Estimating the amplitude spectrum area of ventricular fibrillation during cardiopulmonary resuscitation using only ECG waveform
Introduction
Ventricular fibrillation (VF) is the commonly observed initial rhythm in cardiac arrest patients, and defibrillation is the only effective way to terminate this malignant arrhythmia (1,2). Since the probability of successful defibrillation diminishes as time elapses, earlier defibrillation with concurrent high-quality cardiopulmonary resuscitation (CPR) is critical to survival (3). However, treatment of VF consists of a series of time-sensitive therapies, and not all patients in VF benefit from being treated in the same manner (4,5). Real-time electrocardiogram (ECG) waveform analysis has been advocated for several decades as a potential decision-making tool to optimize CPR (6). The VF signal changes over time, and therefore quantitative measures can help estimate the duration of VF, predict the likelihood of successful defibrillation, and evaluate the effectiveness of CPR (7). Both animal and clinical studies have demonstrated that quantitative VF signal analysis can be used as a non-invasive tool to optimize the timing of defibrillation, and allow CPR to be tailored to each individual heart (8,9).
Amplitude spectrum area (AMSA), as one of the most efficient predictors for successful defibrillation (9-11), has been shown to be correlated with coronary perfusion pressure, and can reflect the energy state of the myocardium (12,13). Typically, the highest value of AMSA is frequently observed at the onset of VF, and declines as time elapses without treatment. When effective CPR is provided in time, a higher AMSA value is usually achieved due to the myocardium regaining perfusion status (7,14). Therefore, real-time monitoring of AMSA may serve as a quality-control for CPR, revealing whether or not the myocardial blood flow has improved and whether or not the heart is ready for defibrillation (14,15). Clinical studies have confirmed that both pre-shock AMSA and relative changes of AMSA during CPR are associated with shock success and may provide an effective real-time strategy to guide individual treatment (16-18).
However, reliable AMSA can only be obtained during the interval of CC pause because CC-produced artifacts may lead to overestimation of AMSA and reduction of predictive performance (11,19). Suppressing the artifact using additional reference recordings, such as transthoracic impedance or acceleration signals, may be a possible solution (20,21). Additional equipment is required to obtain these references, and they are not available in all existing automated external defibrillators (AEDs). A universal algorithm which is capable of estimating AMSA accurately during the resuscitation effort using only the ECG waveform recorded from AEDs, is therefore much anticipated. The present study aimed to develop a method that can reliably estimate AMSA during CPR and preserve its predictive performance of defibrillation outcome using the ECG waveform recorded from cardiac arrest patients. We present the following article in accordance with the MDAR reporting checklist (available at http://dx.doi.org/10.21037/atm-20-7166).
Methods
Data collection and annotation
This study was approved by the Ethical Committee of the Army Medical University (No. 2020-002-02). Written informed consent was waived due to the study’s retrospective nature. The study conformed to the provisions of the Declaration of Helsinki (as revised in 2013). Data were retrospectively collected in emergency departments of the Army Medical University affiliated hospitals. Cases with VF as the presenting rhythm received at least 60 seconds of CC before shock delivery and ECG recorded in conjunction with the CPR references by defibrillators were selected for the study. Since data collection was performed after the resuscitation events had been completed, the electronic data did not contain any identifiable participant information, such as age, sex, or survival outcomes. In 163 cases, ECG waveforms were recorded at a sample rate of 125 Hz, and the transthoracic impedance signals were recorded from the same defibrillation pads at a sample rate of 61 Hz (LifePak 12, Physio-Control, Inc., Redmond, WA, USA). In another 349 cases, ECG and compression depth waveforms were recorded through two standard adhesive adult defibrillation/pacing pads that integrating an accelerometer-based CPR feedback at a sample rate of 250 Hz (R-Series, Zoll Medical Corporation, Chelmsford, MA, USA). The datasets are not publicly available because they are part of the participant’s medical information.
