Connectome-based prediction of brain age in Rolandic epilepsy: a protocol for a multicenter cross-sectional study
Introduction
Rolandic epilepsy (RE), also known as benign epilepsy with centrotemporal spikes, is the most prevalent idiopathic epilepsy syndrome, accounting for approximately 20% of epilepsy diagnoses in children aged under 15 years (1). There is a growing bank of evidence to show that children with RE have an increased rate of cognitive and learning disabilities compared to age-matched healthy children (2-5), especially in regard to reading comprehension (6,7), semantic language processing, discourse levels (8), attention span, executive functions, and memory (9-11).
In individuals with epilepsy, age-related neuroanatomic and cognitive changes differ considerably from those observed in the healthy brain, and this phenomenon has been described in previous studies as accelerated brain aging (12). In their study, Kim et al. analyzed the relationship between resting-state brain network functional connectivity (FC) and cognitive ability in RE patients. They found that the enhancement of FC was closely related to a decline in cognitive ability in both RE patients and control subjects, as measured with the verbal intelligence quotient and full-scale intelligence quotient (13). Furthermore, Li et al. found that decreased variability of dynamic FC density in the orbital frontal cortex, anterior cingulate cortex, and striatum of RE patients was more than likely related to disruption of cognitive control and language function (14). In a prospective study, children with RE also showed higher global clustering and efficiency than normal children, which may indicate that they have a higher development than the normal (15). Brain age, as an index of brain maturity (16,17), can be used to evaluate brain development. Resting-state functional magnetic resonance imaging (rs-fMRI) data can be easily obtained and has the potential to provide clinically-relevant biomarkers (18), and it can also be used to assess individual differences in neural connectivity across multiple whole-brain networks (19). A number of researchers have successfully applied rs-fMRI to predict brain age (16,18,20,21).
In recent years, with the development of machine learning, the method of predicting brain age by machine learning based on brain imaging has aroused great interest among researchers. Using machine learning methods, an age prediction model can be established using brain imaging features and the chronological age of the training data set, after which the model can be used to estimate the age of individuals in the test data set. The estimated age, or brain age, was described by Niu et al. (22). This method has been successfully applied in patients with mild cognitive impairment (23), schizophrenia (24,25), Alzheimer’s disease (26), bipolar disorder (27,28), acquired immunodeficiency syndrome (AIDS) (29), and type 2 diabetes (30). To date, only 3 studies on brain age in epilepsy have been conducted. Pardoe et al. found that the predicted brain age of individuals with medically refractory epilepsy was on average 4.5 years higher than their chronological age (12). Another similar study on brain age in diverse forms of epilepsy reported that all included types of epilepsy had a trend of accelerated aging (31). Furthermore, a recent study of patients with temporal lobe epilepsy observed that both the structural and functional brain ages were increased compared to the chronological age (32). However, it has yet not been established whether children with RE display the same trend.
In this paper, we propose the hypothesis that children with RE have higher brain age than their chronological age, which means that they have an ‘older’ brain. To test this hypothesis, we will firstly use connectome-based predictive modeling (CPM) to predict brain age based on rs-fMRI. CPM, as a machine learning method, is able to predict brain–behavior relationships using whole-brain connectivity measured with neuroimaging data. In our study, we will develop a model based on subjects’ brain FC and age. The brain development of children will be evaluated by calculating the brain-predicted age difference (PAD = predicted age-chronological age).
The aim of the study will be to develop a brain-based model to predict brain age in patients with RE by using CPM, a recently developed data-driven protocol for whole-brain FC–age predictive modeling.
We present the following article in accordance with the MDAR checklist (available at http://dx.doi.org/10.21037/atm-21-574).
Methods
Study design
A multicenter, cross-sectional study of children with RE will be conducted. The study has been registered with the Chinese Clinical Trial Registry (ChiCTR2000032984).
Study setting
The study will be performed at The Affiliated Hospital of Zunyi Medical University, Medical Imaging Center of Guizhou Province (No. 149 Dalian Road, Zunyi, Guizhou, China), The First Affiliated Hospital of Xi’an Jiaotong University (No. 277 Yanta West Road, Xi’an, Shaanxi, China), The First Affiliated Hospital of Henan University of Chinese Medicine (No. 19 Renmin Road, Zhengzhou, Henan, China), Chongqing University Central Hospital (No.1 Jiankang Road, Yuzhong District, Chongqing, China), and the Second Affiliated Hospital of Zunyi Medical University (Xinglong Road, Zunyi, Guizhou, China). The study will be beginning in June 2021 and is expected to be completed in June 2023.
