Intra-abdominal infection in acute pancreatitis in eastern China: microbiological features and a prediction model
Introduction
Acute pancreatitis is an inflammatory condition of the pancreas that is also known to involve the peri-pancreatic tissues (1). It has various underlying etiologies, such as acute cholecystitis, hyperlipidemia, and excessive alcohol consumption (1). Secondary intra-abdominal infection of acute pancreatitis is one of the leading complications that has been of great concern in recent years. Epidemiological studies have shown that infection of the pancreas and peripancreatic necrosis occurs in about 20–40% of patients with severe acute pancreatitis, which can cause life-threatening organ dysfunction and lead to poor prognosis (2). In a meta-analysis of 6,970 patients, it was indicated that the mortality rate could be as high as 35.2% in acute pancreatitis with intra-abdominal infection and organ failure (3). Therefore, the early identification of these infectious diseases is a major challenge in managing patients with acute pancreatitis, especially in severe cases.
Prophylactic antibiotics in acute pancreatitis was once believed to reduce the incidence of infection-related complications (4,5). However, the effects of treatment are still controversial due to the heterogeneity of enrolled patients in clinical trials and different antimicrobial applications (6). The abuse of antibiotics will lead to gut microflora dysbiosis and increased medical expenses (7). Therefore, it is important to identify those at high risk of developing an infection and to recognize microbiological features after infection onset. It was reported that some of the host factors like diabetes and gallstones were once recognized as the risk factors for secondary intra-abdominal infection (1,2). However, no comprehensive systematic analysis with large sample size has been performed and the valuable information provided to clinical practice is limited. Thus, the present study was performed to establish a reliable clinical prediction model by least absolute shrinkage and selection operator (LASSO) regression and was further validated in the logistic regression model, random forest model, and artificial neural network model, respectively. With the valuable nomogram established, we hope it could optimize the antimicrobial prescription for acute pancreatitis patients with suspected intra-abdominal infection.
We present the following article in accordance with the TRIPOD reporting checklist (available at http://dx.doi.org/10.21037/atm-21-399).
Methods
The retrospective clinical study was conducted at 6 pancreatitis diagnosis and treatment centers in eastern China (Ruijin Hospital, Ren Ji Hospital, Zhongda Hospital, Sir Run Run Shaw Hospital, The Second Zhejiang Hospital, and Shu Guang Hospital). The analyzed data were obtained from electronic medical records of each hospital between January 2015 and June 2020 by 6 attending physicians. The Ethics Committee of Shanghai Jiaotong University School of Medicine approved the study (No. 2019-RES-082), and the study was conducted in accordance with the declaration of Helsinki (as revised in 2013). Written informed consent was obtained from all patients.
Study population
Inpatients who were diagnosed with acute pancreatitis and aged 18–80 years were included in the study. The exclusion criteria were as follows: (I) was diagnosed with an extra-abdominal infectious disease during hospital stay; (II) was pregnancy; and (III) had incomplete medical history.
Extra-abdominal infectious diseases include bloodstream infections, pneumonia (hospital-acquired pneumonia or ventilator-acquired pneumonia), urinary tract infections, and skin and soft tissue infections. All diagnostic criteria of the infection were based on the practice guideline of the European Society of Clinical Microbiology and Infectious Diseases (8).
Disease definitions
Based on the guidelines of the World Society of Emergency Surgery 2019 (1), acute pancreatitis can be diagnosed by any 2 of the following 3 criteria: (I) acute epigastric pain, which can radiate to the back or waist; (II) the level of amylase and/or lipase in blood samples is at least 3 times higher than the normal limit; and (III) enhanced computed tomography (CT) or magnetic resonance imaging (MRI) was performed, and typical pancreatic lesions can be detected.
Secondary intra-abdominal infection is suspected in patients with acute pancreatitis when the 1 of the following criteria is met: (I) new-onset fever (body temperature >38.5 °C) or persistent fever; (II) the level of inflammatory biomarkers [leukocyte count, neutrophil count, procalcitonin (PCT), and C-reactive protein (CRP)] is increased; and (III) clinical symptoms of continuous deterioration and organ dysfunction are detected. The diagnostic methods consist of the following: (I) gas configurations in the necrotic pancreatic tissue can be detected via enhanced CT (Figure S1); and (II) positive culture results are acquired from the fine-needle aspiration (FNA) sample (1,2).