For each case, an 8-second episode of ECG and CPR reference waveforms preceding each defibrillation shock was extracted from the recordings. As shown in Figure 1A, each episode contained 2 segments: a 4-second-artifact-corrupted waveform during CC followed by an adjacent 4-second-artifact-free waveform without CC. A total of 1,008 episodes that consisted of 461 first shock and 547 subsequent shocks were obtained from 512 cases. For each episode, both pre-shock and post-shock ECG rhythms were annotated by 2 experienced emergency physicians who were blinded to the quantitative waveform analysis. A defibrillation was regarded as successful when VF was converted to an organized rhythm within 60 seconds after shock delivery with a heart rate greater than 40 beats/minute and sustained for a period greater than 30 seconds (9,15,16). The data were distributed into a training set (480 episodes from 259 cases) and a testing set (528 episodes from 253 cases) for the derivation and validation of the algorithm. Signals were resampled to 250 Hz for compatibility and analyzed using MATLAB (The MathWorks, Inc., Natick, MA, USA) software.
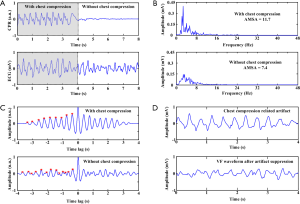
Proposed method
In order to obtain reliable analysis, the CC-produced artifacts needed to be suppressed from the VF signal prior to AMSA calculation as the spectral components of the artifact overlapped the dominant frequencies of the VF (Figure 1B). On the contrary, AMSA needed to be calculated from the original VF signal when CC was paused because filtering the clean ECG would lead to underestimation of AMSA. Based on the fact that the CC-related artifact presented an almost periodic waveform while the VF signal exhibited a nonchaotic stochastic signal, we proposed a method incorporating VF signal quality index (SQI) and least mean square (LMS) filter to estimate AMSA (Figure 1C,D). In this way, AMSA could be adaptively calculated either during ongoing CC or during CC pause from the VF signals. The flowchart of the proposed method is shown in Figure 2, and the major process of the algorithm consisted of 4 steps. The generated code will not be available for the time being due to ongoing patent and software copyright application process.
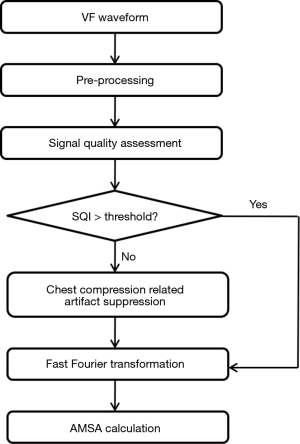
The first step was signal preprocessing. The VF signal underwent preprocessing using a high-pass filter with a cutoff frequency at 1 Hz to minimize baseline drift. A low-pass filter with a cutoff frequency at 48 Hz was then used to remove high frequency noise.
The second step was VF signal quality assessment. In order to determine whether the VF signal was corrupted by CC, we proposed a novel SQI that could measure the quality of VF, providing an irregularity evaluation for each segment of the signal. The autocorrelation of the VF signal was calculated to extract the periodic components, and the peaks were detected from the first half of the normalized autocorrelation function due to its symmetrical characteristic (Figure 1C) (22). The distribution of the peaks was incorporated using 2 weighted measures: i.e., the slope of fitting curve among successive peaks and mean interval of successive peaks in each segment were combined using a sigmoid function to assign a score between 0 to 1 to indicate the SQI of VF.
The third step consisted of CC-related artifact construction and suppression. If the SQI was lower than the predefined threshold, the VF signal was more likely to be corrupted by CC. The CC-related artifact was constructed by modeling the fundamental frequency of CC and its sinusoidal harmonics (23). The estimated VF signal was derived by subtraction of the CC-related artifact from the corrupted ECG waveform, using a LMS filter reported by Irusta (24) and de Gauna (25) (Figure 1D). In contrast with these 2 studies, we estimated the fundamental frequency of CC by the average time interval of the peaks in time domain, rather than from the spectral analysis of ECG or CPR reference. Additionally, the candidate spikes that might have been produced by CC were detected and suppressed, as described in our previous study (26).
The fourth step was AMSA calculation. The original/filtered VF signal was converted from the time to the frequency domain by fast Fourier transformation, using a Tukey window. The AMSA was calculated as the sum of the products of individual frequencies and their amplitudes, between 2 Hz and 48 Hz (16).