Ethics and dissemination
The study will be conducted in accordance with the amended Declaration of Helsinki (as revised in 2013), and the study protocol has been approved by the ethics board of The Affiliated Hospital of Zunyi Medical University (KLLY-2019-119), Zunyi, Guizhou. All the participants will be informed of the purpose of the study, and written informed consent must be obtained from the parents or guardians of each participating child before the study commences. Participating families will receive a summary of the final results of this study, which will be published in peer-reviewed national and international journals.
Participants
Children with RE aged 6–16 years old and meeting the diagnostic criteria for RE published by the Classification Committee of the International League Against Epilepsy (ILAE) in 2010 (33) will be recruited from the 5 hospitals mentioned above. A healthy control (HC) group matched by sex, age, and years of education will be recruited from children undergoing their annual health checkup at the Outpatient Clinic for General Pediatrics.
Inclusion criteria
The inclusion criteria for patients include: diagnosed as RE by pediatric neurologists and visited the pediatric clinic at 1 of the above-mentioned hospitals. The inclusion criteria for healthy controls: no history of neuropsychiatric disease, craniocerebral trauma or surgery.
Exclusion criteria
People with RE caused by a progressive brain disorder (such as tumor or neurodegenerative disorder) will be excluded. Children in the case group or in the healthy control group meeting any of the following criteria will also be excluded from the study: (I) vital organ failure; (II) contraindications to MRI, such as claustrophobia, or severe MRI artifacts.
Withdrawal criteria
If it is determined that a subject has not complied with the research procedure, or safety concerns arise in relation to the study, the withdrawal of the subject from the study will be considered by the investigator or guardian, as appropriate.
Sample size
The difficulty in predicting brain development in patients with RE is attributable to the lack of firsthand accessible data to estimate the relationship between brain MRI and behavioral measures. Calculations of sample size are based on a similar previous study which used CPM to develop a predictive model. Yip et al. developed a model for the prediction of cocaine abstinence with 53 individuals (34). Another study enrolled 168 healthy college students to develop a model to predict individual differences in propensity to trust (35). Based on previous studies, we intend to recruit 100 healthy children and 100 RE patients (including 50 patients receiving anti-epileptic drug therapy and 50 drug-naïve patients).
Data collection
Patient clinical information including demographic characteristics (sex, chronological age, and years of education) and information about epilepsy (frequency of seizures, duration of epilepsy, age of onset, and the number and time of anti-epileptic drug (AED) treatments) will be recorded. Both the RE patients and the healthy controls will undergo neuropsychological testing using the Wechsler Intelligence Scale for Children, 4th edition (WISC-IV), revised China version. Participants’ who fulfilling the inclusion and exclusion criteria will receive brain MRI scans after the neuropsychological testing on the same day. These data will be collected for the identification of potential predictive factors and development of predictive variables.
Study procedures
The flowchart of the study procedures is shown in Figure 1. After preliminary screening, subjects meeting the inclusion and exclusion criteria described above will undergo neuropsychological tests. Brain MRI scans will also be performed on the same day.
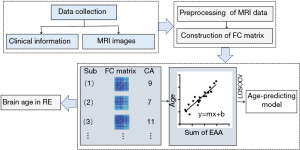
Acquisition of MRI images
All participants will undergo an MRI scan lasting about 20 minutes using GE 3.0T scanners with standard 8-channel head coils. The children will need to wear sponge micro-earplugs to protect their hearing and reduce noise. The children will be instructed to stay still, with their eyes closed, and to think of nothing in particular during the MRI examination, while not falling asleep. To reduce motion artifacts, a special sponge will be used to keep the head in place. Sedatives will not be used for any of the subjects. The MRI sequences will include 3-dimensional brain volume (3D-BRAVO), T2-weighted imaging (T2WI), T2 fluid-attenuated inversion recovery (T2-FLAIR) imaging, and rs-fMRI. Scan parameters of the sequences are shown in Table 1.