Identification of strains and antimicrobial susceptibility testing operation
CT-guided FNA was performed to obtain drainage fluid from peri-pancreatic tissues (Figure S2). To identify pathogens, samples were gram and fluorescence stained and were also amplified by bacteria culture. Identification of pathogens was also conducted by the VITEK-2 compact automated microbiology system (BioMerienx, France, Version 1.7), and the results of the antimicrobial susceptibility testing were determined based on the standards of the Clinical and Laboratory Standards Institute (CLSI). Information on the pathogens that caused intra-abdominal infection was obtained from each research center’s microbiological database.
Only the first isolated pathogen from the drainage fluid samples was tested in our study. Multidrug-resistant (MDR) strain was defined as acquired non-susceptibility to at least 1 agent in 3 or more antimicrobial categories (9). China Antimicrobial Surveillance Network data were obtained from the internet (http://www.chinets.com) to compare antimicrobial susceptibility.
Clinical assessment and data collection
Clinical medical information of the hospitalized acute pancreatitis patients included in the present study was obtained from the electronic medical record management system of each hospital. The clinical characteristics of the enrolled patients were as follows: (I) basic information, including age, sex, comorbidity (hypertension, diabetes), and body mass index; (II) etiology of pancreatitis; (III) severity of the disease [Acute Physiology and Chronic Health Evaluation II (APACHE II) score, CT severity index (CTSI)]; (IV) intra-abdominal pressure (IAP); (V) biomarkers of inflammation (PCT, CRP, and white blood cell counts); (VI) change in coagulation index; and (VII) change in clinical biochemical index on days 1, 3, 7, and 14 and the prognosis (intra-abdominal infection, dead).
Statistical analysis
Categorical variables were presented as counts (n) and percentages (%), and compared using χ2-test or Fisher’s exact test. Normally distributed variables were compared using Student’s t-test, and were expressed as means (standard deviations), whereas non-normally distributed data was compared using Wilcoxon rank sum test and reported as medians (interquartile range). Kaplan-Meier plot and log-rank test were used for the survival analysis.
To identify risk factors for intra-abdominal infection in patients with acute pancreatitis, we applied a series of artificial intelligence algorithms. The LASSO regression model with the “lambda.1se” criterion was used for the selection of variables. This analytic strategy enables us to exclude non-essential variables, retaining a subset of the most important variables for outcome prediction. The predictive performance of the selected variables was further validated in the logistic regression model, random forest model, and artificial neural network model, respectively. Model discrimination and calibration were assessed by receiver-operating characteristic (ROC) curves and calibration curves, respectively. In addition, ROC curve parameters, including sensitivity, specificity, correctly classified rate, positive likelihood ratio, and negative likelihood ratio, were also computed for each model. To reduce the risk of overfitting, the whole cohort was randomly divided into the training cohort and validation cohort with a ratio of 6:4. In each model, the training cohort was used for model development and the validation cohort was used for the unbiased assessment of model performance. Finally, a novel nomogram based on the selected predictors was established to provide individualized risk of developing intra-abdominal infection in patients with acute pancreatitis. Statistical analyses were performed using R (University of Auckland, New Zealand, Version 3.6.2), SPSS (IBM, USA, Version 21.0), and GraphPad Prism (GraphPad Software, USA, Version 7.0). A 2-sided P value <0.05 was considered statistically significant (10,11).
Results
Study population
A total of 874 hospitalized patients who were diagnosed with acute pancreatitis were initially screened, and of these, 163 were excluded due to factors, such as extra-abdominal infection and pregnancy. Finally, 711 patients were included in the study and 182 (25.6%) of these were diagnosed with intra-abdominal infection (Figure 1).
Isolated bacteria and antimicrobial susceptibility
In total, 247 pathogens from 182 FNA samples were isolated. Of these, 146 (59.1%) were gram-negative bacteria, 47 (19%) were gram-positive bacteria, while the other 32 were fungi. Enterobacteriaceae accounted for the main gram-negative bacteria. Forty-five (18.2%) pathogens were identified as MDR bacteria (Table 1). Antimicrobial susceptibility of the 4 main gram-negative bacteria (Escherichia coli, Klebsiella pneumoniae, Pseudomonas aeruginosa, and Acinetobacter baumannii) is shown in Figure 2, and a relatively high incidence of resistance to carbapenem and 3rd-generation cephalosporin was indicated. For gram-positive bacteria, vancomycin, linezolid, teicoplanin, and tigecycline were considered the best choices for antimicrobial susceptibility (close to 100%) (Figure S3).