Performance evaluation
Data from the training set were employed to tune the parameters of the algorithm, including the coefficients for SQI calculation, threshold of SQI for quality assessment, number of harmonics used to model CC-related artifacts, and threshold of AMSA for the prediction of a successful shock. Data from the testing set were employed to evaluate the algorithm, including the ability to detect CC-corrupted VF and the estimated AMSA during CC and CC pause, and the ability to predict the defibrillation outcome.
For each 4-second segment, the AMSA calculated from original VF signal using the traditional method (AMSA_t) was compared with calculated using the proposed method (AMSA_p). The AMSA_t calculated from the 4-second segment without CC also served as the true AMSA value of the preceding 4-second segment of ongoing CC.
The performance of SQI for detection of the CC-corrupted VF was evaluated using sensitivity and specificity. The correlations between AMSA_t and AMSA_p were evaluated by correlation analysis. The predictive performance of AMSA was evaluated by sensitivity, specificity, accuracy, and area under the receiver operating characteristic curve (AUC).
Statistical analysis
The Kolmogorov-Smirnov test was used to verify the normality of quantitative variables. The continuous data are reported as the means ± standard deviations for variables with a normal distribution or as medians (interquartile ranges) for variables with a skewed distribution. The normally distributed continuous variables between groups were compared using Student’s t-test, while those with a skewed distribution were analyzed using Wilcoxon test. The categorical variables are reported as percentages and were compared using chi-square test. The correlations between continuous variables were investigated by Pearson’ correlation analysis. The AUCs were compared using the Hanley and McNeil method. Two-sided P values ≤0.05 were considered statistically significant, and all analyses were performed using SPSS 22 (IBM Corp., Armonk, NY, USA).
Results
A total of 1,008 countershocks were included for analysis, and the median CC rate prior to shock delivery was 2.25 (2.0–2.75) compressions per minute. For each episode, the fundamental frequency of the VF signal calculated from the 4-second CC-corrupted segment was significantly lower than that calculated from the adjacent 4-second-artifact-free segment [2.5 (2.0–4.75) Hz vs. 3.75 (2.75–6.75) Hz, P<0.01]. Among the 1,008 ECG episodes, 311 (30.9%) were extracted from successful defibrillations while the remaining 697 (69.1%) were extracted from unsuccessful ones according to the definition of defibrillation. The AMSA values calculated from segments without CC using AMSA_t was significantly higher in successful shocks than in unsuccessful shocks, both in the training [9.3 (6.3–14.0) vs. 5.7 (4.1–8.6) mVHz, P<0.01] and in the testing [9.9 (7.3–13.6) vs. 6.0 (4.3–9.4) mVHz, P<0.01] sets.
A SQI threshold of 0.55 was established to identify the CC-corrupted VF signal, and an AMSA threshold of 8.0 mVHz was used to predict a potentially successful defibrillation shock with optimal balance between sensitivity and specificity using the training data, as reported in previous clinical studies (9,16). Additionally, the lowest LMS error was achieved when the first 5 harmonics was used to model the CC-related artifact.
The testing results are summarized in Figure 3. The SQI was significantly lower when the VF signal was corrupted by CC (Figure 3A) [0.15 (0.04–0.61) vs. 0.75 (0.61–0.83), P<0.01]. The SQI identified the CC-corrupted low-quality VF segments with a sensitivity of 81.4% and a specificity of 82.2%. In segments with CC (Figure 3B), AMSA_t was significantly higher than AMSA_p [11.2 (7.7–16.2) vs. 7.1 (5.0–15.2) mVHz, P<0.01] and true AMSA [11.2 (7.7–16.2) vs. 7.2 (4.9–10.6) mVHz, P<0.01], but there was no statistical difference between AMSA_p and true AMSA [7.1 (5.0–15.2) vs. 7.2 (4.9–10.6) mVHz, P=0.24]. The correlation coefficient was 0.53 between AMSA_t and true AMSA (Figure 3D), was 0.77 between AMSA_p and true AMSA (Figure 3E). In segments without CC (Figure 3C), AMSA_p was significantly lower than the true AMSA [6.8 (4.2–10.3) vs. 7.2 (4.9–10.6) mVHz, P<0.01]. The correlation coefficient between AMSA_p and the true AMSA was 0.98 (Figure 3F).