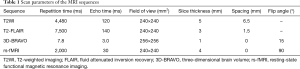
Full table
Harmonization analysis
Technical variations, including differences in MRI scanners, acquisition protocols, and scanning parameters, tend to occur in images collected from multiple centers (36). These unwanted sources of variation are defined as site effects. The ComBat model will be used to remove site effects and to harmonize data from different scanners and sites. This model combines the location/scale model and empirical Bayes framework to adjust the mean value and variance across different groups (37,38).
FC
Preprocessing of all rs-fMRI data including removal of the first 10 volumes, slice timing, realignment, normalization of the individual images into the space of the Montreal Neurological Institute (MNI), smoothing using a Gaussian kernel, detrending, filtering, and regressing out common nuisance variables will be performed with Data Processing Assistant for rs-fMRI Advanced Edition (http://rfmri.org/DPARSF).
Network nodes will be defined using the Shen 268-node brain atlas, which includes the cortex, subcortex, and cerebellum (39). For each participant, a mean time course for each of the 268 nodes will be calculated by averaging the time course of voxels within the node at each time point. Network edges will be taken to represent the FC between each pair of nodes. The Pearson correlation coefficients of time courses between each pair of nodes will be computed. Fisher’s r-to-z transformation will be employed to create a 268×268 symmetric FC matrix for each subject.
CPM
CPM will be executed by using previously validated custom MATLAB scripts (40). The steps for building the model are shown in Figure 1. Sum of the most important edges associated with age among extracted FC matrices and behavioral data (the chronological age) of the healthy participants will be input to generate a predictive model. Edges and the chronological age of each subject will be correlated using Pearson’s correlation or partial correlation to determine the positive and negative predictive networks. Positive networks will be those in which the strength of the edges is significantly positively associated with the chronological age, and negative networks will be those in which the strength of the edges is significantly negatively associated with the chronological age.
The prediction performance of the model will be tested by leave-one-site-out cross-validation, which means healthy participants of N-1 site will be used as the training set to develop the model, and the brain age of the test data set (here is the healthy participants from the remaining site) will be predicted. This step will be repeated until all healthy subjects have a predicted value. The accuracy of the model will be measured by calculating the correlation between the predicted age and the chronological age, the amount of variance in age explained by the model (R2), the mean absolute error (MAE), and the root mean square error (RMSE).
The final model established using the overall healthy control dataset will be applied to the RE patients.
Prediction model in RE patients
After the age-predicting model has been established, connectivity matrices of patients will be input into the model to predict the brain age of the RE patients. After all patients have been given their individual brain age value, the predicted age difference (PAD = predicted age-chronological age) of the brain will be obtained by comparing the predicted age (brain age) with the chronological age.
Previous structural and functional neuroimaging studies have verified that accumulative medication exposure can obviously affect brain activation and connectivity (5,41-43). Moreover, studies of rs-fMRI showed that the local regional homogeneity and white-matter FC were changed in new-onset drug-naive RE patients, but were normal in the medicated group, indicating that antiepileptic drugs could reverse abnormal FC and even achieve a normalized level (43,44). In this trial, we will divide participants into a new-onset drug-naïve group and an antiepileptic medication group, and will predict the brain age of the 2 groups separately.
Confidentiality
The original data of this study will be preserved uniformly by The Affiliated Hospital of Zunyi Medical University, China. Patient information will not be disclosed unless required by law. The publication of anonymous research data will only be used for scientific purposes.
Study status
The study is currently in the preparation phase.
Discussion
Our study will connect clinical information with brain rs-fMRI to develop a predictive model for the prediction of brain age in children with RE in order to evaluate their brain development.
There are some strengths to the study. Firstly, it will use CPM, an advanced machine learning method for establishing models capable of predicting brain–behavior relationships from FC data using the cross-validation method (40). Compared with other predictive models, CPM develops models using the most associated features (edges) selected throughout the whole brain. Differed from IQ and other clinical indicators, which were affected easily by subjective factors, the chronological age of the subjects was correctly calculated according to their date of birth. Furthermore, since the subjects of our study are all children, they will not be asked to perform specific tasks during the acquisition of rs-fMRI images, and will simply be instructed to remain still with their eyes closed, which may ensure the quality of the images. Also, all participants in the study can undergo MRI examination without sedatives, which will eliminate the effect of sedatives on the results.