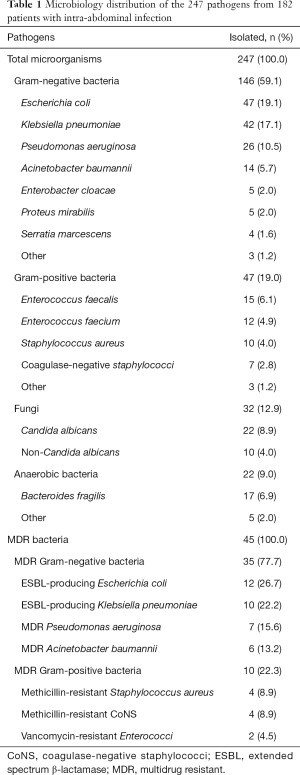
Full table
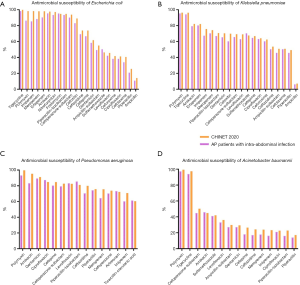
Clinical features and outcomes of patients with or without intra-abdominal infection
The clinical features of patients with or without intra-abdominal infection are listed in Table 2. It was indicated that more acute pancreatitis patients with intra-abdominal infection were admitted to intensive care unit (ICU). A high CTSI, APACHE II score, and IAP were found in the intra-abdominal infection group, as well as more patients with diabetes and excessive alcohol consumption (Table 2). The laboratory results showed a higher serum PCT level and neutrophil count in patients with intra-abdominal infection (Tables S1-S4).
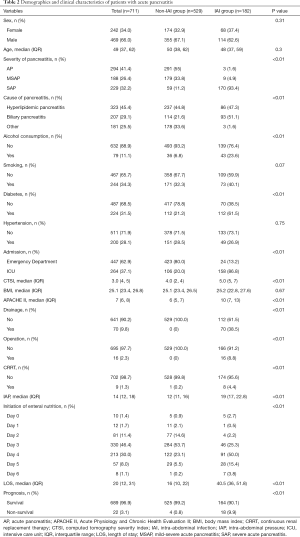
Full table
It was also noted that high mortality (9.9% vs. 0.8%) and long hospital stay (40.5 vs. 16 days) were associated with acute pancreatitis patients diagnosed with intra-abdominal infection (P<0.01) (Figure 3).
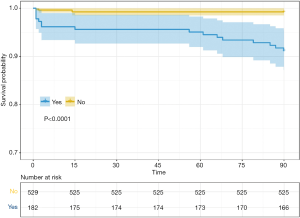
Identification and validation predictive factors for intra-abdominal infection in patients with acute pancreatitis
Variable selection using the LASSO regression model
The whole cohort was randomly divided into the training cohort and validation cohort with a ratio of 6:4. The demographic and clinical data of patients in the training and validation groups are summarized in Table 3 and Table S5. Most of the included variables and outcomes were well balanced between the two groups. Using the LASSO model with the “lambda.1se” criterion, 5 variables (intra-abdominal pressure, APACHE II score, CTSI, ICU admission, and severity grade) were retained for subsequent model construction and intra-abdominal infection prediction (Figure 4).
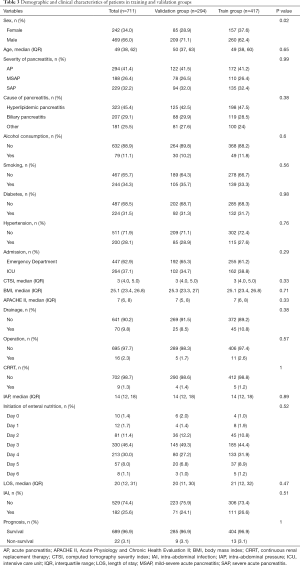
Full table
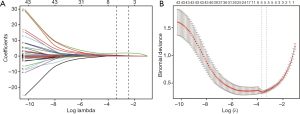
Development, validation, and assessment of models for predicting intra-abdominal infection
Three predictive models were built by incorporating the five selected variables. The logistic regression model (Figure 5), random forest model (Figure 6), and artificial neural network (Figure 7) were used. ROC curve parameters at the optimal cut-off point according to different models were compared (Table 4). It was indicated that all of the areas under the curve were >0.95 and had high sensitivity and specificity in both the training set and validation set (all >93%). In addition, calibration curves indicated good agreement between predicted probability and observed probability for intra-abdominal infection in both the training set and validation set.