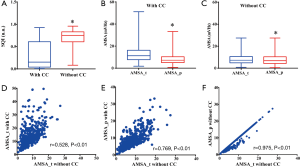
The predictive performances of AMSA calculated from the VF waveform with and without CC are listed in Table 1. Compared with true AMSA, the sensitivity was significantly increased but the specificity, accuracy and AUC were markedly decreased when AMSA_t with CC were used to predict the defibrillation outcome. On the contrary, no statistical differences in sensitivity, specificity, accuracy, and AUC were observed when either AMSA_p with CC or AMSA_p without CC was compared with the results of true AMSA.
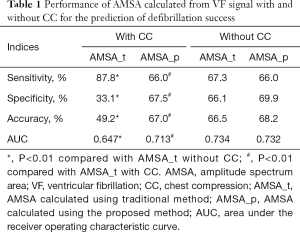
Full table
Figure 4 shows 4 examples of continuous AMSA monitoring with a time interval of 1 second during CPR. The AMSA values calculated using AMSA_t were overestimated during CC and returned to normal levels during CC pause in all cases. The overestimated AMSA value would keep prompting a defibrillation shock since it was always greater than the predefined defibrillation threshold during CC. However, AMSA values estimated using AMSA_p stayed in a relatively stable state both during CC and during CC pause, apart from a few improper and unsuccessful suppressions. The resuscitation effort, therefore can be monitored by the absolute AMSA value and its relative change during CPR. For instance, AMSA value was constantly increased and exceeded the defibrillation threshold after 2 minutes of CC, which resulted in a successful defibrillation in Figure 4A. On the contrary, the defibrillation shock could be delivered before CC in Figure 4B because of the high initial AMSA value. In Figure 4C, AMSA value was constantly increased during CC but steadily dropped during the prolonged CC pause, which led to an unsuccessful shock. In Figure 4D, although CC was consistently implemented, the steadily decreasing AMSA value indicated that either the quality of CC needed to be improved or other interventions were necessary to successfully resuscitate the victim.
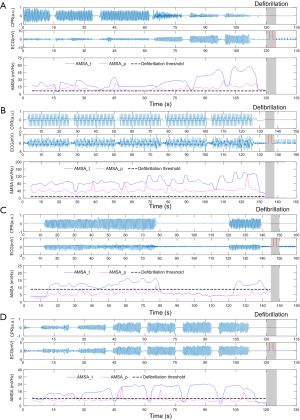
Discussion
This study introduced an algorithm to estimate AMSA during CPR by assessing the VF signal quality based solely on the surface ECG waveform recorded from AEDs. We demonstrated that CC-corrupted low quality VF signals can be correctly identified and that AMSA can be reliably estimated from the VF signals both during ongoing CC and during CC pause. Furthermore, the method was validated by preserving the predictive performance of defibrillation success in patients with presenting shockable rhythms.
Early and uninterrupted CPR, together with early defibrillation, is critical for a successful outcome after cardiac arrest. A recent study further confirmed that the analysis of real-time variations and trends of ECG features could help in selecting the timing of defibrillation to avoid unnecessary shocks and improve outcomes in extended cases of refractory VF (27). However, the CC-produced artifact modifies the underlying ECG and precludes reliable waveform analysis, either for shockable rhythm identification or for determining the probability of defibrillation success evaluation (20). Earlier studies indicated that the CC-produced artifact is an additive noise, predominantly arising from the electrode skin interface (28). The corrupted ECG waveform might also include signals generated by direct impact of the compressions on the chest wall and signals generated by the contraction of thoracic muscles. The characteristics of CC-induced artifact has been deeply investigated by previous studies through analysis ECG waveforms recorded on patients with the underlying cardiac rhythm of asystole (24,26,29). In the time domain, the artifact presents an almost periodic waveform, with slow changes in waveform from cycle to cycle. In the frequency domain, the energy content is concentrated around the harmonics, with a fundamental frequency being that of the CC. Through the analysis of the power spectral density of the CC artifact recorded from cardiac arrest patients with other cardiac rhythms, the CC-related artifact was revealed to have an important spectral overlap with human ECG, either for non-shockable or shockable rhythms (29). Recent studies investigating quantitative VF signal measures during CC have confirmed a remarkable reduction in defibrillation predictive performance compared with that during CC pause (11,30). The CC-induced artifact challenges the routine use of real-time quantitative VF signal measures to guide resuscitation during uninterrupted CPR.