As the study is being conducted across multiple sites, to ensure the consistency of the imaging data and feasibility of the process, an individualized examination procedure, including the same MRI scan parameters, has been designed for subjects and will be carried out in the 5 participating centers. Moreover, the neuropsychological testing is already widely applied in clinical practice.
Brain activation and connectivity can be affected by cumulative exposure to medication (5,41-43). Antiepileptic treatment may be a confounding factor of brain FC, and thus could affect the study results. To address this problem, we will divide the RE patients into a new-onset drug-naïve group and an antiepileptic medication group and will analyze the results separately.
Previous imaging studies of RE have mainly focused on the changes in brain structure and function, and their relationship with cognition. A longitudinal study based on graph theory suggested that children with RE may have a higher level of development than normal children, along with regional configuration deviating from normality. However, there is no relevant research to prove whether brain development in children with RE have divergence from normal developmental trajectory. In our proposed study, the association of children’s brain FC and their behavioral characteristics will be examined, and the specific changes in brain development will be described.
It must be specified that our proposed research has certain limitations. Our protocol, like most other neuroimaging studies on RE currently, is a cross-sectional study. Our study will only compare the brain development of children with RE with that of healthy children in order to explore whether children with RE have advanced or delayed brain development than normal children. In order to study whether the brains of patients with RE experience accelerated aging, the study could serve as a foundation for conducting a longitudinal follow-up study of the study subjects.
Acknowledgments
Funding: This work was supported by grants from the National Natural Science Foundation of China (No. 81760309) and the Science and Technology Foundation of Health and Family Planning Commission of Guizhou Province (No. gzwjkj2017-1-013).
Footnote
Reporting Checklist: The authors have completed the MDAR checklist. Available at http://dx.doi.org/10.21037/atm-21-574
Conflicts of Interest: All authors have completed the ICMJE uniform disclosure form (available at http://dx.doi.org/10.21037/atm-21-574). The authors have no conflicts of interest to declare.
Ethical Statement: The authors are accountable for all aspects of the work in ensuring that questions related to the accuracy or integrity of any part of the work are appropriately investigated and resolved. The study will be conducted in accordance with the amended Declaration of Helsinki (as revised in 2013), and the study protocol has been approved by the ethics board of The Affiliated Hospital of Zunyi Medical University (KLLY-2019-119), Zunyi, Guizhou. All the participants will be informed of the purpose of the study, and written informed consent must be obtained from the parents or guardians of each participating child before the study commences.
Open Access Statement: This is an Open Access article distributed in accordance with the Creative Commons Attribution-NonCommercial-NoDerivs 4.0 International License (CC BY-NC-ND 4.0), which permits the non-commercial replication and distribution of the article with the strict proviso that no changes or edits are made and the original work is properly cited (including links to both the formal publication through the relevant DOI and the license). See: https://creativecommons.org/licenses/by-nc-nd/4.0/.
References
- Panayiotopoulos CP, Michael M, Sanders S, et al. Benign childhood focal epilepsies: assessment of established and newly recognized syndromes. Brain 2008;131:2264-86. [Crossref] [PubMed]
- Wickens S, Bowden SC, D'Souza W. Cognitive functioning in children with self-limited epilepsy with centrotemporal spikes: A systematic review and meta-analysis. Epilepsia 2017;58:1673-85. [Crossref] [PubMed]