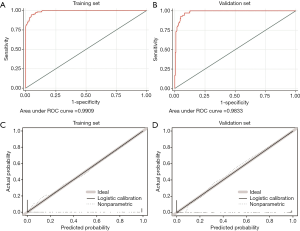
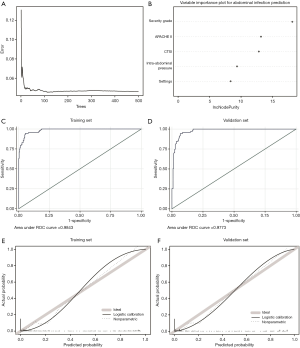
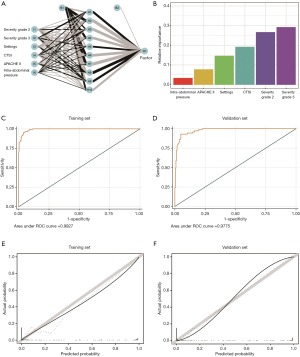
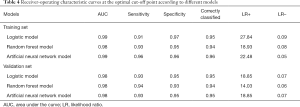
Full table
Estimating the probability of intra-abdominal infection using the nomogram
For the development of the nomogram, we incorporated the five selected predictors as prognostic features: intra-abdominal pressure, APACHE II score, CTSI, ICU admission, and severity grade. This novel 5 predictor-based nomogram can be utilized to predict individualized risk of intra-abdominal infection in patients with acute pancreatitis (Figure 8).
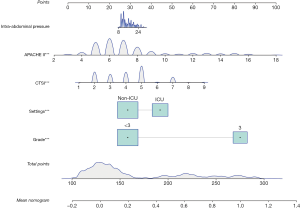
Discussion
Data of the 711 patients with acute pancreatitis were analyzed in our study and indicated that gram-negative bacteria were still the main cause of intra-abdominal infection with high antimicrobial resistance trends. A new nomogram model that consisted of five risk factors (IAP, ICU admission, APACHE II score, CTSI, and severity of pancreatitis) was built and verified, and revealed a high predictive value.
Gut is the largest microecological reservoir of the human body which is mainly filled with Enterococcus, Enterobacter and Bacteroides fragilis (1,12). As an inflammatory disease, the permeability of intestinal mucosa could be increased by neutrophil-derived elastase, which could also destroy the tissue, increase the vascular permeability, and promotes the microecological imbalance. Thus, during the period of acute pancreatitis, the integrity of mucosa could also be destroyed. Therefore, bacteria translocation was easily recognized from the intestine to abdomen, and Enterococcus or Enterobacter could be isolated from the FNA samples (12). What’s more, high concentrations of inflammatory mediators like IL-1 and IL-6 created during the cause of acute pancreatitis could lead to the obstruction of lymphatic reflux which is a “helping hand” to bacteria translocation (13).
Most abdominal infections secondary to acute pancreatitis should be classified as hospital-acquired infections. For patients with severe acute pancreatitis, especially for those who need to be admitted to the ICU, isolated pathogens were often MDR, which might relate to the previous antimicrobial exposure in the ICU (13,14). For gram-negative bacteria, the β-lactam/β-lactamase inhibitor complex was a better choice than carbapenem. For gram-negative bacteria, vancomycin, linezolid, and teicoplanin were found to have high susceptibility.
The APACHE II score can be used to comprehensively evaluate the acute and chronic physiological status of patients (15). The acute physiology score includes 12 physiological measurements, such as body temperature and respiratory rate, that clearly reflects the inflammatory state (16). Chronic health evaluation can assess the immune condition of the host, plays an important role in the anti-inflammatory response. Therefore, more patients with a high APACHE II score could be recognized in the intra-abdominal infection group (10 vs. 6) in our study, and more patients with a higher score were admitted to the ICU (86.8% vs. 20%), which was proved to be a risk factor for secondary intra-abdominal infection.
Many of the 711 included patients with acute pancreatitis could not be treated with injections alone, but also required drainage of peritoneal effusion (38.5%), operation (8.8%), and continuous renal replacement therapy (4.4%), especially those admitted to the ICU. Surgery and minimally invasive treatment can destroy the integrity of skin and mucosa which pose a hidden danger for secondary infection (17,18).