In order to perform reliable and accurate VF signal analyses without interrupting CPR, numerous strategies have been developed to suppress the CC-related artifacts. One study used the fact that the fundamental frequency of CC is approximately 2 Hz to successfully remove the CPR artifact from animal ECGs using high-pass digital filters with cut-off frequencies between 4–4.5 Hz (31). In the human VF signal, however, the fundamental frequency range is 3–8 Hz, and the harmonics of the CC artifacts lie within the spectral range, making the separation of VF and artifacts by such filters infeasible (32,33). Subsequently, adaptive filtering techniques have been adopted to clean the CC-induced artifact using references that are synchronously recorded with ECG, such as the compression depth, compression force, transthoracic impedance, and arterial blood pressure waveform (28,34). These methods have significantly improved the signal-to-noise ratio (SNR) of the artifact-corrupted ECG, as well as the accuracy of rhythm classification. However, the acquisition of the reference signals requires AED hardware alterations, and most current AEDs only record and use the surface ECG waveforms for rhythm interpretation. Efforts therefore have been made to adaptively filter the CC artifact based on the ECG alone. For instance, Ruiz de Gauna et al. (25) designed an artifact suppression method based on the Kalman filter, in which the artifact was modeled using the fundamental frequency of the compressions and the relative power content of the artifact from the spectral analysis of the corrupted ECG. Amann et al. (35) adopted the coherent line removal algorithm to reduce the CC-related artifact in VF, using a windowed Fourier transform to capture characteristic features of VF signal and CC artifact. However, the adaptive filters based only on the ECG waveform had relatively poorer performance compared with those using additional references, when evaluated by accuracy of shockable rhythm classification and improvement in SNR. Additionally, little is known about how these filters preserving the VF quantitative features and their prognostic performances. In order to estimate AMSA from the CC-corrupted VF, Lo et al. (36) developed a method combining empirical mode decomposition and LMS filtering. Using an additive data model, the authors demonstrated that the method could preserve the cut-off value for the CC artifact with a power ratio to VF ranging from 0 to 6 dB. However, the authors did not assess the predictive ability of AMSA calculated with their proposed method. Additionally, the method was designed to filter the CC-related artifact from the corrupted ECG, but the CPR artifact suppression method based only on the recorded ECG has no way of determining whether or not the CC is being given.
In the current study, we proposed a method consisting of an LMS technique which has been previously used to filter CPR artifacts for the estimation of AMSA during CPR (24,25,37). In contrast to previous studies, the algorithm assessed the signal quality of VF in order to determine whether it was corrupted by CC. As reported in a previous study, autocorrelation could detect and extract minuscule periodic components present in the ECG waveform, which may show an apparently irregular type of activity (7). For a periodic signal, the peaks of its autocorrelation function lie exactly on a straight line. In contrast, the autocorrelation function of an aperiodic signal has peaks that are not linearly distributed. Based on the knowledge that clean VF exhibits a stochastic signal but presents an almost periodic signal when corrupted by the CC artifact, we inferred that the distribution of peaks of the autocorrelation of the VF signal can be used to detect whether or not it has been contaminated by a CC artifact. Our results confirmed that the peaks of the autocorrelation of a clean VF showed an irregular interval and scattered amplitude distribution. However, the autocorrelation of a corrupted VF showed characteristic peaks that occurred at regular intervals and had successively escalating amplitudes. To generate a more quantitative distinction between the VF with and without CC, a sigmoid function that combined linear regression fit and peak interval was adapted to SQI. During CC pause, SQI is high because the aperiodic and irregular nature of VF is retained. Therefore, AMSA can be calculated from the original VF signal. Conversely, SQI is low during ongoing CC because VF is governed by the periodic and regular artifact. Thus, artifacts need to be constructed and suppressed from VF before AMSA calculation. Our results indicated that the presence or absence of CC could be reliably detected and AMSA could be accurately estimated using the proposed method. The AMSA calculated directly from the CC-corrupted VF signal increased sensitivity but decreased specificity. As a result, both the accuracy and AUC were significantly reduced compared with those calculated from the clean VF. The defibrillation outcome-predicting ability of AMSA was well preserved both during ongoing CC and during CC pause, as shown in the comparable sensitivity/specificity and accuracy/AUC using the proposed method. This method, therefore, is eligible for incorporation into AEDs and has the potential to implement a real-time AMSA-driven protocol of defibrillation and CPR.