- Besag F, Gobbi G, Aldenkamp A, et al. Psychiatric and Behavioural Disorders in Children with Epilepsy (ILAE Task Force Report): Behavioural and psychiatric disorders associated with childhood epilepsy syndromes. Epileptic Disord 2016; Epub ahead of print. [Crossref] [PubMed]
- Vannest J, Szaflarski JP, Eaton KP, et al. Functional magnetic resonance imaging reveals changes in language localization in children with benign childhood epilepsy with centrotemporal spikes. J Child Neurol 2013;28:435-45. [Crossref] [PubMed]
- Datta AN, Oser N, Bauder F, et al. Cognitive impairment and cortical reorganization in children with benign epilepsy with centrotemporal spikes. Epilepsia 2013;54:487-94. [Crossref] [PubMed]
- Currie NK, Lew AR, Palmer TM, et al. Reading comprehension difficulties in children with rolandic epilepsy. Dev Med Child Neurol 2018;60:275-82. [Crossref] [PubMed]
- Croona C, Kihlgren M, Lundberg S, et al. Neuropsychological findings in children with benign childhood epilepsy with centrotemporal spikes. Dev Med Child Neurol 1999;41:813-8. [Crossref] [PubMed]
- Overvliet GM, Besseling RM, van der Kruijs SJ, et al. Clinical evaluation of language fundamentals in Rolandic epilepsy, an assessment with CELF-4. Eur J Paediatr Neurol 2013;17:390-6. [Crossref] [PubMed]
- Cerminara C, D'Agati E, Lange KW, et al. Benign childhood epilepsy with centrotemporal spikes and the multicomponent model of attention: a matched control study. Epilepsy Behav 2010;19:69-77. [Crossref] [PubMed]
- Bennett-Back O, Keren A, Zelnik N. Attention-deficit hyperactivity disorder in children with benign epilepsy and their siblings. Pediatr Neurol 2011;44:187-92. [Crossref] [PubMed]
- Northcott E, Connolly AM, Berroya A, et al. Memory and phonological awareness in children with Benign Rolandic Epilepsy compared to a matched control group. Epilepsy Res 2007;75:57-62. [Crossref] [PubMed]
- Pardoe HR, Cole JH, Blackmon K, et al. Structural brain changes in medically refractory focal epilepsy resemble premature brain aging. Epilepsy Res 2017;133:28-32. [Crossref] [PubMed]
- Kim HJ, Lee JH, Park CH, et al. Role of Language-Related Functional Connectivity in Patients with Benign Childhood Epilepsy with Centrotemporal Spikes. J Clin Neurol 2018;14:48-57. [Crossref] [PubMed]
- Li R, Wang L, Chen H, et al. Abnormal dynamics of functional connectivity density in children with benign epilepsy with centrotemporal spikes. Brain Imaging Behav 2019;13:985-994. [Crossref] [PubMed]
- Garcia-Ramos C, Dabbs K, Lin JJ, et al. Network analysis of prospective brain development in youth with benign epilepsy with centrotemporal spikes and its relationship to cognition. Epilepsia 2019;60:1838-1848. [Crossref] [PubMed]
- Dosenbach NU, Nardos B, Cohen AL, et al. Prediction of individual brain maturity using fMRI. Science 2010;329:1358-61. [Crossref] [PubMed]
- Franke K, Luders E, May A, et al. Brain maturation: predicting individual BrainAGE in children and adolescents using structural MRI. Neuroimage 2012;63:1305-12. [Crossref] [PubMed]
- Liem F, Varoquaux G, Kynast J, et al. Predicting brain-age from multimodal imaging data captures cognitive impairment. Neuroimage 2017;148:179-88. [Crossref] [PubMed]
- Thomason ME, Dennis EL, Joshi AA, et al. Resting-state fMRI can reliably map neural networks in children. Neuroimage 2011;55:165-75. [Crossref] [PubMed]
- Vergun S, Deshpande AS, Meier TB, et al. Characterizing Functional Connectivity Differences in Aging Adults using Machine Learning on Resting State fMRI Data. Front Comput Neurosci 2013;7:38. [Crossref] [PubMed]
- Smyser CD, Dosenbach NU, Smyser TA, et al. Prediction of brain maturity in infants using machine-learning algorithms. Neuroimage 2016;136:1-9. [Crossref] [PubMed]
- Niu X, Zhang F, Kounios J, et al. Improved prediction of brain age using multimodal neuroimaging data. Hum Brain Mapp 2020;41:1626-1643. [Crossref] [PubMed]