CT outperforms all other scores, not only for diagnostic accuracy but also for demonstrating the extent of the disease, as well as for guiding interventional procedures. Moreover, contrast-enhanced CT is the gold standard modality in the imaging evaluation of patients with acute pancreatitis (19). In 1990 Balthazar et al. established the CT severity index (CTSI) (20). This system grades pancreatic inflammation and necrosis, and is able to predict the morbidity and mortality of acute pancreatitis. Therefore, it was still found to be an independent risk factor for intra-abdominal infection, as well as IAP.
A prediction model with 5 main risk factors was built during our 6-year study, and a high predictive value for intra-abdominal infection was confirmed. However, there are many limitations. First, prophylactic antimicrobial application was not further analyzed. Therefore, the relationship between antimicrobial resistance and its consumption could not be established. Second, no indicators related to immune status were analyzed, such as the human leukocyte antigen D-related expression or the CD4+Th/CD8+Th cell ratio, which was reported to be a risk factor for MDR infection in patients with severe acute pancreatitis (21-23). Third, although the sample size of the present study was adequate, the mortality was relatively low, which led to the uncompleted subgroup analysis. Further prospective trials with large sample size are needed in the future to obtain a higher grade of evidence.
Conclusions
Gram-negative bacteria, especially Enterobacteriaceae, are the main cause of intra-abdominal infection. Therefore, more attention should be paid to MDR pathogens. A new nomogram model that consisted of 5 risk factors (IAP, ICU admission, APACHE II score, CTSI, and severity of pancreatitis) was built for intra-abdominal infection prediction for patients with acute pancreatitis. Multicenter prospective validation cohorts should be used in subsequent studies to achieve a higher level of evidence.
Acknowledgments
We would like to express our gratitude to all the staff who participated in the data collection.
Funding: This work was supported by the Scientific Research Project of Shanghai Municipal Health Bureau (No. 201840006).
Footnote
Reporting Checklist: The authors have completed the TRIPOD reporting checklist. Available at http://dx.doi.org/10.21037/atm-21-399
Data Sharing Statement: Available at http://dx.doi.org/10.21037/atm-21-399
Conflicts of Interest: All authors have completed the ICMJE uniform disclosure form (available at http://dx.doi.org/10.21037/atm-21-399). YY serves as an unpaid section editor of Annals of Translational Medicine from Oct 2019 to Sep 2021. The other authors have no conflicts of interest to declare.
Ethical Statement: The authors are accountable for all aspects of the work in ensuring that questions related to the accuracy or integrity of any part of the work are appropriately investigated and resolved. The study was conducted in accordance with the declaration of Helsinki (as revised in 2013). The Ethics Committee of Shanghai Jiaotong University School of Medicine approved the study (No. 2019-RES-082), and written informed consent was obtained from all patients.
Open Access Statement: This is an Open Access article distributed in accordance with the Creative Commons Attribution-NonCommercial-NoDerivs 4.0 International License (CC BY-NC-ND 4.0), which permits the non-commercial replication and distribution of the article with the strict prov iso that no changes or edits are made and the original work is properly cited (including links to both the formal publication through the relevant DOI and the license). See: https://creativecommons.org/licenses/by-nc-nd/4.0/.