There were several limitations to the current study. First, although SQI was significantly lower for CC-corrupted VF, the sensitivity/specificity for identifying a CC-corrupted VF using SQI still needs to be improved. Additionally, the artifact produced by mechanical CC may differ from that of manual CC in morphology and SNR. Therefore, the efficacy of the algorithm during mechanical versus manual CC still requires evaluation. Second, although the proposed method has the potential to provide more detailed information about the dynamic changes of AMSA during CPR, this was a retrospective study, and improvements are still needed before real-time application, as there was abrupt change of the AMSA curve induced by improper detection and unsuccessful suppression of the artifact. Third, the algorithm cannot be utilized without the help of a reliable VF detection algorithm. Although several algorithms have demonstrated the capability to correctly distinguish VF from other rhythms during CC using only the ECG waveform, the performance of combining the current algorithm with the VF detection algorithm also needs to be validated in future studies.
Conclusions
This study introduced an algorithm to estimate AMSA through the signal quality assessment-based artifact suppression method using solely the ECG waveform. The CC-corrupted low-quality signal can be reliably detected and AMSA can be accurately estimated both during ongoing CC and during CC pause from VF. The preservation of defibrillation outcome-predicting performance indicated that this algorithm is eligible for incorporation into AEDs for the purpose of continuously and precisely monitoring AMSA during uninterrupted CPR.
Acknowledgments
Funding: This work was supported by the Natural Science Foundation Project of Chongqing (No. cstc2017jcyjBX0053) and the National Natural Science Foundation of China (No. NSFC31771070 and No. NSFC31900977).
Footnote
Reporting Checklist: The authors have completed the MDAR reporting checklist. Available at http://dx.doi.org/10.21037/atm-20-7166
Data Sharing Statement: Available at http://dx.doi.org/10.21037/atm-20-7166
Conflicts of Interest: All authors have completed the ICMJE uniform disclosure form (available at http://dx.doi.org/10.21037/atm-20-7166). Dr. YL has a patent “A method and instrument for real-time assessment of ventricular fibrillation signal quality and defibrillation success rate” in pending. The other authors have no conflicts of interest to declare.
Ethical Statement: The authors are accountable for all aspects of the work in ensuring that questions related to the accuracy or integrity of any part of the work are appropriately investigated and resolved. This study was approved by the Ethical Committee of the Army Medical University (No. 2020-002-02). Written informed consent was waived due to the study’s retrospective nature. The study conformed to the provisions of the Declaration of Helsinki (as revised in 2013).
Open Access Statement: This is an Open Access article distributed in accordance with the Creative Commons Attribution-NonCommercial-NoDerivs 4.0 International License (CC BY-NC-ND 4.0), which permits the non-commercial replication and distribution of the article with the strict proviso that no changes or edits are made and the original work is properly cited (including links to both the formal publication through the relevant DOI and the license). See: https://creativecommons.org/licenses/by-nc-nd/4.0/.
