after cardiac surgery: a cohort study. BMC Med 2009;7:37. [Crossref] [PubMed]
- Stover EP, Siegel LC, Parks R, et al. Variability in transfusion practice for coronary artery bypass surgery persists despite national consensus guidelines: a 24-institution study. Institutions of the Multicenter Study of Perioperative Ischemia Research Group. Anesthesiology 1998;88:327-33. [Crossref] [PubMed]
- Snyder-Ramos SA, Mohnle P, Weng YS, et al. The ongoing variability in blood transfusion practices in cardiac surgery. Transfusion 2008;48:1284-99. [Crossref] [PubMed]
- Marik PE, Corwin HL. Efficacy of red blood cell transfusion in the critically ill: a systematic review of the literature. Crit Care Med 2008;36:2667-74. [Crossref] [PubMed]
- Engoren MC, Habib RH, Zacharias A, et al. Effect of blood transfusion on long-term survival after cardiac operation. Ann Thorac Surg 2002;74:1180-6. [Crossref] [PubMed]
- Leal-Noval SR, Rincon-Ferrari MD, Garcia-Curiel A, et al. Transfusion of blood components and postoperative infection in patients undergoing cardiac surgery. Chest 2001;119:1461-8. [Crossref] [PubMed]
- Michalopoulos A, Tzelepis G, Dafni U, et al. Determinants of hospital mortality after coronary artery bypass grafting. Chest 1999;115:1598-603. [Crossref] [PubMed]
- Koch CG, Li L, Duncan AI, et al. Morbidity and mortality risk associated with red blood cell and blood-component transfusion in isolated coronary artery bypass grafting. Crit Care Med 2006;34:1608-16. [Crossref] [PubMed]
- Murphy GJ, Reeves BC, Rogers CA, et al. Increased mortality, postoperative morbidity, and cost after red blood cell transfusion in patients having cardiac surgery. Circulation 2007;116:2544-52. [Crossref] [PubMed]
- Reeves BC, Murphy GJ. Increased mortality, morbidity, and cost associated with red blood cell transfusion after cardiac surgery. Curr Opin Cardiol 2008;23:607-12. [Crossref] [PubMed]
- Khanna MP, Hébert PC, Fergusson DA. Review of the clinical practice literature on patient characteristics associated with perioperative allogeneic red blood cell transfusion. Transfus Med Rev 2003;17:110-9. [Crossref] [PubMed]
- Moskowitz DM, Klein JJ, Shander A, et al. Predictors of transfusion requirements for cardiac surgical procedures at a blood conservation center. Ann Thorac Surg 2004;77:626-34. [Crossref] [PubMed]
- Clevenger B, Mallett SV, Klein AA, et al. Patient blood management to reduce surgical risk. Br J Surg 2015;102:1325-37; discussion 1324. [Crossref] [PubMed]
- Anthes E. Evidence-based medicine: Save blood, save lives. Nature 2015;520:24-6. [Crossref] [PubMed]
- Pieri M, Nardelli P, De Luca M, et al. Predicting the Need for Intra-operative Large Volume Blood Transfusions During Thoraco-abdominal Aortic Aneurysm Repair. Eur J Vasc Endovasc Surg 2017;53:347-53. [Crossref] [PubMed]
- Chan YK, Chen YF, Pham T, et al. Artificial Intelligence in Medical Applications. J Healthc Eng 2018;2018:4827875 [Crossref] [PubMed]
- McLean E, Cogswell M, Egli I, et al. Worldwide prevalence of anaemia, WHO Vitamin and Mineral Nutrition Information System, 1993-2005. Public Health Nutr 2009;12:444-54. [Crossref] [PubMed]
- Levey AS, Eckardt KU, Dorman NM, et al. Nomenclature for kidney function and disease: report of a Kidney Disease: Improving Global Outcomes (KDIGO) Consensus Conference. Kidney Int 2020;97:1117-29. [Crossref] [PubMed]
- Prokhorenkova L, Gusev G, Vorobev A, et al. editors. CatBoost: unbiased boosting with categorical features. Advances in neural information processing systems; 2018.
- Lundberg S, Lee SI. A Unified Approach to Interpreting Model Predictions. 2017.
- Štrumbelj E, Kononenko I. Explaining prediction models and individual predictions with feature contributions. Knowledge and Information Systems 2014;41:647-65. [Crossref]
- Chang C, Hung J, Hu Y, et al. Prediction of Preoperative Blood Preparation for Orthopedic Surgery Patients: A Supervised Learning Approach. Appl Sci 2018;8:1559. [Crossref]
- Musallam KM, Tamim HM, Richards T, et al. Preoperative anaemia and postoperative outcomes in non-cardiac surgery: a retrospective cohort study. Lancet 2011;378:1396-407. [Crossref] [PubMed]
- Vincent JL, Piagnerelli M. Transfusion in the intensive care unit. Crit Care Med 2006;34:S96-101. [Crossref] [PubMed]
- Schafer AI. Thrombocytosis. N Engl J Med 2004;350:1211-9. [Crossref] [PubMed]
- Mirza FG, Abdul-Kadir R, Breymann C, et al. Impact and management of iron deficiency and iron deficiency anemia in women's health. Expert Rev Hematol 2018;11:727-36. [Crossref] [PubMed]
- Grant SW, Hickey GL, Modi P, et al. Propensity-matched analysis of minimally invasive approach versus sternotomy for mitral valve surgery. Heart 2019;105:783-9. [Crossref] [PubMed]
- Nishimura RA, Otto CM, Bonow RO, et al. 2014 AHA/ACC guideline for the management of patients with valvular heart disease: a report of the American College of Cardiology/American Heart Association Task Force on Practice Guidelines. J Thorac Cardiovasc Surg 2014;148:e1-e132. [Crossref] [PubMed]
- Kelly BJ, Luxford JMH, Butler CG, et al. Severity of tricuspid regurgitation is associated with long-term mortality. J Thorac Cardiovasc Surg 2018;155:1032-1038.e2. [Crossref] [PubMed]