- Gaser C, Franke K, Klöppel S, et al. BrainAGE in Mild Cognitive Impaired Patients: Predicting the Conversion to Alzheimer's Disease. PLoS One 2013;8:e67346 [Crossref] [PubMed]
- Koutsouleris N, Davatzikos C, Borgwardt S, et al. Accelerated brain aging in schizophrenia and beyond: a neuroanatomical marker of psychiatric disorders. Schizophr Bull 2014;40:1140-53. [Crossref] [PubMed]
- Schnack HG, van Haren NE, Nieuwenhuis M, et al. Accelerated Brain Aging in Schizophrenia: A Longitudinal Pattern Recognition Study. Am J Psychiatry 2016;173:607-16. [Crossref] [PubMed]
- Beheshti I, Maikusa N, Matsuda H. The association between "Brain-Age Score" (BAS) and traditional neuropsychological screening tools in Alzheimer's disease. Brain Behav 2018;8:e01020 [Crossref] [PubMed]
- Van Gestel H, Franke K, Petite J, et al. Brain age in bipolar disorders: Effects of lithium treatment. Aust N Z J Psychiatry 2019;53:1179-88. [Crossref] [PubMed]
- Hajek T, Franke K, Kolenic M, et al. Brain Age in Early Stages of Bipolar Disorders or Schizophrenia. Schizophr Bull 2019;45:190-8. [Crossref] [PubMed]
- Cole JH, Underwood J, Caan MW, et al. Increased brain-predicted aging in treated HIV disease. Neurology 2017;88:1349-1357. [Crossref] [PubMed]
- Franke K, Gaser C, Manor B, et al. Advanced BrainAGE in older adults with type 2 diabetes mellitus. Front Aging Neurosci 2013;5:90. [Crossref] [PubMed]
- Sone D, Beheshti I, Maikusa N, et al. Neuroimaging-based brain-age prediction in diverse forms of epilepsy: a signature of psychosis and beyond. Mol Psychiatry 2021;26:825-34. [Crossref] [PubMed]
- Hwang G, Hermann B, Nair VA, et al. Brain aging in temporal lobe epilepsy: Chronological, structural, and functional. NeuroImage. Clinical 2020;25:102183 [Crossref] [PubMed]
- Berg AT, Berkovic SF, Brodie MJ, et al. Revised terminology and concepts for organization of seizures and epilepsies: report of the ILAE Commission on Classification and Terminology, 2005-2009. Epilepsia 2010;51:676-85. [Crossref] [PubMed]
- Yip SW, Scheinost D, Potenza MN, et al. Connectome-Based Prediction of Cocaine Abstinence. Am J Psychiatry 2019;176:156-64. [Crossref] [PubMed]
- Lu X, Li T, Xia Z, et al. Connectome-based model predicts individual differences in propensity to trust. Hum Brain Mapp 2019;40:1942-54. [Crossref] [PubMed]
- Zhu T, Liu X, Gaugh MD, et al. Evaluation of measurement uncertainties in human diffusion tensor imaging (DTI)-derived parameters and optimization of clinical DTI protocols with a wild bootstrap analysis. J Magn Reson Imaging 2009;29:422-35. [Crossref] [PubMed]
- Fortin JP, Parker D, Tunç B, et al. Harmonization of multi-site diffusion tensor imaging data. NeuroImage 2017;161:149-70. [Crossref] [PubMed]
- Johnson WE, Li C, Rabinovic A. Adjusting batch effects in microarray expression data using empirical Bayes methods. Biostatistics 2007;8:118-27. [Crossref] [PubMed]
- Shen X, Tokoglu F, Papademetris X, et al. Groupwise whole-brain parcellation from resting-state fMRI data for network node identification. NeuroImage 2013;82:403-15. [Crossref] [PubMed]
- Shen X, Finn ES, Scheinost D, et al. Using connectome-based predictive modeling to predict individual behavior from brain connectivity. Nat Protoc 2017;12:506-18. [Crossref] [PubMed]
- Yang T, Guo Z, Luo C, et al. White matter impairment in the basal ganglia-thalamocortical circuit of drug-naïve childhood absence epilepsy. Epilepsy Res 2012;99:267-73. [Crossref] [PubMed]
- Yang T, Luo C, Li Q, et al. Altered resting-state connectivity during interictal generalized spike-wave discharges in drug-naïve childhood absence epilepsy. Hum Brain Mapp 2013;34:1761-7. [Crossref] [PubMed]
- Zeng H, Ramos CG, Nair VA, et al. Regional homogeneity (ReHo) changes in new onset versus chronic benign epilepsy of childhood with centrotemporal spikes (BECTS): A resting state fMRI study. Epilepsy Res 2015;116:79-85. [Crossref] [PubMed]
- Jiang Y, Song L, Li X, et al. Dysfunctional white-matter networks in medicated and unmedicated benign epilepsy with centrotemporal spikes. Human brain mapping 2019;40:3113-24. [Crossref] [PubMed]