References
- Go AS, Mozaffarian D, Roger VL, et al. Executive summary: Heart Disease and Stroke Statistics - 2014 Update: A report from the American Heart Association. Circulation 2014;129:399-410. [Crossref] [PubMed]
- Berdowski J, Berg RA, Tijssen JGP, et al. Global incidences of out-of-hospital cardiac arrest and survival rates: Systematic review of 67 prospective studies. Resuscitation 2010;81:1479-87. [Crossref] [PubMed]
- Panchal AR, Bartos JA, Cabañas JG, et al. Part 3: Adult Basic and Advanced Life Support: 2020 American Heart Association Guidelines for Cardiopulmonary Resuscitation and Emergency Cardiovascular Care. Circulation 2020;142:S366-468. [Crossref] [PubMed]
- Weisfeldt ML, Becker LB. Resuscitation after cardiac arrest: A 3-phase time-sensitive model. Journal of the American Medical Association 2002;288:3035-8. [Crossref] [PubMed]
- Cheskes S, Schmicker RH, Christenson J, et al. Perishock pause: an independentpredictor of survival from out-of-hospital shockable cardiac arrest. Circulation 2011;124:58-66. [Crossref] [PubMed]
- Li Y, Tang W. Ventricular Fibrillation Waveform Analysis during Cardiopulmonary Resuscitation. Signa Vitae 2010;5:63-5. [Crossref]
- Li Y, Tang W. Optimizing the timing of defibrillation: the role of ventricular fibrillation waveform analysis during cardiopulmonary resuscitation. Crit Care Clin 2012;28:199-210. [Crossref] [PubMed]
- Aiello S, Perez M, Cogan C, et al. Real-time ventricular fibrillation amplitude-spectral area analysis to guide timing of shock delivery improves defibrillation efficacy during cardiopulmonary resuscitation in swine. J Am Heart Assoc 2017;6:e006749 [Crossref] [PubMed]
- Ristagno G, Mauri T, Cesana G, et al. Amplitude spectrum area to guide defibrillation a validation on 1617 patients with ventricular fibrillation. Circulation 2015;131:478-87. [Crossref] [PubMed]
- He M, Gong Y, Li Y, et al. Combining multiple ECG features does not improve prediction of defibrillation outcome compared to single features in a large population of out-of-hospital cardiac arrests. Crit Care 2015;19:425. [Crossref] [PubMed]
- Coult J, Blackwood J, Sherman L, et al. Ventricular fibrillation waveform analysis during chest compressions to predict survival from cardiac arrest. Circ Arrhythm Electrophysiol 2019;12:e006924 [Crossref] [PubMed]
- Reynolds JC, Salcido DD, Menegazzi JJ. Correlation between coronary perfusion pressure and quantitative ECG waveform measures during resuscitation of prolonged ventricular fibrillation. Resuscitation 2012;83:1497-502. [Crossref] [PubMed]
- Salcido DD, Menegazzi JJ, Suffoletto BP, et al. Association of intramyocardial high energy phosphate concentrations with quantitative measures of the ventricular fibrillation electrocardiogram waveform. Resuscitation 2009;80:946-50. [Crossref] [PubMed]
- Li Y, Ristagno G, Bisera J, Tang W, et al. Electrocardiogram waveforms for monitoring effectiveness of chest compression during cardiopulmonary resuscitation. Crit Care Med 2008;36:211-5. [Crossref] [PubMed]
- Jin D, Dai C, Gong Y, et al. Does the choice of definition for defibrillation and CPR success impact the predictability of ventricular fibrillation waveform analysis? Resuscitation 2017;111:48-54. [Crossref] [PubMed]
- Ristagno G, Li Y, Fumagalli F, et al. Amplitude spectrum area to guide resuscitation-A retrospective analysis during out-of-hospital cardiopulmonary resuscitation in 609 patients with ventricular fibrillation cardiac arrest. Resuscitation 2013;84:1697-703. [Crossref] [PubMed]
- Schoene P, Coult J, Murphy L, et al. Course of quantitative ventricular fibrillation waveform measure and outcome following out-of-hospital cardiac arrest. Heart Rhythm 2014;11:230-6. [Crossref] [PubMed]
- Indik JH, Conover Z, McGovern M, et al. Association of amplitude spectral area of the ventricular fibrillation waveform with survival of out-of-hospital ventricular fibrillation cardiac arrest. J Am Coll Cardiol 2014;64:1362-9. [Crossref] [PubMed]