References
- Leppäniemi A, Tolonen M, Tarasconi A, et al. 2019 WSES guidelines for the management of severe acute pancreatitis. World J Emerg Surg 2019;14:27. [Crossref] [PubMed]
- Banks PA, Bollen TL, Dervenis C, et al. Classification of acute pancreatitis--2012: revision of the Atlanta classification and definitions by international consensus. Gut 2013;62:102-11. [Crossref] [PubMed]
- Werge M, Novovic S, Schmidt PN, et al. Infection increases mortality in necrotizing pancreatitis: A systematic review and meta-analysis. Pancreatology 2016;16:698-707. [Crossref] [PubMed]
- Bassi C, Larvin M, Villatoro E. Antibiotic therapy for prophylaxis against infection of pancreatic necrosis in acute pancreatitis. Cochrane Database Syst Rev 2003;CD002941 [PubMed]
- Villatoro E, Bassi C, Larvin M. Antibiotic therapy for prophylaxis against infection of pancreatic necrosis in acute pancreatitis. Cochrane Database Syst Rev 2006;CD002941 [Crossref] [PubMed]
- Villatoro E, Mulla M, Larvin M. Antibiotic therapy for prophylaxis against infection of pancreatic necrosis in acute pancreatitis. Cochrane Database Syst Rev 2010;CD002941 [Crossref] [PubMed]
- Cresci GA, Bawden E. Gut Microbiome: What We Do and Don't Know. Nutr Clin Pract 2015;30:734-46. [Crossref] [PubMed]
- Tacconelli E, Cataldo MA, Dancer SJ, et al. ESCMID guidelines for the management of the infection control measures to reduce transmission of multidrug-resistant Gram-negative bacteria in hospitalized patients. Clin Microbiol Infect 2014;20:1-55. [Crossref] [PubMed]
- Magiorakos AP, Srinivasan A, Carey RB, et al. Multidrug-resistant, extensively drug-resistant and pandrug-resistant bacteria: an international expert proposal for interim standard definitions for acquired resistance. Clin Microbiol Infect 2012;18:268-81. [Crossref] [PubMed]
- Fernández-Delgado M, Sirsat MS, Cernadas E, et al. An extensive experimental survey of regression methods. Neural Netw 2019;111:11-34. [Crossref] [PubMed]
- Alhamzawi R, Ali HTM. The Bayesian adaptive lasso regression. Math Biosci 2018;303:75-82. [Crossref] [PubMed]
- Lu JD, Cao F, Ding YX, et al. Timing, distribution, and microbiology of infectious complications after necrotizing pancreatitis. World J Gastroenterol 2019;25:5162-73. [Crossref] [PubMed]
- Li Y, Shen H, Zhu C, et al. Carbapenem-Resistant Klebsiella pneumoniae Infections among ICU Admission Patients in Central China: Prevalence and Prediction Model. Biomed Res Int 2019;2019:9767313 [Crossref] [PubMed]
- Zheng B, Dai Y, Liu Y, et al. Molecular Epidemiology and Risk Factors of Carbapenem-Resistant Klebsiella pneumoniae Infections in Eastern China. Front Microbiol 2017;8:1061. [Crossref] [PubMed]
- Knaus WA, Draper EA, Wagner DP, et al. APACHE II: a severity of disease classification system. Crit Care Med 1985;13:818-29. [Crossref] [PubMed]
- Kumar S, Gattani SC, Baheti AH, et al. Comparison of the Performance of APACHE II, SOFA, and mNUTRIC Scoring Systems in Critically Ill Patients: A 2-year Cross-sectional Study. Indian J Crit Care Med 2020;24:1057-61. [Crossref] [PubMed]
- Yu Y, Shen H, Zhu C, et al. Infections Caused by Extended-Spectrum beta-Lactamase Producing Escherichia Coli in Systemic Lupus Erythematosus Patients: Prevalence, Risk Factors, and Predictive Model. Biomed Res Int 2018;2018:8296720 [Crossref] [PubMed]
- Zhang Z, Smischney NJ, Zhang H, et al. AME evidence series 001-The Society for Translational Medicine: clinical practice guidelines for diagnosis and early identification of sepsis in the hospital. J Thorac Dis 2016;8:2654-65. [Crossref] [PubMed]
- Balthazar EJ. Acute pancreatitis: assessment of severity with clinical and CT evaluation. Radiology 2002;223:603-13. [Crossref] [PubMed]
- Balthazar EJ, Robinson DL, Megibow AJ, et al. Acute pancreatitis: value of CT in establishing prognosis. Radiology 1990;174:331-6. [Crossref] [PubMed]
- Yu ZX, Chen XC, Zhang BY, et al. Association between HLA-DR Expression and Multidrug-resistant Infection in Patients with Severe Acute Pancreatitis. Curr Med Sci 2018;38:449-54. [Crossref] [PubMed]
- Ferrian S, Ross M, Conradie F, et al. Frequency of Circulating CD4(+)Ki67(+)HLA-DR(-) T Regulatory Cells Prior to Treatment for Multidrug Resistant Tuberculosis Can Differentiate the Severity of Disease and Predict Time to Culture Conversion. Front Immunol 2018;9:2438. [Crossref] [PubMed]
- Shen H, Yu Y, Chen SM, et al. Dectin-1 Facilitates IL-18 Production for the Generation of Protective Antibodies Against Candida albicans. Front Microbiol 2020;11:1648. [Crossref] [PubMed]
(English Language Editor: R. Scott)