- Gundersen K, Kvaløy JT, Kramer-Johansen J, et al. Development of the probability of return of spontaneous circulation in intervals without chest compressions during out-of-hospital cardiac arrest: an observational study. BMC Med 2009;7:6. [Crossref] [PubMed]
- Affatato R, Li Y, Ristagno G. See through ECG technology during cardiopulmonary resuscitation to analyze rhythm and predict defibrillation outcome. Curr Opin Crit Care 2016;22:199-205. [Crossref] [PubMed]
- Neurauter A, Eftestøl T, Kramer-Johansen J, et al. Improving countershock success prediction during cardiopulmonary resuscitation using ventricular fibrillation features from higher ECG frequency bands. Resuscitation 2008;79:453-9. [Crossref] [PubMed]
- Li Y, Bisera J, Geheb F, et al. Identifying potentially shockable rhythms without interrupting cardiopulmonary resuscitation. Crit Care Med 2008;36:198-203. [Crossref] [PubMed]
- Aramendi E, Irusta U, Ayala U, et al. Filtering mechanical chest compression artefacts from out-of-hospital cardiac arrest data. Resuscitation 2016;98:41-7. [Crossref] [PubMed]
- Irusta U, Ruiz J, de Gauna SR, et al. A least mean-square filter for the estimation of the cardiopulmonary resuscitation artifact based on the frequency of the compressions. IEEE Trans Biomed Eng 2009;56:1052-62. [Crossref] [PubMed]
- Ruiz de Gauna S, Ruiz J, Irusta U, et al. A method to remove CPR artefacts from human ECG using only the recorded ECG. Resuscitation 2008;76:271-8. [Crossref] [PubMed]
- Gong Y, Gao P, Wei L, et al. An enhanced adaptive filtering method for suppressing cardiopulmonary resuscitation artifact. IEEE Trans Biomed Eng 2017;64:471-8. [Crossref] [PubMed]
- Azeli Y, Herrero J, Fortuny G, et al. Variation and correlation of end-tidal CO2 and amplitude spectrum area in a refractory ventricular fibrillation. A case from the ReCaPTa study. Resuscitation 2018;122:e19-20. [Crossref] [PubMed]
- Fitzgibbon E, Berger R, Tsitlik J, et al. Determination of the noise source in the electrocardiogram during cardiopulmonary resuscitation. Crit Care Med 2002;30:S148-53. [Crossref] [PubMed]
- Ruiz de Gauna S, Irusta U, Ruiz J, et al. Rhythm analysis during cardiopulmonary resuscitation: past, present, and future. Biomed Res Int 2014;2014:386010 [Crossref] [PubMed]
- Coult J, Kwok H, Sherman L, et al. Ventricular fibrillation waveform measures combined with prior shock outcome predict defibrillation success during cardiopulmonary resuscitation. J Electrocardiol 2018;51:99-106. [Crossref] [PubMed]
- Povoas HP, Weil MH, Tang W, et al. Predicting the success of defbrillation by electrocardiographic analysis. Resuscitation 2002;53:77-82. [Crossref] [PubMed]
- Strohmenger HU, Lindner KH, Brown CG. Analysis of the ventricular fibrillation ECG signal amplitude and frequency parameters as predictors of countershock success in humans. Chest 1997;111:584-9. [Crossref] [PubMed]
- Langhelle A, Eftestøl T, Myklebust H, et al. Reducing CPR artefacts in ventricular fibrillation in vitro. Resuscitation 2001;48:279-91. [Crossref] [PubMed]
- Gong Y, Chen B, Li Y. A review of the performance of artifact filtering algorithms for cardiopulmonary resuscitation. J Healthc Eng 2013;4:185-202. [Crossref] [PubMed]
- Amann A, Klotz A, Niederklapfer T, et al. Reduction of CPR artifacts in the ventricular fibrillation ECG by coherent line removal. Biomed Eng Online 2010;9:2. [Crossref] [PubMed]
- Lo MT, Lin LY, Hsieh WH, et al. A new method to estimate the amplitude spectrum analysis of ventricular fibrillation during cardiopulmonary resuscitation. Resuscitation 2013;84:1505-11. [Crossref] [PubMed]
- Li Y, Bisera J, Weil MH, et al. An algorithm used for ventricular fibrillation detection without interrupting chest compression. IEEE Trans Biomed Eng 2012;59:78-86. [Crossref] [PubMed]
(English Language Editors: J. Jones and J. Gray)